ABSTRACT
Over the past 25 years, the study of driving forces of landscape change has developed into a central theme in land change science by contributing to theory development, promoting the analysis of causation of change and gaining insights into how landscape development could be steered into a societally more desirable direction. Based on this progress, we designate important research avenues, reviewing critical challenges forming the base for advancing the study of driving forces of landscape change and addressing the question on how the study of driving forces can contribute to system transformative research. For each of the research avenues, we describe the current dominant approach and provide some specific ways of advancing both the conceptualization and the research methods. Together, advancing on these research avenues will promote a more social-ecological systems perspective to the study of driving forces of landscape change.
1. Introduction
Landscapes are manifestations of the interplay of natural processes and cultural practices, such as land use and social norms, over time (Plieninger et al., Citation2015). Over the past decades, landscapes have become increasingly recognized as central arenas for sustainable development, for example, in the European Landscape Convention (ELC, Citation2000). This trend reflects the fact that many environmental and sustainability challenges have been assigned to land-use practices surpassing local ecological thresholds or resulting in unintended negative side effects. Also, the increasing attention gained by ‘landscape approaches’ (Arts et al., Citation2017; Reed et al., Citation2016; Sayer et al., Citation2013) in land system science can be seen as an expression of the relevance landscapes have gained as an access point for the analysis of sustainability issues. Insights into how land-use practices shape landscapes is therefore highly relevant. There is a great diversity of definitions and uses of the term ‘landscape’ – a diversity, also well reflected in the variety of landscape perspectives and approaches (Arts et al., Citation2017). Whereas within empirical projects, the exact meaning of the term certainly has to be specified, conceptual considerations allow for greater openness in this regard. The study of driving forces of landscape changes has its roots in geography, where already in 1969, Eugen Wirth proclaimed that geography of culture should ‘show regularities and laws in the building-plan of the cultural landscape’, and he distinguished between economic (e.g. costs of transport), social (e.g. norms, traditions), and public (e.g. policy, planning) forces, which are ‘united in a common sphere of activity’ (Wirth, Citation1969). Bürgi et al. (Citation2004) suggested addressing the spatial, temporal and institutional scales and characteristics of socioeconomic, political, technological, natural and cultural driving forces. During the last decades, analyses of driving forces of landscape change became widespread and advanced, supported by the rapidly increasing data availability and related processing technology (Antrop, Citation2005; Geist & Lambin, Citation2002). A broad collection of empirical studies on land change drivers allowed the performance of detailed meta-analyses (Munteanu et al., Citation2014; Plieninger et al., Citation2016; van Vliet et al., Citation2016), trying to operationalize what Wirth had been demanding 50 years ago, i.e. identifying a general pattern in land-use change processes. Notwithstanding this progress, there is still a need to advance research on driving forces, specifically on the two dimensions a) to better understand and address the multiple dimensions of landscape change rather than the focus on land-use and land-cover change processes and b) the use of knowledge of past and current driving forces and relevant actors for informing land-use decisions about the future and therefore contributing to transformative change toward sustainability.
Studies claiming to evaluate the drivers of landscape change (Bürgi et al., Citation2017) are often limited in their analyses on the two dimensions of land use and land cover (Lambin & Geist, Citation2006; LULC). Whereas these dimensions certainly encompass the core aspect of landscape change, they do not include the multitude of dimensions covered in a more encompassing definition of landscapes. Moving from analyzing changes in land use and land cover to analyzing landscape changes, requires addressing a series of additional dimensions, depending on the definition of landscape applied. The European Landscape Convention (ELC, Citation2000) defines landscape as ‘ … an area, as perceived by people … ’, and consequently as something that is created in our minds based on our individual perceptions. Pinto-Correia and Kristensen (Citation2013) on the other hand conceptualize landscape ‘as the arena where structural features and social constructions converge’, a proposal which certainly can only be followed by considering aspects such as decision-making processes, actor constellation, and landscape perception, to name just a few.
Given the challenges of ongoing and expected global change, and the resulting urgency for addressing the pressing sustainability challenges, the question arises how the study of driving forces can support the guidance of development into a societally desirable direction. Much of the analysis done and insights gained on the drivers of landscape change are foremost useful for understanding past and current drivers. However, much less is known on the question of how then driving forces research can inform future changes. As future transformations of landscapes may not have historical analogues, a prerequisite for such a translation of insights from the past into lessons for the future has to be based on a thorough analysis of contextual developments over time.
In this paper, we have selected eight research avenues related to the two dimensions mentioned above (multiple dimensions of landscape change, and inferring future landscape transformations based on better understanding the drivers of landscape change), that are deemed, based on literature review and expert judgement, critical in further advancing the study of driving forces of landscape change. The research avenues are selected from the perspective that the importance of such studies goes beyond a purely scientific one. Rather, it is precisely the context of transformative change in the context of sustainability challenges in which they find their relevance. Therefore, we reflect at the end of this paper on how the defined research avenues of driving forces can contribute to understand, envision and steer the system transformative changes.
2. Research avenues and challenges
In the following, we present eight research avenues relevant for the study of driving forces, actors, and landscape change (Hersperger et al., Citation2010) (). The avenues were drafted in a workshop held at the Swiss Federal Research Insitute WSL in Birmensdorf, Switzerland in February 2021 and subsequently further refined and sharpened based on literature reviews and intensive exchange between the workshop participants. The participants included at the workshop represent a diversity in scientific backgrounds and expertises, but given the diversity in definitions of the core terms and concepts used in this field of research, we certainly do not claim the list to be exhaustive.
Figure 1. The eight research avenues relevant for the study of driving forces, actors and landscape change (Hersperger et al., Citation2010) presented can be grouped into four groups, related to actors and decision making (1, 2), representation and evaluation (3–5), conceptualization (6) and applicability (7, 8).
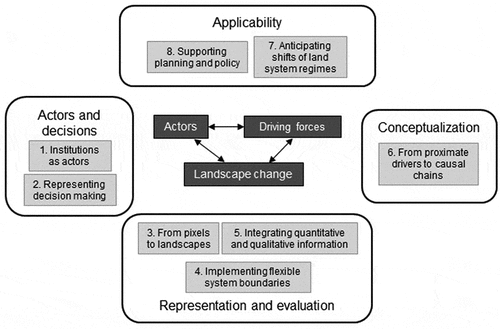
Avenues 1 and 2 focus on how actors and their role in landscape changes are assessed. Avenues 3 to 5 address the way landscape changes are represented and evaluated, and avenue 6 looks into the way driving forces are conceptualized. The remaining two research avenues relate to the use of findings of studies of driving forces. Avenue 7 looks into the challenges posed by regime shifts, which limit the predictability of system changes. Avenue 8 finally addresses an important field of applications of such studies. With this set of avenues, we intend to sketch the state and direction of the field and also provide considerations on the main challenges and how they might be overcome.
2.1. Research avenue 1: conceptualizing the role of institutions as actors
We conceptualize institutions here as organizations that play a formal role in land use, especially government entities, such as the department of agriculture or spatial planning, as well as organized and broadly acknowledged groups, such as farmers organizations and political parties, that are important actors in land change. Institutions can affect land use changes through the development of policies, policy implementation, information, education, and empowerment and the management of their natural resources, among others. So far little research has focused on institutions as actors, while increasingly studies document that institutional settings and policies influence landscape change profoundly, e.g. in the context of agricultural abandonment in post-soviet Eastern Europe (Prishchepov et al., Citation2021). Also, in meta-analysis of case studies on driving forces, the role of institutions and policies came out as important (van Vliet et al., Citation2015).
However, much remains unknown, e.g. how institutions act and interact with other actors in actor networks during policy making and implementation processes, or how institutions interpret their roles and make their decisions that ultimately affect land use. This is partly due to underdeveloped theoretical understanding of the role of institutions in land change (Hersperger et al., Citation2018) but also by the fact that the role of institutions is studied often in isolation as a topic in itself, in the form of an institutional analysis (O’Brien et al., Citation2020; Schomers et al., Citation2021).
A crucial aspect for pursuing avenue 1 will be to understand public policy and planning processes as an ongoing activity, guided by institutions and dominated by negotiation and conflict resolution, among a diversity of public and private actors under ever-changing multi-scalar power relation and funding regimes. These processes tend to take place as successive limited comparisons, as described by Lindblom (Citation1959), rather than following a rational comprehensive procedure. Promising for progress in Avenue 1 are studies that focus on how institutions interact with other actors and form coalition of actors to develop policies, (e.g. Hersperger et al. Citation2014), assess the role and importance of actors in governance and related land use decisions (e.g. Ariti et al. Citation2019, Hauck et al. Citation2016) and address pressing issues such as how institutional actors enable or even engage in illicit activities (Tellman et al., Citation2020) or exploit the margins of discretions inherent in many planning instruments. These activities may be critical in explaining the gap between institutional visions and policy ambitions, and the resulting impacts of these on the landscape.
To pursue avenue 1 and adequately conceptualize the role of institutions as actors, interdisciplinary researchers on driving forces and landscape change can benefit from engaging with the disciplinary traditions of political sciences, spatial, urban and conservation planning, and from adapting theories and applying methods from these disciplines. Especially promising are social network analysis (Cvetinovic et al., Citation2017; Hauck et al., Citation2016), interdisciplinary institutional analysis, grounded theory approaches (Coral et al., Citation2021) and the use of agent-based modelling (Holzhauer et al., 2019). Despite limited spatial coverage, agent-based models allow to better understand the roles of land-use decision-making agents by mimicking institutions and decision-makers interactions and by representing essential components of the institutional processes that are exerting influence on land use.
2.2. Research avenue 2: representing actors’ decision making in the analysis and simulation of driving forces
The importance of decision-making processes of specific actors for shaping the landscapes has long been recognized, and the literature contains many case studies describing the role of land-use decisions. However, a recent systematic review of decision making in land-use change case studies (Malek et al., Citation2019) indicated that the mechanisms of decision making by land-use change actors are often not documented in a consistent manner that allows straightforward synthesis and comparison across case studies. The lack of explicit description of the way actor decision making affects land-use change has repercussions on the way this driving force is represented in land-use models. In land-use models, decision making is implicitly assumed (like in economic models) or explicitly represented (like in agent-based models). However, in both ways, a strong empirical base is often missed and either decision making is based on strong assumptions or economic theory, or on case-study-based heuristic rules derived from local surveys or interviews (An, Citation2012; Muller-Hansen et al., Citation2017; Schlüter et al., Citation2017). Studies on decision-making modelling often focus on a single group of actors, ignoring the complex interrelationship within the relevant system and ignoring the drivers at different levels of the system. Consequently, decision making is described at the level of the farmer or land manager and not representing processes at different spatial and institutional scales. While it is possible to document multi-level governance and decision making for land use change processes, as shown e.g. by Piketty et al. (Citation2015), there is still a strong focus on case studies on the decision making of the primary actor and the dominant land-use change process only. This approach ignores that primary actor decision making is strongly impacted by other decision makers, such as distant land owners, farmer organizations, government institutions and companies (Debonne et al., Citation2021; van der Ploeg, Citation2021). Moreover, the same actor type may have different relations to land change and behavioral aspects and the decision making might differ accordingly in space and time, as found by systematic analyses for specific land use processes, quantifying the role of different behavioral factors and the variations in decision making (e.g. Jones-Garcia & Krishna, Citation2021). Variations in actor behavior have also been explicitly addressed in multi-agent models by differentiating between the actor abilities and its personal ambitions and attitude (Groeneveld et al., Citation2017). In most cases, such attitudes are assumed to be a stable characteristic of an actor. However, the personal ambitions and attitudes can be strongly influenced by personal circumstances, higher level institutions, or the social context in which they operate. It is often unknown if changes in decision making in time originate from a change in contextual factors, or are a real change in the attitudes and decision-making priorities. Also, with time the agency of different actors may change, for example, through the increased influence of global value chain actors (Debonne et al., Citation2021).
In an attempt to quantify the diversity in land-use decision making taking stock of the many available case studies, Malek et al. (Citation2019) conducted a meta-analysis describing decision making in land-use processes. The results indicate that different types of decision making can be distinguished that are often strongly linked to the contextual conditions in which the actors operate. While such analyses help to generalize on the role that diversity in decision making may have, they are semi-quantitative at best and not directly suitable to quantify the impacts that alternative policies or changes in contextual conditions may have on decision making. Similar considerations hold for theories on decision making. While providing very useful frameworks for depicting decision making, they often lack quantification or even the option for formalization and operationalization toward more quantitative assessment or modelling (Schlüter et al., Citation2017). Where operationalization is possible, there is often a large variation in the actual formalization of the theory, leading to a lack of transparency and uncertainty (Muelder & Filatova, Citation2018). New approaches are to be developed to better quantify behaviors and link theoretical foundations towards empirical data and model algorithms. Such approaches start with new and more standardized methods to address decision making in case studies, acknowledging the actor networks and variations between actors. Methods developed in environmental psychology or behavioral economy, such as choice experiments (Bateman et al., Citation2009), can be adopted to help quantification of currently mostly descriptive approaches. Such progress would allow studies of driving forces of land use change to explicitly address decision making in quantitative assessments and, therefore, provide insight in possible contextual or behavioral factors that lead to changed decision making. This way, interventions, policies and incentives can be better targeted recognizing the multi-level structure of decision making, the variations between actors of decision making, and the ways in which decision making is likely to respond to changes.
2.3. Research avenue 3: from pixels to landscapes
Studies of driving forces of landscape change relate the supposed driving factors to observed landscape changes. The way landscape is represented is essential for the analysis and will impact the outcomes and interpretation. Assessing landscapes and analyzing landscape changes requires reducing the complexity of landscapes to the degree of information that can be methodologically handled. Whereas studies analyzing landscape change quite often work with categorized representations of landscapes as provided in topographical maps (Bürgi et al., Citation2017; Fuchs et al., Citation2015; Kienast, Citation1993) or aerial photographs (Gerard et al., Citation2010), most quantitative approaches are based on reduction of the landscape to homogenous units of observation, often pixels of land use or land cover. Landscape change is measured by the aggregated change of individual pixels. Even in approaches that focus on individual actors (i.e. agent-based modeling), the challenge remains to link these decisions to landscape-level change. Reducing landscapes to pixels affects the potential to analyze landscape features not directly showing up in pixels, such as habitat structure or aesthetic quality of landscapes, topics of high ecological and societal relevance. On the other hand, transforming landscapes into pixels bears the potential to systematically consider their spatial context, such as neighborhood effects, and allows structured multi-scale analysis.
Novel techniques of image analysis, such as processing of ‘big’ environmental, social and remote-sensing data, advancement in remote sensing, machine learning, including Convolutional Neural Networks (Y. Liu et al., Citation2018), allow recognizing the spatial object of interest rather than single pixels. At the same time, more detailed spatial and temporal resolution, as well as free-access data availability on the landscape representation allow to switch from traditional point-in-time mapping and several time-steps, change detection to continuous monitoring of changes at any point in the Earth observation record (Woodcock et al., Citation2020). Both approaches open up new possibilities for analyzing the driving forces and for an improved understanding of the interactions and feedbacks between land-use decisions leading to changes in land management intensity across landscapes with various landscape compositions. Further topics that will profit from these new analytical possibilities are responses of biodiversity to changing landscapes (e.g. insect communities and links to blue-green infrastructure) and provision of ecosystem services of current and planned landscapes (e.g. pest control, pollination, carbon sequestration, landscape esthetics; Karasov et al., Citation2020).
2.4. Research avenue 4: implementing flexible system boundaries
Studies of driving forces of landscape change face the challenge to acknowledge the complexity of interactions and linkages to different temporal, spatial and institutional scales, as well as to address teleconnections, telecouplings and spill-over effects (Friis et al., Citation2016), without getting lost in this complexity, i.e. to restrict the analysis to the relevant factors. Interventions on land use often lead to leakage and displacement of production (Meyfroidt et al., Citation2013): a transition to sustainable management at one site may lead to a shift of production for the same goods to other locations. However, our understanding of driving factors are often constraint to local drivers, whereas drivers from outside of the case study area might not receive appropriate atten
To assess potential impacts in terms of displacement effects, spillovers and feedbacks, we need to better understand and identify flows of commodities and ecosystem services, the institutions and networks of actors operating along their supply chains and the instruments deployed to govern them (Munroe et al., Citation2019). Consequently, the geographical area in which effects are to be expected from the processes of interest can no longer be assumed to be necessarily continuous, and territorial-based system boundaries (e.g. landscapes, governance units) have to be combined with network-based ones (e.g. material and financial flows of commodities and services, actors’ relationships and interactions). Designing studies in such a way that geographical spill-over effects due to e.g. teleconnections can be analyzed in a system of interlinked case study areas, will enhance the relevance of driving forces analyses in the context of sustainability challenges (e.g. corporate responsibilities, effects of changes in consumer behavior, policies), but at the same time requires thorough, open-ended ex-ante assessments regarding the expected and interesting effects. An example of such an approach is presented by Dou et al. (Citation2020). These authors study interconnected dynamics in two case study areas across the world in connection, by focusing on the process of agricultural trade as a mutual driving force causing impacts of changing in one case study region on another case study region. While the conceptual challenges of discontinuous spatial interactions have been well addressed in the telecoupling literature (Friis & Nielson, Citation2019; J. Liu et al., Citation2013), there are many methodological challenges for quantifying the role of such distant drivers, especially in the ways of dealing with dynamic feedbacks and distinguishing where spatial explicit representation is required and where not (Dou et al., Citation2019). Telecoupling not only relates to value chains and trade of land use commodities but also relates to large-distance governance of land use. In particular, accounting for the increasing influence of distant land-owners and investors through large-scale land acquisitions and contract farming should receive special attention and requires mapping of actor-networks beyond a single landscape or region (Verburg et al., Citation2019). Where land managers are not the prime decision makers, but absentee land owners located in distant cities determine the most important land use decisions (Zelaya et al., Citation2016), it is no longer sufficient to interview land managers on the site or understand the farm characteristics. Social network mapping should, in land use studies, therefore use flexible system boundaries to better understand the ways in which distant drivers affect land use in case studies.
2.5. Research avenue 5: integrating quantitative and qualitative information
The current literature can be classified in approaches focusing on either quantitative or qualitative information on drivers. Quantitative approaches focus on those drivers that can be integrated in statistical analysis or quantitative modelling. Given that quantitative information on potential driving forces is only available for specific drivers, either from biophysical measurements, surveys or socio-economic statistics, the resulting analysis is foremost data-driven and may ignore drivers that are not easily quantifiable or are not included in common data collection efforts. A complete understanding of driving forces of landscape change requires the integration of qualitative information. One way of including qualitative information is through coding from documents or interviews, which is very time-consuming and requires categorizations based on ex-ante knowledge as a theoretical or an inductive thematic analysis (Braun & Clarke, Citation2006). Narrative approaches allow to consider and integrate a higher degree of complexity of, e.g. the decision-making process or cultural factors (Lambin et al., Citation2003; Young et al., Citation2006). However, narratives often do not provide quantitative information, which is needed to understand the respective role of these drivers as opposed to other drivers and to be able to assess and possibly model land-use change based on these drivers. To date, established standards of how to integrate information from qualitative studies into more quantitative driving forces analysis, are lacking.
Recent advances in text analysis with artificial intelligence are expected to foster a big step forward in efficiently coding qualitative information from documents and interviews. For example, self-organizing maps applied to consultation reports have been used to analyze policy integration (Hossu et al., Citation2017). Furthermore, approaches are increasingly developed to tackle the integration of qualitative and quantitative data. A prototypical case of this challenge is found in participatory modelling exercises (Mallampalli et al., Citation2016). Modelers aim to quantify participants’ mental models and need to translate fuzzy arguments into numbers, for instance, by using Bayesian networks (Celio & Grêt-Regamey, Citation2016; Marcot & Penman, Citation2019). Co-developing Bayesian networks with local experts and stakeholders allows to include information that is otherwise hard to grasp, while the Bayesian approach helps to quantify that in information in conjunction to other conditions (Prishchepov et al., Citation2021). In general, interviews can help to setup modelling frameworks, test underlying assumptions and interactions of different statistical data, but also local experts and stakeholders should be included for reviewing modelling results (Voinov & Bousquet, Citation2010). Others have tried participatory games as a means to uncover decision-making strategies that are otherwise hard to quantify (Ornetsmüller et al., Citation2018), allowing the translation of these games into more formal conceptualization of the way driving factors operate, or possibly, the representation of these in simulation models (Celio et al., Citation2019; Souchère et al., Citation2010). A specific method that helps to uncover more qualitative drivers is Q-methodology. Researchers using this approach found hidden views, understood opinions in depth and discovered points of consensus that facilitated unlocking difficult disagreements across a range of environmental and sustainability issues (Sneegas et al., Citation2021). Within landscape studies the approach is less frequently used, although there are examples related to conservation issues (Zabala et al., Citation2018) and the drivers of deforestation (Huaranca et al., Citation2019). The above methods all can contribute to include a wider range of driving forces while, at the same time, avoiding that important driving forces are omitted from more quantitative driving forces analysis.
2.6. Research avenue 6: from proximate/underlying drivers to causal chains
Much of the work on driving factors in land use studies is based on the original conceptualization that distinguishes between proximate causes/drivers and underlying driving forces (Geist & Lambin, Citation2002), a wording originally proposed for the study of land-cover changes. Changes in land use, resulting in changes in land cover, are consequently termed proximate causes or proximate drivers. However, in studies focusing on understanding changes in land use, it makes no sense to also refer to land use as proximate drivers of land cover, as land use is the main outcome of interest rather than land cover. Therefore, new ways have to be found to conceptualize the nested, hierarchical structure of interlinked and scaled drivers.
Empirical studies on driving forces of landscape change, inevitably lead to the insight, that drivers are interlinked, scaled and show various, context-specific strengths. Various approaches have been developed to address the causal complexity driving landscape changes, e.g. landscape biographies (Kolen & Renes, Citation2015), nested chains of explanations and causal eventism (Walters, Citation2017), Landscape Change Trajectory Analyses (LCTAs; Käyhkö & Skånes, Citation2008) or path dependence (Tappeiner et al., Citation2020; Zarina, Citation2013). Especially promising to expand the study of driving forces is the concept of causal chains (Efroymson et al., Citation2016; Loran et al., Citation2018), which are similar to the ‘nested chains of explanation’ proposed in Walters (Citation2017). Based on the causal chain structure, we can employ various qualitative and quantitative methods, for different parts of the chain, and in sum a more encompassing depiction of how interrelated drivers are causing the changes under study, can be reached. Such an approach moves beyond a simple classification of drivers as underlying or proximate towards describing and unpacking the causalities. In doing this, we suggest to adopt the distinction between causal effects and causal mechanisms (Meyfroidt, Citation2016), as the links in the chain will certainly be of very different causal strength, including statistical evidence, i.e. correlation, as well as links based on insights into the underlying mechanisms. While meta-analyses of driving forces studies have shown the co-occurrence of different drivers (Plieninger et al., Citation2016), the analysis of causal chains, including causal effects and causal mechanisms will help to understand if these are the result of correlations in the spatial and temporal distribution of these drivers, or a result of interactions between these drivers that together provide a different impact on land use than in isolation. At the same time, given the unstable and contingent dynamics between people and the environment, Walters and Vayda (Citation2020) recommend for research in human ecology ‘the more general, serviceable concept of cause’ or causal histories, instead of searching for causal mechanisms, thereby, rightfully, nuancing the ambition to uncover causal mechanisms.
2.7. Research avenue 7: anticipating shifts of land system regimes
Responses to driving forces can be assessed based on historic and current evidence and are, therefore, relatively predictable under a given land system regime (Müller et al., Citation2014; Ramankutty & Coomes, Citation2016). Real-world examples of shifts to alternate states where the characteristics and the dynamics of the system fundamentally differ from the previous state, indicate that the predictive power of the driving forces of landscape change is reduced significantly under those conditions (Müller et al., Citation2014). With regime shifts we refer to a shift from one dominant land-use regime to another, wherein the enduring land-use dynamics change, often, but not always, accompanied by rapid land-cover change (Ramankutty & Coomes, Citation2016). Such regime shifts may mean that the relations between the drivers and land use/landscape outcomes changes fundamentally, often related to a reconfiguration of the socio-ecological system. Ramankutty and Coomes (Citation2016) called for more attention to the study of drivers of land-use regime shifts to anticipate future land-use changes or to enhance identification of system vulnerabilities to regime shifts. Given the limits to predictability, it is often impossible to predict when such shifts to a new land system regime might happen (Jepsen et al., Citation2015), but the conditions that favor such system transformation and associated leverage points may be identified and included in future outlooks. Moreover, the spatial and temporal scale of the phenomenon under study is crucial: what extent does a transformation have to cover and how rapidly does it need to happen, to be called a regime shift? While this refers to the system boundaries (avenue 4), it also relates to a better understanding of the causal chains that drive land use change (avenue 7).
To operationalize this, we propose to distinguish between driving forces of land-use change within a specific land-use regime (with a rather continuous relation between drivers and land use outcomes, and in this sense predictable impact), and the driving forces of the shifts in the land-use regime itself, which can be caused by drivers surpassing a critical threshold or by new drivers not formerly part of the system (e.g. new incoming land owners, or a change in behavior of the decision makers driven by changing environmental cognition). The predictability of events is highly variable, ranging from very predictable, such as the release of a new legislation in response to a disaster, restricting e.g. a certain type of land use, and small scale, such as transitional ruptures on the farm level as described by Wilson (Citation2008), to the events with low prediction power and greater uncertainty, such as large-scale earthquakes, technogenic catastrophes, wars or pandemics. In-between, events such as development and dissemination of new technologies, but also foreign direct investments (Ceddia, Citation2020), economic crisis, changing global demands on certain products (Müller et al., Citation2014), outbreaks of crop-diseases, etc., with potentially far-reaching consequences on land system regimes, can be grouped along a continuous gradient of predictability, but also along a gradient of spatial scale, representing the spatial extent affected. Classifying regime-shifting events and drivers and systematically assessing the specific context would allow for an improved understanding of preconditions, triggers, and self-reinforcing processes of change and feedbacks as asked for by Ramankutty and Coomes (Citation2016). Such an approach requires a strong documentation of individual cases of regime shifts. To document and identify those not only a focus should be on the landscape patterns that are the result of these shifts, but also on identifying the underlying processes. Examples of methods used to document such processes leading to regime shifts include those on boom crops (Zaehringer et al., Citation2020) that both use participatory approaches; transformations in livestock systems through re-constructing narratives (Ornetsmüller et al., Citation2018) and the role of remittances in avoiding a regime shift in forest dynamics (Ospina et al., Citation2019) using a modelling approach. These examples provide evidence that existing methods are able to better investigate such issues and build up a body of evidence of the way in which such regime shifts are driven or avoided.
2.8. Research avenue 8: supporting planning and policy
Many driving forces studies examine landscape changes that are perceived as problematic, such as urban sprawl, deforestation and the disappearance of traditional cultural landscapes. It is therefore understandable that the driving forces research community sees great potential in its research findings for combating unsustainable landscape change and supporting planning and policy in general. However, there is surprisingly little evidence about how the information generated by driving forces research (as discussed in this manuscript) assists contemporary practice in planning and policy-making and implementation. Empirically and conceptually, it is extremely difficult to identify which knowledge plays which role in the planning and policy process and in the corresponding decisions. This is especially true for the many cases in which this process unfolds as a political negotiation process or as design practice. It is easier when mathematical optimizations are shaping the modus operandi, as is often the case in conservation planning where design-based approaches play a major role, but tend to ignore the drivers that enable or constrain the actual changes required (O’Connor et al., Citation2021). To trace the role of knowledge in the various planning activities and steps, ethnographic studies are probably needed, in which researchers immerse themselves in everyday planning and closely observe the planning process (e.g. Majoor Citation2018).
It is very important for the pursuit of Avenue 8 that planning and policy making are better informed on the landscape changes, the driving forces as well as the actors on all relevant spatial scales. Accounting for the right drivers and leverage points in planning and policy making helps finding those policies/interventions that steer in the right direction, avoiding ineffective policies, unwanted side effects and even illicit actions. The data needs not only be generated by research, but also be easily accessible to all those involved in planning and policy (open data). Many institutions are building geoportals for environmental and planning data that seem an appropriate venue for making data on land change and its causes accessible (e.g. the Copernicus initiative (http://land.copernicus.eu and http://opengeohub.org)). It will support the system analysis of those involved in the process and a solid system analysis is essential for the development of goals and measures, regardless of the modus operandi.
Scenario studies, either narrative or modelling, participatory or expert driven, are a further way in which driving forces research is able to enter planning. Scenario studies either sketch how the drivers may create different futures and highlight options for action (exploratory or descriptive scenario) or start with a desired future situation and work backward to identify policy options that will be needed to attain the vision (backcasting or anticipatory scenario; Goodspeed, Citation2020). Important in this regard will be to anticipate future problems and account for how drivers might change within the planning and policy horizon, helping to make the planning of interventions more resilient to future change rather than only responding to current-day problems. Driving forces studies can also be useful if conducted as ex-ante assessments, evaluating how, in the context of drivers, potential policies might work out.
A way forward to effectively pursue avenue 8 long term will be to develop methods to understand the roles and influence of different knowledge in the planning and policy process. Such research may provide insights into the leverage points of better adopting data and insights of landscape driving factor studies into operational planning and policy.
3. Conclusions and outlook
The eight research avenues address the different dimensions of the analyses that landscape science needs to progress scientifically and to enable it to effectively contribute to overcoming the sustainability crisis. Some of the avenues relate to technological and methodological advancements, that allow more and different types of analysis than in the preceding decade. Technological developments allow for much more detailed, large-scale analyses of landscape change based on high-resolution multisource remote sensing while interdisciplinary science provides examples of approaches for no longer limiting this analysis to just the aspects that can be quantified, and incorporating a broader range of drivers into a consistent analysis framework. The new technologies also allow to address societally and ecologically highly relevant questions on different scales, increasing however at the same time pressure to find adequate ways for including decision making processes in the analysis, and to deal with the diversity of relevant drivers. In a globalized world, landscapes can no longer be studied in isolation, but as elements in a global network of interconnected places. The definition of system boundaries has thus become more demanding. Case-studies, therefore, no longer have fixed geographic boundaries and the drivers and feedbacks that extent beyond the geographic case-study extent cannot simply be treated as exogenous to the case-study.
While the methodological and technical advances are helping to conduct more detailed analysis, they also require new ways of data analysis. Advancing such analysis, at the same time, necessitates an advancement of our conceptual understanding of several aspects related to driving forces, such as behavioral change and the role of institutions. Implementation of these avenues of advancement not only requires methodological and theoretical skills but also bridging the different disciplines that are core to these topics. Separate studies, using different methods, of the institutional and environmental driving forces do not provide the full picture: it is the interaction between the different drivers that is essential for understanding the system behavior. Only by stepping beyond the traditional approaches of these disciplines, real integrated knowledge will be achieved that helps to better represent the different types of drivers in a consistent way. Simply adding expertise from different disciplines to research teams addressing these issues is not sufficient, it also requires the analytical and social skills to bring the different perspectives and methods together in a more holistic understanding of the drivers, and operationalize this toward planning and policy. These are typically inter- and transdisciplinary skills that require further development.
It is broadly acknowledged that landscape and land management have huge potential for tackling some of the most urgent sustainability problems: both in providing climate mitigation and adaptation options as well as in the context of biodiversity conservation and environmental justice. To address the sustainability challenges, transformative change is needed, resulting in novel land systems and landscapes, differing greatly from situations in recent history for which the drivers are studied. This only makes more obvious what was already clear otherwise, namely that it is not possible to simply learn from the past. However, the study of driving forces and actors of the past may provide insights into which drivers are leverage points for systemic change and which lead to persistence in a land system (desirable or not; Oliver et al. Citation2018), or a process of continuation of ongoing gradual change and illustrate the multitude of actors and actor constellations have been involved in transformative landscape change, be it initiated by planning and policy, or e.g. by bottom-up initiatives. Analysis of past changes can provide insights in the processes and time frames of land system transitions, and therefore help detect leverage points and early signals of structural change, or lock-in. To be able to do this, the analysis of drivers has to move from cause-effect relations to analysis of systemic change. Informing future sustainable landscapes, therefore, requires also transformation of the ways in which we analyze driving forces. The avenues described in this paper provide entry points and inspiration of moving this type of analysis further. The perfect approach for driving factors analysis in landscape change does not exist, but the avenues sketched in this paper provide a pathway to advance existing analysis and enable driving factor analysis to support the expected and needed transformation of landscapes.
Disclosure statement
No potential conflict of interest was reported by the author(s).
References
- An, L. (2012). Modeling human decisions in coupled human and natural systems: Review of agent-based models. Ecological Modelling, 229, 25–36. https://doi.org/10.1016/j.ecolmodel.2011.07.010
- Antrop, M. (2005). Why landscapes of the past are important for the future. Landscape and Urban Planning, 70(1–2), 21–34. https://doi.org/10.1016/j.landurbplan.2003.10.002
- Ariti, A.T., van Vliet, J., & Verburg, P.H. (2019). The role of institutional actors and their interactions in the land use policy making process in Ethiopia. Journal of Environmental Management, 237, 235–246. https://doi.org/10.1016/j.jenvman.2019.02.059
- Arts, B., Buizer, M., Horlings, L., Ingram, V., Oosten, C.V., & Opdam, P. (2017). Landscape approaches: A state-of-the-art review. Annual Review of Environment and Resources, 42(1), 439–463. https://doi.org/10.1146/annurev-environ-102016-060932
- Bateman, I.J., Day, B.H., Jones, A.P., & Jude, S. (2009). Reducing gain–loss asymmetry: A virtual reality choice experiment valuing land use change. Journal of Environmental Economics and Management, 58(1), 106–118. https://doi.org/10.1016/j.jeem.2008.05.003
- Braun, V., & Clarke, V. (2006). Using thematic analysis in psychology. Qualitative Research in Psychology, 3(2), 77–101. https://doi.org/10.1191/1478088706qp063oa
- Bürgi, M., Bieling, C., Von Hackwitz, K., Kizos, T., Lieskovský, J., Martín, M.G., McCarthy, S., Müller, M., Palang, H., Plieninger, T., & Printsmann, A. (2017). Processes and driving forces in changing cultural landscapes across Europe. Landscape Ecology, 32(11), 2097–2112. https://doi.org/10.1007/s10980-017-0513-z
- Bürgi, M., Hersperger, A.M., & Schneeberger, N. (2004). Driving forces of landscape change - current and new directions. Landscape Ecology, 19(8), 857–868. https://doi.org/10.1007/s10980-004-0245-8
- Ceddia, M.G. (2020). The super-rich and cropland expansion via direct investments in agriculture. Nature Sustainability, 3(4), 312–318. https://doi.org/10.1038/s41893-020-0480-2
- Celio, E., Andriatsitohaina, R.N.N., & Zaehringer, J.G. (2019). A serious game to parameterize Bayesian networks: Validation in a case study in northeastern Madagascar. Environmental Modelling and Software, 122, 104525. https://doi.org/10.1016/j.envsoft.2019.104525
- Celio, E., & Grêt-Regamey, A. (2016). Understanding farmers‘ influence on land-use change using a participatory Bayesian network approach in a pre-Alpine region in Switzerland. Journal of Environmental Planning and Management, 59(11), 2079–2101. https://doi.org/10.1080/09640568.2015.1120713
- Coral, C., Bokelmann, W., Bonatti, M., Carcamo, R., & Sieber, S. (2021). Understanding institutional change mechanisms for land use: Lessons from Ecuador’s history. Land Use Policy, 108, 105530. https://doi.org/10.1016/j.landusepol.2021.105530
- Cvetinovic, M., Nedovic-Budic, Z., & Bolay, J.-C. (2017). Decoding urban development dynamics through actor-network methodological approach. Geoforum, 82, 141–157. https://doi.org/10.1016/j.geoforum.2017.03.010
- Debonne, N., van Vliet, J., Metternicht, G., & Verburg, P. (2021). Agency shifts in agricultural land governance and their implications for land degradation neutrality. Global Environmental Change-Human and Policy Dimensions, 66, 13. https://doi.org/10.1016/j.gloenvcha.2020.102221
- Dou, Y., Millington, J.D.A., Bicudo Da Silva, R.F., McCord, P., Viña, A., Song, Q., Yu, Q., Wu, W., Batistella, M., Moran, E., & Liu, J. (2019). Land-use changes across distant places: Design of a telecoupled agent-based model. Journal of Land Use Science, 14(3), 191–209. https://doi.org/10.1080/1747423X.2019.1687769
- Dou, Y., Yao, G., Herzberger, A., Da Silva, R.F.B., song, Q., Hovis, C., Batistella, M., Moran, E., Wu, W., & Liu, J. (2020). Land-use changes in distant places: Implementation of a telecoupled agent-based model. Journal of Artificial Societies and Social Simulation, 23(1), 11. https://doi.org/10.18564/jasss.4211
- Efroymson, R.A., Kline, K.L., Angelsen, A., Verburg, P.H., Dale, V.H., Langeveld, J.W.A., & McBride, A. (2016). A causal analysis framework for land-use change and the potential role of bioenergy policy. Land Use Policy, 59, 516–527. http://dx.doi.org/10.1016/j.landusepol.2016.09.009
- ELC. (2000). European landscape convention. In European treaty series
- Friis, C., Nielsen, J.Ø., Otero, I., Haberl, H., Niewöhner, J., & Hostert, P. (2016). From teleconnection to telecoupling: Taking stock of an emerging framework in land system science. Journal of Land Use Science, 11(2), 131–153. https://doi.org/10.1080/1747423X.2015.1096423
- Friis, C., & Nielson, J.O. (2019). Telecoupling. Exploring land-use change in a globalised world. In Palgrave studies in natural resource management (Vol. 463). 402. Palgrave Macmillan.
- Fuchs, R., Verburg, P.H., Clevers, J.G.P.W., & Herold, M. (2015). The potential of old maps and encyclopaedias for reconstructing historic European land cover/use change. Applied Geography, 59, 43–55. https://doi.org/10.1016/j.apgeog.2015.02.013
- Geist, H.J., & Lambin, E.F. (2002). Proximate causes and underlying driving forces of tropical deforestation. Bioscience, 52(2), 143–150. h ttps://d oi.o rg/1 0.1641/00 06-35 68(2 002)0 52[01 43:PCAUDF]2.0.CO;2
- Gerard, F., Petit, S., Smith, G., Thomson, A., Brown, N., Manchester, S., Wadsworth, R., Bugar, G., Halada, L., Bezák, P., Boltiziar, M., De Badts, E., Halabuk, A., Mojses, M., Petrovic, F., Gregor, M., Hazeu, G., Mücher, C.A., Wachowicz, M., … Feranec, J. (2010). Land cover change in Europe between 1950 and 2000 determined employing aerial photography. Progress in Physical Geography: Earth and Environment, 34(2), 183–205. https://doi.org/10.1177/0309133309360141
- Goodspeed, R. (2020). Scenario planning for cities and regions: Managing and envisioning uncertain futures. Lincoln Institute of Land Policy.
- Groeneveld, J., Müller, B., Buchmann, C.M., Dressler, G., Guo, C., Hase, N., Hoffmann, F., John, F., Klassert, C., Lauf, T., Liebelt, V., Nolzen, H., Pannicke, N., Schulze, J., Weise, H., & Schwarz, N. (2017). Theoretical foundations of human decision-making in agent-based land use models – A review. Environmental Modelling and Software, 87, 39–48. https://doi.org/10.1016/j.envsoft.2016.10.008
- Hauck, J., Schmidt, J., & Werner, A. (2016). Using social network analysis to identify key stakeholders in agricultural biodiversity governance and related land-use decisions at regional and local level. Ecology and Society, 21(2). https://doi.org/10.5751/ES-08596-210249
- Hersperger, A.M., Gennaio Franscini, M.-P., & Kübler, D. (2014). Actors, decisions and policy changes in local urbanization. European Planning Studies, 22(6), 1301–1319. https://doi.org/10.1080/09654313.2013.783557
- Hersperger, A.M., Gennaio, M.P., Verburg, P.H., & Bürgi, M. (2010). Linking land change with driving forces and actors: Four conceptual models. Ecology and Society, 15(4). https://doi.org/10.5751/ES-03562-150401
- Hersperger, A.M., Oliveira, E., Pagliarin, S., Palka, G., Verburg, P., Bolliger, J., & Grădinaru, S. (2018). Urban land-use change: The role of strategic spatial planning. Global Environmental Change, 51, 32–42. https://doi.org/10.1016/j.gloenvcha.2018.05.001
- Hossu, C.A., Ioja, I.C., Nita, M.R., Hartel, T., Badiu, D.L., & Hersperger, A.M. (2017). Need for a cross-sector approach in protected area management. Land Use Policy, 69, 586–597. https://doi.org/10.1016/j.landusepol.2017.10.012
- Huaranca, L.L., Iribarnegaray, M.A., Albesa, F., Volante, J.N., Brannstrom, C., & Seghezzo, L. (2019). Social perspectives on deforestation, land use change, and economic development in an expanding agricultural frontier in Northern Argentina. Ecological Economics, 165, 106424. https://doi.org/10.1016/j.ecolecon.2019.106424
- Jepsen, M.R., Kuemmerle, T., Müller, D., Erb, K., Verburg, P.H., Haberl, H., Vesterager, J.P., Andrič, M., Antrop, M., Austrheim, G., Björn, I., Bondeau, A., Bürgi, M., Bryson, J., Caspar, G., Cassar, L.F., Conrad, E., Chromý, P., Daugirdas, V., … Reenberg, A. (2015). Transitions in European land-management regimes between 1800 and 2010. Land Use Policy, 49, 53–64. https://doi.org/10.1016/j.landusepol.2015.07.003
- Jones-Garcia, E., & Krishna, V.V. (2021). Farmer adoption of sustainable intensification technologies in the maize systems of the Global South. A review. Agronomy for Sustainable Development, 41(1), 8. https://doi.org/10.1007/s13593-020-00658-9
- Karasov, O., Vieira, A.A.B., Külvik, M., & Chervanyov, I. (2020). Landscape coherence revisited: GIS-based mapping in relation to scenic values and preferences estimated with geolocated social media data. Ecological Indicators, 111, 105973. https://doi.org/10.1016/j.ecolind.2019.105973
- Käyhkö, N., & Skånes, H. (2008). Retrospective land cover/land use change trajectories as drivers behind the local distribution and abundance patterns of oaks in south-western Finland. Landscape and Urban Planning, 88(1), 12–22. https://doi.org/10.1016/j.landurbplan.2008.07.003
- Kienast, F. (1993). Analysis of historic landscape patterns with a Geographical Information System — A methodological outline. Landscape Ecology, 8(2), 103–118. https://doi.org/10.1007/BF00141590
- Kolen, J., & Renes, J. (2015). Landscape biographies: Key issues. In J. Kolen, J. Renes, & R. Hermans (Eds.), Landscape biographies (pp. 21–48). Amsterdam University Press.
- Lambin, E.F., Geist, H.J., & Lepers, E. (2003). Dynamics of land-use and land-cover change in tropical regions. Annual Review of Environment and Resources, 28(1), 205–241. https://doi.org/10.1146/annurev.energy.28.050302.105459
- Lambin, E.F., & Geist, H. (2006). Land-use and land-cover change: Local processes and global impacts. Springer.
- Lindblom, C.E. (1959). The science of “muddling through”. Public Administration Review, 19(2), 79–88. https://doi.org/10.2307/973677
- Liu, J., Hull, V., Batistella, M., DeFries, R., Dietz, T., Fu, F., Hertel, T.W., Izaurralde, R.C., Lambin, E.F., Li, S., Martinelli, L.A., McConnell, W.J., Moran, E.F., Naylor, R., Ouyang, Z., Polenske, K.R., Reenberg, A., de Miranda Rocha, G., Simmons, C.S., … Zhu, C. (2013). Framing sustainability in a telecoupled world. Ecology and Society, 18(2). https://doi.org/10.5751/ES-05873-180226
- Liu, Y., Chen, X., Wang, Z., Wang, Z.J., Ward, R.K., & Wang, X. (2018). Deep learning for pixel-level image fusion: Recent advances and future prospects. Information Fusion, 42, 158–173. https://doi.org/10.1016/j.inffus.2017.10.007
- Loran, C., Kienast, F., & Bürgi, M. (2018). Change and persistence: Exploring the driving forces of long-term forest cover dynamics in the Swiss lowlands. European Journal of Forest Research, 137(5), 693–706. https://doi.org/10.1007/s10342-018-1134-z
- Majoor, S.J.H. (2018). Coping with ambiguity: An urban megaproject ethnography. Progress in Planning, 120, 1–28. https://doi.org/10.1016/j.progress.2016.07.001
- Malek, Ž., Douw, B., Van Vliet, J., Van Der Zanden, E.H., & Verburg, P.H. (2019). Local land-use decision-making in a global context. Environmental Research Letters, 14(8), 083006. https://doi.org/10.1088/1748-9326/ab309e
- Mallampalli, V.R., Mavrommati, G., Thompson, J., Duveneck, M., Meyer, S., Ligmann-Zielinska, A., Druschke, C.G., Hychka, K., Kenney, M.A., Kok, K., & Borsuk, M.E. (2016). Methods for translating narrative scenarios into quantitative assessments of land use change. Environmental Modelling and Software, 82, 7–20. https://doi.org/10.1016/j.envsoft.2016.04.011
- Marcot, B.G., & Penman, T.D. (2019). Advances in Bayesian network modelling: Integration of modelling technologies. Environmental Modelling and Software, 111, 386–393. https://doi.org/10.1016/j.envsoft.2018.09.016
- Meyfroidt, P., Lambin, E.F., Erb, K.-H., & Hertel, T.W. (2013). Globalization of land use: Distant drivers of land change and geographic displacement of land use. Current Opinion in Environmental Sustainability, 5(5), 438–444. https://doi.org/10.1016/j.cosust.2013.04.003
- Meyfroidt, P. (2016). Approaches and terminology for causal analysis in land systems science. Journal of Land Use Science, 11(5), 501–522. https://doi.org/10.1080/1747423X.2015.1117530
- Muelder, H., & Filatova, T. (2018). One theory - many formalizations: Testing different code implementations of the theory of planned behaviour in energy agent-based models. Journal of Artificial Societies and Social Simulation, 21(4), 5. https://doi.org/10.18564/jasss.3855
- Muller-Hansen, F., Schluter, M., Mas, M., Donges, J.F., Kolb, J.J., Thonicke, K., & Heitzig, J. (2017). Towards representing human behavior and decision making in Earth system models - an overview of techniques and approaches. Earth System Dynamics, 8(4), 977–1007. https://doi.org/10.5194/esd-8-977-2017
- Müller, D., Sun, Z.L., Vongvisouk, T., Pflugmacher, D., Xu, J.C., & Mertz, O. (2014). Regime shifts limit the predictability of land-system change. Global Environmental Change-Human and Policy Dimensions, 28, 75–83. https://doi.org/10.1016/j.gloenvcha.2014.06.003
- Munroe, D.K., Batistella, M., Friis, C., Gasparri, N.I., Lambin, E.F., Liu, J., Meyfroidt, P., Moran, E., & Nielsen, J.Ø. (2019). Governing flows in telecoupled land systems. Current Opinion in Environmental Sustainability, 38, 53–59. https://doi.org/10.1016/j.cosust.2019.05.004
- Munteanu, C., Kuemmerle, T., Boltiziar, M., Butsic, V., Gimmi, U., Lúboš, H., Kaim, D., Király, G., Konkoly-Gyuró, É., Kozak, J., Lieskovský, J., Mojses, M., Müller, D., Ostafin, K., Ostapowicz, K., Shandra, O., Štych, P., Walker, S., & Radeloff, V.C. (2014). Forest and agricultural land change in the Carpathian region—A meta-analysis of long-term patterns and drivers of change. Land Use Policy, 38, 685–697. https://doi.org/10.1016/j.landusepol.2014.01.012
- O’Brien, P., Lord, A., & Dembski, S. (2020). How do planners manage risk in alternative land development models? An institutional analysis of land development in the Netherlands. Land Use Policy, 91, 104409. https://doi.org/10.1016/j.landusepol.2019.104409
- O’Connor, L.M.J., Pollock, L.J., Renaud, J., Verhagen, W., Verburg, P.H., Lavorel, S., Maiorano, L., & Thuiller, W. (2021). Balancing conservation priorities for nature and for people in Europe. Science, 372(6544), 856-+. https://doi.org/10.1126/science.abc4896
- Oliver, T.H., Boyd, E., Balcombe, K., Benton, T.G., Bullock, J.M., Donovan, D., Feola, G., Heard, M., Mace, G.M., Mortimer, S.R., Nunes, R.J., Pywell, R.F., & Zaum, D. (2018). Overcoming undesirable resilience in the global food system. Global Sustainability, 1, e9. https://doi.org/10.1017/sus.2018.9
- Ornetsmüller, C., Castella, J.-C., & Verburg, P.H. (2018). A multiscale gaming approach to understand farmer’s decision making in the boom of maize cultivation in Laos. Ecology and Society, 23(2). https://doi.org/10.5751/ES-10104-230235
- Ospina, D., Peterson, G., & Crépin, A.-S. (2019). Migrant remittances can reduce the potential of local forest transitions—a social-ecological regime shift analysis. Environmental Research Letters, 14(2), 024017. https://doi.org/10.1088/1748-9326/aaf0ee
- Piketty, M.-G., Poccard-Chapuis, R., Drigo, I., Coudel, E., Plassin, S., Laurent, F., & Thâles, M. (2015). Multi-level governance of land use changes in the Brazilian Amazon: Lessons from Paragominas, State of Pará. Forests, 6(12), 1516–1536. https://doi.org/10.3390/f6051516
- Pinto-Correia, T., & Kristensen, L. (2013). Linking research to practice: The landscape as the basis for integrating social and ecological perspectives of the rural. Landscape and Urban Planning, 120, 248–256. https://doi.org/10.1016/j.landurbplan.2013.07.005
- Plieninger, T., Draux, H., Fagerholm, N., Bieling, C., Bürgi, M., Kizos, T., Kuemmerle, T., Primdahl, J., & Verburg, P.H. (2016). The driving forces of landscape change in Europe: A systematic review of the evidence. Land Use Policy, 57, 204–214. http://dx.doi.org/10.1016/j.landusepol.2016.04.040
- Plieninger, T., Kizos, T., Bieling, C., Le Du-Blayo, L., Budniok, M.A., Burgi, M., Crumley, C.L., Girod, G., Howard, P., Kolen, J., Kuemmerle, T., Milcinski, G., Palang, H., Trommler, K., & Verburg, P.H. (2015). Exploring ecosystem-change and society through a landscape lens: Recent progress in European landscape research. Ecology and Society, 20(2). https://doi.org/10.5751/ES-07443-200205
- Prishchepov, A.V., Ponkina, E.V., Sun, Z., Bavorova, M., & Yekimovskaja, O.A. (2021). Revealing the intentions of farmers to recultivate abandoned farmland: A case study of the Buryat Republic in Russia. Land Use Policy, 107, 105513. https://doi.org/10.1016/j.landusepol.2021.105513
- Ramankutty, N., & Coomes, O.T. (2016). Land-use regime shifts: An analytical framework and agenda for future land-use research. Ecology and Society, 21(2). https://doi.org/10.5751/ES-08370-210201
- Reed, J., Van Vianen, J., Deakin, E.L., Barlow, J., & Sunderland, T. (2016). Integrated landscape approaches to managing social and environmental issues in the tropics: Learning from the past to guide the future. Global Change Biology, 22(7), 2540–2554. https://doi.org/10.1111/gcb.13284
- Sayer, J., Sunderland, T., Ghazoul, J., Pfund, J.L., Sheil, D., Meijaard, E., Venter, M., Boedhihartono, A.K., Day, M., Garcia, C., van Oosten, C., & Buck, L.E. (2013). Ten principles for a landscape approach to reconciling agriculture, conservation, and other competing land uses. Proceedings of the National Academy of Sciences, 110(21), 8349–8356. https://doi.org/10.1073/pnas.1210595110
- Schlüter, M., Baeza, A., Dressler, G., Frank, K., Groeneveld, J., Jager, W., Janssen, M.A., McAllister, R.R.J., Müller, B., Orach, K., Schwarz, N., & Wijermans, N. (2017). A framework for mapping and comparing behavioural theories in models of social-ecological systems. Ecological Economics, 131, 21–35. https://doi.org/10.1016/j.ecolecon.2016.08.008
- Schomers, S., Meyer, C., Matzdorf, B., & Sattler, C. (2021). Facilitation of public payments for ecosystem services through local intermediaries: An institutional analysis of agri-environmental measure implementation in Germany. Environmental Policy and Governance, N/a, 31(5), 520–532. https://doi.org/10.1002/eet.1950
- Sneegas, G., Beckner, S., Brannstrom, C., Jepson, W., Lee, K., & Seghezzo, L. (2021). Using Q-methodology in environmental sustainability research: A bibliometric analysis and systematic review. Ecological Economics, 180, 106864. https://doi.org/10.1016/j.ecolecon.2020.106864
- Souchère, V., Millair, L., Echeverria, J., Bousquet, F., Le Page, C., & Etienne, M. (2010). Co-constructing with stakeholders a role-playing game to initiate collective management of erosive runoff risks at the watershed scale. Environmental Modelling and Software, 25(11), 1359–1370. https://doi.org/10.1016/j.envsoft.2009.03.002
- Tappeiner, U., Leitinger, G., Zarina, A., & Burgi, M. (2020). How to consider history in landscape ecology: Patterns, processes, and pathways. Landscape Ecology, 36(1), 2317–2328. h ttps://d oi.org/1 0.1 007/s109 80-02 0-01163-w
- Tellman, B., Magliocca, N.R., Turner, B.L., & Verburg, P.H. (2020). Understanding the role of illicit transactions in land-change dynamics. Nature Sustainability, 3(3), 175–181. https://doi.org/10.1038/s41893-019-0457-1
- van der Ploeg, J.D. (2021). The political economy of agroecology. The Journal of Peasant Studies, 48(2), 274–297. https://doi.org/10.1080/03066150.2020.1725489
- van Vliet, J., de Groot, H.L.F., Rietveld, P., & Verburg, P.H. (2015). Manifestations and underlying drivers of agricultural land use change in Europe. Landscape and Urban Planning, 133, 24–36. https://doi.org/10.1016/j.landurbplan.2014.09.001
- van Vliet, J., Magliocca, N.R., Buchner, B., Cook, E., Benayas, J.M.R., Ellis, E.C., Heinimann, A., Keys, E., Lee, T.M., Liu, J.G., Mertz, O., Meyfroidt, P., Moritz, M., Poeplau, C., Robinson, B.E., Seppelt, R., Seto, K.C., & Verburg, P.H. (2016). Meta-studies in land use science: Current coverage and prospects. Ambio, 45(1), 15–28. https://doi.org/10.1007/s13280-015-0699-8
- Verburg, P.H., Alexander, P., Evans, T., Magliocca, N.R., Malek, Z., Rounsevell, M.D.A., & van Vliet, J. (2019). Beyond land cover change: Towards a new generation of land use models. Current Opinion in Environmental Sustainability, 38, 77–85. https://doi.org/10.1016/j.cosust.2019.05.002
- Voinov, A., & Bousquet, F. (2010). Modelling with stakeholders. Environmental Modelling and Software, 25(11), 1268–1281. https://doi.org/10.1016/j.envsoft.2010.03.007
- Walters, B.B., & Vayda, A.P. (2020). Mechanisms and causal histories: Explanation-oriented research in human ecology. Human Ecology, 48(6), 641–650. https://doi.org/10.1007/s10745-020-00202-z
- Walters, B.B. (2017). Explaining rural land use change and reforestation: A causal-historical approach. Land Use Policy, 67, 608–624. https://doi.org/10.1016/j.landusepol.2017.07.008
- Wilson, G.A. (2008). From ‘weak’ to ‘strong’ multifunctionality: Conceptualising farm-level multifunctional transitional pathways. Journal of Rural Studies, 24(3), 367–383. https://doi.org/10.1016/j.jrurstud.2007.12.010
- Wirth, E. (1969). Zum Problem einer allgemeinen Kulturgeographie: Raummodelle – Kulturgeographische Kräftelehre – Raumrelevante Prozesse – Kategorien. Die Erde, 100(2-4), 155–193.
- Woodcock, C.E., Loveland, T.R., Herold, M., & Bauer, M.E. (2020). Transitioning from change detection to monitoring with remote sensing: A paradigm shift. Remote Sensing of Environment, 238, 111558. https://doi.org/10.1016/j.rse.2019.111558
- Young, O.R., Lambin, E.F., Alcock, F., Haberl, H., Karlsson, S.I., McConnell, W.J., Myint, T., Pahl-Wostl, C., Polsky, C., Ramakrishnan, P.S., Schroeder, H., Scouvart, M., & Verburg, P.H. (2006). A Portfolio approach to analyzing complex human-environment interactions: Institutions and land change. Ecology and Society, 11(2). https://doi.org/10.5751/ES-01799-110231
- Zabala, A., Sandbrook, C., & Mukherjee, N. (2018). When and how to use Q methodology to understand perspectives in conservation research. Conservation Biology, 32(5), 1185–1194. https://doi.org/10.1111/cobi.13123
- Zaehringer, J.G., Lundsgaard-Hansen, L., Thein, T.T., Llopis, J.C., Tun, N.N., Myint, W., & Schneider, F. (2020). The cash crop boom in southern Myanmar: Tracing land use regime shifts through participatory mapping. Ecosystems and People, 16(1), 36–49. https://doi.org/10.1080/26395916.2019.1699164
- Zarina, A. (2013). Path dependence and landscape: Initial conditions, contingency and sequences of events in Latgal e, Latvia. Geografiska Annaler Series B-Human Geography, 95(4), 355–373. https://doi.org/10.1111/geob.12030
- Zelaya, K., van Vliet, J., & Verburg, P.H. (2016). Characterization and analysis of farm system changes in the Mar Chiquita basin, Argentina. Applied Geography, 68, 95–103. https://doi.org/10.1016/j.apgeog.2016.02.001