ABSTRACT
Urban land change and transportation infrastructure development often interact and collectively lead to significant socioeconomic and biophysical impacts. Here, we performed a systematic review to identify how urban land change modeling studies account for transportation infrastructure. We found that less than one-fifth of urban land modeling studies explicitly incorporated a transportation component. Of these, most incorporated transportation based on relatively simple distance-based variables. Despite the recognized influence of transportation on urban growth, only a few studies attempted to capture the dynamic interaction between the two. We present a conceptual modeling framework and argue for a renewed focus on capturing the dynamic interaction between urban land change and transportation development in modeling studies. Such focus is essential to develop a well-informed understanding of the implications for urban forms and landscapes of the wide-ranging changes in transportation systems that accompany rapid urbanization around the world.
1. Introduction
The scale and pace of contemporary urbanization highlight the importance of understanding the dynamics behind urban land change to plan for and manage its impacts on landscapes (Forman & Wu, Citation2016; Grimm et al., Citation2008; Güneralp et al., Citation2020). While the world is rapidly becoming more urban, profound changes are taking place in technologies and modes of transportation. To accommodate and further stimulate urban and economic development, new transportation infrastructure from light-rail transport systems to highways at city and metropolitan scales to new transportation corridors at large regional scales are built, proposed, or under construction around the world (Miller, Citation2013), sometimes in connection with ambitious projects such as the African ‘development corridors’ (Enns, Citation2018) and the Belt and Road Initiative (BRI; Z. Liu et al., Citation2020). Furthermore, significant changes are taking place in technologies and modes of transportation such as new generation of Electric Vehicles (EV) and Autonomous Vehicles (AV; Stead & Vaddadi, Citation2019; Zhang & Guhathakurta, Citation2021) and ride-hailing and ride-sharing (Shaheen et al., Citation2021).
Transportation development interacts with urban form through land prices, location choices of individuals that lead to land-use decisions, activity locations, and density (Anas et al., Citation1998; Giannopoulos & Curdes, Citation1992; Mackett, Citation1985). Therefore, urban land change and changes in transportation policy and infrastructure influence each other (Rode et al., Citation2017; Wegener, Citation2005). The ongoing broad and profound changes in transportation modes and infrastructure will likely have long-lasting impacts on the spatial patterns and extent of urban areas with significant implications for natural as well as built environments (Ascensão et al., Citation2018; Güneralp et al., Citation2021; Hasnat et al., Citation2021; Laurance et al., Citation2015; Shaheen et al., Citation2021; Stead & Vaddadi, Citation2019). Therefore, it is crucial to account for interactions between the changes in transportation infrastructure and urban-land expansion to develop an accurate understanding of the socioeconomic and biophysical impacts of future urban-land and transportation-infrastructure development.
Initial attempts to model urban land change (LC) in the 1960s emphasized understanding how complex subsystems that make up an urban area interact with each other and how the urban area grows as a consequence of these interactions (Batty, Citation2008). Along with others, transportation system was included as a distinct subsystem in these models. While the Lowry model incorporated the spatial interaction between land-use and transportation as early as the 1960s (Lowry, Citation1964), the emphasis initially was on accurate representation of trips across a given static urban land-use composition and configuration. In what would later come to be called as land-use transportation (LUT) models, there has been a shift over time towards studying dynamic spatial interactions between activity locations, land-use, and transportation demand (Berling-Wolff & Wu, Citation2004). However, the emphasis on LUT models remained to be optimization of land-use patterns according to specific objectives rather than understanding and forecasting urban land expansion (NRC, Citation2014).
With the emergence of land change science in the early 1990s (Turner et al., Citation2007), LC models, including those specifically developed to forecast the amount and spatial pattern of urban areas, gained substantial momentum (Musa et al., Citation2017; Santé et al., Citation2010). However, unlike LUT models, representation of transportation remained relatively simple in a large portion of the body of work on LC modeling (NRC, Citation2014). Likewise, to date, there has not been a detailed assessment of how transportation is incorporated in LC models. Considering the innovations in transportation technology and service modes, and the extensive development of transportation infrastructures at local, regional, and global scales concomitant with rapid urbanization, this presents a significant gap in our knowledge. Therefore, in this study, we performed a systematic review of the LC models that incorporated land-transportation infrastructure in understanding and forecasting urban land change. In our review, we only considered those LC models in which urban land is one of the land types considered; we refer to these models, for simplicity, as ‘urban LC models’ throughout the rest of this article. We established a broad scope for land transportation in our review since we are interested in capturing the entire range of approaches to incorporating transportation in urban LC models as they exist in the peer-reviewed literature. Therefore, our definition of transportation encompasses a wide range from road and rail networks to nodes of access (e.g. metro stations) to operational (e.g. cost), service-related (e.g. mobility and accessibility) and policy dimensions as represented in the literature. Our objective is to (i) assess how urban LC models account for the influences of land transportation on urban land expansion and (ii) identify specific gaps in, and opportunities for, incorporating the interaction between land-transportation development and urban growth in urban LC models. We discuss our findings along with a conceptual framework on how we believe these interactions can be represented in urban LC models.
2. Methodology
2.1. Systematic review
2.1.1. Guidelines and data source
In our systematic review, we considered the use of transport networks, infrastructures, policies, operations, service, accessibility, and modal distribution as transport-related variables used in urban LC models. We systematically searched the ISI Web of Science database for publications with a set of keywords covering different aspects of urban growth, urban growth modeling, urban LC modeling, and urban land-use land cover growth modeling (detailed search query and process included in the supplementary materials Table S1). We considered peer-reviewed journal articles that were published in English and available online by 31 August 2021. We followed the reporting guidelines asserted in the Preferred Reporting Items for Systematic Reviews and Meta-Analyses (PRISMA) statement (Moher et al., Citation2009).
2.1.2. Selection of studies and evidence synthesis
After retrieving the records from the database search, we screened them using the Rayyan, a web application for conducting systematic reviews (Ouzzani et al., Citation2016). We first removed the duplicate records (n = 31) from the entire list. We then screened the remaining records (n = 11,357) based on the titles, keywords, and abstracts and removed 10,680 records in the process. It is possible that a number of papers used transportation in various forms as standard practice and, thus, did not mention that explicitly in any of the three fields (title, abstract, keywords) we used for the initial screening. If any of the papers retrieved from database search did not mention the use of transportation or accessibility in their title, abstract or keywords, those papers were excluded in the screening processes and not included in our study. Following that, we reviewed the full text of the records (n = 677), eliminated the papers that did not comply with the preset inclusion criteria (n = 549) and settled the final list of papers (n = 128; ).
We included papers in our review only if they fulfilled the following criteria: (1) use urban modeling techniques as part of the study; (2) incorporate transportation-related variables in the model; and (3) have an application on the real-world sites. Once the papers were finalized based on the inclusion criteria, we used a predesigned matrix to extract specific information from the papers. This includes – (1) purpose of the study, (2) methods/models used, (3) calibration and forecast interval, (4) study location, and (5) method of incorporating transportation development. Note that, in our review, we assessed how land transportation is represented within urban LC models in relation to the well-established notion that transportation and land change are often tightly interrelated (Giannopoulos & Curdes, Citation1992; Mackett, Citation1985; Rode et al., Citation2017; Wegener, Citation2005); we did not assess how well suited the particular representation was in relation to the stated objectives of the study in question. All the papers in our review along with the coded information are included in supplementary material.
3. Results
3.1. Summary and characteristics of the reviewed papers
Studies that explicitly used transportation network and any form of transportation development constitute a small subset within the published urban LC modeling literature. We found a total of 128 papers, out of 677 screened full texts (less than one-fifth), that used transportation-related variables as part of an urban LC model. These variables include transportation network and infrastructure location, distance to the transportation network, and accessibility measures. We classified the studies based on their land-change focus – i.e. whether focused exclusively on urban growth (n = 109) or used urban as one of the several land-use types in the LC model (n = 19). The case study locations come from 38 countries ()). China (n = 36) had the largest number of case-study locations followed by the United States (n = 13; )). The earliest paper included in our review was published in 2002. Nevertheless, the number of papers that explicitly account for transportation increased substantially over time: more than half of the total papers included in our review (n = 84) were published in the past five years ()). This increase in the total number of papers has shown a spatial trend as well (Table S2). For instance, 34 out of the 36 papers from China were published in the past six years. In the case of India, 6 out of 10 papers published in 2021 only, and 9 out of 10 papers published in the past four years.
Figure 2. Geographic distribution of the case study locations and number of papers published over time. (a) Location of the case study sites. A particular location may appear in more than one paper, and an individual paper could include multiple locations. Papers in our review with global (a total of 8) and country-wide forecasts (a total of 4– Bangladesh, China, Luxembourg, Indonesia) are excluded from the visualization, (b) Countries with more than one case study location (a total of 14). (c) Number of papers by year (up to August 31 in 2021).
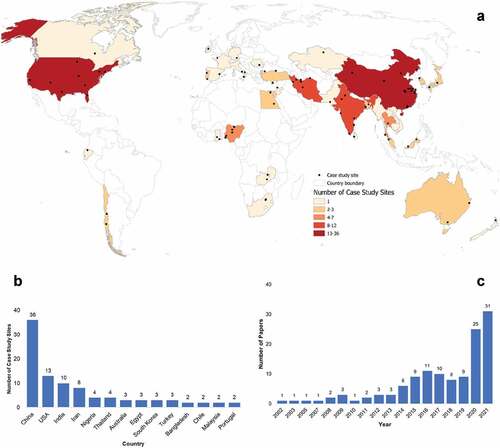
Almost half of the papers were focused on forecasting the urban growth of a specific city or country (n = 61). The second most common motivation was to develop or modify an existing urban LC model (n = 50). Most of these city-scale studies (hereafter ‘local’) used a comparatively smaller forecast interval compared to the large, country-level (hereafter ‘regional’) or global-scale studies (hereafter ‘global’; ). Similarly, in contrast to the local studies, global studies tend to forecast over a longer time span – all of the included global studies forecasted at least 30 years into the future. Based on the primary motivations in the reviewed papers, we classified them into ‘mainly applied’ (n = 99), ‘mainly method development’ (n = 16), and ‘mixed-method development and applied’ (n = 13). They correspond to, respectively, 77%, 13%, and 10% of all the papers in our review.
Figure 3. Distribution of forecast interval (i.e. time step) and forecast span used in the studies based on their spatial scale – ‘local’ indicates the city-scale studies, ‘regional’ studies focused on urban agglomeration, country-scale studies, multiple neighboring countries or such region, and ‘global’ scale studies included the entire terrestrial land area in their studies.
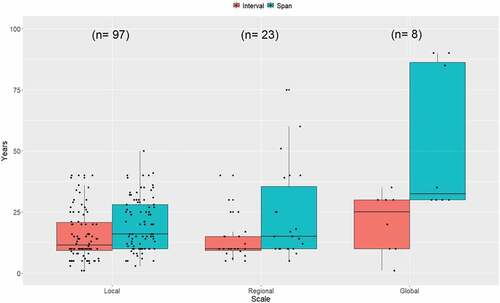
A co-occurrence analysis with the author keywords of the papers (Figure S1) revealed that the most frequently used keyword is ‘Cellular Automata’ (10%) along with ‘urban expansion’ (4.5%) and ‘urban growth’ (4.5%). There are other frequently used keywords such as ‘land-use change’ (2.3%), ‘land use’ (1.5%), or ‘urban sprawl’ (1.5%). Less than 1% of the keywords were related to transportation network (n = 2), transportation modeling (n = 1), or accessibility (n = 1).
3.2. Transportation in urban land change modeling
3.2.1. The representation of transportation in urban land change models
Based on how transportation-related information is used in respective urban LC models, three broad thematic groups emerge from the papers in our review (Table S3). Among those studies that included transportation-related variables in an urban LC model, the most frequently used method is representing transportation’s influence through ‘distance-based approaches’. The ‘distance-based approaches’, implemented in 75% of the papers in our review, use some representation of the distance to the transportation network in forecasting future urban land expansion. The second most employed method (in 47% the papers; n = 60) is using transportation facilities, e.g. railway stations, bus stations in addition to, or in place of, a ‘distance-based approach’. ‘Accessibility-based approaches’ are the most advanced and yet the least frequently employed method (in 18% of the papers), again often in conjunction with the other two methods (). The studies that employed ‘accessibility-based approaches’ computed accessibility based on travel time, travel cost, travel speed, and congestion on roads. Additionally, one study also utilized road density as a proxy both for urban area and accessibility assuming higher road density will indicate shorter distance to major roads. Several studies used various combinations of these approaches and thus belong to more than one group (Table S3). Notably, only five papers (Behan et al., Citation2008; Zhao & Peng, Citation2012; 2014; Waddell, Citation2002; Waddell et al., Citation2007) represented the dynamic interaction between transportation and land change in their respective urban LC models. All five incorporated the principles of a well-established approach in transportation planning, known as the four-step model, used to forecast future transportation demand (McNally, Citation2007; Southworth & Garrow, Citation2010). These papers implemented separate transportation submodules in accordance with the principles of the four-step model while also making use of one or more of the three approaches to represent transportation’s influence on urban land change.
Figure 4. Different approaches implemented to represent transportation in urban LC modeling literature. The papers are assigned to each group based on how they reported their incorporation of transportation in their respective urban LC models. If this information is not reported, the paper is listed under the ‘only road/transportation network’. Since, more than one approach are implemented in a number of papers, the total number of implementations under each group is larger than the corresponding number of papers.
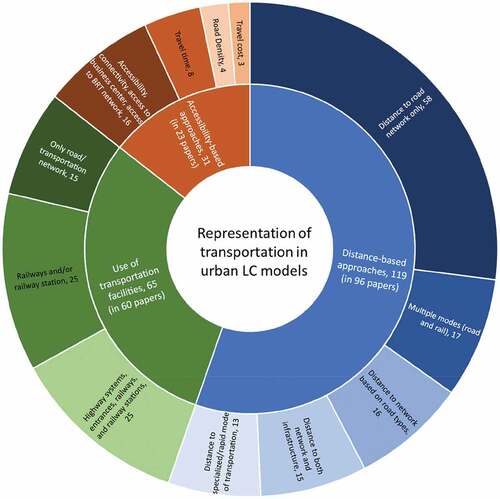
Only about 5% of papers (n = 6) implemented a scenario-based approach to forecast urban growth and incorporated transportation-related variables into each scenario (Table S4). In addition to the information on existing road network, these studies incorporated planned road network developments in forecasting urban land expansion. Furthermore, they included different road growth scenarios depending upon the development plans in question (i.e. rapid growth, natural growth, or conservative growth strategies) and generated scenario-specific urban land expansion forecasts. Other studies that adopted a scenario-based approach for forecasting urban land expansion implemented scenarios on network connectivity, transportation development, railway development, and environmental protection.
There are a few papers that used the transportation-related variables in a way that is distinct from all the others listed in Table S3. Four of these studies considered urban growth induced by transportation development (Guzman et al., Citation2020; Kakaraparthi & Kockelman, Citation2011; Novin & Khosravi, Citation2017; Yang & Lo, Citation2003). One study explicitly analyzed the impact of transportation policies on urban growth (Liang et al., Citation2018). This study modified an existing LC model to integrate the effects of different planning policies and high-speed rail planning in forecasting urban land change. They concluded that higher forecast accuracy is achieved by considering traffic planning into the LC model they employed. Another study considered the interaction between transportation development and population diffusion where they used the latter as a proxy for urban expansion (Wu et al., Citation2016).
Distance-based approaches. About 70% of all the papers in our review ―93% of all that implemented a ‘distance-based approach’― used ‘distance to the road network’ as a transportation-related variable (n = 89). These studies ―all but two of which were at city or metro-level― used transportation as a proximate factor that enabled them to calculate how proximity to transportation network influences urban growth. All used a fixed transportation network that did not grow over time and did not change in conjunction with the forecasted land-use changes. Furthermore, most studies did not report the type of distance measure they implemented (e.g. Euclidean, network) and what they considered as entry points to the network (e.g. stations, nearest points, or highway entrance points). In the absence of representation of such entry points, the models calculate the shortest distance to the network (e.g. Akin et al., Citation2015; Gharbia et al., Citation2016; Ozturk, Citation2015; X. P. Liu et al., Citation2018; Yang & Lo, Citation2003; Yuan, Citation2010), which may fail to reflect the actual accessibility of the mode of transportation in question. In addition to calculating distance to major roads or highways, few studies (n = 16) classified different road types and used those in their modeling approaches (classified as major and minor roads, urban or rural roads, or primary and secondary roads; e.g. Gharbia et al., Citation2016; Wang et al., Citation2019).
Use of transportation facilities. In addition to the transportation network, several studies used transportation facilities in their models (n = 62). Using these facilities in the urban LC model provides greater information on connectivity compared to the use of distance to the network. Among the facilities mentioned, railway-related facilities were most frequently used (e.g. Abolhasani et al., Citation2016; Bardhan, Citation2013; Omrani et al., Citation2017; Zhao & Peng, Citation2014), along with bus stops, airport locations, and highway systems entrance (e.g. Chuangchang et al., Citation2016; X. P. Liu et al., Citation2018; Yang et al., Citation2020; Yuan, Citation2010). The difference between using a specific entry point to the transportation network (e.g. the entrance to a highway system or stations) and the shortest distance to the same network (e.g. distance to the highway or major roads) is that the first considers whether a passenger or a vehicle can use a given entry point, whereas the latter only considers the Euclidean distance to the nearest vertices regardless of the placement of entry points.
Several studies were specific to what type of modes are associated with these facilities. For example, one-fifth of the 60 papers that represented transportation facilities in their urban LC models considered the distance to subway stations, metro rails and other rapid rail transits (e.g. Abolhasani et al., Citation2016; Bardhan, Citation2013; Y. L. Liu et al., Citation2016; Ozturk, Citation2015; Zhou et al., Citation2020). Nearly one-fourth (n = 15) considered both the distance to the road network and the distance to transportation facilities (e.g. Iacono & Levinson, Citation2009; Omrani et al., Citation2017; Yu et al., Citation2018; Zhao & Peng, Citation2014; Zhou et al., Citation2020). However, these studies too assumed that the transportation infrastructure – including the entry points – remain invariant over time.
Accessibility-based measures. In only 18% of the papers in our review (n = 23), urban LC models incorporated information on how fast the users could move along the transportation network. These studies measured how different land-use classes are accessible or connected to each other through the transportation network. At the same time, they also considered access to urban centers, commercial areas, and central business districts (CBD). In addition to the land-use classes, some also considered the access to different transportation facilities (e.g. railway stations, airports, light rail transit (LRT) stations, subways). Ten out of these 23 papers used the distance-based metrics of accessibility with shorter distance indicating higher access (e.g. Abolhasani et al., Citation2016; An & Lee, Citation2020; Dinda et al., Citation2018; Khamchiangta & Dhakal, Citation2021). This type of modeling approach was typically implemented in case study locations in China and India. In fact, 12 out of the 21 case-study locations were in these two countries (9 in China and 3 in India) compared to the 9 case-study locations from all other countries and regions.
In place of the simpler distance-based accessibility, metrics on travel cost, travel time, speed and congestion, and road density were used to calculate accessibility in 11 out the 23 papers (e.g. Bardhan, Citation2013; Behan et al., Citation2008; Iacono & Levinson, Citation2009; Zhao & Peng, Citation2012). One study appears to have used road density as a measure of accessibility with the assumption that higher density of major roads indicates higher access to the road network (Terando et al., Citation2014). The same study also used road density as a proxy for urban growth. Only three papers used ‘travel speed’ to measure accessibility. These studies calculated travel time to the destination using the posted travel speed of the specific segment of the road network (e.g. Behan et al., Citation2008; Linard et al., Citation2013; Valencia et al., Citation2020). Studies that used travel speed and access to networks and railway stations reported accessibility as the most influential variable in their models in influencing urban growth (Linard et al., Citation2013).
3.2.2. Transportation submodule in urban land change models
Although the history of travel demand modeling for transportation forecasting goes back to the 1950s (McNally, Citation2007; Southworth & Garrow, Citation2010), its use in urban LC modeling remained limited. One of the relatively recent examples of integrating the travel demand modeling into urban growth prediction is the UrbanSim model (Waddell, Citation2002). This model sub-divides the entire process into a series of smaller sub-models. Each sub-model captures one specific aspect of the study area: housing, land-use development, environment, and real-estate. The model also incorporates a transportation module. Once all the sub-models complete the computation, they are combined to predict the urban growth in a future year. However, there are a few issues with this approach: Significant limitations reported in this approach are that the time and budget required to collect the necessary data were substantial. At the same time, it also requires substantial computational efficiency to run all the sub-models. The immense resource requirement of UrbanSim is one of the major reasons why the approach has not been widely adopted in later modeling studies on urban expansion (Waddell, Citation2002; Zhao & Peng, Citation2012, Citation2014).
More recent studies focused on incorporating simpler stand-alone transportation modules into existing urban LC models. These studies used transportation submodule to forecast future trips in different traffic analysis zones (TAZ; Behan et al., Citation2008; Zhao & Peng, Citation2012, Citation2014). The models used in these studies have separate submodules for land-use and transportation modeling. The outputs from the land-use submodule are population density and employment locations, which are used by the transportation submodule as its input. Following the trip forecast, the model then uses logit models for modal split calculation and uses the stochastic user equilibrium assignment model for network assignment. The use of submodules enabled these models capture the bidirectional relationship between land-use and transportation. These studies reported a substantial relationship between congestion, travel time, and land-use choice, which the urban LC models in question calculate through their respective transportation submodules.
3.2.3. Representation of transportation variables across regions and spatial scales
Representation of transportation in the urban LC models in the reviewed papers varies with the scale of analysis (). Compared to the local studies, global studies undertook simpler representations of transportation in their models. Out of the 27 global LC modeling studies, we found eight that had an explicit transportation component (Table S5). Most of these eight global-scale studies used ‘distance-based approaches’ (n = 6). This could indicate a lack of data availability or considerations of computational efficiency that may have limited the capacity to consider more detailed aspects of transportation. Notably, only one of these studies (Li et al., Citation2016) considered both road network and railways, and none incorporated the interaction between the transportation network and urban land expansion.
Figure 5. Representation of transportation-related variables in different spatial-scales – ‘local’ indicates the city-scale studies, ‘regional’ studies focused on urban agglomerations, country-scale studies, or large areas encompassing multiple countries, and ‘global’ scale studies included the entire terrestrial land area in their studies.
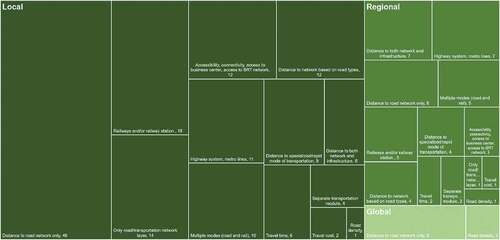
Although the use of ‘distance-based approaches’ is also common in local and regional studies, unlike global studies, both local and regional studies collectively incorporated a more diverse sets of variables. Over half of the local studies (n = 46) used ‘distance to road network only’ as the transportation-related variable in their model. Adopting a separate transportation (sub)-module to integrate the feedback between transportation development and urban land change has also been most visible in local-scale studies – 4 out of 6 such studies are performed in local case studies. Though limited in number, both local- (n = 12) and regional-scale (n = 3) studies incorporated accessibility-related variables. Both local- and regional-scale studies also used specialized transportation modes such as subway and metro rail in their respective studies.
There are notable differences across geographies in how transportation-related factors are incorporated in urban LC models. Importantly, studies from both China and India emphasized railway-related variables in their respective urban LC models. Approximately one-third of the studies from both countries used such variables (11 from China, and 4 from India). Similarly, all the studies that included some form of specialized or rapid transit mode (e.g. bus rapid transit and metro) are from Asia – China in particular with the highest number of such studies (n = 5). On the other hand, there are only three studies that considered travel cost as a measure of accessibility and all are from the U.S. Furthermore, all but two studies that considered travel time as a proxy for accessibility are from the U.S. Similarly, the case studies that represented the transportation with a separate submodule or considered transportation interaction with urban growth are predominantly ― 4 out of the 6 case studies ―from the U.S.
4. Discussion
Transportation infrastructure development improves accessibility and connectivity of growing urban areas (Miller, Citation2013) and, thus, influence the extent and pattern of urban land change (Rode et al., Citation2017). In turn, urban growth and economic development typically necessitate further development of transportation networks (Cervero, Citation2003; Collier & Venables, Citation2016). Yet, despite the importance of the interaction between transportation development and urban growth (Rui & Ban, Citation2011; Wegener, Citation2005), its representation in urban LC modeling virtually remained absent. This is evidenced from our systematic review of LC modeling studies that included urban as one of the land classes: only 128 out of 677 studies (less than one-fifth) had an explicit transportation component and those that had mostly employed relatively simple, distance-based representations of the transportation network (; Table S2). Past studies reported limited computational capabilities and data availability as the reasons behind the limited representation of the interaction between urban land change and transportation development (Berling-Wolff & Wu, Citation2004; Moeckel, Citation2018). Nevertheless, with improvements in computational technology and increasing availability of higher resolution data on land cover, land-use, and infrastructure, the number of studies with explicit representation of transportation in urban LC models has been increasing over the past 10 years ().
Our review also showed geographic clustering of transport-related variables reflecting the varying contexts and dominant modes of transportation in different parts of the world. For example, a relatively large number of railway-related variables appear in the papers with case study sites in China and India. China has the world’s largest and most rapidly growing high-speed railway network (Lawrence et al., Citation2019; Yao et al., Citation2019) and railway has been the most common mode of passenger transportation in India for more than a century (Gangwar, Citation2020). Alternatively, the use of highway or freeway systems or submodules in the US-based studies coincides with the dominant mode of transportation in that country.
Using only data on transportation network or distance to the network without taking accessibility of transportation into account may not adequately capture transport infrastructure’s impact on urban growth and, thus, lead to misleading forecasts and understandings. There are only a few studies that represented accessibility by incorporating the network distance to the closest entry points, e.g. highway entrances, train stations, etc. (e.g. Chen et al., Citation2017; X. P. Liu et al., Citation2018; Yang et al., Citation2020; Yuan, Citation2010). The use of facility locations with a separate transportation network provides a more realistic representation of users’ trip-making behavior as well as how transportation system in question functions. Furthermore, even fewer number of papers adopted travel time and cost to measure accessibility in their models even though the use of travel speed through the network provides a more accurate representation of varying levels of access to different places throughout the city, and hence, of mobility. Such urban LC models therefore capture more accurately how transportation impacts urban land change. However, papers in our review that incorporated these aspects of the transportation system in addition to the transportation network still assumed that the transportation infrastructure – including the entry points – remains invariant over time. Thus, by ignoring the dynamic relationship between the transportation and urban growth, they, in effect, assume invariant accessibility throughout the simulation horizon, which in some applications spans decades (e.g. Gharbia et al., Citation2016; Varquez et al., Citation2020) over study areas that can range from metropolitan regions to continents (e.g. Güneralp & Seto, Citation2013; Omrani et al., Citation2017).
Urban LC models also often lack the relevant social, economic, and policy factors that mediate the interaction between transportation and urban land change. Early urban economic models explained the urban spatial structure by considering the distance between different land-use classes, presuming people will commute from residential zones to the CBDs (Irwin & Geoghegan, Citation2001). Von Thünen model, based on the economic bid-rent theory, is one of the earliest examples of such attempts (Von Thünen, Citation1966). These distance and location-based urban economic models and the LUT models that were developed during the same period focused, however, more on explaining the spatial structure of different land uses within urban areas. In addition, despite the importance of policy and planning choices in transportation development (Xie & Levinson, Citation2009), these were given little attention in urban LC models. While adding these components to urban LC models is mired with issues about data availability and veracity, a relatively simple but effective approach to study the implications of such policy and planning choices, scenario analysis (Guzman et al., Citation2020; Huang et al., Citation2014; Liang et al., Citation2018), is surprisingly little employed in urban LC models (Table S4). The use of scenario-based modeling allows to experiment with different policy formulations regarding, for example, economy, resource use or climate change mitigation, and hence to study the likely behavioral responses of agents (e.g. individuals and institutions) to any policy changes or adaptation to new technologies. Scenario analysis can also help in accounting for the uncertainties of the future (Güneralp & Seto, Citation2013).
Taking the individual actors’ cognitive processes of perception and decision-making would allow the models to more comprehensively capture the role of human behavior, as well as other relevant social, environmental, and cultural factors (Roy Chowdhury & Turner, Citation2019). Considering the cognitive processes would also help make LC models move away from the traditional assumptions of rational choice theory (Meyfroidt, Citation2013). Individual decision-making has a direct influence on the role of transportation in urban growth. Individual users’ residential location choices as well as their preferences for mobility and mode of transportation ultimately influence the urban form (Shaheen et al., Citation2021). One way of incorporating decision-making processes of agents is through integrated agent-based and microsimulation models (Huang et al., Citation2014). However, these approaches require large amounts of data at the individual level and, hence, may not be feasible, especially in the developing-country contexts.
Recent technological and service-mode innovations in transportation may also be highly consequential for urban growth (Giannopoulos & Curdes, Citation1992; Rode et al., Citation2017). For example, the growth of EVs, AVs, and ride-hailing services is expected to modify travel behavior (Harb et al., Citation2021), contribute to higher accessibility, lower travel time, higher vehicle miles traveled, exacerbate mobility, and subsequently, influence the urban form. Thus, it is essential to consider how these technological and service-mode innovations will inform the location choice and shape urban land change in the future.
Although LUT models have been used since the 1970s, transportation as part of any urban LC model was introduced much later. Remarkably, only five out of a total of 128 studies incorporated the interaction between transportation and land change in an urban LC model (Table S3). Furthermore, despite the recent surge in transportation projects (Laurance et al., Citation2015; Miller, Citation2013), recent advances in transportation technology (Shaheen et al., Citation2021; Stead & Vaddadi, Citation2019), and increasing number of studies that focus on their various implications (Ascensão et al., Citation2018; Güneralp et al., Citation2021; Hasnat et al., Citation2021), a focus on these transformative changes in transportation has so far been absent in the urban LC modeling literature. Yet, land system science provides a rich theoretical foundation (Roy Chowdhury and Turner, Citation2019; Turner et al., Citation2020) and land change (LC) modeling, a potentially powerful analytical tool (NRC, Citation2014), to examine the interactions between transportation infrastructure development and urban land change as well as the resulting impacts on landscapes and livelihoods.
To this end, employing a conceptual framework where the dynamic interaction between urban growth and transportation development is adequately captured in LC models has growing importance to understand likely impacts of this interaction on the spatial extents and patterns of urban land expansion and on transportation networks as well as the resulting changes across landscapes. Here, we put forward such a framework where a submodule for transportation system can be implemented to model the interaction between urban land-use change and transportation development (). Hence, the magnitude of new urban land is forecasted based on socio-economic variables, which would then be allocated across the landscape based on several spatial factors such as topography, accessibility afforded by transportation network, existing land-use land cover, and population density. In turn, the accessibility to urban areas is expected to change. Based on the updated urban amount and accessibility, the road network would change and influence the forecasted amount of urban land and its spatial allocation in the next time step. The most significant aspect of this framework is that it has a dynamic feedback loop to incorporate the transportation-urban growth interaction.
5. Conclusion
Sixty years after the development of the first urban land use model with an explicit transportation component, and despite significant advances in LC modeling in recent decades, representation of transportation in urban LC models is still inadequate to capture the interactions between transportation development and urban land expansion. Our systematic review showed that less than one-fifth of LC modeling studies with a distinct urban land class explicitly incorporated transportation-related factors in their respective models. Of these, most used simple representations of the transportation system such as a road network with no distinction among the types of roads. The transportation infrastructure developments concomitant with rapid urbanization and economic development will continue to transform landscapes with cascading impacts across social, economic, and ecological systems. It is imperative to capture the interaction between transportation and urban land change in urban LC models to develop a more comprehensive understanding of the likely impacts on urban forms and landscapes and inform policy.
Supplemental Material
Download MS Word (621.2 KB)Supplemental Material
Download MS Excel (77.2 KB)Acknowledgement
This study contributes to the Global Land Programme (https://glp.earth) and was supported by funds from the Triads for Transformation (T3) Program as part of the President’s Excellence Fund Initiative at Texas A&M University.
Disclosure statement
No potential conflict of interest was reported by the author(s).
Data availability statement
The authors confirm that the data supporting the findings of this study are available within the manuscript and its supplementary materials.
Supplementary material
Supplemental data for this article can be accessed online at https://doi.org/10.1080/1747423X.2022.2086639
References
- Abolhasani, S., Taleai, M., Karimi, M., & Node, A.R. (2016). Simulating urban growth under planning policies through parcel-based cellular automata (ParCA) model. International Journal of Geographical Information Science, 30(11), 2276–2301. https://doi.org/10.1080/13658816.2016.1184271
- Akin, A., Sunar, F., & Berberoglu, S. (2015). Urban change analysis and future growth of Istanbul. Environmental Monitoring and Assessment, 187(8), 1–15. https://doi.org/10.1007/s10661-015-4721-1
- An, Y., & Lee, S. (2020). A spatial disaggregation model to improve long-term land use forecasting with transport models based on zonal data. Applied Spatial Analysis and Policy, 13(1), 187–208. https://doi.org/10.1007/s12061-019-09298-3
- Anas, A., Arnott, R., & Small, K.A. (1998). Urban spatial structure. Journal of Economic Literature, 36(3), 1426–1464 https://www.jstor.org/stable/2564805.
- Ascensão, F., Fahrig, L., Clevenger, A.P., Corlett, R.T., Jaeger, J.A.G., Laurance, W.F., & Pereira, H.M. (2018). Environmental challenges for the belt and road initiative. Nature Sustainability, 1(5), 206–209. https://doi.org/10.1038/s41893-018-0059-3
- Ayazli I Ercument, Kilic F, Lauf S, Demir H and Kleinschmit B. (2015). Simulating urban growth driven by transportation networks: A case study of the Istanbul third bridge. Land Use Policy, 49 332–340. 10.1016/j.landusepol.2015.08.016
- Bardhan, R. (2013). Simulation of land use consequences of urban corridor in Kolkata: An integrated spatial and expert system model. Environment and Urbanization ASIA, 4(2), 267–286. https://doi.org/10.1177/0975425313510767
- Batty, M. (2008). Fifty years of urban modeling: macro-statics to micro-dynamics. In S. Albeverio, D. Andrey, P. Giordano, & A. Vancheri (Eds.), The dynamics of complex urban systems: An interdisciplinary approach (pp. 1–20). Physica-Verlag HD. https://doi.org/10.1007/978-3-7908-1937-3_1
- Behan, K., Maoh, H., & Kanaroglou, P. (2008). Smart growth strategies, transportation and urban sprawl: Simulated futures for Hamilton, Ontario. Canadian Geographer-Geographe Canadien, 52(3), 291–308. https://doi.org/10.1111/j.1541-0064.2008.00214.x
- Berling-Wolff, S., & Wu, J. (2004). Modeling urban landscape dynamics: A review. Ecological Research, 19(1), 119–129. https://doi.org/10.1111/j.1440-1703.2003.00611.x
- Cervero, R. (2003). Road expansion, urban growth, and induced travel: A path analysis. Journal of the American Planning Association, 69(2), 145–163. https://doi.org/10.1080/01944360308976303
- Chen, Y.M., Liu, X.P., & Li, X. (2017). Calibrating a land parcel cellular automaton (LP-CA) for urban growth simulation based on ensemble learning. International Journal of Geographical Information Science, 31(12), 2480–2504. https://doi.org/10.1080/13658816.2017.1367004
- Chuangchang, P., Thinnukool, O., & Tongkumchum, P. (2016). Modelling urban growth over time using grid-digitized method with variance inflation factors applied to spatial correlation. Arabian Journal of Geosciences, 9(5) 1–13. https://doi.org/10.1007/s12517-016-2375-0
- Collier, P., & Venables, A.J. (2016). Urban infrastructure for development. Oxford Review of Economic Policy, 32(3), 391–409. https://doi.org/10.1093/oxrep/grw016
- Dinda, S., Das, K., Chatterjee, N.D., & Ghosh, S. (2018). Integration of GIS and statistical approach in mapping of urban sprawl and predicting future growth in Midnapore town, India. Modeling Earth Systems and Environment, 5(1), 331–352. https://doi.org/10.1007/s40808-018-0536-8
- Enns, C. (2018). Mobilizing research on Africa’s development corridors. Geoforum, 88, 105–108. https://doi.org/10.1016/j.geoforum.2017.11.017
- Forman, R.T.T., & Wu, J. (2016). Where to put the next billion people. Nature, 537(7622), 608–611. https://doi.org/10.1038/537608a
- Gangwar, R. (2020). Handbook on railway regulation. In Finger, Matthias, Montero, Juan. Railways in India: Challenges and opportunities. Edward Elgar Publishing 146–164 . https://doi.org/10.4337/9781789901788.00015.
- Gharbia, S.S., Abd Alfatah, S., Gill, L., Johnston, P., & Pilla, F. (2016). Land use scenarios and projections simulation using an integrated GIS cellular automata algorithms. Modeling Earth Systems and Environment, 2(3), 1–20. https://doi.org/10.1007/s40808-016-0210-y
- Giannopoulos, G.A., & Curdes, G. (1992). Innovations in urban transport and the influence on urban form. An historical review. Transport Reviews, 12(1), 15–32. https://doi.org/10.1080/01441649208716801
- Grimm, N.B., Faeth, S.H., Golubiewski, N.E., Redman, C.L., Wu, J., Bai, X., & Briggs, J.M. (2008). Global change and the ecology of cities. Science, 319(5864), 756–760. https://doi.org/10.1126/science.1150195
- Güneralp, B., & Seto, K. (2013). Futures of global urban expansion: Uncertainties and implications for biodiversity conservation. Environmental Research Letters, 8(1), 014025. https://doi.org/10.1088/1748-9326/8/1/014025
- Güneralp, B., Reba, M., Hales, B.U., Wentz, E.A., & Seto, K.C. (2020). Trends in urban land expansion, density, and land transitions from 1970 to 2010: A global synthesis. Environmental Research Letters, 15(4), 044015. https://doi.org/10.1088/1748-9326/ab6669
- Güneralp, B., Xu, X., & Lin, W. (2021). Infrastructure development with(out) ecological conservation: The northern forests in İstanbul. Regional Environmental Change, 21(3), 86. https://doi.org/10.1007/s10113-021-01807-w
- Guzman, L.A., Escobar, F., Pena, J., & Cardona, R. (2020). A cellular automata-based land-use model as an integrated spatial decision support system for urban planning in developing cities: The case of the Bogota region. Land Use Policy, 92, 104445. https://doi.org/10.1016/j.landusepol.2019.104445
- Harb, M., Stathopoulos, A., Shiftan, Y., & Walker, J.L. (2021). What do we (not) know about our future with automated vehicles? Transportation Research Part C: Emerging Technologies, 123, 102948. https://doi.org/10.1016/j.trc.2020.102948
- Hasnat, M.M., Bardaka, E., Samandar, M.S., Rouphail, N., List, G., & Williams, B. (2021). Impacts of private autonomous and connected vehicles on transportation network demand in the triangle region, North Carolina. Journal of Urban Planning and Development, 147(1), 04020058. https://doi.org/10.1061/(ASCE)UP.1943-5444.0000649
- Huang, Q., Parker, D.C., Filatova, T., & Sun, S. (2014). A review of urban residential choice models using agent-based modeling. Environment and Planning. B, Planning & Design, 41(4), 661–689. https://doi.org/10.1068/b120043p
- Iacono, M.J., & Levinson, D.M. (2009). Predicting land use change how much does transportation matter? Transportation Research Record: Journal of the Transportation Research Board, 2119(1), 130–136. https://doi.org/10.3141/2119-16
- Irwin, E.G & Geoghegan, J. (2001). Theory, data, methods: Developing spatially explicit economic models of land use change. Agriculture, Ecosystems & Environment, 85(1), 7–24. https://doi.org/10.1016/S0167-8809(01)00200-6
- Kakaraparthi, S.K., & Kockelman, K.M. (2011). Application of UrbanSim to the Austin, Texas, Region: Integrated-model forecasts for the year 2030. Journal of Urban Planning and Development, 137(3), 238–247. https://doi.org/10.1061/(ASCE)UP.1943-5444.0000065
- Khamchiangta, D., & Dhakal, S. (2021). Future urban expansion and local climate zone changes in relation to land surface temperature: Case of Bangkok metropolitan administration, Thailand. Urban Climate, 37, 100835. https://doi.org/10.1016/j.uclim.2021.100835
- Laurance, W.F., Sloan, S., Weng, L., & Sayer, J.A. (2015). Estimating the environmental costs of Africa’s massive “development corridors”. Current Biology, 25(24), 3202–3208. https://doi.org/10.1016/j.cub.2015.10.046
- Lawrence, M., Bullock, R., & Liu, Z. (2019). China’s high-speed rail development. World Bank Publications. doi:http://hdl.handle.net/10986/31801
- Li, X.C., Yu, L., Sohl, T., Clinton, N., Li, W.Y., Zhu, Z.L., Liu, X.P., & Gong, P. (2016). A cellular automata downscaling based 1 km global land use datasets (2010-2100). Science Bulletin, 61(21), 1651–1661. https://doi.org/10.1007/s11434-016-1148-1
- Liang, X., Liu, X.P., Li, D., Zhao, H., & Chen, G.Z. (2018). Urban growth simulation by incorporating planning policies into a CA-based future land-use simulation model. International Journal of Geographical Information Science, 32(11), 2294–2316. https://doi.org/10.1080/13658816.2018.1502441
- Linard, C., Tatem, A.J., & Gilbert, M. (2013). Modelling spatial patterns of urban growth in Africa. Applied Geography, 44, 23–32. https://doi.org/10.1016/j.apgeog.2013.07.009
- Liu, Y.L., He, Q.S., Tan, R.H., Liu, Y.F., & Yin, C.H. (2016). Modeling different urban growth patterns based on the evolution of urban form: A case study from Huangpi, central China. Applied Geography, 66, 109–118. https://doi.org/10.1016/j.apgeog.2015.11.012
- Liu, X.P., Hu, G.H., Ai, B., Li, X., Tian, G.J., Chen, Y.M., & Li, S.Y. (2018). Simulating urban dynamics in China using a gradient cellular automata model based on S-shaped curve evolution characteristics. International Journal of Geographical Information Science, 32(1), 73–101. https://doi.org/10.1080/13658816.2017.1376065
- Liu, Z., Schindler, S., & Liu, W. (2020). Demystifying Chinese overseas investment in infrastructure: Port development, the belt and road initiative and regional development. Journal of Transport Geography, 87, 102812. https://doi.org/10.1016/j.jtrangeo.2020.102812
- Lowry, I.S. (1964). A model of metropolis. RAND Corporation.
- Mackett, R.L. (1985). Integrated land use—Transport models. Transport Reviews, 5(4), 325–343. https://doi.org/10.1080/01441648508716610
- McNally, M.G. (2007). The four-step model. In A.H. David & J.B. Kenneth (Eds.), Handbook of transport modelling (Vol. 1, pp. 35–53). Emerald Group Publishing Limited. https://doi.org/10.1108/9780857245670-003
- Meyfroidt, P. (2013). Environmental cognitions, land change, and social–ecological feedbacks: An overview. Journal of Land Use Science, 8(3), 341–367. https://doi.org/10.1080/1747423X.2012.667452
- Miller, J.D. (2013). Infrastructure 2013: Global priorities, global insights. Ernst & Young.
- Moeckel, R. (2018). Integrated transportation and land use models 520 (Washington DC: Transportation Research Board) (Project 20-05, Topic 48-06). http://www.trb.org/Main/Blurbs/177870.aspx
- Moher, D., Liberati, A., Tetzlaff, J., Altman, D.G., & Group, P. (2009). Preferred reporting items for systematic reviews and meta-analyses: The PRISMA statement. PLoS medicine, 6(7), e1000097. https://doi.org/10.1371/journal.pmed.1000097
- Musa, S.I., Hashim, M., & Reba, M.N.M. (2017). A review of geospatial-based urban growth models and modelling initiatives. Geocarto International, 32(8), 813–833. https://doi.org/10.1080/10106049.2016.1213891
- Novin, M.S., & Khosravi, F. (2017). Simulating urban growth by emphasis on connective routes network (case study: Bojnourd city). Egyptian Journal of Remote Sensing and Space Sciences, 20(1), 31–40. https://doi.org/10.1016/j.ejrs.2016.10.001
- NRC. (2014). Advancing land change modeling: Opportunities and research requirements. The National Academies Press. https://doi.org/10.17226/18385
- Omrani, H., Tayyebi, A., & Pijanowski, B. (2017). Integrating the multi-label land-use concept and cellular automata with the artificial neural network-based land transformation model: An integrated ML-CA-LTM modeling framework. GIScience & Remote Sensing, 54(3), 283–304. https://doi.org/10.1080/15481603.2016.1265706
- Ouzzani, M., Hammady, H., Fedorowicz, Z., & Elmagarmid, A. (2016). Rayyan—A web and mobile app for systematic reviews. Systematic Reviews, 5(1), 1–10. https://doi.org/10.1186/s13643-016-0384-4
- Ozturk, D. (2015). Urban growth simulation of Atakum (Samsun, Turkey) using cellular automata-Markov chain and multi-layer perceptron-Markov chain models. Remote Sensing, 7(5), 5918–5950. https://doi.org/10.3390/rs70505918
- Rode, P., Floater, G., Thomopoulos, N., Docherty, J., Schwinger, P., Mahendra, A., & Fang, W. (2017). Accessibility in cities: Transport and urban form. In G. Meyer & S. Shaheen (Eds.), Disrupting mobility: Impacts of sharing economy and innovative transportation on cities (pp. 239–273). Springer International Publishing. https://doi.org/10.1007/978-3-319-51602-8_15
- Roy Chowdhury, R., & Turner, B.L. (2019). The parallel trajectories and increasing integration of landscape ecology and land system science. Journal of Land Use Science, 14(2), 135–154. https://doi.org/10.1080/1747423x.2019.1597934
- Rui, Y., & Ban, Y. (2011). Urban growth modeling with road network expansion and land use development. In Ruas, Anne Advances in cartography and GIScience. 2 (pp. 399–412 https://doi.org/10.1007/978-3-642-19214-2_27). Berlin: Springer.
- Santé, I., García, A.M., Miranda, D., & Crecente, R. (2010). Cellular automata models for the simulation of real-world urban processes: A review and analysis. Landscape and Urban Planning, 96(2), 108–122. https://doi.org/10.1016/j.landurbplan.2010.03.001
- Shaheen, S., Martin, E., & Hoffman-Stapleton, M. (2021). Shared mobility and urban form impacts: A case study of peer-to-peer (P2P) carsharing in the US. Journal of Urban Design, 26(2), 141–158. https://doi.org/10.1080/13574809.2019.1686350
- Southworth, F., & Garrow, L.A. (2010). Travel demand modeling. In J.J. Cochran (Ed.), Wiley encyclopedia of operations research and management science. John Wiley & Sons, Inc 1–13 . https://doi.org/10.1002/9780470400531.eorms0922
- Stead, D., & Vaddadi, B. (2019). Automated vehicles and how they may affect urban form: A review of recent scenario studies. Cities, 92, 125–133. https://doi.org/10.1016/j.cities.2019.03.020
- Terando, A.J., Costanza, J., Belyea, C., Dunn, R.R., McKerrow, A., & Collazo, J.A. (2014). The southern megalopolis: Using the past to predict the future of urban sprawl in the southeast U.S. PLoS ONE, 9(7), e102261. https://doi.org/10.1371/journal.pone.0102261
- Turner, B.L., Lambin, E.F., & Reenberg, A. (2007). The emergence of land change science for global environmental change and sustainability. Proceedings of the National Academy of Sciences, 104(52), 20666–20671. https://doi.org/10.1073/pnas.0704119104
- Turner, B.L., Meyfroidt, P., Kuemmerle, T., Müller, D., & Roy Chowdhury, R. (2020). Framing the search for a theory of land use. Journal of Land Use Science, 15(4), 489–508. https://doi.org/10.1080/1747423X.2020.1811792
- Valencia, V., Levin, G., & Hansen, H. (2020). Modelling the spatial extent of urban growth using a cellular automata-based model: A case study for Quito, Ecuador. GEOGRAFISK TIDSSKRIFT-DANISH JOURNAL OF GEOGRAPHY, 120(2), 156–173. https://doi.org/10.1080/00167223.2020.1823867
- Varquez, A.C.G., Dong, S., Hanaoka, S., & Kanda, M. (2020). Improvement of an urban growth model for railway-induced urban expansion. Sustainability, 12(17), 6801. https://doi.org/10.3390/su12176801
- Von Thünen, J.H. (1966). Isolated state: An English edition of der isolierte staat. Pergamon Press.
- Waddell, P. (2002). UrbanSim—Modeling urban development for land use, transportation, and environmental planning. Journal of the American Planning Association, 68(3), 297–314. https://doi.org/10.1080/01944360208976274
- Wang, W.L., Jiao, L.M., Dong, T., Xu, Z.B., & Xu, G. (2019). Simulating urban dynamics by coupling top-down and bottom-up strategies. International Journal of Geographical Information Science, 33(11), 2259–2283. https://doi.org/10.1080/13658816.2019.1647540
- Wegener, M. (2005). Urban land-use transportation models. In D.J. Maguire, M. Batty, & M.F. Goodchild (Eds.), GIS, spatial analysis, and modeling (1st ed., pp. 203–220). Esri Press.
- Wu, J.J., Li, R., Ding, R., Li, T.F., & Sun, H.J. (2016). City expansion model based on population diffusion and road growth. Applied Mathematical Modelling, 43, 1–14. https://doi.org/10.1016/j.apm.2016.08.002
- Xie, F., & Levinson, D. (2009). Modeling the growth of transportation networks: A comprehensive review. Networks and Spatial Economics, 9(3), 291–307. https://doi.org/10.1007/s11067-007-9037-4
- Yang, X.J., & Lo, C.P. (2003). Modelling urban growth and landscape changes in the Atlanta metropolitan area. International Journal of Geographical Information Science, 17(5), 463–488. https://doi.org/10.1080/1365881031000086965
- Waddell, P., Ulfarsson, G.F., Franklin, J.P., & Lobb, J. (2007). Incorporating land use in metropolitan transportation planning. Transportation Research Part A-Policy and Practice, 41(5), 382–410. https://doi.org/10.1016/j.tra.2006.09.008
- Yang, J.X., Gong, J., Tang, W.W., & Liu, C. (2020). Patch-based cellular automata model of urban growth simulation: Integrating feedback between quantitative composition and spatial configuration. Computers, Environment and Urban Systems, 79, 101402. https://doi.org/10.1016/j.compenvurbsys.2019.101402
- Waddell P, Ulfarsson G F, Franklin J P and Lobb J. (2007). Incorporating land use in metropolitan transportation planning. Transportation Research Part A: Policy and Practice, 41(5), 382–410. 10.1016/j.tra.2006.09.008
- Yao, S., Zhang, F., Wang, F., & Ou, J. (2019). Regional economic growth and the role of high-speed rail in China. Applied Economics, 51(32), 3465–3479. https://doi.org/10.1080/00036846.2019.1581910
- Yu, Y., He, J.H., Tang, W.W., & Li, C. (2018). Modeling urban collaborative growth dynamics using a multiscale simulation model for the Wuhan urban agglomeration area, China. Isprs International Journal of Geo-Information, 7(5), 176. https://doi.org/10.3390/ijgi7050176JL
- Yuan, F. (2010). Urban growth monitoring and projection using remote sensing and geographic information systems: A case study in the Twin Cities metropolitan area, Minnesota. Geocarto International, 25(3), 213–230. https://doi.org/10.1080/10106040903108445
- Zhang, W., & Guhathakurta, S. (2021). Residential location choice in the era of shared autonomous vehicles. Journal of Planning Education and Research, 41(2), 135–148. https://doi.org/10.1177/0739456x18776062
- Zhao, L.Y., & Peng, Z.R. (2012). Land sys: An agent-based cellular automata model of land use change developed for transportation analysis. Journal of Transport Geography, 25, 35–49. https://doi.org/10.1016/j.jtrangeo.2012.07.006
- Zhao, L.Y., & Peng, Z.R. (2014). LandSys II: Agent-based land use forecast model with artificial neural networks and multiagent model. Journal of Urban Planning and Development, 141(4), 1–10 . https://doi.org/10.1061/(ASCE)UP.1943-5444.0000255
- Zhou, L., Dang, X.W., Sun, Q.K., & Wang, S.H. (2020). Multi-scenario simulation of urban land change in Shanghai by random forest and CA-Markov model. Sustainable Cities and Society, 55, 102045. https://doi.org/10.1016/j.scs.2020.102045