ABSTRACT
Modelling has been used extensively by all national governments and the World Health Organisation in deciding on the best strategies to pursue in mitigating the effects of COVID-19. Principally these have been epidemiological models aimed at understanding the spread of the disease and the impacts of different interventions. But a global pandemic generates a large number of problems and questions, not just those related to disease transmission, and each requires a different model to find the best solution. In this article we identify challenges resulting from the COVID-19 pandemic and discuss how simulation modelling could help to support decision-makers in making the most informed decisions. Modellers should see the article as a call to arms and decision-makers as a guide to what support is available from the simulation community.
1. Introduction
COVID-19 looks set to be the worst infectious disease pandemic of a generation in terms of numbers infected, mortalities and the unprecedented demand for healthcare services. To date (March 27 2020) 463,000 people have tested positive for the disease and 20,800 have been recorded as having died (WHO, Citation2020). The economic consequences from organisational shutdowns and other measures taken, such as school closures, are just beginning to become real and estimates suggest that quarantine and social isolation measures could be needed for as long as 18 months, with these being turned on and off during the period based on the observed demand for intensive care unit (ICU) beds (Ferguson et al., Citation2020). Likewise, the detrimental effect on other routine emergency and elective patients will be affected by the health service response to COVID-19, and its impact on these groups should not be ignored.
As the enormity of the threat has become clear, governments appear to have relied heavily on computer simulations to determine how best to reduce its impact, with a particular emphasis on how to flatten the growth curve of the disease to reduce the pressure on the healthcare systems. While considerable efforts have been made on epidemiological modelling of the spread of COVID-19 via computer simulation (e.g., Ferguson et al., Citation2020) the pandemic raises many more challenges that computer simulation could be equally useful in addressing. The modelling and simulation community should now consider their role in contributing to both improving the understanding of the disease and planning to make better decisions and reduce its impact. The modelling environment provides the opportunity to play out different scenarios in silico rather than experimenting on the real population and, in developing the models, often helps to engender a much better understanding of the system as a whole.
This article has two aims. First, to provide a guide to how simulation models and which types of simulation models can be used to support different decisions that arise during a public health emergency such as this one. Second, to act as a call to arms for simulation modellers by providing a research agenda.
One of the key takeaways from this article should be that different decisions require different models and that the field of modelling and simulation is equipped with a range of approaches that can provide support to decisions at different levels of complexity. Modelling approaches can also be connected or combined – for example, forecasts from epidemiological models can and should feed into operational models of hospitals.
Epidemiological models are ideal for predicting the number of new cases or for identifying the best measures to reduce transmission, but they will not help to organise Intensive Care Unit (ICU) beds and medical staff, or consider the interplay of the impact of individuals’ behaviour on the capacity of every essential system, particularly the health care system. Furthermore simulation can also explore questions relating to the disruption of essential supply chains inundated by unprecedented demands (e.g., hoarding food, medicines and hygiene products); and support decisions about how best to move on from the initial isolation period in order to return to business-as-usual. It is clear that models will need to evolve over time as the research questions, the epidemic and the available data change.
In what follows, we provide some initial background information about the current state of the COVID-19 pandemic and an introduction to the key simulation modelling techniques. We then go on to identify a set of problems raised by the COVID-19 pandemic and particularly suited to simulation modelling. For each, we describe the problem, how it might be modelled and any specific data requirements. The article concludes with a research agenda for the simulation modelling community.
2. Background
2.1. COVID-19: Current Knowledge
COVID-19 was first reported to the World Health Organisation (WHO) in December 2019 and is a coronavirus disease caused by severe acute respiratory syndrome coronavirus 2 (SARS-CoV-2). Typical symptoms include a fever, cough and shortness of breath and severe cases develop pneumonia, requiring respiratory support and specialist care in ICU. While there is still some uncertainty, COVID-19 appears to have a basic reproduction number (R0) of 2.4 − 3.3 (Walker et al., Citation2020), while at the same time a large proportion of those infected, estimated to be between 6-10%, become critically ill requiring hospitalisation and access to ventilation support in an ICU (Pagel & Utley, Citation2020). Based on recent research, the overall case fatality rate is estimated as 0.25%–3.0% but this is dependent on the quality of care available (Wilson et al., Citation2020). The risk of developing symptomatic disease and the risk of death following symptoms increases with age (Wu et al., Citation2020). As the epidemic develops, more data are being collected, improving the understanding of the natural history and WHO has set up protocols for data collection to develop a better understanding of the epidemiology.
Recent modelling papers on COVID-19 focus principally on the epidemiology, attempting to both estimate the basic reproductive number of the disease and to provide estimates of the effectiveness of different interventions in flattening the growth curve of the epidemic in order to reduce the burden on the healthcare system. The most popular model for describing the epidemiology is the well-known SEIR model (Susceptible – Exposed – Infectious- Recovered), frequently used at a population level to describe the proportion of the population in each state at any given time. Using data from China, Lin et al. (Citation2020), Fang et al. (Citation2020), and Tang et al. (Citation2020) all use an SEIR model to both describe the epidemic and assess the impact of mass social isolation policies. Hellewell et al. (Citation2020) use a stochastic transmission model to assess the effectiveness of contact tracing and isolation of cases. Both Wells et al. (Citation2020) and Gostic et al. (Citation2020) determine the impact of international travel, with Wells et al. (Citation2020) focusing on how limiting international travel will impact the course of the epidemic, combining data on the probability of transmission with data on global connectivity, while Gostic et al. (Citation2020) estimate the effectiveness of screening of travellers.
2.2. Simulation modelling methods
We consider four main modelling methods in what follows: discrete event simulation, agent-based modelling, system dynamics and hybrid simulation. It is suggested that the choice of modelling method is made based on decision makers’ requirements, type of problem and system complexity and its characteristics (Tako & Robinson, Citation2009, Citation2012; Borshchev & Filippov, Citation2004; Brennan et al., Citation2006; Chahal & Eldabi, Citation2010; McHaney et al., Citation2018).
System dynamics (SD): differential equation based models that represent real world systems in terms of stocks (e.g., of material resources, knowledge, people, money), flows between these stocks, and information that determines the values of the flows (Borshchev & Filippov, Citation2004). Feedback effects and delays are key SD elements used to explain system behaviour. SD was first developed by Jay Forrester in the late 1950’s to help managers better understand industrial problems (Forrester, Citation1961). The best-known within infectious disease epidemiology is the SIR model (Kermack & McKendrick, Citation1927). These models are typically used for strategic decisions or decisions affecting a whole population.
Agent based modelling (ABM): can be used to model the interactions of individuals within a population, allowing a decision-maker to determine how small changes in behaviour and interaction may influence population level outputs. In models of disease spread, the modelling of social networks and spatial movements are also vital for accurately describing transmission and these can be incorporated into ABMs. ABMs are stochastic models, enabling the variability of human behaviour to be incorporated into the model to help understand the variability in the likely effectiveness of proposed interventions. In an opinion piece, Epstein (Citation2009) suggests that ABM is appropriate for modelling pandemics.
Discrete event simulation (DES): stochastic models that take account of variability in the time taken to carry out activities and the times between arrivals into the system. Goldsman et al. (Citation2010) point out that while the roots of DES go back to 1777, DES as we know it today was mainly developed in the years 1945–1970. DES models are typically used to model the operation of systems over time, where entities (people, parts, tasks, messages) flow through a number of queues and activities. They are generally suitable for determining the impact of resource availability (doctors; nurses), on waiting times and the number of entities waiting in the queues or going through the system.
Hybrid simulation (HS): models that combine two or more of the above modelling techniques (Brailsford et al., Citation2019; Mustafee et al., Citation2017). HS is typically used to represent a complex system behaviour where different parts of the system can be better captured by two or more simulation methods.
For all of the modelling methods described above, when simulation models become very complex, they can take a long while to run. Distributed simulation (DS) can be used to either speed up the simulation of a discrete model or link together many simulations to form a single, large-scale simulation. More recently DS has focussed on speeding up simulation experimentation (Taylor, Citation2019). Essentially, this involves the use of multiple computers to run the experiments in parallel. DS tends to play a “supporting role” with other simulation modelling methods (e.g., a potential hybrid large-scale simulation of a citywide Emergency Medical Service (Anagnostou & Taylor, Citation2017), agent-based simulations of epidemics, social interaction and people movement (Bisset et al., Citation2014; Suleimenova et al., Citation2017)).
3. Decisions supported by modelling and simulation
In what follows, we identify a set of crucial decisions that need to be made as the epidemic progresses. These are split into three sections: (1) decisions affecting disease transmission and interventions; (2) decisions regarding resource management; and (3) decisions about care. Obviously, no classification is perfect and several of the decisions we identify here could fit into two or more of these sections. This is not an exhaustive list of decisions that modelling and simulation can support but we hope covers the majority of different decision types.
For each of the identified decisions, we provide a brief description, discuss potential modelling techniques that could be used, and the data that would be needed to address these challenges effectively. We also indicate the geographic and time scales over which the decision is being made. Our geographic scale ranges from global to national to organisational to individual. When defining the time scale, we couch it in terms of the phase of the response that the challenge falls into. Here, we have used the well-known disaster operations management (DOM) framework (Altay & Green, Citation2006) that splits into four phases: mitigation – activities to prevent the onset of disaster or reduce its impact; preparedness – plans to handle an emergency; response – implementation of plans, policies and strategies from the preparedness phase; recovery – long-term planning actions to bring the community back to normality. Note that these definitions differ from those used by the UK government (https://www.gov.uk/government/publications/coronavirus-action-plan/coronavirus-action-plan-a-guide-to-what-you-can-expect-across-the-uk). We also note that with a pandemic, the phases are not as distinct as in other disaster types because of its evolving nature. summarises the information, showing the identified decisions and how they fit into the geographic and time-based scales. In , we list the key modelling methodologies suggested for each decision.
Figure 1. Summary of the key decisions and how they fit into the geographic and time-based scales. As the key below the diagram shows, different colours describe the potential modelling techniques to be used.
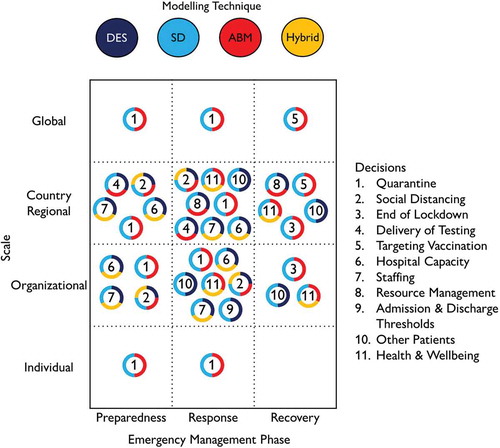
Table 1. Summary of the main modelling methodologies suggested for each of the decisions identified below.
3.1. Decisions affecting disease transmission
This section covers decisions that must be made about interventions to manage the spread of disease throughout the course of the epidemic. The main focus is on quarantine strategies and case isolation, moving from the initial decisions about how these should be imposed to decisions about managing the end of the social isolation measures and the return to normality.
3.1.1. Decision 1: Quarantine strategies and case isolation
Quarantine strategies and case isolation are important at every level (global, national, organisational and individual) and fall into the preparedness and response phases of the disaster response model. The questions vary with different phases and at different levels but the modelling is similar.
When index cases are discovered, contact tracing allows known contacts of these individuals to be traced, quarantined, and isolated from others so that they do not cascade the infection to more people than necessary. A variety of techniques can be used, such as social network analysis, cellphone tracking of movements, etc. On a larger scale, transportation networks globally are being disrupted by national governments in an attempt to reduce transmission between countries, and it seems likely that this will continue in some form in the future. As the epidemic progresses, questions will arise as to which geographical areas should be isolated, and for how long, including questions on the size of the populations to isolate.
WHO included research into “Comparative analysis of different quarantine strategies and contexts for their effectiveness and social acceptability” as one of their key research questions in a recent commentary paper (Bedford et al., Citation2020) and there has been some modelling on this already (Gostic et al., Citation2020; Hellewell et al., Citation2020; Wells et al., Citation2020). While Hellewell et al. (Citation2020) have demonstrated that a Monte Carlo Simulation provides useful results, another method that is commonly used to model the dynamics of a complex system at the global level is System Dynamics. System dynamics can capture the number of people in various states (or stocks in System Dynamics’ terminology) such as incubation and in isolation, as well as the rates that control the flow of people transitioning between states.
Quarantine strategies are used to control one of the flows (i.e. move people to state “in isolation”). For example, Sharareh et al. (Citation2016) demonstrate that quarantine has a significant impact on the spread of Ebola. The simulation models that are used to estimate the impact of quarantine strategies at the global level require many simplifying assumptions such as perfect mixing (i.e. each individual has the same probability to be infected by anyone in the population). While some of these assumptions are accepted to model the impact of quarantine strategies at the global (and large regional) level, they may be unrealistic for the organisational/individual level. For example, the spread of the virus at the local level is affected by networks (e.g., social, public transport). Hence, ABM is more suitable as we can model the interaction between individuals through the networks. Furthermore, ABM can model the heterogeneity of individuals in terms of their characteristics (e.g., age and underlying condition) and behaviour (e.g., compliance to quarantine measure and self-isolation practice). For example, Yang et al. (Citation2011) use an ABM to represent the contact networks and different levels of compliance to quarantine and isolation measures. The experiments show that household quarantine is the most effective measure to control the epidemic in a city. Interestingly, although set for two different spatial levels, the two examples, Hellewell et al. (Citation2020) and Yang et al. (Citation2011) conclude that the effectiveness of quarantine and self-isolation for an epidemic with high reproduction factor is limited.
Healthcare staff, especially those in the front-line, are exposed to the risk of contracting the disease from patients even allowing for protective measures. Quarantine strategies can therefore affect staffing of hospitals and a hybrid model that links an operational DES model of a hospital with an SD or ABM of quarantine strategies may be useful (see Decision 7).
3.1.2. Decision 2: Social distancing measures
Social distancing measures of some form are in force in the majority of countries around the world at this time (March 2020) and recent modelling suggests that this may continue in some form for 18 months. Social distancing is reducing the space between people in order to reduce the spread of the disease and measures include: 1) keeping a distance of two metres (six feet) between people; 2) working from home; 3) cancelling large gatherings of people; 4) closing schools or going to online classes; and 5) visiting relatives and friends electronically rather than physically. In addition to attempting to reduce the overall number of cases of the disease, the goal of social distancing is to flatten the growth curve of the disease in order to not overload the healthcare system. Flattening the curve provides additional time to add resources (e.g., respirators, medical staff, etc.) to handle the increased burden on the healthcare system.
Modelling of social distancing and quarantining (see Decision 1) can be treated as a spatial transmission problem (Robertson, Citation2019) with a variety of modelling techniques available. This can be done at a local level using a SD model or by modelling individual agents in the population using ABM, including behavioural rules on their movement and hence spread of the disease. Incorporating behavioural responses is important as all countries have seen unwanted reactions to the social distancing measures, e.g., full beaches, large numbers of people in parks. Each individual may believe that they are following the government advice but as a whole, the societal effect may not actually have reduced transmission and may have spread the infection more widely. An interesting question for these behavioural models is to determine how behaviour varies between different global regions. For example, population flow between provinces in China is likely to follow different patterns from movement between states in the USA or countries in Europe. Also, different populations may have different social connectivity within local areas. Incorporating data on social contact patterns into transmission models (e.g., Mossong et al., Citation2008 who carried out a large-scale, prospectively collected, population-based survey of epidemiologically relevant social contact patterns in eight different European countries) may help to explain variations in rates of disease transmission and suggest how different control measures will perform more or less effectively in different countries.
One of the key social distancing strategies is educational closures. In the UK, school closures are mainly at the national level at present but as the epidemic progresses may become more focused at the organisational level. In the US, school closure decisions are more often made at the local (or state) level. These, and identifying the most effective social isolation strategies, are important in the preparedness and response phases. Data from countries implementing these strategies as to how they affect public behaviour will be vital in managing later stages of the epidemic and in learning for potential future epidemics.
Universities have student and staff populations with significant social contact within institutional boundaries. Universities have the ability and responsibility to administer policies to foster social distancing while providing medical and housing services to students. A university health services office typically provides the primary healthcare services on a campus and collaborates with other external healthcare organisations and emergency personnel. University administrators are responsible for critical decisions during a pandemic, including cancellation of classes, closure of research facilities and communication with university populations. Owing to the frequency of international travel and the high density of students and faculty on campus, university populations can have a large impact on the spread of infectious diseases within a community.
Many universities have developed pandemic influenza emergency response plans in an attempt to control a potential outbreak and balance the financial, operational and public health consequences of a pandemic, but most are quite simple. Some universities, including Arizona State University, have developed more sophisticated plans based on the use of modelling and simulation (as described in Araz et al. (Citation2011)). The objective of these plans is to control the pandemic through proper actions and appropriate policies to reduce the spread of the disease while still maintaining essential university services. Simulation models that address the population dynamics with the disease characteristics are useful for identification of preferred policies, improving understanding of consequences of policy decisions, and covering gaps in emergency response plans and public health policies. It is possible to simulate the disease spread for a university along with the policies that the university developed for their response to pandemic influenza (Araz et al., Citation2011). Since the university population is formed by several groups of people (faculty, staff, students living on campus, students living off campus, etc.), the model should include the whole university population in terms of several subpopulations based on their different roles, responsibilities and behaviours. Because the social distancing and isolation policies will force all individuals on campus to have different mixing rates with different individuals at various locations on and off campus, new subpopulations after social distancing policies are activated including evacuated students, students on campus after evacuation, evacuated faculty and staff, students in infirmary, overflow infirmary (if infirmary capacity is exceeded). The model divides the population into several compartments (susceptible, exposed, infected and removed), and based on the defined rates it moves individuals from one compartment to another.
The disease dynamics start with a number of infectious individuals introduced into community and the rest of the population is assumed to be susceptible. Susceptible individuals have random mixing with infectious individuals and they become exposed to disease. Exposed individuals are typically assumed to be infectious and asymptomatic. After a certain time period (incubation period) these individuals start showing symptoms and continue being infectious. Finally, after the completion of the infection period, individuals either recover or die. The simulation is used to compare the spread of the disease with and without school closure. The difficult part of the exercise is to accurately predict the parameters of the model including the contact rate, infection rate, incubation period, mortality rates, etc.
School closures for primary and secondary schools have the same goals, but generally do not have to be concerned about having students on campus after classes are suspended or moved to an online format, with the possible exception of boarding schools. On the other hand, there are some different challenges, including the impacts of alternative education delivery services and the impacts on both students and their parents (Araz et al., Citation2012; Araz et al., Citation2013). In the US, children from low income families are eligible to receive free lunches and when schools are closed they may miss meals. Parents that must work outside the home are also forced to choose between going to work and staying at home to supervise their children. This can impact on the staff available in key roles such as the health service and transportation. Balancing the need for vital staff with the detrimental effect of social mixing within school communities is a key decision for governments. Modelling to address this challenge may begin with a strategic overview using an SD model but there is a real need to link models of disease spread with operational models describing the numbers of staff needed to maintain the necessary health and other services. These are typically DES models, or potentially hybrid models that link SD models of disease spread with the DES operational models.
3.1.3. Decision 3: How to manage the end of lock down
As the impact of social distancing or lock down measures takes hold and the number of new cases falls, governments will need to make a decision about when and how to reduce restrictions in order to avoid the number of cases occurring during secondary epidemics again exceeding the capacity of ICU departments. Initial modelling suggests waiting until ICU admissions from COVID-19 dip below a threshold value to relax the restrictions, bringing them back into force when cases exceed a given value (Ferguson et al., Citation2020), and continuing until a vaccination has been developed. This has implications at country, regional and organisational levels and falls into the recovery phase.
Decisions to be made include the threshold values for when to relax restrictions and when to re-impose them; which social distancing measures to maintain and which to relax during the interim periods; and how resources might be managed across countries and even worldwide as different regions employ these strategies at different times. Inputs to the model will include results of other modelling studies indicating the supply of resources (principally ICU beds with the trained staff needed to monitor critically ill patients and respiratory equipment) and the likely usage (discussed in the following section) as well as the impact of social distancing measures on the general health and well-being of the population. There could also be a link with the testing strategy (Decision 4), which may impact on how social isolation measures are relaxed.
Different models could work well here to help answer subtly different questions. An SD model would provide a strategic view of the system, allowing competing demands to be taken into account, while an ABM would allow individual behaviour to be accounted for. Determining how best to manage the end of the social distancing measures is well-suited to so-called optimisation via simulation techniques (Fu, Citation2015); and, if data are sufficiently accurate and timely, using some of the more novel methods in real-time optimisation and digital twins to finely control the isolation measures could work well (Xu et al., Citation2016). Colbourn (Citation2020) discusses the potential impacts of the timing of relaxing social distancing measures in Hubei, China.
3.1.4. Decision 4: Delivery of testing
The delivery of testing could also fall into the following section where we discuss the management of resources. We put it here as testing, with accompanying isolation, is a valuable intervention against the spread of the virus. These decisions fall into the preparedness and responses phases and tend to be made at the national level, partly due to the connectivity between the population within a country.
Testing is important to identify how widespread the infection is. For this purpose, the delivery of widespread testing and isolation strategies seems to have worked well in South Korea and Iceland, although to date little scientific literature exists to justify these claims. There are two clear roles for modelling here.
Demonstrate the effectiveness of mass testing and isolation strategies to determine how they compare with other prevention interventions such as those observed in other countries. For large-scale models, SD would work well but ABM is likely to prove most effective at generating a nuanced answer.
Determine demand and efficient procedures for rolling out such a large-scale programme nationwide, including supply chain questions. DES is typically used for modelling operations of this nature.
If large-scale testing is considered infeasible, using ABM to determine how best to allocate a limited number of tests would be beneficial. Optimisation via simulation techniques can be used to find the optimal strategy. Modelling should also account for the imperfect sensitivity and specificity of tests. Initial reports from Wuhan suggest that the standard RT-PCR test only detected 59% of patients with COVID-19 after one test, with this rising following subsequent tests (Ai et al., Citation2020). More recent articles suggest that newly developed rapid tests (IgM-IgG combined antibody test) have better sensitivity and specificity (88.7% and 90.6% respectively) and a faster turnaround of results. Accounting for these potential inaccuracies in the simulation model is straightforward and examining how an inaccurate test affects disease transmission is a useful question.
Testing has another role to play in terms of ensuring that key staff are able to return to work quickly and we discuss this further in Decision 7. Home or mobile testing kits can be used to reduce the burden of ambulance use and to avoid unnecessary hospital visits. This is particularly useful to avoid such incidents as taking ambulances out of service for decontamination after carrying potential cases to hospital for testing, where the decontamination can take up to eight hours. DES can be used to estimate the impact of home testing and testing staff on capacity for care.
As the epidemic progresses, determining the percentage of the population who have been exposed to the virus will help considerably in determining future interventions. In this case, a different form of testing is needed – to determine who has been exposed to the virus rather than who is currently suffering from it. This benefit needs to be factored in when deciding the optimal strategy for testing delivery.
3.1.5. Decision 5: Targeting vaccination
Like testing, the delivery of vaccinations raises many operational decisions and modelling studies exist for how vaccination programmes can be run efficiently (e.g., Lee et al., Citation2010; Özaltin et al., Citation2014). ABM seems most likely to work well at modelling these decisions (e.g., Lee et al., Citation2010; Özaltin et al., Citation2014), although SD could also be beneficial (e.g., Araz, Citation2013; Pruyt & Hamarat, Citation2010). In a new epidemic scenario, where vaccinations will become available during the epidemic and it will take some time to ramp up production, there is also a need to target the initial vaccinations at sectors of society where they will have the most impact. For COVID-19 this will form part of the recovery phase with decisions likely being made on a national (and possibly global) scale. The strategy for vaccination delivery affects the decision to manage the end of lock down (Decision 3).
3.2. Decisions regarding resource management
Management of resources is vital in dealing with the implications of the COVID-19 epidemic. Principally, we consider medical resources: hospital beds, respiratory equipment and staffing, but we also discuss the supply of wider resources such as food, pharmaceutical products, protective equipment, testing kits and vaccinations.
3.2.1. Decision 6: Capacity of inpatient hospital beds and critical care
Decisions over the capacity of hospital beds both in critical care and on standard wards form part of the preparedness and response phases of the epidemic and are likely to be made on a large scale nationally and at an organisational level as the epidemic continues.
High COVID-19 disease transmission rates and a relatively high percentage of patients requiring medical care together pose an unprecedented pressure on health service providers, as it presents a large demand for critical care resources that exceeds their capacity. Critical care resources including critical care beds and ventilators, are limited within most hospitals in the UK, USA and Europe. Italy for example, has been experiencing extreme stress on its intensive care system (Grasselli et al., Citation2020; Remuzzi & Remuzzi, Citation2020), with patients being unable to access critical care beds and dying in hospital corridors while waiting for beds to be released. The same is starting to be experienced in the UK at the time of writing this article (March 2020), with critical care services expected to be overwhelmed, due to an upsurge of admissions in London hospitals. In normal operating conditions, Intensive Care Units (ICU) are most efficient if operated at around 70-75% capacity (Tierney & Conroy, Citation2014) but this does not leave sufficient spare capacity to cater for the increased demands of COVID-19 patients requiring intensive care support. Hence, national healthcare services and individual hospitals are required to optimise the use of these resources and to create additional capacity in order to respond effectively to the unprecedented demand they are faced with. For this, Public Health England has established a COVID-19 Hospitalisation in England Surveillance System (CHESS) to record daily data on COVID-19 patients and critical care utilisation, which can be used to forecast and estimate the utilisation of health services (PHO, Citation2020).
Simulation models can effectively support decisions taken at national (governments), inter organisational (local governments or municipalities) and organisational level (individual hospitals) to create capacity and manage scarce resources for the effective care of critically ill patients. What-if scenarios can be implemented to evaluate two types of decision policies on the ability of the healthcare system capacity to cope with expected demand.
Creating extra bed capacity, such as decisions to repurpose wards from other hospital units or private hospitals; conversions of non-hospital facilities such as hotels; cancellation of scheduled operations; earlier discharge of patients in the community.
Managing demand for services, through interventions such as reducing the height of the peak cases and pushing it further into the future; rationing care, triage protocols that aim to optimise the availability of intensive care beds (Pagel & Utley, Citation2020).
These models can play a crucial role in the preparedness phase to inform plans to respond to the pandemic and later in the response phase they can be further updated and calibrated to support the implementation of plans at shorter timescales.
At hospital level, DES models can represent the stochastic arrival and flow of patients in ICU departments, based on expected infection rates and length of stay. Model outputs could provide estimates of the number of resources required and their utilisation levels, including ICU beds, ventilators, and personal protective equipment (PPE), the number of patients expected to die waiting for care, and the number of patients that can be treated. DES has been widely used to model ICU in hospitals (e.g., Bai et al., Citation2018) to consider primarily issues regarding ICU capacity problems (Griffiths et al., Citation2010; Litvack et al., Citation2008; Ridge et al., Citation1998; Zhu et al., Citation2012). SD has also been used to model acute patient flows, in workshops with healthcare stakeholders, to improve patients’ experience within the system (Lane & Husemann, Citation2008).
Similarly, DES models can model the overall ICU resource requirements at national level, aggregating data from individual hospitals. In this area, the use of hybrid simulation that combine SD models for describing the progression of the epidemic and DES models for describing the workings of the hospital system can be invaluable. Such hybrid models have been used previously to model the management of chlamydia infections (Viana et al., Citation2014).
3.2.2. Decision 7: Staffing
Staffing decisions tend to be made at the organisational level with only generic policy being made at the national level (e.g., the initiative in the UK to bring back retired health workers). These decisions are made in the preparedness and response phases of the epidemic but it could be argued that such modelling should have taken place during the mitigation phase to ensure that sufficient trained staff were available to cope with an emergency such as that we are experiencing now. In the UK, this capacity decision is made at the national level.
Looking at a hospital level, DES modelling is particularly beneficial for identifying the best mix of staff to have within a workforce, taking account of staff sickness and differing resource needs of patients. DES has already been used to investigate staff planning in ICU (Griffiths et al., Citation2005). More strategic models may be better described using SD to represent workforce availability at a national level, accounting for aspects such as stress in medical staff due to work overload, staff infection rates and time off work.
A particular question that needs to be answered during an infectious disease epidemic is how to ensure that key staff such as frontline healthcare staff can return to work quickly following an illness. A testing strategy that can allow key staff to return to work sooner after an illness or a suspected case within their household, has the potential to increase the number of staff working significantly (see Decision 1). Similarly, when a vaccination becomes available, careful planning of vaccinations among staff could ensure a greater number remain healthy and able to work (see Decision 5). School closure (see Decision 2) may also affect the staff availability if they have to stay at home with their children. One way to mitigate this, as shown in the UK, is to provide special treatment for the children of key workers to allow them to go to school. Modelling could help in two ways here. First, to show the benefits of rapid testing or vaccination of staff and second to determine the most effective way of doing this. Operational models, using DES, would work well in both cases.
Of potentially equal importance is maintaining the mental health of front line staff. While difficult to model explicitly, it can be accounted for when setting up potential operating strategies, e.g., ensuring that schedules allow for adequate breaks, provision of occupational health support.
The epidemic has necessitated changes in working practices among the whole population and in particular in healthcare settings there has been an increase in the use of telemedicine, where patients are either triaged or consulted with over the phone and/or via video conferencing software. This will have an impact in the healthcare system and resource needs of providers in the aftermath of the pandemic, in the recovery phase and beyond. It may also change the way that demand arrives into the healthcare system. Operational modelling, typically DES, will help with a transition to a system in which more primary care is delivered remotely.
3.2.3. Decision 8: Management of resources within a region
As the COVID-19 pandemic spreads in different geographical regions, a coordination of sharing mobile medical resources such as staff and PPE may be required within countries. Resources may be repurposed from areas that are past the peak to areas that are at an earlier phase in the epidemiological spread to support the response to changing demand in areas most affected by the virus. Operational planners need to know the best mechanism to adjust to jumps in both supply and demand of resources, and need techniques to optimise the distribution networks to take account of changes in demand. Such decisions will be made during the response and recovery phases of the epidemic, most likely at a national or regional level. There is also a need during a severe epidemic to manufacture more medical equipment. In the case of COVID-19 there is increased demand for PPE for health service workers; respiratory equipment; testing kits and hand gel. When a vaccination has successfully passed clinical trials there will also be a need for its rapid rollout globally.
The food sector has seen changes in demand for different food items such as pasta, rice, etc. in the supply chain. Responding to this demand quickly and forecasting how it will vary as the epidemic progresses is a key modelling question. The famous beer game is a typical example of a SD model that shows how small variations in customer demand can increase exponentially upstream the supply chain (Sterman, Citation1992). SD would be a suitable method to model the supply chain at global or national level to support decisions regarding order and production quantities at supplier and manufacturer level, as well as restrictions imposed to ensure customer demand for these basic supplies is effectively matched during the epidemic.
Logistics modelling using DES or SD is also needed to satisfy the demand for deliveries among members of the public who are self-isolating. For example, Ivanov (Citation2020) builds a DES model to predict the impact of epidemic outbreaks on global supply chains. Behavioural modelling (typically using an ABM framework) could also be beneficial in determining how best to limit buying within the community. The latter is particularly important in helping to reduce transmission by reducing the queues within shops and the need to visit several shops to obtain essentials.
3.3. Decisions about care
There are many decisions that must be made about the care of individuals as a result of the COVID-19 epidemic. Here, we consider decisions that affect the care of a group of individuals or individual patients, using modelling to determine their impact on the system as a whole. For example, medical decisions on admissions and discharges of patients may change when health services are overwhelmed and determining the wider impact is important. We must also consider the impact on non-COVID-19 patients and how the epidemic may have implications on other health indicators such as mental health and wellbeing.
3.3.1. Decision 9: Investigation of the thresholds for admission and discharge of patients
We consider here admission and discharge of all patients, not just those with COVID-19. Admission and discharge from hospital is usually calibrated against health service capacity taking account of patient needs and, under normal operations, the flow of admissions into a hospital is of the same order as the flow of discharges. The majority of hospitals will have plans in place to adapt to changes in demand and staff availability such as those observed during a pandemic, e.g., the Operational Pressures Escalation Levels (OPEL) Framework in the UK (NHS England, Citation2018). When under the most extreme pressures, hospitals will aim to discharge as many patients as possible (“clear the decks” strategies) and to cancel routine procedures. Changing thresholds for admission and discharge will likely have a negative impact on other patients and this must be balanced against the benefit of additional beds for COVID-19 patients. DES and SD are both beneficial here to provide operational and strategic viewpoints respectively.
Principally when deciding on admission to ICU, but also relevant in other areas of the hospital is the idea of triaging. Pagel and Utley (Citation2020) discuss the difficulties of triaging for an ICU, showing that thought needs to be given both to length-of-stay and patient need when making decisions over who to admit in a situation where there are insufficient beds to meet demand.
3.3.2. Decision 10: Minimising the impact on other patients
During the pandemic there will be a large number of services that care for vulnerable and unwell members of society that will come under considerable pressure. Indeed a major concern within a pandemic is that overloaded health systems result in high mortality in patient cohorts both with and without the infectious disease. The latter is caused by difficulties accessing health services. Patients needing transport to clinics for regular life-critical treatment are likely to be amongst this vulnerable group. Patient transport providers and suppliers of regular medical services, e.g., dialysis, need to reorganise their services to protect patients from potential infection.
DES has a long history of success in improving the efficiency of routine health service delivery in areas such as whole hospital flow (Ben-Tovim et al., Citation2016; Günal & Pidd, Citation2011); blood and transplant supply chains (Katsaliaki & Brailsford, Citation2007; Osorio et al., Citation2017); and discharges from acute to the community (Penn et al., Citation2019). DES must now go further and support analyses of health system reconfiguration under pandemic conditions.
An example of a group at risk are people suffering from acute kidney injury or chronic kidney disease. These patients require regular dialysis (a medical process to replace the function of the kidney in filtering toxins from the blood) and are at high risk of developing further complications from COVID-19. Renal services need to determine both how to manage COVID-19 negative, suspected, positive, and recovered patients and, as the pandemic progresses, to understand the consequences of rationing care. Modelling could investigate how best to separate patients to avoid transmission (either by time or by using different facilities) using DES, and how changing the frequency of dialysis will affect outcomes.
3.3.3. Decision 11: Health & well being
Health and well-being decisions can arise at national, regional and individual levels during the response and recovery phases; it would however be useful to put policies in place in the mitigation phase as communities prepare for the onset of the pandemic.
Due to the lock-down measures taken in different geographical regions and countries and the psychological impact caused due to the health risks associated with COVID-19, an imminent issue to be considered is the impact this could have on the mental health and well-being of members of the public and the repercussions on mental health at individual level. Lack of (or limited) physical exercise and lack of social contact can impact people’s mental health. In addition, high levels of anxiety and concerns about health, loss of income and jobs can in turn result in mental health problems and other societal problems leading to use of drugs and criminality. Other health and social care services, such as counselling and occupational therapy, psychology and psychiatry could play a crucial role in supporting individuals during the pandemic and the recovery phase. Such decisions can be overlooked during the pandemic as they are considered of a lower priority compared to saving the lives of those critically ill. However, if plans and measures to reduce these adverse effects are made during the mitigation phase, these could support public health quality and the take up of social distancing measures discussed above (Decision 2).
SD can be used to model the public’s mental health and to understand the impact that support measures and care resources can have at a community level. A small number of SD models have been used to evaluate public health policies for the management of mental health (Langellier et al., Citation2019; Rutter et al., Citation2017), however there are opportunities to extend modelling efforts in this area, especially in community-based SD. HS models that combine SD with ABM to represent behavioural aspects related to social distancing would be also useful.
4. The call to arms
The challenges we face as a result of COVID-19 will evolve over the next months and possibly years, as will the modelling that is needed. The paper has outlined some preliminary ideas for decisions that can be supported by the simulation community. The quantitative approaches that we suggest have the potential to inform policy leaders needing to make scarce resources go further, identify weaknesses in the system and potentially save more lives. The aim of this paper is to bring the community together and to create momentum, as well as capacity, to better deal with disasters such as COVID-19 by shaping, sharing and supporting good practice, good models and research findings. This is our call to arms.
A number of opportunities will emerge for the modelling community to support the efforts made globally and locally to deal with the phases of the unfolding disaster brought on by the COVID-19. Optimising our approach and engagement at this critical time could mean that we are able to support more decisions and make the most of this specialist community. The following paragraphs outline some general issues that we need to address as a modelling community so that we can rise to the challenge of COVID-19 and other future disasters.
4.1. Bringing the community together
Bletchley Park in the UK was the home of the WW2 codebreakers made famous through the work of Alan Turing. It offered a physical setting for teams from diverse backgrounds to collaborate on wicked coding problems emerging from the war, such as cracking the Enigma. Whilst the majority of codebreakers were British, other allied forces were also present at Bletchley. What this shows is that good teams help to solve difficult problems. In this pandemic, the teams are, by necessity, virtual and, due to the global reach of COVID-19, international. This presents challenges and opportunities, with the principal challenge being the need for a lead within the community to bring it together to solve the right problems.
Opportunities exist for learning from regions with more advanced epidemics. Data has been shared effectively during the COVID-19 pandemic but developing clear methodologies for fast updating of models within the community would be beneficial for any future crises. This could be helped significantly by ensuring that all of the modelling that gets carried out for government departments or for individual hospitals is made public and reproducible using standardised guidelines (e.g., STRESS guidelines for simulation; Monks et al., Citation2019) and Open Science practices (Taylor et al., Citation2017). Standardisation allows other experts to comment and refine the model assumptions or update them as new data become available. It will also enable models to easily be reused and avoid reinventing the wheel. Openness and transparency of the modelling is important and ideally one might hope that a model repository is created alongside a data repository.
4.2. Managing uncertainty in models
One related issue to transparency is providing an effective description of the uncertainty inherent in the results of simulation models, which is often not understood by those using them in their decision-making. Simulation models are often based on input data that are still very uncertain or missing and in the case of a fast-moving pandemic, inputs may be based only on confirmed cases (Roda et al., Citation2020). Similarly, uncertainties in how the disease spreads could mean that the logic used is not completely verified. To mitigate this for COVID-19 or responding to other time-critical situations, we would advise keeping prediction horizons short, updating parameters and model logic as more data comes in and rerunning models frequently.
Modellers also have a responsibility to highlight the uncertainty in the outputs from simulations. This uncertainty is not always obvious, particularly in sophisticated models and can lead to misplaced confidence. Two kinds of uncertainty exist in simulation models: input uncertainty, which describes our lack of knowledge of the true parameters of models (Xiao et al. (Citation2020); and intrinsic variation in processes, e.g., travel times and durations of treatment (Barton & Nelson, Citation2013). In addition, as soon as the situation moves outside the tested domain of the model, the model outputs will become much less reliable. Uncertainty should be recognised and discussed with all stakeholders, including clinicians, decision-makers and the public and, when there is a high level of uncertainty, focusing on conservative or robust solutions seems the best policy. Identifying important sources of input uncertainty of models will also help to prioritise data collection.
4.3. Engaging effectively with policy makers and experts in other disciplines
Research in health simulation has advocated stakeholder involvement in simulation studies (Eldabi et al., Citation2007; Fone et al., Citation2003). Dealing with COVID-19 will require several decision makers, with a different understanding of the situation and/or expertise and/or power to actively engage with the modelling process to have an input into the design of the model or just to engage with the model for the purpose of exploring scenarios. Involving stakeholders in the modelling process will also help to avoid the potential problem of having several competing models for a decision-maker to choose between.
Two issues arise from this need to engage others. The first issue is that whilst facilitated simulation modelling in healthcare has received some attention (Tako & Kotiadis, Citation2015; Kotiadis et al., Citation2014; Richardson & Andersen, Citation1995; Robinson et al., Citation2014) this has not been addressed when using ABM or HS and we have less of an understanding of how to engage multiple people in their design. Furthermore, the engagement has been in face-to-face workshop environments and COVID-19 will require remote meetings that may not be suited to the current tools; for example, developed for Participatory Simulation (PartiSim) (Tako & Kotiadis, Citation2015). New collaborative simulation practices that can support virtual meetings will need to be developed.
Appropriate engagement will require a good communication of the problem situation and that could be done with the support of a problem structuring method (Mingers, Citation2001) but there is still a need to translate them for a virtual environment and a need for training modellers in soft skills. The modelling and simulation community has generally approached modelling in the expert mode (favouring observation and data driven models) rather than the facilitated mode of interaction (favouring expert views in addition to data), which would necessitate some collaboration with facilitators and problem structurers or upskilling the modelling and simulation community.
4.4. Rapid development of conceptual models
The rapid onset of the COVID-19 pandemic has meant that models are needed quickly. This could be achieved through rapid modelling or by repurposing existing models, e.g., adjusting existing epidemiological models for other diseases to COVID-19. Conceptual modelling has been described in one of the key stages in the modelling process and should be the initial stage of any simulation study (Robinson, Citation2008). This precoding stage, undertaken by a modeller, involves finding out about the problem situation, determining the objectives, inputs, outputs and model content. It is essentially the design stage of a simulation study.
Currently this is the stage potentially leading to delays for COVID-19 as it involves the modeller liaising with busy stakeholders and accessing data and other relevant information that may be hard to obtain. Hence it might be more efficient if templates are created that can be used by stakeholders to detail essential information to speed up the commissioning of models and the potential to source more than one model for a particular problem situation. Doing this in advance could also help to highlight which data are crucial to the development of a particular model, enabling data collection protocols to be developed. This could also mean quicker repurposing of existing models. Kotiadis et al. (Citation2014) propose the use of facilitated modelling to support stakeholder involvement but this approach still requires modellers to lead the process.
Considering the limited timelines required to support decisions during the response phase of the pandemic, modellers should consider designing the simplest possible models. Careful model abstraction during conceptual modelling can reduce model complexity to develop simple models that are suitable for the purpose they are built for (Robinson et al., Citation2014). We appreciate that not all the decisions presented in this paper can be represented by simple models; however, where possible we call for modellers to consider simplifying their requirements and assumptions in their models, to enable rapid model development.
4.5. Developing research and tools to support the mitigation phase
We considered the decisions that could be explored for COVID-19 by the modelling and simulation community in its four phases of mitigation; preparedness; response and recovery (Altay & Green, Citation2006; Amideo et al., Citation2019) but given the time at which we are writing the article, none of our decisions appear in the mitigation phase: this time has already passed. In places, we have identified decision areas where modelling could support the mitigation phase by considering developing models for stress testing of operational readiness. This is envisaged as model-based game playing by decision-makers – war games (see Araz et al., Citation2012). It is also important for the models to be created during the mitigation phase because managers would not have much time to engage in model building activities when they are busy dealing with a crisis. Ideally during the crisis, they can use these ready-made models to support their decision making. Even though managers would be busy, remote model building practices could be developed to enable their effective engagement with the models and the modelling team as discussed earlier.
4.6. Immediate priorities
For the current pandemic, models will be needed for managing the exit from the lock-downs globally without overloading hospitals with a second (hopefully much reduced) peak of infections. There is also a need to update models of ICUs and hospitals in general to take account of the new threat of corona viruses based on what we have seen here. Our health facilities may need to be resourced differently if this virus is likely to persist in the next few years. If the positive talk of a vaccine is true then determining who to vaccinate first and how to roll out a population-wide vaccination programme will also be vital; these decisions can definitely be supported by modelling and simulation.
4.7. Concluding remarks
There are clearly a host of research questions raised by the COVID-19 pandemic and we do not assume that we have identified all of them. Funding is needed for modelling studies to help with improving and focusing the response to this deadly virus and those that will occur in the future. The number and scale of the decisions identified here suggests that there may not be sufficient modellers to answer all of them effectively and thoroughly, and it is important that we choose what we work on carefully and share findings and models quickly via our international networks in order to maximise the benefits of simulation modelling to reducing the impact of COVID-19 globally.
Disclosure statement
No potential conflict of interest was reported by the authors.
References
- Ai, T., Yang, Z., Hou, H., Zhan, C., Chen, C., Lv, W., Tao, Q., Sun, Z., & Xia, L. (2020). Correlation of chest CT and RT-PCR testing in coronavirus disease 2019 (COVID-19) in China: A report of 1014 cases. Radiology, 200642. In Press. https://doi.org/10.1148/radiol.2020200642
- Altay, N., & Green, W. G. (2006). OR/MS research in disaster operations management. European Journal of Operational Research, 175(1), 475–493. https://doi.org/10.1016/j.ejor.2005.05.016
- Amideo, A. E., Scaparra, M. P., & Kotiadis, K. (2019). Optimising shelter location and evacuation routing operations: The critical issues. European Journal of Operational Research, 279(2), 279–295. 0377-2217. https://doi.org/10.1016/j.ejor.2018.12.009
- Anagnostou, A., & Taylor, S. J. E. (2017). A distributed simulation methodological framework for OR/MS applications. Simulation Modelling Practice and Theory, 70, 101–119. https://doi.org/10.1016/j.simpat.2016.10.007
- Araz, O., Jehn, M., Lant, T., & Fowler, J. W. (2012). A new method of exercising pandemic preparedness through an interactive simulation and visualization. Journal of Medical Systems, 36(3), 1475–1483. https://doi.org/10.1007/s10916-010-9608-7
- Araz, O. M. (2013). Integrating complex system dynamics of pandemic influenza with a multi-criteria decision making model for evaluating public health strategies. Journal of Systems Science and Systems Engineering, 22(3), 319–339. https://doi.org/10.1007/s11518-013-5220-y
- Araz, O. M., Lant, T., Fowler, J. W., & Jehn, M. (2011). A simulation model for policy decision analysis: A case of pandemic influenza on a university campus. Journal of Simulation, 5(2), 89–100. https://doi.org/10.1057/jos.2010.6
- Araz, O. M., Lant, T., Fowler, J. W., & Jehn, M. (2013). Simulation modeling for pandemic decision making: A case study with bi-criteria analysis on school closures. Decision Support Systems, 55(2), 564–575. https://doi.org/10.1016/j.dss.2012.10.013
- Bai, J., Fügener, A., Schoenfelder, J., & Brunner, J. O. (2018). Operations research in intensive care unit management: A literature review. Health Care Management Science, 21(1), 1–24. https://doi.org/10.1007/s10729-016-9375-1
- Barton, R. R., & Nelson, B. L. (2013). Quantifying input uncertainty via simulation confidence intervals. INFORMS Journal on Computing, 26(1), 74–87. https://doi.org/10.1287/ijoc.2013.0548
- Bedford, J., Enria, D., Giesecke, J., Heymann, D. L., Ihekweazu, C., Kobinger, G., Clifford Lane, H., Memish, Z., Oh, M., Alpha Sall, A., Schuchat, A., Ungchusak, K., & Wieler, L. H. (2020). COVID-19: Towards controlling of a pandemic. The Lancet, 395(10229), 1015–1018. In Press. https://doi.org/10.1016/S0140-6736(20)30673-5
- Ben-Tovim, D., Filar, J., Hakendorf, P., Qin, S., Thompson, C., & Ward, D. (2016). Hospital event simulation model: Arrivals to discharge–design, development and application. Simulation Modelling Practice and Theory, 68, 80–94. https://doi.org/10.1016/j.simpat.2016.07.004
- Bisset, K. R., Chen, J., Deodhar, S., Feng, X., Ma, Y., & Marathe, M. V. (2014). INDEMICS: An interactive high performance computing framework for data intensive epidemic modeling. ACM TOMACS, 24(1), 4. https://doi.org/10.1145/2501602
- Borshchev, A., & Filippov, A. (2004, July 25-29). From system dynamics and discrete event to practical agent based modeling: Reasons, techniques, tools. Proceedings of the 22nd International Conference of the System Dynamics Society. https://proceedings.systemdynamics.org/2004/SDS_2004/PAPERS/381BORSH.pdf
- Brailsford, S. C., Eldabi, T., Kunc, M., Mustafee, N., & Osorio, A. F. (2019). Hybrid simulation modelling in operational research: A state-of-the-art review. European Journal of Operational Research, 278(3), 721–737. https://doi.org/10.1016/j.ejor.2018.10.025
- Brennan, A., Chick, S. E., & Davies, R. (2006). A taxonomy of model structures for economic evaluation of health technologies. Health Economics, 15(12), 1295–1310. https://doi.org/10.1002/hec.1148
- Chahal, K., & Eldabi, T. (2010). A multi-perspective comparison for selection between system dynamics and discrete event simulation. International Journal of Business Information Systems, 6(1), 4–17. https://doi.org/10.1504/IJBIS.2010.034001
- Colbourn, T. (2020). COVID-19: Extending or relaxing distancing control measures. Lancet Public Health, 1–2. https://doi.org/10.1016/S2468-2667(20)30072-4
- Eldabi, T., Paul, R. J., & Young, T. (2007). Simulation modelling in healthcare: Reviewing legacies and investigating futures. Journal of the Operational Research Society, 58(2), 262–270. https://doi.org/10.1057/palgrave.jors.2602222
- Epstein, J. M. (2009). Modelling to contain pandemics. Nature, 460(7256), 687. https://doi.org/10.1038/460687a
- Fang, Y., Nie, Y., & Penny, M. (2020). Transmission dynamics of the COVID‐19 outbreak and effectiveness of government interventions: A data‐driven analysis. Journal of Medical Virology. https://doi.org/10.1002/jmv.25750
- Ferguson, N., Laydon, D., Nedjati-Gilani, G., Imai, N., Ainslie, K., Baguelin, M., Bhatia, S., Boonyasiri, A., Cucunubá, Z., Cuomo-Dannenburg, G., Dighe, A., Dorigatti, I., Fu, H., Gaythorpe, K., Green, W., Hamlet, A., Hinsley, W., Okell, L. C., van Elsland, S., Thompson, H., … Ghani, A. C. (2020). Impact of non-pharmaceutical interventions (NPIs) to reduce COVID-19 mortality and healthcare demand. https://doi.org/10.25561/77482
- Fone, D., Hollinghurst, S., Temple, M., Round, A., Lester, N., Weightman, A., Roberts, K., Coyle, E., Bevan, G., & Palmer, S. (2003). Systematic review of the use and value of computer simulation modelling in population health and health care delivery. Journal of Public Health, 25(4), 325–335. https://doi.org/10.1093/pubmed/fdg075
- Forrester, J. W. (1961). Industrial dynamics. MIT Press.
- Fu, M. C. (2015). Handbook of simulation optimization. Springer-Verlag.
- Goldsman, D., Nance, R. E., & Wilson, J. R. (2010). A brief history of simulation revisited. Proceedings of the 2010 Winter Simulation Conference (pp. 567–574). IEEE.
- Gostic, K., Gomez, A. C. R., Mummah, R. O., Kucharski, A. J., & Lloyd-Smith, J. O. (2020). Estimated effectiveness of symptom and risk screening to prevent the spread of COVID-19. eLife, 9, e55570. https://doi.org/10.7554/eLife.55570
- Grasselli, G., Pesenti, A., & Cecconi, M. (2020). Critical care utilization for the COVID-19 outbreak in Lombardy, Italy: Early experience and forecast during an emergency response. JAMA. https://doi.org/10.1001/jama.2020.4031
- Griffiths, J. D., Jones, M., Read, M. S., & Williams, J. E. (2010). A simulation model of bed-occupancy in a critical care unit. Journal of Simulation, 4(1), 52–59. https://doi.org/10.1057/jos.2009.22
- Griffiths, J. D., Price-Lloyd, N., Smithies, M., & Williams, J. E. (2005). Modelling the requirement for supplementary nurses in an intensive care unit. Journal of the Operational Research Society, 56(2), 126–133. https://doi.org/10.1057/palgrave.jors.2601882
- Günal, M., & Pidd, M. (2011). DGHPSIM: Generic simulation of hospital performance. ACM Transactions on Modeling and Simulation, 21(4), 23. https://doi.org/10.1145/2000494.2000496
- Hellewell, J., Abbott, S., Gimma, A., Bosse, N. I., Jarvis, C. I., Russell, T. W., Munday, J. D., Kucharski, A. J., Edmunds, W. J., Funk, S., Eggo, R. M., Sun, F., Flasche, S., Quilty, B. J., Davies, N., Liu, Y., Clifford, S., Klepac, P., Jit, M., Diamond, C., & van Zandvoort, K. (2020). Feasibility of controlling COVID-19 outbreaks by isolation of cases and contacts. Lancet Global Health, 8(4), 488–496. https://doi.org/10.1016/S2214-109X(20)30074-7
- Ivanov, D. (2020). Predicting the impacts of epidemic outbreaks on global supply chains: A simulation-based analysis on the coronavirus outbreak (COVID-19/SARS-CoV-2) case. Transportation Research Part E: Logistics and Transportation Review, 136, 101922. https://doi.org/10.1016/j.tre.2020.101922
- Katsaliaki, K., & Brailsford, S. C. (2007). Using simulation to improve the blood supply chain. Journal of the Operational Research Society, 58(2), 219–227. https://doi.org/10.1057/palgrave.jors.2602195
- Kermack, W., & McKendrick, A. (1927). Contributions to the mathematical theory of epidemics. Proceedings of the Royal Society, 115A, 700–721. Reprinted Bulletin of Mathematical Biology (1991), 53(1-2):33-55. https://doi.org/10.1098/rspa.1927.0118
- Kotiadis, K., Tako, A. A., & Vasilakis, C. (2014). A participative and facilitative conceptual modelling framework for discrete event simulation studies in healthcare. Journal of the Operational Research Society, 65(2), 197–213. https://doi.org/10.1057/jors.2012.176
- Lane, D. C., & Husemann, E. (2008). System dynamics mapping of acute patient flows. Journal of the Operational Research Society, 59(2), 213–224. https://doi.org/10.1057/palgrave.jors.2602498
- Langellier, B. A., Kuhlberg, J. A., Ballard, E. A., Slesinski, S. C., Stankov, I., Gouveia, N., Meisel, H. D., Kroker-Lobos, M. F., Sarmiento, O. L., Caiaffa, W. T., & Roux, A. V. D. (2019). Using community-based system dynamics modeling to understand the complex systems that influence health in cities: The SALURBAL study. Health & Place, 60, 102–215. https://doi.org/10.1016/j.healthplace.2019.102215
- Lee, B. Y., Brown, S. T., Korch, G. W., Cooley, P. C., Zimmerman, R. K., Wheaton, W. D., Zimmer, S. M., Grefenstette, J. J., Bailey, R. R., Assi, T.-M., & Burke, D. S. (2010). A computer simulation of vaccine prioritization, allocation, and rationing during the 2009 H1N1 influenza pandemic. Vaccine, 28(31), 4875–4879. https://doi.org/10.1016/j.vaccine.2010.05.002
- Lin, Q., Zhao, S., Gao, D., Lou, Y., Yang, S., Musa, S. S., Wang, M. H., Cai, Y., Wang, W., Yang, L., & He, D. (2020). A conceptual model for the coronavirus disease 2019 (COVID-19) outbreak in Wuhan, China with individual reaction and governmental action. International Journal of Infectious Diseases, 93, 211–216. https://doi.org/10.1016/j.ijid.2020.02.058
- Litvack, N., van Rijsbergen, M., Boucherie, R. J., & van Houdenhoven, M. (2008). Managing the overflow of intensive care patients. European Journal of Operational Research, 185(3), 998–1010. https://doi.org/10.1016/j.ejor.2006.08.021
- McHaney, R., Tako, A., & Robinson, S. (2018). Using LIWC to choose simulation approaches: A feasibility study. Decision Support Systems, 111, 1–12. 0167-9236. https://doi.org/10.1016/j.dss.2018.04.002
- Mingers, J. (2001). Rational analysis for a problematic world revisited: Problem structuring methods for complexity, uncertainty and conflict (J. Rosenhead, Ed.). Chichester.
- Monks, T., Currie, C. S. M., Onggo, B. S., Robinson, S., Kunc, M., & Taylor, S. J. E. (2019). Strengthening the reporting of empirical simulation studies: Introducing the STRESS guidelines. Journal of Simulation, 13(1), 55–67. https://doi.org/10.1080/17477778.2018.1442155
- Mossong, J., Hens, N., Jit, M., Beutels, P., Auranen, K., Mikolajczyk, R., Massari, M., Salmaso, S., Scalia Tomba, G., Wallinga, J., Heijne, J., Sadkowska-Todys, M., Rosinska, M., Edmunds, W. J., & Riley, S. (2008). Social contacts and mixing patterns relevant to the spread of infectious diseases. PLoS Medicine, 5(3), 381–391. https://doi.org/10.1371/journal.pmed.0050074
- Mustafee, N., Brailsford, S., Djanatliev, A., Eldabi, T., Kunc, M., & Tolk, A. (2017). Purpose and benefits of hybrid simulation: Contributing to the convergence of its definition. Proceedings of the 2017 Winter Simulation Conference (WSC) (pp. 1631–1645). IEEE.
- NHS England. (2018). Operational pressures escalation levels framework. NHS England. https://www.england.nhs.uk/wp-content/uploads/2019/02/operational-pressures-escalation-levels-framework-v2.pdf
- Osorio, A. F., Brailsford, S. C., Smith, H. K., Forero-Matiz, S. P., & Camacho-Rodríguez, B. A. (2017). Simulation-optimization model for production planning in the blood supply chain. Health Care Management Science, 20(4), 548–564. https://doi.org/10.1007/s10729-016-9370-6
- Özaltin, O. Y., Dalgiç, O. O., & Erenay, F. S. (2014). Optimal distribution of the influenza vaccine. Proceedings of the 2014 Winter Simulation Conference, 1411–1420. doi:10.1109/WSC.2014.7019995
- Pagel, C., & Utley, M. (2020). Covid-19: how to triage effectively in a pandemic. BMJ, accessed 8 April 2020. https://blogs.bmj.com/bmj/2020/03/09/covid-19-triage-in-a-pandemic-is-even-thornier-than-you-might-think/
- Penn, M. L., Monks, T., Kazmierska, A. A., & Alkoheji, M. R. A. R. (2019). Towards generic modelling of hospital wards: Reuse and redevelopment of simple models. Journal of Simulation, 1–12. In Press. https://doi.org/10.1080/17477778.2019.1664264
- Pruyt, E., & Hamarat, C. (2010, July 25-29). The influenza A (H1N1) v pandemic: An exploratory system dynamics approach. Proceedings of the 18th International Conference of the System Dynamics Society. https://proceedings.systemdynamics.org/2010/proceed/papers/P1389.pdf
- Public Health England. (2020). New surveillance system for early detection of COVID-19. Public Health England. Retrieved March 26, 2020, from https://www.gov.uk/government/news/new-surveillance-system-for-early-detection-of-covid-19
- Remuzzi, A., & Remuzzi, G. (2020). COVID-19 and Italy: What next? The Lancet. https://doi.org/10.1016/S0140-6736(20)30627-9
- Richardson, G. P., & Andersen, D. F. (1995). Teamwork in group model building. System Dynamics Review, 11(2), 113–137. https://doi.org/10.1002/sdr.4260110203
- Ridge, C., Jones, S. K., Nielsen, M. S., & Shahani, A. K. (1998). Capacity planning for intensive care units. European Journal of Operational Research, 105(2), 346–355. https://doi.org/10.1016/S0377-2217(97)00240-3
- Robertson, D. A. (2019). Spatial transmission models: A taxonomy and framework. Risk Analysis, 39(1), 225–243. https://doi.org/10.1111/risa.13142
- Robinson, S. (2008). Conceptual modelling for simulation Part I: Definition and requirements. Journal of the Operational Research Society, 59(3), 278–290. https://doi.org/10.1057/palgrave.jors.2602368
- Robinson, S., Worthington, C., Burgess, N., & Radnor, Z. J. (2014). Facilitated modelling with discrete-event simulation: Reality or myth? European Journal of Operational Research, 234(1), 231–240. https://doi.org/10.1016/j.ejor.2012.12.024
- Roda, W. C., Varughese, M. B., Han, D., & Li, M. Y. (2020). Why is it difficult to accurately predict the COVID-19 epidemic? Infectious Disease Modelling, 5, 271–281. In Press. https://doi.org/10.1016/j.idm.2020.03.001
- Rutter, H., Savona, N., Glonti, K., Bibby, J., Cummins, S., Finegood, D. T., Greaves, F., Harper, L., Hawe, P., Moore, L., Petticrew, M., Rehfuess, E., Shiell, A., Thomas, J., & White, M. (2017). The need for a complex systems model of evidence for public health. The Lancet, 390(10112), 2602–2604. https://doi.org/10.1016/S0140-6736(17)31267-9
- Sharareh, N., Sabounchi, N. S., Sayama, H., & MacDonald, R. (2016, November 3). The Ebola crisis and the corresponding public behavior: A system dynamics approach. PLoS Currents, 8. https://www.ncbi.nlm.nih.gov/pubmed/27974995
- Sterman, J. D. (1992). Teaching takes off: Flight simulators for management education. OR/MS Today, 35(3), 40–44.
- Suleimenova, D., Bell, D., & Groen, D. (2017). A generalized simulation development approach for predicting refugee destinations. Scientific Reports, 7(1), 13377. https://doi.org/10.1038/s41598-017-13828-9
- Tako, A. A., & Kotiadis, K. (2015). PartiSim: A multi-methodology framework to support facilitated simulation modelling in healthcare. European Journal of Operational Research, 244(2), 555–564. https://doi.org/10.1016/j.ejor.2015.01.046
- Tako, A. A., & Robinson, S. (2009). Comparing discrete-event simulation and system dynamics: Users’ perceptions. Journal of the Operational Research Society, 60(3), 296–312. 0160-5682. https://doi.org/10.1057/palgrave.jors.2602566
- Tako, A. A., & Robinson, S. (2012). The application of discrete event simulation and system dynamics in the logistics and supply chain context. Decision Support Systems, 52(4), 802–815. 0167-9236. https://doi.org/10.1016/j.dss.2011.11.015
- Tang, B., Wang, X., Li, Q., Bragazzi, N. L., Tang, S., Xiao, Y., & Wu, J. (2020). Estimation of the transmission risk of the 2019-nCoV and its implication for public health interventions. Journal of Clinical Medicine, 9(2), 462. https://doi.org/10.3390/jcm9020462
- Taylor, S. J. E. (2019). Distributed simulation: State-of-the-art and potential for operational research. European Journal of Operational Research, 273(1), 1–19. https://doi.org/10.1016/j.ejor.2018.04.032
- Taylor, S. J. E., Anagnostou, A., Fabiyi, A., Currie, C., Monks, T., Barbera, R., & Becker, B. (2017). Open science: Approaches and benefits for modeling and simulation. Proceedings of the 2017 Winter Simulation Conference, 535–549. https://doi.10.1109/WSC.2017.8247813
- Tierney, L. T., & Conroy, K. M. (2014). Optimal occupancy in the ICU: A literature review. Australian Critical Care, 27(2), 77–84. https://doi.org/10.1016/j.aucc.2013.11.003
- Viana, J., Brailsford, S. C., Harindra, V., & Harper, P. R. (2014). Combining discrete-event simulation and system dynamics in a healthcare setting: A composite model for Chlamydia infection. European Journal of Operational Research, 237(1), 196–206. https://doi.org/10.1016/j.ejor.2014.02.052
- Walker, P. G. T., Whittaker, C., Watson, O., Baguelin, M., Ainslie, K. E. C., Bhatia, S., Bhatt, S., Boonyasiri, A., Boyd, O., Cattarino, L., Cucunubá, Z., Cuomo-Dannenburg, G., Dighe, A., Donnelly, C. A., Dorigatti, I., van Elsland, S., FitzJohn, R., Flaxman, S., Fu, H., Gaythorpe, K., … Ghani, A. C. (2020). The global impact of COVID-19 and strategies for mitigation and suppression. Imperial College London. Retrieved March 29, 2020, from https://doi.org/10.25561/77735
- Wells, C. R., Sah, P., Moghadas, S. M., Pandey, A., Shoukat, A., Wang, Y., Wang, Z., Meyers, L. A., Singer, B. H., & Galvani, A. P. (2020). Proceedings of the National Academy of Science U S A, In Press. https://doi.org/10.1073/pnas.2002616117
- WHO. (2020). Retrieved March 20, 2020, from https://experience.arcgis.com/experience/685d0ace521648f8a5beeeee1b9125cd
- Wilson, N., Kvalsvig, A., Barnard, L. T., & Baker, M. G. (2020). Case-fatality risk estimates for COVID-19 calculated by using a lag time for fatality. Emerging Infectious Diseases, 26(6). Retrieved March 27, 2020, from https://doi.org/10.3201/eid2606.200320
- Wu, J. T., Leung, K., Bushman, M., Kishore, N., Niehus, R., de Salazar, P. M., Cowling, B. J., Lipsitch, M., & Leung, G. M. (2020). Estimating clinical severity of COVID-19 from the transmission dynamics in Wuhan, China. Nature Medicine. In Press. https://doi.org/10.1038/s41591-020-0822-7
- Xiao, H., Gao, F., and Lee, L.H. (2020). Optimal computing budget allocation for complete ranking with input uncertainty. IISE Transactions, 52(5), 489–499. https://doi.org/10.1080/24725854.2019.1659524
- Xu, J., Huang, E., Hsieh, L., Lee, L. H., Jia, Q.-S., & Chen, C.-H. (2016). Simulation optimization in the era of industrial 4.0 and the industrial internet. Journal of Simulation, 10(4), 310–320. https://doi.org/10.1057/s41273-016-0037-6
- Yang, Y., Atkinson, P. M., & Ettema, D. (2011). Analysis of CDC social control measures using an agent-based simulation of an influenza epidemic in a city. BMC Infectious Diseases, 11(Article), 199. https://doi.org/10.1186/1471-2334-11-199
- Zhu, Z., Hen, B. H., & Teow, K. L. (2012). Estimating ICU bed capacity using discrete event simulation. International Journal of Health Care Quality Assurance, 25(2), 134–144. https://doi.org/10.1108/09526861211198290