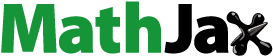
ABSTRACT
A pre-performance routine (PPR) refers to a set of task-relevant thoughts and actions an athlete systematically engages in prior to performance execution. The aim of this meta-analysis was to determine the effectiveness of the PPR intervention to facilitate sport performance. The meta-analysis included 112 effect sizes from pre–post and experimental designs in low-pressure and pressurised conditions. Extensive PPRs with several preparatory elements as well as specific stand-alone PPRs, such as left-hand dynamic handgrip and quiet eye, were analysed. Three-level random-effects models were used for analysis, utilising Knapp-Hartung adjustments and restricted maximum likelihood estimation. Results showed a significant but small effect of PPRs on sport performance in pre–post designs (SMC = 0.31, 95% CI [0.18, 0.44]) and moderate-to-large effects in experimental designs, both under low-pressure (Hedges’ g = 0.64, 95% CI [0.45, 0.83]) and pressurised conditions (Hedges’ g = 0.70, 95% CI [0.24, 1.16]). These effects were not moderated by the type of PPR, age, gender, skill level, or intervention characteristics. Overall, the meta-analytic results support the benefits of the PPR intervention in practice regardless of the type of routine. Both extensive and stand-alone PPRs are effective in optimising sport performance.
Introduction
Many great athletes have a routine they do right before they perform. When Tiger Woods, an elite golfer, approaches a shot, he stands behind the ball holding his club, visualises where he wants the shot to land, then walks up to the ball, and aligns himself a few feet in front of the ball. Once in this position, he looks at the ball and target one more time, and then executes the shot (www.ussportscamps.com). Michael Jordan, a former basketball star who made 84% of his career free throws (National Basketball Association, Citation2019), used to take a shoulder width stance, spin the ball in his hands, bounce the ball three times, and then spin the ball once again while fixating the rim before he finally threw the ball. Such behaviours have been referred to as a pre-performance routine (PPR), a sequence of cognitive and behavioural elements prior to performance execution (Moran, Citation1996), which nowadays represents one of the most common interventions delivered by sport psychologists in the field (Cotterill, Citation2010). However, despite this widespread application by practitioners, a meta-analytic quantification that would support the benefit of a PPR for performance is lacking. The aim of this meta-analysis therefore is to determine the effect of the PPR intervention on performance in sports, along with testing variables that might potentially moderate this effect, such as the type of PPR, age, gender, skill level, and pressure.
Pre-performance routines and performance
A PPR, also called a pre-shot (e.g. in golf) or pre-serve routine (e.g. in tennis), has been defined as ‘a sequence of task-relevant thoughts and actions which an athlete engages in systematically prior to his or her performance of a specific sport skill’ (Moran, Citation1996, p. 177). Systematic engagement and task-relevance are inherent in this definition to distinguish a PPR from superstitious behaviour (i.e. the behaviour that results from accidental reinforcement of an action so that the person continues to repeat it; https://dictionary.apa.org, Citationn.d.), such as wearing ‘lucky socks’. There seems to be a widespread agreement among practitioners that PPRs enhance performance and several single studies are supportive in this regard (Cotterill, Citation2010). Performance benefits of a PPR were documented for a number of self-paced, closed skill tasks, such as putting in golf (Hill et al., Citation2011), serving in tennis (Lautenbach et al., Citation2015), free throw shooting in basketball (Lonsdale & Tam, Citation2008), execution of a tenpin bowling delivery (Mesagno et al., Citation2015), and performing a routine in gymnastics (Clowes & Knowles, Citation2013). Moreover, positive effects were evidenced for both novices (e.g. Lidor & Mayan, Citation2005; McCann et al., Citation2001) and experienced athletes (e.g. Lonsdale & Tam, Citation2008; Mesagno et al., Citation2015), and for performance in both low-pressure (Cotterill, Citation2010) and pressurised situations (Gröpel & Mesagno, Citation2019). The effectiveness of PPRs was typically inferred from pre–post designs where participants learned a new PPR and their performance was tested before and after learning the routine. Alternatively (or additionally), participants’ performance was tested against a control group that did not use a PPR (i.e. experimental design).
Mechanisms of pre-performance routines
Researchers have proposed several mechanisms through which a PPR may facilitate performance, including improvement of attention, increase of self-efficacy, anxiety regulation, and the use of action planning (Cotterill, Citation2010; Singer, Citation2002). Already Boutcher (Citation1992) suggested that the main function of a PPR was to enhance concentration, such as to direct attention to task-specific cues (e.g. the golf ball and hole in golf) and minimise internal or external distractions (e.g. negative thoughts or noises made by the audience). This notion was later corroborated by Cotterill et al. (Citation2010) who interviewed six experienced male golfers on their use of a PPR; all six golfers stated that PPRs substantially improved their attention. Furthermore, a PPR is likely to enhance performers’ self-efficacy (Cotterill et al., Citation2010; Perry & Katz, Citation2015). In an interview study with elite golfers and their coaches, the golfers reported that using a PPR improved their feeling of control prior to performing (Hill et al., Citation2010). Similarly, Perry and Katz (Citation2015) observed that novice athletes who learned a PPR subsequently increased their self-efficacy. A PPR may also help performance by down-regulating athletes’ anxiety levels. Hazell et al. (Citation2014) found that football players who used a PPR reduced their anxiety and subsequently performed better in a penalty shootout than control participants. Finally, a PPR can give athletes a plan of action and allow for mental rehearsal prior to motor skill execution (Cohn et al., Citation1990; Cotterill, Citation2010).
Types of pre-performance routines
In general, a PPR may consist of a single preparatory element (referred to as stand-alone PPR hereafter) or combine several preparatory elements into an extensive PPR. The most typical elements include (but are not limited to) physical routines, imagery, self-talk, relaxation, and external focus of attention (Cohn et al., Citation1990; Mesagno et al., Citation2008). Physical routines comprise a variety of sport-specific motor actions, such as bouncing a ball before serving in tennis, dribbles before shooting a free throw in basketball, or a stick tap before executing a penalty shot in ice hockey. Imagery (or visualisation) refers to the mental creation and re-creation of an experience, such as imagery of successful performance (Morris et al., Citation2005). Self-talk describes the use of self-instructional strategies to facilitate motivation and performance (e.g. ‘I can do it’; Hatzigeorgiadis et al., Citation2011). Relaxation elements typically consist of taking a couple of deep breaths before performing (Mesagno & Mullane-Grant, Citation2010). External focus of attention is usually induced by focusing on a task-relevant object in the environment (e.g. a target) or by using a cue word (e.g. ‘hit’; Mesagno et al., Citation2008).
More recently, researchers introduced left-hand dynamic handgrip and quiet eye as additional PPR elements. Left-hand dynamic handgrip refers to clenching the left fist, or squeezing a ball in the left hand, approximately twice a second, for 30–45 s prior to task execution (Gröpel & Beckmann, Citation2017; Mesagno et al., Citation2019). Researchers have found that left-hand dynamic handgrip produces a state of reduced cortical activity, which may prevent suboptimal interferences in the brain and hence optimise performance (Cross-Villasana et al., Citation2016). Quite eye (QE) refers to the final visual fixation at a target (e.g. the basketball rim in basketball) before the performance execution (Vine et al., Citation2013). It also serves as a marker of adaptive, task-related visual attention, with experts showing a longer QE than novices (Lebeau et al., Citation2016; Vine & Wilson, Citation2010).
Research evidence supports the benefits of both stand-alone and extensive PPRs on performance (e.g. Brown & Fletcher, Citation2017; Gröpel & Mesagno, Citation2019), but a comparison of whether the benefits of stand-alone PPRs cumulate when used in combination (i.e. extensive PPR) is rare and inconclusive. Mesagno and Mullane-Grant (Citation2010) found that an extensive PPR, comprising of different preparation elements (e.g. deep breath, cue word, and temporal consistency), had a stronger effect on performance than the respective elements in isolation. In contrast, Mesagno et al. (Citation2019) and Wergin et al. (Citation2020) did not find stronger effects of an extensive PPR compared to respective stand-alone routines.
The present meta-analysis
There are three main aims of this meta-analysis. First, we aim to quantify the effect of the PPR intervention on sport performance in general, and the effect of stand-alone versus extensive PPRs in particular. This may help both researchers and practitioners working with athletes to gain a greater understanding of the magnitude of PPR effectiveness and to decide which type of PPR to use. Meta-analyses on single techniques such as imagery (Simonsmeier et al., Citation2020), self-talk (Hatzigeorgiadis et al., Citation2011), and QE (Lebeau et al., Citation2016) exist, but a meta-analysis that would combine and compare several preparatory strategies is still lacking. Moreover, the above meta-analyses did not specifically address the role of imagery, self-talk, and QE as a PPR.
Second, we aim to test the effect of the PPR intervention on sport performance in pressurised conditions. Pressure refers to any factor or combination of factors that increases performers’ anxiety and includes features such as competition, the presence of audience, reward or punishment contingency, and ego relevance (Baumeister & Showers, Citation1986). Researchers have suggested that using a PPR may be especially beneficial under pressure because it helps to reduce pressure-induced distraction and anxiety (Gröpel & Mesagno, Citation2019). The knowledge of how much the PPR intervention contributes to performance under pressure might thus help practitioners and athletes to optimise the use of PPR in competitions.
Third, we aim to test variables that potentially moderate the relationship between PPRs and sport performance, such as age, gender, skill level, learning time, and the use of individualised PPRs. Crews and Boutcher (Citation1986) found that a PPR improved the full swing of male but not female golfers, and Brown and Fletcher (Citation2017) observed that gender accounted for 4% of variance in the intervention-performance effect. Regarding skill level, benefits of the PPR intervention were observed for both novices and experts, but whether there is a difference in the magnitude of effectiveness is still unclear. It is also not known how much time is necessary to learn a PPR in order to gain performance-related benefits. Blumenstein et al. (Citation2016) reported that eight learning sessions were more effective than four sessions, and Hazell et al. (Citation2014) postulated that many studies on PPRs employed too little time to learn a PPR sufficiently. Nonetheless, researchers also found PPR benefits despite short learning time, with some studies using as little as 5–10 min of learning in total (Mesagno & Mullane-Grant, Citation2010). Finally, there is a considerable debate regarding the necessity to individualise PPRs. Some researchers emphasised the importance to tailor a PPR to each individual (e.g. Cotterill et al., Citation2010; Lidor & Mayan, Citation2005), while other researchers observed positive effects with standardised, non-individualised PPRs as well (e.g. Mesagno et al., Citation2019; Mesagno & Mullane-Grant, Citation2010). Detecting significant moderators is thus necessary for optimal application of the PPR intervention and for the identification of potential future directions.
We compute three meta-analyses using random-effects models to estimate the magnitude of the effects of performing a sport task with vs. without a PPR: (1) a meta-analysis on pre–post data to compare how performance changes from before to after learning a PPR; (2) a meta-analysis on experimental data where performance is tested against a control (or comparison) group that does not use a PPR; and (3) a meta-analysis on experimental data in pressurised conditions. To be included in the category ‘under pressure’, the study must either incorporate an effective pressure manipulation (as validated by significantly increased anxiety compared to a baseline; cf. Gröpel & Mesagno, Citation2019) or use a ‘real-world’ pressure (e.g. an actual competition; Baumeister & Showers, Citation1986). In the case that a study contains pressure induction but it does not affect athletes’ anxiety levels, the study is included in the second meta-analysis featuring low-pressure experimental designs.
Method
The Preferred Reporting Items for Systematic Reviews and Meta-Analysis (PRISMA) guidelines (Moher et al., Citation2009) were used to conduct this review.
Study eligibility criteria
Studies were included that used the PPR intervention to facilitate sport performance. The specific inclusion criteria were: (a) reported a clearly identifiable effect of the PPR on performance. Studies that administered a PPR within a broad training programme (e.g. cognitive–behavioural intervention programme, mental skills programme) were excluded, because the effect of the PPR on performance was not unambiguously detectable; (b) indicated that the PPR was performed immediately before executing a sport task or the author(s) had to deliberately specify that they employed a PPR; (c) measured the effect of the PPR in an objective and quantitative manner. Studies that relied on scores made by professional referees, such as in gymnastics, were also included, as this kind of scoring is standard in such sports and thus ecologically valid. Studies that used qualitative data or subjective ratings made by athletes themselves were excluded; (d) tested how performance changed from before to after the PPR intervention (pre–post design) or in comparison to a control group (experimental design) or both; (e) reported an effect size or provided sufficient data to calculate an effect size; (f) included ≥ 3 participants to enable the calculation of Hedges’ g with correction for small sample sizes (Borenstein et al., Citation2009; Viechtbauer, Citation2010); and (g) were available in full-text in English or German language.
Search strategy and data extraction
A systematic literature search was undertaken on the computerised psychological and sport databases PsycINFO (1806 to present), ScienceDirect (1967 to present), SPORTDiscus (1970 to present), and Web of Science (1898 to present) to identify all available studies, both published and unpublished (e.g. PhD theses or preprints). In addition, we used Google Scholar, which is a fast-growing and relatively comprehensive database that also records non-published and non-English records (de Winter et al., Citation2014; Prins et al., Citation2016), and sifted the first 500 records sorted by relevance. The covered time period ranged from inception of the respective database up until November 30, 2020. Keyword combinations used were: ‘pre-performance routine’ OR ‘pre-shot routine’ OR ‘pre-serve routine’ OR ‘performance routine’ OR ‘preparation routine’. In addition, we hand searched reference lists of relevant reviews (Brown & Fletcher, Citation2017; Cotterill, Citation2010; Lebeau et al., Citation2016) and retrieved articles for other articles.
We sifted the retrieved studies into two stages. First, we screened titles and abstracts, with duplicates and clearly irrelevant records excluded. Second, we retrieved the full-text papers of the remaining records and performed an eligibility assessment. To characterise each study, we extracted author(s), publication year, type of sport, type of routine, and several other relevant moderators (see next section). If studies did not report sufficient data to be included in the analysis, we contacted the respective first authors by e-mail or via ResearchGate (one reminder being sent two weeks after the initial contact). In case of missing data to calculate the main effect, studies were excluded if contact requests remained unsuccessful. In case of missing data for moderators, the respective study was not incorporated in the given meta-regression or subgroup analysis but was still part of the analysis of the main effect.
Moderator variables and data coding
The following moderators were coded and tested: (a) gender (coded as percentage of males included in sample); (b) mean age of participants (years); (c) learning time to acquire the PPR (in minutes); (d) whether or not the PPR was individualised (yes/no); (e) skill level of participants (novice/subelite/elite); and (f) the type of routine (stand-alone PPR/extensive PPR). The skill level of participants was classified using the distinction by Macnamara et al. (Citation2016), categorising the samples in novices (no previous experience with the performed sport task), subelite (contestants at state/provincial level or a lower level), and elite (contestants at national level or a higher level). Stand-alone PPRs consisted of only one mental or behavioural element, whereas extensive PPRs combined two or more elements.
Furthermore, year of publication was included as a centred moderator to detect time trends in effect sizes. Because experimental data were gathered from both randomised and non-randomised (quasi-experimental) studies, we controlled for randomisation, that means, whether or not the allocation of participants was randomised (yes/no). Three further moderating variables (peer-review, study quality, and a measure for precision) are discussed below together with the publication bias analysis. In line with the recommendations by Assink and Wibbelink (Citation2016), a separate moderator analysis was performed for each variable, followed by a joint analysis with all significant moderators.
Quality assessment
In order to assess the risk of bias of each selected study, we employed Version 11 of the Mixed Methods Appraisal Tool (MMAT; Pluye et al., Citation2011). The MMAT enables researchers to concomitantly appraise the methodological quality of qualitative, quantitative, and mixed methods studies, by using up to four methodological criteria for each of five types of study design (qualitative studies, randomised-controlled trials, non-randomised quantitative studies, quantitative descriptive studies, and mixed methods studies). Studies retrieved for the present review were either randomised-controlled trials or non-randomised quantitative studies or mixed methods studies. For randomised-controlled trials, methodological criteria included the quality of randomisation, allocation concealment or blinding (when applicable), outcomes reporting, and drop-out rate. For non-randomised quantitative studies, methodological criteria were the quality of selection, comparability, appropriate exposure, and outcomes reporting. For mixed methods studies, the criteria included the relevance of research design, the relevance of data, and the consideration of limitations. The resulting quality score of each study could range from 0 (no criterion met) to 1 (all criteria met), with all criteria receiving equal weight. The MMAT has been used beneficially in sport and exercise psychology (Gayman et al., Citation2016; Gröpel & Mesagno, Citation2019).
Effect sizes and meta-analytic procedure
Statistical analyses were conducted using the meta-analysis package metafor for R (Viechtbauer, Citation2010). The analyses were twofold: First, a meta-analysis on pre–post data was conducted. The effect size was the standardised mean change (SMC) with raw score standardisation (Morris, Citation2008):
(1)
(1)
This measure bears the advantage that it is comparable with Hedges’ g (Morris, Citation2000):
(2)
(2)
In case that studies did not report the correlation between pre- and post-test results, the median correlation (of all studies that reported the correlation) was chosen.
Second, the data on experimental studies were analysed, using Hedges’ g as the effect size:
(3)
(3)
For all analyses, a three-level model was fitted to the data to account for the fact that samples were often nested within studies, with studies often testing more than one experimental group (Harrer et al., Citation2019). With a three-level model, effect sizes could vary not only on the participant level (Level 1) and between studies (Level 3), but also within studies (Level 2; Assink & Wibbelink, Citation2016). The three-level model accounted for the dependency of effect sizes within studies, which may otherwise bias results. As estimator for τ, the restricted maximum likelihood estimation (REML) was selected. Veroniki et al. (Citation2016) recommend REML for continuous data as preferable option regarding bias and efficiency of the parameter estimates. To avoid false positive results regarding the estimation of coefficients, the Knapp-Hartung adjustment was used in the present analysis (Assink & Wibbelink, Citation2016). Forest plots for the three-level meta-analyses were built using the R code supplied by Fernández-Castilla et al. (Citation2020).
Pre–post data were summarised if participants delivered more than one data point for the pre-test and post-test phases, such as when participants’ scores from a laboratory task and an actual competition was used as two separate performance measures in either phase. The calculations to summarise individual effect sizes within the same participants were conducted using formula 24.1 from Borenstein et al. (Citation2009). Analogously, the sampling variances were summarised using formula 24.2 from Borenstein et al. (Citation2009). The correlation between laboratory task and league competition was assumed to be r = 0.50 in case it was not obtainable from the original study. For experimental data, a variance–covariance matrix of the effect size estimates was computed (Gleser & Olkin, Citation2009). Numerous studies tested the effects of different PPRs in comparison to a single control group. In such cases, effect size estimates were calculated using the same information (i.e. mean and standard deviation of the control group) repeatedly. Hence effect size estimates shared information and were no longer independent, which could bias results. Therefore, a variance-covariance matrix was fitted to the data, employing a block-diagonal structure to account for the particular interdependence among effect sizes obtained from the same control group (Gleser & Olkin, Citation2009).
The individual studies were expected to be heterogeneous regarding participants’ age, gender, and skill level, type of PPR, learning time, and regarding setting in which participants performed. Consequently, a random-effects model was fitted to the data for all analyses (pre–post and experimental). Cochran’s Q-test was used to test the null hypothesis of a shared common effect size among the individual primary studies (Borenstein et al., Citation2009). However, in case of a small number of primary studies, the test has low statistical power and might deliver false-negative results. Therefore, I2 was used to assess heterogeneity in relative terms. I2 compares the amount of true heterogeneity to the overall amount of heterogeneity, is independent from the number of studies k and from the scale used for the meta-analysis, and can be split among the distinct variance levels of a meta-analysis (Borenstein et al., Citation2009). An ANOVA compared within-study variance () with between-study variance (
) and aided in analysing the distribution of variance over the three different study levels (Harrer et al., Citation2019). The p-value of the corresponding log-likelihood-ratio test (LRT) was divided by two to allow for one-sided testing (Assink & Wibbelink, Citation2016; Harrer et al., Citation2019). In addition, the estimate and 95% confidence intervals of a profile likelihood plot of τ2 were reported to assess the range of heterogeneity of the different variance levels (Viechtbauer, Citation2010). Outliers were defined as studies with effect sizes which were in a distance of more than three standard deviations from the mean effect size. A sensitivity analysis for the main effect with and without outliers was then performed to assess the impact of outliers on the main effect (Harrer et al., Citation2019).
There is considerable debate regarding tests for publication bias in multilevel models (Assink & Wibbelink, Citation2016). Publication bias may distort meta-analytic results. To account for risk of bias across studies (i.e. publication bias), the present analysis deployed a number of statistical methods: First, it used a meta-regression model with the sampling variance (for pre–post data) or with the sample size of the intervention group (for experimental data) to detect small-study effects (i.e. larger effects among smaller vs. larger studies, which may be indicative of publication bias). Second, whether studies were peer-reviewed (yes/no) was an additional moderator to test for publication bias (Borenstein et al., Citation2009). Third, contour-enhanced funnel plots were used to detect questionable research practices. In order to get published, researchers could engage in a practice termed p-hacking – i.e. modifying and repeating statistical analyses to obtain (barely) significant p-values. A contour-enhanced funnel plot allows for detection of effect size estimates that are barely significant by highlighting different significance regions. Fourth, p-uniform analyses were conducted to further explore potential publication bias. P-uniform analysis is a tool to detect publication bias and assess its impact on effect size estimates (van Assen et al., Citation2014). It relies exclusively on significant and published studies, requires no assumptions regarding missing data, and has been shown to outperform other publication bias analyses such as trim-and-fill (van Assen et al., Citation2014).
Results
Initial search results returned 1,633 records, which were reduced to 50 records after screening. A total of 33 articles with k = 61 separate intervention samples (i.e. 16 articles were multi-study articles or included more than one intervention sample) were included in the meta-analyses (). Sample characteristics, type of routine, study design, and methodological quality are summarised in . Notably, the included samples yielded multiple sets of data points to calculate effect sizes, that means, the same sample could yield data points for the meta-analysis of pre–post data as well as the meta-analysis of experimental data and/or experimental data under pressure. In total, the included samples yielded 112 effect sizes; the meta-analysis of pre–post data was based on 51 effect sizes, the meta-analysis of low-pressure experimental data on 42 effect sizes, and the meta-analysis of experimental data from pressurised conditions on 19 effect sizes (Supplementary Table S1 shows all effect size estimates with corresponding confidence intervals).
Figure 1. PRISMA (Preferred Reporting Items for Systematic Reviews and Meta-Analysis) chart of search strategy.
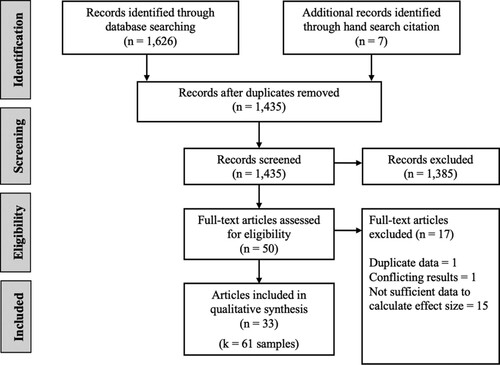
Table 1. Summary of included studies.
Characteristics of samples
Sixteen samples (26%) included less than 10 participants, half of the samples (k = 32; 52%) between 10 and 19 participants, and 13 samples (21%) more than 20 participants. In terms of participants’ skill level, the majority of samples (70%) comprised experienced athletes, either subelite (k = 34) or elite (k = 9), and 13 samples included novice athletes (21%). Performance data were gathered from 15 different sports, which were golf (k = 15), basketball (k = 13), tennis (k = 6), tenpin bowling (k = 6), Australian football (k = 4), football (k = 3), beach volleyball (k = 3), volleyball (k = 2), gymnastics (k = 2), karate (k = 2), air pistol (k = 1), badminton (k = 1), weight lifting (k = 1), taekwondo (k = 1), and water polo (k = 1). An extensive PPR was tested in 33 samples (54%), whereas a stand-alone PPR was implemented in 27 samples (44%); one study did not specify the type of PPR. Of the stand-alone PPRs, the intervention consisted of left-hand dynamic handgrip (k = 7), imagery (k = 6), physical routine (k = 5), quiet eye (k = 3), relaxation (k = 2), self-talk (k = 2), external focus (k = 1), and temporal consistency (i.e. a 5 seconds countdown; k = 1).
Model results
summarises the meta-analytic results and shows the forest plots for the three meta-analyses. For pre–post data, the model resulted in a significant main effect of 0.31 (SMC) with a 95% CI ranging from 0.18 to 0.44. The removal of two outlying samples did not alter the magnitude of the main effect, but slightly improved precision of the effect (SMC = 0.28, 95% CI = [0.17, 0.39]). There was a significant amount of effect heterogeneity (Q[50] = 128.06, p < 0.001). Consequently, the null hypothesis of a shared common effect size was rejected. In relative terms, the heterogeneity of effect was moderate (I2 = 61.99%). There was more within-study variance (I2Level 2 = 47.88%) than between-study variance (I2Level 3 = 14.11%). The removal of within-study variance led to a significantly worse model fit (LRT[2] = 4.51, p = 0.02), whereas the removal of between-study variance did not (LRT[2] = 0.22, p = 0.32), indicating variability between effect sizes within, but not between, studies.
Figure 2. Summary forest plots for the pre–post data (a), low-pressure experimental data (b), and experimental data under pressure (c). The thickness of the grey confidence intervals is proportional to the number of effect sizes reported within studies (J).
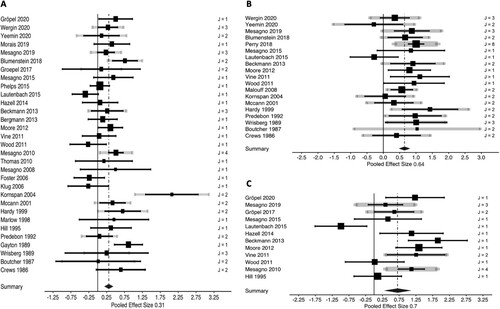
Table 2. Model results.
For low-pressure experimental data, the model yielded a Hedges’ g of 0.64 with corresponding 95% CI from 0.45 to 0.83. Again, removing one outlier did not change the magnitude of the main effect (g = 0.62, 95% CI = [0.43, 0.82]). The null hypothesis of shared common effect sizes was not rejected (Q[41] = 55.51, p = 0.06). However, this could also be indicative for imprecise primary studies and does not necessarily exclude effect heterogeneity. I2Level 1 = 79.59% indicated that most variance is caused by sampling error. Almost the entire remaining variance (I2Level 3 = 20.41) was explained by between-study differences. Removal of this level did not lead to a significantly worse model fit (LRT[2] = 2.22, p = 0.07).
For experimental data under pressure, fitting the random-effects, three-level model to the data led to Hedges’ g of 0.70 with 95% CI ranging from 0.24 to 1.16. The large CI indicated a substantial amount of uncertainty regarding the estimated main effect. This might be caused by the small number of effect sizes (k = 19). The null hypothesis of shared common effect sizes was rejected (Q[18] = 49.92, p < 0.001). In relative terms, there was a high amount of effect heterogeneity (I2 = 70.62%), mostly accounted for by between-study variance (I2Level 3 = 66.27%). The removal of between-study variance led to a significantly worse model fit (LRT[2] = 2.94, p = 0.04). Since there was a significant amount of between-study heterogeneity, a prediction interval (PI) was calculated, using τ as standard deviation. One can expect that 95% of future studies fall in the interval between −0.54 and 1.95. This is a relatively large range, further indicative for uncertainty regarding the estimates based on data under pressure.
Moderator analysis
and display the results of meta-regressions and subgroup analyses, respectively, conducted for each moderator in all three meta-analyses. In case of meta-regressions, the results refer to the slope of the coefficient of a regression and the corresponding SE. In case of subgroup analyses, results refer to an effect size estimate, with corresponding SE, for the particular subgroup and the test of moderators (QM). Of the tested moderating variables, none turned out to have a significant effect on the relationship between PPRs and performance.
Table 3. Results of meta-regressions.
Table 4. Results of subgroup analyses.
Assessment of bias between samples
For pre–post data, a meta-regression with sampling variance as predictor was significant (b = 3.07, p = 0.01). This indicates that there were small-study effects, such that studies with smaller samples systematically reported larger effect sizes. Neither publication status nor study quality emerged as significant moderators ( and ). (a) shows the contour-enhanced funnel plot for pre–post data. Upon visual inspection, a rather heterogeneous set of samples could be identified. A p-uniform analysis did not yield evidence for publication bias (Lμ = −1.88, p = 0.97) and the null hypothesis of no effect could be rejected (L0 = −3.18, p < 0.001).
Figure 3. Funnel plots for the pre–post data (a), low-pressure experimental data (b), and experimental data under pressure (c).
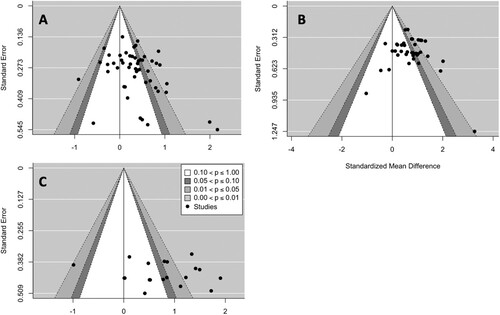
For low-pressure experimental data, neither sample size (b < 0.01, p = 0.87) nor publication status nor study quality were significant moderators. The contour-enhanced funnel plot showed that some studies were barely significant (i.e. bordering on the grey areas) and at least one sample appeared to be an outlier ((b)). Based on the plot, questionable research practices could be present in these samples as well. However, the p-uniform analysis indicated the robustness of results (L0 = −3.35, p < 0.001) and that the overall effect of PPRs on performance could not be explained by publication bias (Lμ = 0.08, p = 0.47).
Similarly, for experimental data under pressure, neither sample size (b = 0.02, p = 0.78) nor publication status nor study quality were significant moderators. The contour-enhanced funnel plot showed a pair of studies bordering on the grey shaded areas ((c)). As indicated by p-uniform analysis, publication bias did not play a role (Lμ = −1.69, p = 0.95) and the assumption of no effect could be rejected (L0 = −3.16, p < 0.001).
Discussion
The aim of this meta-analysis was to determine the effect of the PPR intervention on performance in sports, and to address some of the perplexing issues in the PPR literature (e.g. are individualised PPRs more effective?). A total of 61 separate intervention samples across 15 different sports met all inclusion criteria. These samples yielded 112 effect sizes to analyse. Results showed a significant but small effect of PPRs on sport performance in pre–post designs and moderate-to-large effects in experimental designs, both under low-pressure and pressurised conditions. These effects were robust to sensitivity analyses when outlying samples were removed and were unlikely to be caused by publication bias. The findings indicate that using a PPR before performance execution brings a benefit to the performer, both in general and under pressure in particular.
The effect of PPRs on performance obtained from pre–post designs was smaller than that from experimental designs. This may be explained by the fact that the effect from pre–post designs was summarised across a variety of settings, including performances under artificial or actual pressure in which a stable rather than improved performance was expected. For example, Beckmann et al. (Citation2013; Study 1) found that participants who applied dynamic handgrip performed equally well under pressure as in the baseline, whereas control participants worsened their performance. Indeed, the typical aim of using a PPR under pressure is to prevent performance failure or ‘choking under pressure’ (Gröpel & Mesagno, Citation2019). The advantage of analysing pre–post designs in the present study was that intraindividual differences of participants could be ruled out as an explanation for PPRs’ effectiveness. For experimental designs, on the other side, the advantage was that many primary studies were conducted in a randomised-controlled fashion, giving robust evidence for the effectiveness of PPRs as an intervention.
Results further revealed that the effects from (low-pressure) experimental designs and experimental design under pressure were comparable in magnitude. This indicates that using a PPR is helpful in low-pressure and pressurised situations alike, and supports that the PPR intervention can give general advantage to performance (Cotterill, Citation2010) as well as ameliorate performance-harming effects of pressure (Gröpel & Mesagno, Citation2019). However, the results from pressurised settings must be interpreted with caution because the large confidence interval indicated a lack of precision in the mean effect and the prediction interval displayed a high level of dispersion in effect sizes. This might also have been due to the relatively small number of available effect sizes.
There was initial evidence that small-study effects and questionable research practices might have played a role in retrieved studies on PPRs. Smaller studies systematically yielded larger effects in the pre–post analysis. This could indicate a publication bias and there might have been studies with small samples that yielded insignificant results and thus were not published. In addition, the contour-enhanced funnel plots displayed several studies that were just barely significant, which might be an indication of p-hacking (Simonsohn et al., Citation2014). However, applying p-uniform analyses indicated the robustness of results and the null hypothesis of no effect could be rejected for all three analyses. Consequently, the overall effect of PPR on performance did not appear to be caused by publication bias.
Regarding heterogeneity, there was not much between-study heterogeneity in analyses of pre–post and (low-pressure) experimental data, implying that effect sizes did not vary much among primary studies. In experimental designs, a three-level model was not statistically superior – apparently, there was not significantly more within-study and between-study heterogeneity than caused by sampling variance. We found significant within-study heterogeneity in pre–post designs, indicating that effect sizes varied within samples presumably due to participant or intervention characteristics. However, subsequent moderator analyses found no significant results. Subgroup analyses for the different PPR elements revealed large CI overlaps and thus no indication for a difference in their effectiveness (Supplementary Table S2). This indicates that all types of routines included in the analysis were useful for athletes and that there were no large differences in the effectiveness of separate PPR elements.
In contrast, we found a significant amount of between-study heterogeneity in analysis of experimental data under pressure, meaning that effect sizes varied among individual studies and thus some PPR elements might work better than others under pressure. However, given the large CIs for heterogeneity and the aforementioned CI overlaps in subgroup analyses, this may be rather explained by the relatively small sample size (k = 19). Thus, we have no conclusive evidence for differences related to PPR elements in experimental data under pressure. Conducting more primary studies is needed to increase the statistical power and arrive at better conclusions regarding heterogeneity.
We conducted a number of moderator analyses to explore the relationship between participant and intervention variables and the intervention effect but found no evidence for moderation. First of all, we did not find any moderating effect of the different types of routines. Extensive PPRs that incorporated several preparatory elements were equally effective as stand-alone PPRs, implying that the benefits of single preparatory elements need not cumulate when combined into an extensive PPR. This is in line with Brown and Fletcher (Citation2017) who reported that multiple part interventions and single component interventions in sports lead to similar sport-related outcomes. Consequently, having a preparatory strategy is an advantage in sports, but it does not seem to matter how simple or complex this strategy is. In addition, single PPR elements (e.g. imagery, quiet eye, dynamic handgrip) do not appear to differ in their effectiveness as a PPR, as indicated by large CI overlaps (Supplementary Table S2), but this finding must be interpreted with caution as data on single elements were scarce and the subgroup analyses might have been underpowered.
Further, several researchers emphasised the importance to individualise a PPR and discarded the idea of a one-size-fits-all approach in PPRs (Cotterill et al., Citation2010; Lidor & Mayan, Citation2005; Marlow et al., Citation1998), but we did not find individualised routines to be more effective than standardised, non-individualised PPRs. An explanation can be that the general mechanism underlying a PPR element (e.g. improved concentration, optimal arousal) matters more for performance than whether or not the element is individualised. Cotterill (Citation2008) similarly suggested, while also advocating an individualised approach, that a generic template based upon the psychological demands of a task was crucial for a routine. Consequently, the effectiveness of the PPR intervention itself does not seem to be a question of individualisation. However, we assume, and agree with the above researchers, that individualisation may be important for other reasons, such as acceptance and motivation to use the PPR. It is an avenue for future research to test whether athletes with PPRs being tailored to their individual needs or habits use these PPRs more consistently than athletes with non-individualised PPRs.
Regarding learning time of a PPR, Hazell et al. (Citation2014) have criticised that many researchers do not give participants a sufficient amount of time to learn and internalise their new routines. Indeed, several researchers used rather short learning time (10–15 minutes), which would be presumably not enough for the routine to be embedded in athletes’ everyday performance. Regarding the mere effect on study outcomes, however, we did not find learning time to moderate the PPR effectiveness. Even studies with as little as 10 minutes of PPR learning showed an effect (e.g. Mesagno et al., Citation2019; Mesagno & Mullane-Grant, Citation2010). This might be due to the PPR elements that typically require little time to be learned but are still effective, such as deep breathing and left-hand dynamic handgrip. However, the learning time was only reported in about half of the included samples (k = 27 for pre–post data, k = 18 for experimental data, and k = 9 for experimental data under pressure), which limits the generalisability of this result.
The remaining moderator analyses for participant gender, age, and skill level showed that these variables were unrelated to the PPR effect. We did not replicate Brown and Fletcher’s (Citation2017) result that gender accounted for 4% of variance in the effectiveness of psychological and psychosocial interventions in sports. As in the analysis by Brown and Fletcher, there was a surplus of all-male samples in relation to all-female samples in the included studies, but PPRs were equally effective for both men and women. Athletes’ age ranged from 11 to 40 years in the included samples and had no moderating effect on the PPR effectiveness, indicating that the PPR intervention can already be applied by young individuals. Regarding skill level, PPRs were useful for athletes of all competitive standards. Novices benefitted from routines in learning complex motor tasks just as subelite and elite competitors could facilitate their skilled performance under both low-pressure and pressurised conditions.
Applied implications
This meta-analysis displays robust empirical support for the use of PPRs, which leads to a number of implications for applied practice. First, the use of a PPR provides performance advantage – an advantage that can have substantial consequences. One successfully played dead ball can decide between win or loss in football (Hazell et al., Citation2014), and a few more made free-throws often determine the outcome of a basketball game (Blumenstein et al., Citation2018). Second, the PPR intervention proved effective for both female and male athletes, ranging from 11 to 40 years of age, across all skill levels. Consequently, the intervention can have positive performance effects for a range of athletes in their practice and competition. Third, the retrieved studies tested the PPR intervention in 14 different sports, including both individual and team sports, indicating that the intervention can have benefits for a variety of sport tasks. However, the majority of tasks tested in the studies were self-paced, closed skill tasks (e.g. golf putt, basketball free-throw), which are tasks controlled by the performer who decides on when to execute the skill. We do not have evidence for whether or not the use of a PPR also helps externally paced skills, such as to prevent forced errors after serving in tennis. Fourth, our findings can be generalised for both stand-alone and extensive PPRs and across a range of PPR elements (e.g. imagery, quiet eye, left-hand dynamic handgrip). Hence, practitioners are flexible to suggest one or combine several elements depending on the particular task and situation. Finally, from the performance point of view, it seems not necessary to individualise the PPR intervention; standardised routines help as well. This might be especially relevant for young and novice athletes who yet need to learn basic psychological skills such as the use of deep breathing and external focus of attention. However, for more experienced athletes and higher competitive levels, we would suggest to individualise their PPRs. The reason is subjective benefits of using a PPR, which may be stronger when the PPR is tailored to an athlete’s individual needs and habits (Cotterill, Citation2010). To obtain objective data, we only sampled studies that tested performance in a quantitative and objective fashion. But different qualitative studies speak for the subjective benefits of the PPR intervention, as found in interviews with, and verbal reports from, elite athletes and coaches (Cotterill, Citation2011; Cotterill et al., Citation2010; Hill et al., Citation2011; Mesagno et al., Citation2008; Oudejans et al., Citation2011).
Limitations and suggestions for future research
This study has several limitations. First, at study level, 15 studies were not included because the authors did not report sufficient data (i.e. means and standard deviations or effect sizes and corresponding p-values). Either the data was no longer available or efforts made to contact authors to retrieve the data remained unsuccessful. However, judging from the verbal reporting in the studies that were not included, these studies were not systematically different from the ones included in the final sample. Second, and partly related to the above, the moderation analyses were sometimes underpowered. This was either due to a small number of primary studies that tested a given moderator (e.g. the vast majority of articles was peer-reviewed) or due to the poor reporting (e.g. learning time was not reported in about half of retrieved studies). In addition, several studies using pre–post designs did not specify correlations between pre- and post-measurements. Future researchers are thus encouraged to present their work in accordance with established reporting guidelines (e.g. the CONSORT statement; Moher et al., Citation2012) to prevent reporting deficiencies and to adopt open science practices (Munafò et al., Citation2017). Third, there were few samples that tested the effect of the PPR intervention under pressure (k = 19) and only five of them included data from actual competitions. Consequently, the model was relatively imprecise and further studies are needed to gain more understanding of how well PPRs work on the field. Also, two studies that provided data from actual competitions did not include a true control group because the randomisation of performers was not possible (Gröpel et al., Citation2020; Gröpel & Beckmann, Citation2017); instead, a comparison group was used in these studies. Forth, there was a surplus of all-male samples and no primary study with senior athletes. Future research with more diverse samples is needed to examine whether the PPR effectiveness can be generalised across different groups of performers. Fifth, the majority of included studies did not include a follow-up. There is an evidence that the effect of PPRs on performance can be long-lasting (Cohn et al., Citation1990), but more studies with a longitudinal design are required to support long-term effects of the PPR intervention. Longitudinal studies can also shed more light on the development of routines (e.g. consistency, complexity, individualisation) over time, from novice through to expert. Sixth, in the included studies under pressure, participants learned and practiced their PPRs in a pressure-free intervention phase before the high-pressure post-test. But what if they practiced the PPRs under pressure as well? A recent meta-analysis showed that practicing a skill under pressure helped performers to adjust to pressure and enhance performance (Low et al., Citation2021). It is an important avenue for future research to test whether practicing both a PPR and a skill in pressurised training environments could have an additional (or cumulative) effect on subsequent performance under pressure. Finally, only quantitative studies were included. Quantitative studies serve well the purpose of meta-analysis, but they lack deeper insights into performers’ thoughts, needs, and feelings. The adoption of qualitative and mixed-method designs is necessary to develop a clear understanding of performers who readily use PPR as a core component of their sport performance (Cotterill, Citation2010). In addition, the precise mechanisms of how PPRs improve performance in motor tasks are an interesting avenue for future research. The majority of retrieved studies only tested performance as the outcome, without including potential mediating variables. Valuable exceptions are the studies implementing QE training in which eye-tracking technology was incorporated to measure attention (Moore et al., Citation2012; Vine et al., Citation2011; Wood & Wilson, Citation2011). Future research might benefit from mixed study designs to better understand why PPRs work: Qualitative methodologies to explore what function PPRs actually fulfil could constitute the first step, while quantitative designs, as the next step, would test the identified physiological (e.g. heart rate) and psychological changes (e.g. self-efficacy). Mixed study designs might also help to explain the observed within-study heterogeneity in pre–post designs.
Supplemental Material
Download MS Word (30.8 KB)Disclosure statement
No potential conflict of interest was reported by the author(s).
Data availability statement
The meta-analysis data can be found at https://doi.org/10.6084/m9.figshare.14077091
. The meta-analysis code and Supplementary Tables S1 and S2 can be found at https://doi.org/10.6084/m9.figshare.14076065 .References
- References preceded by an asterisk were included in the meta-analysis.
- American Psychological Association (n.d.). APA dictionary of psychology. https://dictionary.apa.org/superstitious-behavior
- Assink, M., & Wibbelink, C. J. M. (2016). Fitting three-level meta-analytic models in R: A step-by-step tutorial. The Quantitative Methods for Psychology, 12(3), 154–174. https://doi.org/10.20982/tqmp.12.3.p154
- Baumeister, R. F., & Showers, C. J. (1986). A review of paradoxical performance effects: Choking under pressure in sports and mental tests. European Journal of Social Psychology, 16(4), 361–383. https://doi.org/10.1002/ejsp.2420160405
- *Beckmann, J., Gröpel, P., & Ehrenspiel, F. (2013). Preventing motor skill failure through hemisphere-specific priming: Cases from choking under pressure. Journal of Experimental Psychology: General, 142(3), 679–691. https://doi.org/10.1037/a0029852
- *Bergmann, J., Kumpulainen, S., Avela, J., & Gruber, M. (2013). Acute effects of motor imagery on performance and neuromuscular control in maximal drop jumps. Journal of Imagery Research in Sport and Physical Activity, 8, 45–53. https://doi.org/10.1515/jirspa-2013-0001
- Blumenstein, B., Orbach, I., Moran, D., Ziv, D., & Weinstein, Y. (2016). The effect of extra-curricular mental training on self-paced and externally paced tasks in adolescent physical education students. Applied Research in Coaching and Athletics Annual, 31, 102–122.
- *Blumenstein, B., Orbach, I., Gelinski, Y., Shemer, Y., Moran, D., & Weinstein, Y. (2018). The effect of mental relaxation and imagery on free throw shooting accuracy among young basketball players. Applied Research in Coaching and Athletics Annual, 33, 1–12.
- Borenstein, M., Hedges, L. V., Higgins, J. P. T., & Rothstein, H. R. (2009). Introduction to meta-analysis. Wiley.
- Boutcher, S. H. (1992). Attention and athletic performance: An integrated approach. In T. S. Horn (Ed.), Advances in sport psychology (pp. 251–265). Human Kinetics.
- *Boutcher, S. H., & Crews, D. J. (1987). The effect of a preshot attentional routine on a well learned skill. International Journal of Sport Psychology, 18, 30–39.
- Brown, D. J., & Fletcher, D. (2017). Effects of psychological and psychosocial interventions on sport performance: A meta-analysis. Sports Medicine, 47(1), 77–99. https://doi.org/10.1007/s40279-016-0552-7
- Clowes, H., & Knowles, Z. (2013). Exploring the effectiveness of pre-performance routines in elite artistic gymnasts: A mixed method investigation. Science of Gymnastics Journal, 5(2), 27–40.
- Cohn, P. J., Rotella, R. J., & Lloyd, J. W. (1990). Effects of a cognitive-behavioral intervention on the preshot routine and performance in golf. The Sport Psychologist, 4(1), 33–47. . https://doi.org/10.1123/tsp.4.1.33
- Cotterill, S. (2008). Developing effective pre-performance routines in golf. Sport & Exercise Psychology Review, 4(2), 10–15.
- Cotterill, S. (2010). Pre-performance routines in sport: Current understanding and future directions. International Review of Sport and Exercise Psychology, 3(2), 132–153. https://doi.org/10.1080/1750984X.2010.488269
- Cotterill, S. T. (2011). Experiences of developing pre-performance routines with elite cricket players. Journal of Sport Psychology in Action, 2(2), 81–91. https://doi.org/10.1080/21520704.2011.584245
- Cotterill, S., Sanders, R., & Collins, D. (2010). Developing effective pre-performance routines in golf: Why don’t we ask the golfer? Journal of Applied Sport Psychology, 22(1), 51–64. https://doi.org/10.1080/10413200903403216
- *Crews, D. J., & Boutcher, S. H. (1986). Effects of structured preshot behaviors on beginning golf performance. Perceptual and Motor Skills, 62(1), 291–294. https://doi.org/10.2466/pms.1986.62.1.291
- Cross-Villasana, F., Gröpel, P., Doppelmayr, M., & Beckmann, J. (2016). Correction: Unilateral left-hand contractions produce widespread depression of cortical activity after their execution. PLoS One, 11(2), e0150048. https://doi.org/10.1371/journal.pone.0150048
- de Winter, J. C. F., Zadpoor, A. A., & Dodou, D. (2014). The expansion of Google scholar versus web of science: A longitudinal study. Scientometrics, 98(2), 1547–1565. https://doi.org/10.1007/s11192-013-1089-2
- Fernández-Castilla, B., Declercq, L., Jamshidi, L., Beretvas, N., Onghena, P., & Van den Noortgate, W. (2020). Visual representations of meta-analyses of multiple outcomes: Extensions to forest plots, funnel plots, and caterpillar plots. Methodology, 16(4), 299–315. https://doi.org/10.5964/meth.4013
- *Foster, D. J., Weigand, D. A., & Baines, D. (2006). The effect of removing superstitious behavior and introducing a pre-performance routine on basketball free-throw performance. Journal of Applied Sport Psychology, 18(2), 167–171. https://doi.org/10.1080/10413200500471343
- Gayman, A. M., Fraser-Thomas, J., Dionigi, R. A., Horton, S., & Baker, J. (2016). Is sport good for older adults? A systematic review of psychosocial outcomes of older adults’ sport participation. International Review of Sport and Exercise Psychology, 10(1), 164–185. https://doi.org/10.1080/1750984X.2016.1199046
- *Gayton, W. F., Cielinski, K. L., Francis-Keniston, W. J., & Hearns, J. F. (1989). Effects of preshot routine on free-throw shooting. Perceptual and Motor Skills, 68(1), 317–318. https://doi.org/10.2466/pms.1989.68.1.317
- Gleser, L. J., & Olkin, I. (2009). Stochastically dependent effect sizes. In L. V. Cooper, L. V. Hedges, & J. C. Valentine (Eds.), The handbook of research synthesis and meta-analysis (pp. 357–376). Russell Sage Foundation.
- Gröpel, P., & Mesagno, C. (2019). Choking interventions in sports: A systematic review. International Review of Sport and Exercise Psychology, 12(1), 176–201. https://doi.org/10.1080/1750984x.2017.1408134
- *Gröpel, P., & Beckmann, J. (2017). A pre-performance routine to optimize competition performance in artistic gymnastics. The Sport Psychologist, 31(2), 199–207. https://doi.org/10.1123/tsp.2016-0054
- *Gröpel, P., Mesagno, C., & Beckmann, J. (2020). Pre-shot routines to improve competition performance: A case study of a group of elite pistol shooters. Case Studies in Sport and Exercise Psychology, 4(1), 52–57. https://doi.org/10.1123/cssep.2019-0039
- *Hardy, L., & Callow, N. (1999). Efficacy of external and internal visual imagery perspectives for the enhancement of performance on tasks in which form is important. Journal of Sport and Exercise Psychology, 21(2), 95–112. https://doi.org/10.1123/jsep.21.2.95
- Harrer, M., Cuijpers, P., Furukawa, T. A., & Ebert, D. D. (2019). Doing meta-analysis in R: A hand-on guide. https://bookdown.org/MathiasHarrer/Doing_Meta_Analysis_in_R/
- Hatzigeorgiadis, A., Zourbanos, N., Galanis, E., & Theodorakis, Y. (2011). Self-talk and sports performance: A meta-analysis. Perspectives on Psychological Science, 6(4), 348–356. https://doi.org/10.1177/1745691611413136
- *Hazell, J., Cotterill, S. T., & Hill, D. M. (2014). An exploration of pre-performance routines, self-efficacy, anxiety and performance in semi-professional soccer. European Journal of Sport Science, 14(6), 603–610. https://doi.org/10.1080/17461391.2014.888484
- Hill, D. M., Hanton, S., Matthews, N., & Fleming, S. (2010). A qualitative exploration of choking in elite golf. Journal of Clinical Sport Psychology, 4(3), 221–240. https://doi.org/10.1123/jcsp.4.3.221
- Hill, D. M., Hanton, S., Matthews, N., & Fleming, S. (2011). Alleviation of choking under pressure in elite golf: An action research study. The Sport Psychologist, 25(4), 465–488. https://doi.org/10.1123/tsp.25.4.465
- *Hill, K. L., & Borden, F. (1995). The effect of attentional cueing scripts on competitive bowling performance. International Journal of Sport Psychology, 26, 503–512.
- *Klug, J. J. (2006). Effects of an imagery training program on free throw self-efficacy and performance of high school basketball players [Doctoral thesis]. Miami University, Oxford, OH. https://etd.ohiolink.edu/!etd.send_file?accession=miami1156375861&disposition=inline
- *Kornspan, A. S., Overby, L., & Lerner, B. S. (2004). Analysis and performance of pre-performance imagery and other strategies on a golf putting task. Journal of Mental Imagery, 28, 59–74.
- *Lautenbach, F., Laborde, S., Mesagno, C., Lobinger, B. H., Achtzehn, S., & Arimond, F. (2015). Nonautomated pre-performance routine in tennis: An intervention study. Journal of Applied Sport Psychology, 27(2), 123–131. https://doi.org/10.1080/10413200.2014.957364
- Lebeau, J.-C., Liu, S., Sáenz-Moncaleano, C., Sanduvete-Chaves, S., Chacón-Moscoso, S., Becker, B. J., & Tenenbaum, G. (2016). Quiet eye and performance in sport: A meta-analysis. Journal of Sport and Exercise Psychology, 38(5), 441–457. https://doi.org/10.1123/jsep.2015-0123
- Lidor, R., & Mayan, Z. (2005). Can beginning learners benefit from preperformance routines when serving in volleyball? The Sport Psychologist, 19(4), 343–363. https://doi.org/10.1123/tsp.19.4.343
- Lonsdale, C., & Tam, J. T. M. (2008). On the temporal and behavioural consistency of preperformance routines: An intra-individual analysis of elite basketball players’ free throw shooting accuracy. Journal of Sports Sciences, 26(3), 259–266. https://doi.org/10.1080/02640410701473962
- Low, W. R., Sandercock, G. R. H., Freeman, P., Winter, M. E., Butt, J., & Maynard, I. (2021). Pressure training for performance domains: A meta-analysis. Sport, Exercise, and Performance Psychology, 10(1), 149–163. https://doi.org/10.1037/spy0000202
- Macnamara, B. N., Moreau, D., & Hambrick, D. Z. (2016). The relationship between deliberate practice and performance in sports: A meta-analysis. Perspectives on Psychological Science, 11(3), 333–350. https://doi.org/10.1177/1745691616635591
- *Malouff, J. M., McGee, J. A., Halford, H. T., & Rooke, S. E. (2008). Effects of pre-competition positive imagery and self-instructions on accuracy of serving in tennis. Journal of Sport Behavior, 31, 264–275.
- *Marlow, C., Bull, S. J., Heath, B., & Shambrook, C. J. (1998). The use of a single case design to investigate the effect of a pre-performance routine on the water polo penalty shot. Journal of Science and Medicine in Sport, 1(3), 143–155. https://doi.org/10.1016/S1440-2440(98)80010-8
- *McCann, P., Lavallee, D., & Lavallee, R. (2001). The effect of pre-shot routines on golf wedge shot performance. European Journal of Sport Science, 1(5), 1–10. https://doi.org/10.1080/17461390100071503
- *Mesagno, C., Beckmann, J., Wergin, V. V., & Gröpel, P. (2019). Primed to perform: Comparing different pre-performance routine interventions to improve accuracy in closed, self-paced motor tasks. Psychology of Sport and Exercise, 43, 73–81. https://doi.org/10.1016/j.psychsport.2019.01.001
- *Mesagno, C., Hill, D. M., & Larkin, P. (2015). Examining the accuracy and in-game performance effects between pre- and post-performance routines: A mixed methods study. Psychology of Sport and Exercise, 19, 85–94. https://doi.org/10.1016/j.psychsport.2015.03.005
- *Mesagno, C., Marchant, D., & Morris, T. (2008). A pre-performance routine to alleviate choking in “choking-susceptible” athletes. The Sport Psychologist, 22(4), 439–457. https://doi.org/10.1123/tsp.22.4.439
- *Mesagno, C., & Mullane-Grant, T. (2010). A comparison of different pre-performance routines as possible choking interventions. Journal of Applied Sport Psychology, 22(3), 343–360. https://doi.org/10.1080/10413200.2010.491780
- Moher, D., Hopewell, S., Schulz, K. F., Montori, V., Gøtzsche, P. C., Devereaux, P. J., Elbourne, D., Egger, M., & Altman, D. G. (2012). CONSORT 2010 explanation and elaboration: Updated guidelines for reporting parallel group randomised trials. International Journal of Surgery, 10(1), 28–55. https://doi.org/10.1016/j.ijsu.2011.10.001
- Moher, D., Liberati, A., Tetzlaff, J., & Altman, D. G. (2009). Preferred reporting items for systematic reviews and meta-analyses: The PRISMA statement. PLoS Medicine, 6(7), e1000097. https://doi.org/10.1371/journal.pmed1000097
- *Moore, L. J., Vine, S. J., Cooke, A., Ring, C., & Wilson, M. R. (2012). Quiet eye training expedites motor learning and aids performance under heightened anxiety: The roles of response programming and external attention. Psychophysiology, 49(7), 1005–1015. https://doi.org/10.1111/j.1469-8986.2012.01379.x
- *Morais, C., & Gomes, A. R. (2019). Pre-service routines, mental toughness and performance enhancement of young tennis athletes. International Journal of Sport Psychology, 50, 176–192. https://doi.org/10.7352/IJSP.2019.50.176
- Moran, A. P. (1996). The psychology of concentration in sport performers: A cognitive analysis. Psychology Press.
- Morris, S. B. (2000). Distribution of the standardized mean change effect size for meta-analysis on repeated measures. British Journal of Mathematical and Statistical Psychology, 53(1), 17–29. https://doi.org/10.1348/000711000159150
- Morris, S. B. (2008). Estimating effect sizes from pretest-posttest-control group designs. Organizational Research Methods, 11(2), 364–386. https://doi.org/10.1177/1094428106291059
- Morris, T., Spittle, M., & Watt, A. P. (2005). Imagery in sport. Human Kinetics.
- Munafò, M. R., Nosek, B. A., Bishop, D. V. M., Button, K. S., Chambers, C. D., du Sert, P., Simonsohn, N., Wagenmakers, U., Ware, E.-J., & Ioannidis, J. J., & A, J. P. (2017). A manifesto for reproducible science. Nature Human Behaviour, 1(1), e0021. https://doi.org/10.1038/s41562-016-0021
- National Basketball Association. (2019). NBA advanced stats. https://stats.nba.com/player/893/career/
- Oudejans, R. R. D., Kuijpers, W., Kooijman, C. C., & Bakker, F. C. (2011). Thoughts and attention of athletes under pressure: Skill-focus or performance worries? Anxiety, Stress & Coping, 24(1), 59–73. https://doi.org/10.1080/10615806.2010.481331
- Perry, I. S., & Katz, Y. J. (2015). Personality characteristics and motor-mental pre-performance routines on accuracy in sports. https://www.researchgate.net/profile/Yaacov_Katz2/publication/282846849_Pre-Performance_Routines_Accuracy_in_Athletic_Performanceand_Self-Control/links/5881b2894585150dde3f4a40/Pre-Performance-Routines-Accuracy-in-Athletic-Performanceand-Self-Control
- *Perry, I. S., Chow, G. M., Tenenbaum, G., & Katz, Y. J. (2018). The effect of motor-mental preperformance routines on motor performance in novice learners. Journal of Applied Sport Psychology, 30(4), 402–420. https://doi.org/10.1080/10413200.2017.1411404
- *Phelps, A., & Kulinna, P. (2015). Pre- performance routines followed by free throw shooting accuracy in secondary basketball players. Biomedical Human Kinetics, 7. https://doi.org/10.1515/bhk-2015-0025
- Pluye, P., Robert, E., Cargo, M., Bartlett, G., O’Cathain, A., Griffiths, F., Boardman, F., Gagnon, M. P., Rousseau, M. C., & Robert, E. (2011). Proposal: A mixed methods appraisal tool for systematic mixed studies reviews. http://mixedmethodsappraisaltoolpublic.pbworks.com
- *Predebon, J., & Docker, S. B. (1992). Free-throw shooting performance as a function of preshot routines. Perceptual and Motor Skills, 75(1), 167–171. https://doi.org/10.2466/PMS.75.4.167-171
- Prins, A. A. M., Costas, R., van Leeuwen, T. N., & Wouters, P. F. (2016). Using Google Scholar in research evaluation of humanities and social science programs: A comparison with Web of Science data. Research Evaluation, 25(3), 264–270. https://doi.org/10.1093/reseval/rvv049
- Simonsmeier, B. A., Androniea, M., Buecker, S., & Frank, C. (2020). The effects of imagery interventions in sports: A meta-analysis. International Review of Sport and Exercise Psychology, 1–22. https://doi.org/10.1080/1750984X.2020.1780627
- Simonsohn, U., Nelson, L. D., & Simmons, J. P. (2014). P-curve: A key to the file-drawer. Journal of Experimental Psychology: General, 143(2), 534–547. https://doi.org/10.1037/a0033242
- Singer, R. N. (2002). Preperformance state, routines, and automaticity: What does it take to realize expertise in self-paced events? Journal of Sport and Exercise Psychology, 24(4), 359–375. https://doi.org/10.1123/jsep.24.4.359
- *Thomas, J. A. (2010). Considering intervention efficacy: The effect of a pre-shot routine on competitive youth golf performance [Doctoral thesis]. University of North Carolina., Chapel Hill, NC. Proquest Dissertations and Theses database. (Publication No. 3434198).
- van Assen, M. A. L. M., van Aert, R. C. M., & Wicherts, J. M. (2014). Meta-analysis using effect size distributions of only statistically significant studies. Psychological Methods, 20(3), 293–309. https://doi.org/10.1037/met0000025
- Veroniki, A. A., Jackson, D., Viechtbauer, W., Bender, R., Bowden, J., Knapp, G., Kuss, O., Higgins, J. P. T., Langan, D., & Salanti, G. (2016). Methods to estimate the between-study variance and its uncertainty in meta-analysis. Research Synthesis Methods, 7(1), 55–79. https://doi.org/10.1002/jrsm.1164
- Viechtbauer, W. (2010). Conducting meta-analyses in R with the metafor package. Journal of Statistical Software, 36(3), 1–48. https://doi.org/10.18637/jss.v036.i03
- Vine, S. J., Moore, L. J., Cooke, A., Ring, C., & Wilson, M. R. (2013). Quiet eye training: A means to implicit motor learning. International Journal of Sport Psychology, 44, 367–386. https://doi.org/10.7352/IJSP2013.44.367
- Vine, S. J., & Wilson, M. R. (2010). Quiet eye training: Effects on learning and performance under pressure. Journal of Applied Sport Psychology, 22(4), 361–376. https://doi.org/10.1080/10413200.2010.495106
- *Vine, S. J., Moore, L. J., & Wilson, M. R. (2011). Quiet eye training facilitates competitive putting performance in elite golfers. Frontiers in Psychology, 2, 8. https://doi.org/10.3389/fpsyg.2011.00008
- *Wergin, V. V., Beckmann, J., Gröpel, P., & Mesagno, C. (2020). Investigating cumulative effects of pre-performance routine interventions in beach volleyball serving. PLoS One, 15, e0228012. https://doi.org/10.1371/journal.pone.0228012
- *Wood, G., & Wilson, M. R. (2011). Quiet-eye training for soccer penalty kicks. Cognitive Processing, 12(3), 257–266. https://doi.org/10.1007/s10339-011-0393-0
- *Wrisberg, C. A., & Anshel, M. H. (1989). The effect of cognitive strategies on the free throw shooting performance. The Sport Psychologist, 3(2), 95–104. https://doi.org/10.1123/tsp.3.2.95
- www.ussportscamps.com. Golf tip: Tiger Woods pre-shot routine. https://www.ussportscamps.com/tips/golf/golf-tip-tiger-woods-pre-shot-routine
- *Yeemin, W., Kemarat, S., & Theanthong, A. (2020). The effects of post activation potentiation warm-up and pre-shot routine programs on driving performance in amateur golfers. Plos One, 15(10), e0240881. https://doi.org/10.1371/journal.pone.0240881