ABSTRACT
Research on the effects of exercise on cortical activity has grown significantly, with a predominant focus on the technique of electroencephalography (EEG). Technological advancements in EEG render this technique suitable for studies that explore human movement. There is a noticeable gap in the literature concerning how EEG-recorded cortical oscillatory activity is affected by exercise. Accordingly, the aim of this review was to summarise studies that investigated the effects of exercise on brain activity using EEG. This was a systematic review of 47 studies, predicated on PRISMA guidelines. The results were, on the whole, equivocal in nature, with the exception that activity in the alpha and beta frequency bands increased both during and post-exercise across the frontal, central, and limbic regions. The reported increases could be attributed to a variety of neural processes, such as neuro-connectivity, cortical inhibition, and sensory feedback loops. Future researchers need to give careful consideration to exercise parameters (e.g. mode of exercise, intensity, duration) to facilitate their interpretation of experimental data. The present findings support the notion that exercise alters alpha and beta neural oscillations but more work is needed to provide a fuller picture of the brain processes that manifest during exercise.
Introduction
Electroencephalography (EEG) has provided the research community with a vista into the electrical activity of the brain. It has also served to further understanding of the dynamic nature in which this most complex of organs functions. Given the high temporal sensitivity of this method, EEG has been widely used in the assessment of brain function and human behaviour (Babiloni et al., Citation2002; Bevilacqua et al., Citation2019). In the field of sport and exercise sciences in particular, the use of EEG has gained significant traction, as it affords easy access to an ‘under-the-bonnet’ view of human functioning (Cheval et al., Citation2018; Cooke et al., Citation2014). This review therefore aims to provide important insights into emerging trends regarding the recording of brain activity using EEG, as well as the strengths and limitations that characterise the extant literature, in order to stimulate future research.
The EEG signal is produced when multiple brain cells generate electrical activity that incorporates an array of frequencies. EEG is able to detect neural activity, which results from both excitatory and inhibitory postsynaptic potentials; thus, an EEG recording results from multiple frequencies created by the electrical activity of groups of neurons firing in synchronicity (Bigliassi et al., Citation2021). One of the data screening processes, conducted through frequency-domain analyses such as Fast Fourier transform (FFT), enables the brain frequencies to be distinguished in relation to different band waves.
The band waves include delta (0.5–4 Hz), theta (4.5–8 Hz), alpha (8.5–13 Hz), beta (13.5–30 Hz), and gamma waves (30.5–100 Hz; Steriade et al., Citation1990). Alpha and beta ranges are sometimes subdivided to discriminate alpha-1 (8.5–10 Hz), alpha-2 (10–13 Hz), beta-1 (12–15 Hz), and beta-2 (15–20 Hz). Delta waves are slow-frequency waves normally associated with stages of deep sleep. Theta waves indicate that an individual is in a state of deep relaxation, such as sleep or meditation (Kochupillai, Citation2015). Theta waves also provide a basis for sensorimotor integration and coding of spatial information (Bland & Oddie, Citation2001; Buzsáki & Moser, Citation2013). Although alpha waves can be recorded during sleep, they are normally more prominent when an individual is in a relaxed mental state (Desai et al., Citation2015). In addition, alpha frequency plays a key role in top-down control of information processing and is thought to be a central timing mechanism for cognitive processes (Klimesch et al., Citation1996; Samaha et al., Citation2015). More specifically, alpha-1 waves (8.5–10 Hz) are often observed when an individual is in an alert, yet relaxed state, while alpha-2 waves (10–13 Hz) are observed during deep relaxation (e.g. meditative states; Srinivasan & Nunez, Citation2017).
Beta waves are present during normal waking consciousness, as they are generally observed throughout the completion of a task and during active concentration. Beta oscillatory activity can also be seen during slow movements and isotonic contractions (De & Mondal, Citation2020). More specifically, beta-1 waves (12–15 Hz) are usually associated with introverted, quiet concentration as well as motor planning and control (Kropotov, Citation2016), while beta-2 waves (15–20 Hz) are linked to increases in anxiety, performance, and energy levels (Abhang et al., Citation2016). Finally, gamma waves are indicative of increased attention and usually present during the execution of higher cognitive tasks (e.g. listening attentively to a lecture; Fitzgibbon et al., Citation2004).
EEG in the exercise and physical activity domain
This review refers to ‘physical activity’ and ‘exercise’ in terms of the World Health Organization (Citation2010) definitions. Physical activity is defined as ‘ … any bodily movement produced by skeletal muscles that requires energy expenditure.’ (p. 53) and exercise as ‘A subcategory of physical activity that is planned, structured, repetitive, and purposeful in the sense that the improvement or maintenance of one or more components of physical fitness is the objective.’ (p. 52). The definition considered for sport relates to ‘An activity involving physical exertion and skill (…) one regulated by set rules or customs in which an individual competes against another or others.’ (Oxford English Dictionary, Citation2021). The present review is more strongly predicated on exercise than either physical activity or sport.
For many years, EEG was deemed unsuitable for the analysis of brain activity during exercise. The reason for this is that study participants were generally required to sit relatively still in a quiet environment, in order to minimise movement artefacts (Enders & Nigg, Citation2016a). Recent technological advances have rendered EEG far more suitable to the collection of recordings during dynamic brain activities (Bigliassi et al., Citation2021). For example, EEG sensors allow for the signal to be amplified at source, they are more noise robust (vis-à-vis noise in the electrical signal) and smaller in size. Moreover, the number of EEG sensors applied has been increased from 32 to high-density grids comprised of up to 264 electrodes (Castermans et al., Citation2014), allowing for higher sensitivity and improved source localisation.
An important advancement for the field of sport and exercise science was the adoption of dry EEG in the early 1990s (Di Flumeri et al., Citation2019). The advantages of this method include improved signal-to-noise ratio as well as no requirements for skin preparation (e.g. shaving of the scalp) or the application of conductive paste (Taheri et al., Citation1994). This upstaging feature of dry EEG meant that experiments took less set-up time, as the cleaning of electrodes was made much easier. Moreover, dry EEG has proven to be less sensitive to electromagnetic intrusion from outside noise (Hinrichs et al., Citation2020), which has facilitated a stream of studies in real-world environments (e.g. cityscapes, Tilley et al., Citation2017; roads, Protzak & Gramann, Citation2018; running tracks, Bigliassi et al., Citation2019).
EEG has been used extensively to further understanding of the underlying effects of exercise on cognitive processing and most studies adopted a pre- vs. post-exercise design (e.g. Devilbiss et al., Citation2019; Hicks et al., Citation2018). Accordingly, this is one of the points of comparison in the present systematic review. The comparision of neural oscillations pre- vs. post-exercise will provide valuable insights in regard to the immediate effects of acute exercise, defined as a single bout of exercise (Sellami et al., Citation2018) on brain functioning. Nonetheless, the cognitive processes that occur during exercise have also been suggested to be of particular relevance in predicting exercise-related behaviours (e.g. regular engagement; Buckley et al., Citation2014). Similarly, the effects of long-duration exercise explored in the present review have also been a topic of interest in this field of study (e.g. Chaire et al., Citation2020; Sousa et al., Citation2019). Given that EEG recordings are prone to the vagaries of movement artefacts (Enders & Nigg, Citation2016a), studies investigating cortical activity during exercise were placed in a specific category in this review. The way by which exercise appears to modulate cortical oscillations is dependent on its intensity (see Ekkekakis, Citation2009), which constitutes a further focus of the present review. Moreover, the review addresses the effects of exercise per se on cortical activity through comparing exercise vs. no exercise conditions (e.g. Kao et al., Citation2020).
Although several EEG-related reviews have appeared in the exercise science literature (e.g. Crabbe & Dishman, Citation2004; Gramkow et al., Citation2020; Rahman et al., Citation2019), there has been no systematic review into the effects of exercise on cortical oscillatory activity, with a sole focus on healthy populations. Given that exercise has been shown to have an effect on brain plasticity and, in particular, cortical oscillatory activity, there is a need to summarise studies that report effects of this nature. With the intention to identify common patterns in brain activation, the main purpose of this systematic review was to further knowledge pertaining to the modulation of cortical oscillatory activity due to exercise. Effect sizes (Cohen’s d or partial eta squared [ηp2]) pertaining to each statistical test were computed and are reported herein.
Method
The systematic review was performed following the recommended Preferred Reporting Items in Systematic Reviews (PRISMA) guidelines (Moher et al., Citation2010). Note that this study was not pre-registered.
Search strategy and study selection
Articles were searched using Google Scholar, Medline, PsycINFO, PubMed, and SPORTDiscus for the period of 1 January 1986–31 March 2022 using Boolean logic and the following search terms: ‘EEG OR Electroencephalogram OR Electroencephalography’ AND ‘exercise OR physical activity’ AND ‘oscillation’. A manual search of the references sections of the initially retrieved articles (n = 50) was also conducted, which resulted in an additional 31 studies (n = 81). Of the 81 studies, five were duplicates, leading to the screening of titles and abstracts of 76 studies, of which 29 were removed for not meeting the inclusion criteria. The remaining 47 studies were included in the review. The searches were conducted by the first author in consultation with the last author.
Data extraction
A MicrosoftTM Excel spreadsheet was used to extract basic information in relation to the retrieved studies (n = 81). For each study, the following informational elements were extracted: published year, number of participants, sex of participants, mean age, study design, intervention type (physical exercise type, intensity, duration), EEG paradigm (data processing and analysis method, number of electrodes used, artefact correction method), and main results. These elements are presented in . Informational elements that were unavailable are clearly indicated within each revelant table to acknowledge risk of bias in a transparent manner. Duplicates and studies that did not meet the criteria were highlighted in red with the reason for removal identified and written next to the study title. The remaining 47 studies were then categorised as follows: (a) pre- vs. post-exercise condition (n = 37); (b) pre-/post- vs. during-exercise condition (n = 15); (c) comparisons among different exercise intensities (n = 43); (d) exercise vs. control group (n = 6). A MicrosoftTM Excel spreadsheet was used to ascertain which studies were eligible for each synthesis category; specifically by colour coding each study according to the intervention characteristics.
Table 1. Characteristics of Studies.
Table 2. Results From Studies Investigating Pre- and Post-Exercise Effects
Table 3. Results From Studies Investigating Effects During Exercise
Table 4. Results From Studies Investigating Effects of Exercise Intensity
Table 5. Results From Studies Investigating Effects of Exercise vs. No Exercise
Quality assessment
Study quality was assessed by the first and last authors. A modified version of the Quality Assessment Tool for Quantitative Studies (QATQS; National Collaborating Center for Methods and Tools, Citation2008) was used, with additional quality assessment criteria pertaining to EEG (see Parr et al., Citation2021). The modified QATQS allows researchers to assess study quality with reference to 11 componentsFootnote1 (i.e. selection bias, study design, confounders, blinding, data collection method, withdrawals and dropouts, artefact handling, temporal precision, brain wave definitionFootnote2, regional specificity, and controls to volume conduction). Each component is attached to a scale ranging from 1 (strong) to 3 (weak). The quality rating for each component was assessed and scores then averaged to assign each study with a global quality score of 1 (high quality), 2 (moderate quality), or 3 (low quality). It is noteworthy that double-blind or placebo-controlled designs are not adopted in this literature (see e.g. Renjith, Citation2017; Sedlmeier et al., Citation2012). Nonetheless, blinding represents ∼9% of the total QATQS score, which, invariably, has a bearing upon the quality rating assigned to studies in this literature.
Eligibility criteria
The criteria for studies to be considered for inclusion were as follows: (a) full papers published in English; (b) studies using healthy human adults as participantsFootnote3; (c) studies conducted in an individual exercise or sport setting (indoor [n = 44] and outdoor [n = 3]); (d) studies comprised of physical exercise interventions that entailed analysis of cortical oscillatory activity through the use of EEG.
Studies were excluded if they were characterised by aims that were too specific and thus unrelated to the purpose of the present review; for example, motor memory (Dal Maso et al., Citation2018), motor imagery (Wriessnegger et al., Citation2020), motor learning (Gallicchio et al., Citation2017), and the effects of exercise on sleep (Menicucci et al., Citation2016). Given that a full complement of methodological detail was needed from each study for an in-depth review and synthesis of results, full published papers were the focus of this study, while published abstracts were not considered for inclusion. Theses were also not considered for inclusion due to the fact that they are not subject to peer review.
Analyses
To illustrate the meaningfulness of each effect reported herein, we include effect sizes. Where authors provided sufficient information (i.e. means and standard deviations), we calculated Cohen’s d using an online calculator (https://www.polyu.edu.hk/mm/effectsizefaqs/calculator/calculator.html). In other instances, where we had an F ratio, we calculated partial eta squared (ηp2), again by use of an online calculator (https://effect-size-calculator.herokuapp.com). Effect sizes presented as Cohen’s d were interpreted according to Cohen’s (Citation1988) guidelines. Accordingly, d < 0.5 was considered small, d ≥ 0.5 was considered medium, and d ≥ 0.8 was considered large. In instances where an effect size was 0.45, we rounded it up to one decimal point (i.e. 0.5), thus considering it medium in size. Where effect sizes were presented as partial eta squared (ηp2), in accord with Cohen’s (Citation1988) guidelines, ηp2 = .01 is considered small, ηp2 = .06 is considered medium, and ηp2 = .14 is considered large.
Results
Of the 81 abstracts and journal articles retrieved from the searches, five were duplicates and 29 were deemed irrelevant for (a) not meeting the inclusion criteria (review [n = 1]; (b) applying an intervention that was not exercise/physical activity/sport related [n = 7]; (c) targeting the wrong population (i.e. children) [n = 6]; (d) stating aims that did not align with those of the present review (i.e. investigating sleep-related oscillations) [n = 10]; and (e) not investigating oscillations [n = 5]), leaving 47 relevant studies. The screening and selection process is illustrated in . The selected 47 studies were then categorised as follows: (a) pre- vs. post-exercise condition (n = 37); (b) pre-/post- vs. during-exercise condition (n = 15); (c) comparisons among different exercise intensities (n = 43); (d) exercise vs. control group (n = 6). The quality rating for each study, along with relevant descriptive statistics, can be found in Supplemental Material 1.
Exercise intervention and analytical methods of included studies
The intervention procedures from the 47 included studies, along with their main findings and associated effect sizes are shown in . Given that some studies investigated several exercise modes/intensities and/or applied multiple analytical techniques, it is the number of effects that is most relevant herein, rather than the number of studies per se. The number of effects will be expressed by use of ‘k’ hereafter.
Figure 2. Total Percentage of Studies Across Methods of Analysis, Exercise Intensity and Exercise Mode.
Note. (s)LORETA = (standardised) low-resolution electromagnetic tomography; iAPF = individual alpha peak frequency; RPE = rating of perceived exertion; HRmax = maximal heart rate.
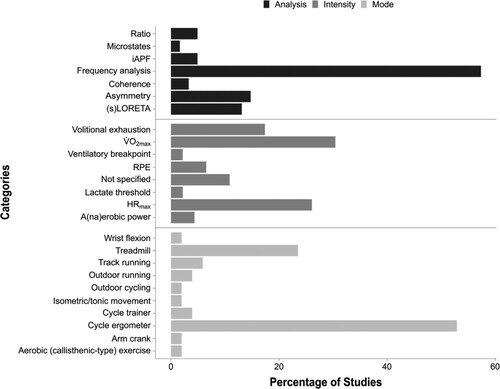
The most frequently investigated exercise mode was cycle ergometry (k = 27), followed by treadmill walking/running (k = 12), track running (k = 3), outdoor running (k = 3), cycle trainer (k = 2), arm crank (k = 1), isokinetic dynamometer wrist flexion (k = 1), isometric and isotonic movements (k = 1), aerobic (callisthenic-type) exercise (k = 1), and outdoor cycling (k = 1; see ). Most studies (n = 39) used acute exercise interventions, while the remaining eight studies used acute exercise interventions over the course of 4–16 weeks, with the exception of one study that used a long-duration acute intervention (Wollseiffen et al., Citation2016b; see ).
The markers used in terms of exercise intensity were: (a) V̇O2max (k = 14); (b) percentages of maximal heart rate (HRmax; k = 12); (c) exercising until volitional exhaustion (k = 8); (d) rating of perceived exertion using a version of Borg’s RPE Scale; (k = 3); (e) maximal aerobic/anaerobic power (k = 2); (f) reaching lactate threshold (k = 1); (g) reaching the ventilatory breakpoint (k = 1). The remaining studies did not report a marker for exercise intensity (k = 5). In order to analyse the effects of different exercise intensities on electrical activity in the brain, studies were categorised into high (≥ 70% V̇O2 max), moderate (56–69% V̇O2 max), and low-intensity exercise (≤ 55% V̇O2 max) bands, according to the American College of Sports Medicine (ACSM, Citation2013) guidelines. Given that some studies used heart-rate based parameters to establish exercise intensity, we used data from Swain et al. (Citation1994) and thus associated 63%, 76%, 89%, and 92% HRmax with 40%, 60%, 80%, and 85% V̇O2max, respectively. The most common analytical method used within the included studies was frequency analysis (k = 35), followed by asymmetry (k = 9), low resolution electromagnetic tomography ([s]LORETA; k = 8), individual peak alpha frequency (k = 3), alpha/beta ratio (k = 3), coherence (k = 2), and microstates (k = 1).
Pre- and post-exercise studies
Alpha activity
The most common effect observed among the studies investigating the effect of pre- vs. post-exercise was a significant increase in alpha brainwave activity (see ). Of the 37 studies in the pre- vs. post-exercise category, 24 reported increases in alpha peak and/or power across the bilateral frontal cortex (k = 7; e.g. Brümmer et al., Citation2011; Chaire et al., Citation2020), left frontal cortex (k = 2; Petruzzello & Landers, Citation1994; Schneider et al., Citation2010a), right prefrontal cortex (k = 1; Contreras-Jordán et al., Citation2022), bilateral frontal and central cortices (k = 1; Bailey et al., Citation2008), anterior cingulate cortex (k = 1; Moraes et al., Citation2011), limbic lobe (k = 1; Guimarães et al., Citation2015), bilateral parietal cortex (k = 3; e.g. Brümmer et al., Citation2011), bilateral central and parietal cortices (k = 3; e.g. Fumoto et al., Citation2010), bilateral temporal cortex (k = 1; Boutcher & Landers, Citation1988), posterior regions (k = 1; Dishman et al., Citation2010), occipital cortex (k = 2; Büchel et al., Citation2021; Schneider et al., Citation2009a), and whole brain (k = 6; e.g. Gutmann et al., Citation2018a; Wollseiffen et al., Citation2016b). Four studies reported increased frontal alpha asymmetry (e.g. Hicks et al., Citation2018; Woo et al., Citation2009), while three studies found no differences (e.g. Petruzzello & Tate, Citation1997). All studies observing increases in alpha peak and/or power reported large effect sizes (d ≥ 0.8; ηp2 ≥ .14 [Cohen, Citation1988]), with the exception of Boutcher and Landers (Citation1988), who reported a large effect for their running task (non-runners: d = 1.64 and runners: d = 0.81), and a small effect for the non-exercise task (reading; non-runners and runners: d = 0.16 [see ]).
Three studies found a decrease in alpha across the left frontal cortex (Woo et al., Citation2010), bilateral frontal cortex (for athletes with spinal cord injuries; Brümmer et al., Citation2011), and in the whole brain (Hottenrott et al., Citation2013). In addition, Ohmatsu et al. (Citation2014) and Schneider et al. (Citation2010a) reported a decrease in alpha-2 in the anterior cingulate cortex and left inferior temporal gyrus, respectively. All studies that observed a decrease in alpha reported large effect sizes, which ranged from d = 0.99 (Schneider et al., Citation2010a ) to d = 2.35 (Brümmer et al., Citation2011), and included ηp2 = .62 (Woo et al., Citation2010; see ). Seven studies reported no significant changes in alpha activity pre- vs. post-exercise in the left prefrontal cortex (Devilbiss et al., Citation2019), anterior region of the brain (Dishman et al., Citation2010), bilateral frontal and temporal cortices (Kubitz & Mott, Citation1996), bilateral frontal and parietal cortices (Petruzzello & Tate, Citation1997), and in the whole brain (k = 3; e.g. Mierau et al., Citation2009).
Beta activity
Eleven studies reported an increase in beta activity across the left prefrontal cortex (k = 1; Devilbiss et al., Citation2019), right frontal cortex (k = 1; Contreras-Jordán et al., Citation2022), bilateral frontal and right central cortex (k = 1; Moraes et al., Citation2007), bilateral cingulate cortex (k = 1; Moraes et al., Citation2011), limbic lobe (k = 2; Guimarães et al., Citation2015; Schneider et al., Citation2009b), bilateral parietal cortex (k = 3; e.g. Schneider et al., Citation2009b), central regions (k = 1; Contreras-Jordán et al., Citation2022), posterior regions (k = 1; Dishman et al., Citation2010), and whole brain (k = 2; Hilty et al., Citation2011; Spring et al., Citation2017). All studies observing an increase in beta activity that reported effect sizes, found these to be large (e.g. d = 1.16 [Brümmer et al., Citation2011]; d = 2.97 [Moraes et al., Citation2011]; ηp2 = .40 [Spring et al., Citation2017]; see ). Six studies reported a decrease in beta activity across the bilateral frontal cortex (Brümmer et al., Citation2011; Wollseiffen et al., Citation2016b), right frontal and temporal cortices (Schneider et al., Citation2009a), bilateral temporal cortex (Schneider et al., Citation2010a), and whole brain (Hottenrott et al., Citation2013; Mierau et al., Citation2009). All studies observing a decrease in beta activity that included effect sizes, reported large effects that ranged from d = 0.92 (Schneider et al., Citation2010a) to d = 2.12 (Brümmer et al., Citation2011), with two exceptions: Schneider et al. (Citation2009a), who reported medium effects (ηp2 = .08 and ηp2 = .07 for beta-1 and beta-2, respectively), and Wollseiffen et al. (Citation2016a) who reported a medium effect (ηp2 = .07) for beta-1 and a small effect (ηp2 = .03) for beta-2 (see ). Three studies reported no significant changes in beta power across the bilateral prefrontal cortex (Wollseiffen et al., Citation2016a), bilateral frontal and temporal cortices (Kubitz & Mott, Citation1996), and in the whole brain (only for beta-2; Schneider et al., Citation2010a).
Delta activity
For delta activity, four studies reported an increase in the bilateral frontal cortex (Woo et al., Citation2009), frontal and central cortices (Mechau et al., Citation1998), limbic lobe (Schneider et al., Citation2010b), and in the whole brain (Schneider et al., Citation2010a), while Spring et al. (Citation2017) reported a decrease across the whole brain. Two studies observing delta changes reported large effects of ηp2 = .24 (Spring et al., Citation2017) and ηp2 = .58 (Woo et al., Citation2009; see ). Two studies reported no significant changes in delta activity (Devilbiss et al., Citation2019; Moraes et al., Citation2011).
Gamma activity
Two studies reported a reduction in gamma activity in the left prefrontal cortex (Devilbiss et al., Citation2019) and left cuneus (Schneider et al., Citation2010a). Three studies reported no significant changes for theta activity (e.g. Moraes et al., Citation2011), while four reported an increase after exercise in the bilateral frontal cortex (k = 2; Contreras-Jordán et al., Citation2022; Woo et al., Citation2009), temporal cortex (Schneider et al., Citation2010a) and whole brain (Spring et al., Citation2017). Among the studies that observed changes in gamma activity, only one (Schneider et al., Citation2010a) provided effect sizes, and these were large: d = 0.97 for the pre- vs. immediately post-exercise comparison and d = 1.67 for the pre- vs. post-15-min exercise comparison (see ).
Theta activity
Three studies found a decrease in theta waves across the left prefrontal cortex (Devilbiss et al., Citation2019), central and parietal cortices (Fumoto et al., Citation2010), and in the whole brain (Hottenrott et al., Citation2013). Only one study reported effect sizes (Fumoto et al., Citation2010), and these were found to be large in relation to both central and parietal cortices (d = 1.86 and d = 1.83, respectively). In addition, Contreras-Jordán et al. (Citation2022) reported an increase in theta activity across the bilateral prefrontal cortex from pre- to post-exercise. One study reported no significant changes in theta activity (Büchel et al., Citation2021).
The overall quality of studies comparing pre- and post-exercise conditions was rated as moderate (M = 2.01, SD = 0.21; see Supplemental Material 1).
Studies taking during-exercise measures of EEG
Alpha activity
The most common effect found within studies investigating changes in cortical activity during exercise was an increase in alpha activity (see ). More precisely, 13 studies reported an increase in alpha activity across the central and parietal cortices (k = 1; Fumoto et al., Citation2010 [only alpha-2]), left frontal cortex (k = 1; Enders et al., Citation2016b), bilateral frontal cortex (k = 3; e.g. Ludyga et al., Citation2017), bilateral frontal and parietal cortices (k = 3; e.g. Bailey et al., Citation2008), bilateral frontal and temporal cortices (k = 1; Mott et al., Citation1995), insular cortex (k = 1; Hilty et al., Citation2011), bilateral parietal cortex (k = 1; Ludyga et al., Citation2016), parieto-occipital cortex (k = 1; Ciria et al., Citation2019), and in the whole brain (k = 2; Hottenrott et al., Citation2013; Mechau et al., Citation1998 [only alpha-1]). Four studies included effect sizes for alpha activity and these were all large, ranging from d = 1.60 (Hilty et al., Citation2011) to d = 1.97 (Fumoto et al., Citation2010), and from ηp2 = .38 for alpha-1 to ηp2 = .25 for alpha-2 (Mechau et al., Citation1998) to ηp2 = .48 for alpha-1 and ηp2 = .69 for alpha-2, respectively (Bailey et al., Citation2008; see ). Two studies found a decrease in alpha activity across the bilateral frontal and temporal cortices (Kubitz & Mott, Citation1996), and insular cortex (Hilty et al., Citation2011). Ciria et al. (Citation2018) reported no significant alpha changes across the whole brain, and Fumoto et al. (Citation2010) reported no significant changes in alpha-1 across central and parietal cortices.
Beta activity
Ten studies found an increase in beta activity across the left frontal cortex (k = 1; Enders et al., Citation2016b), bilateral frontal cortex (k = 1; Nielsen et al., Citation2001), bilateral frontal and parietal cortices (k = 2; Bailey et al., Citation2008; Ludyga et al., Citation2016), supplementary area and left parietal cortex (k = 1; Enders et al., Citation2016b), bilateral frontal and temporal cortices (k = 1; Kubitz & Mott, Citation1996), bilateral parietal cortex (k = 1; Ludyga et al., Citation2016), centro- and parieto-occipital regions (k = 2; Ciria et al., Citation2018, Citation2019), and in the whole brain (k = 2; Hottenrott et al., Citation2013; Mechau et al., Citation1998). Notably, Nielsen et al. (Citation2001) reported that frontal beta activity increased during exercise but steadily declined from 2 min into the exercise bout. All reported effect sizes for beta activity were large, including d = 1.54 (Hilty et al., Citation2011), ηp2 = .75 (Bailey et al., Citation2008), and ηp2 = .18 (Mechau et al., Citation1998; see ). Two studies reported no significant changes in beta activity (Fumoto et al., Citation2010; Mott et al., Citation1995).
Delta activity
Only three studies examined delta activity (Ciria et al., Citation2018; Citation2019; Mechau et al., Citation1998) and reported an increase across the whole brain. Among these, only Mechau et al. (Citation1998) reported an effect size, which was large (ηp2 = .40).
Theta activity
Three studies reported that theta activity increased across the bilateral frontal and parietal cortices (Bailey et al., Citation2008), and in the whole brain (Hottenrott et al., Citation2013; Mechau et al., Citation1998), while one reported that it decreased across central and parietal cortices (Fumoto et al., Citation2010). All studies observing changes in theta activity that included effect sizes, reported large effect sizes. These ranged between d = 1.83 and d = 1.86 for parietal and central cortices, respectively (Fumoto et al., Citation2010). Studies that reported effect sizes using partial eta squared indicated a range between ηp2 = .43 (Mechau et al., Citation1998) and ηp2 = .72 (Bailey et al., Citation2008; see ). Ciria et al. (Citation2018) found no significant change in theta activity across the whole brain.
Gamma activity
Gwin and Ferris (Citation2012) reported small-but-significant coherence between contralateral motor cortex EEG signals and lower-limb EMG in the gamma bands during exercise.
Study quality
The overall quality of studies taking during-exercise measures of EEG was rated as moderate (M = 2.02; SD = 0.21; see Supplemental Material 1).
Exercise intensity studies
Alpha activity
The most common finding observed among studies investigating the effects of different exercise intensities on cortical oscillations concerned an increase in alpha activity, regardless of intensity (see ). Of the 33 studies examining brain oscillations during high-intensity exercise, 25 reported increased alpha activity across the prefrontal cortex (k = 2; Devilbiss et al., Citation2019; Wollseiffen et al., Citation2016a), bilateral frontal cortex (k = 8; e.g. Kao et al., Citation2021), left frontal cortex (k = 1; Petruzzello & Landers, Citation1994), central cortex (k = 3; e.g. Ludyga et al., Citation2016), parietal cortex (k = 6; e.g. Schneider et al., Citation2009b), bilateral temporal cortex (k = 3; e.g. Schneider et al., Citation2010a), parieto-occipital regions (k = 1; Ciria et al., Citation2019), centro-occipital regions (k = 1; Ciria et al., Citation2018), occipital regions (k = 1; Büchel et al., Citation2021), limbic regions (k = 3; e.g. Guimarães et al., Citation2015), and in the whole brain (k = 6; e.g. Ciria et al., Citation2019). Six studies reported a decrease in alpha activity across the left prefrontal cortex (k = 1; Devilbiss et al., Citation2019), bilateral frontal cortex (k = 3: e.g. Woo et al., Citation2010), posterior cortex (k = 1; Dishman et al., Citation2010), and parieto-occipital regions (k = 1; Schneider et al., Citation2009a ). Two studies reported no change across the bilateral frontal and right central brain regions (Moraes et al., Citation2007), and in the whole brain (Mierau et al., Citation2009). Chaire et al. (Citation2020) reported small effects for alpha during a delayed match-to-sample working memory task (d < 0.01) and a visual-attention task (d = 0.03), and Wollseiffen et al. (Citation2016a) reported medium effects for alpha-1 (ηp2 = .07). All other studies observing the effects of high-intensity exercise on alpha activity that included effect sizes, reported large effects. These ranged between d = 0.81 (Boutcher & Landers, Citation1988) and d = 6.11 (Bixby et al., Citation2001), as well as between ηp2 = .14 for alpha-1 (Schneider et al., Citation2009a) and ηp2 = .22 for alpha-2 (Wollseiffen et al., Citation2016a) and ηp2 = .48 and ηp2 = .69 for alpha-1 and alpha-2, respectively (Bailey et al., Citation2008; see ).
Of the 12 studies examining brain activity during moderate-intensity exercise, six reported an increase in alpha activity across the prefrontal cortex (k = 1; Wollseiffen et al., Citation2016a), bilateral frontal cortex (k = 3; e.g. Nielsen et al., Citation2001), parietal cortex (k = 3; e.g. Fumoto et al., Citation2010), central regions (k = 1; Fumoto et al., Citation2010), temporal cortex (k = 1; Kubitz & Mott, Citation1996), occipital cortex (k = 1; Schneider et al., Citation2009a), and in the whole brain (k = 1; Hottenrott et al., Citation2013). Four studies reported a decrease in alpha activity across the bilateral frontal (k = 3; e.g. Woo et al., Citation2010) and in the whole brain (k = 1; Hottenrott et al., Citation2013), while two studies reported no changes (k = 2; Gutmann et al., Citation2015; Ludyga et al., Citation2017). With the exception of Wollseiffen et al. (Citation2016a) who reported a medium effect for alpha-1 (ηp2 = .07) as well as a large effect for alpha-2 (ηp2 = .22), all studies investigating the effects of moderate-intensity exercise on alpha activity that included effect sizes, reported large effects. These ranged from from d = 1.67 (for alpha-2; Fumoto et al., Citation2010) to d = 6.11 (Bixby et al., Citation2001), as well as between ηp2 = .14 for alpha-1 (Schneider et al., Citation2009a) and ηp2 = .62 (Woo et al., Citation2010; see ).
During low-intensity exercise, five studies reported an increase in alpha activity across the frontal cortex (k = 3; e.g. Hicks et al., Citation2018), parietal cortex (k = 1; Brümmer et al., Citation2011), centro-occipital regions (k = 1; Ciria et al., Citation2018), posterior cortex (k = 1; Dishman et al., Citation2010), and in the whole brain (k = 1; Ciria et al., Citation2018). Another five effects related to a decrease across the frontal cortex (k = 3; e.g. Kao et al., Citation2021), temporal cortices (k = 1; Kubitz & Mott, Citation1996), and the anterior cingulate gyrus (k = 1; Ohmatsu et al., Citation2014). In four instances, there were no reported changes (k = 1; Guimarães et al., Citation2015). Two studies exploring the effects of low-intensity exercise on alpha activity included effect sizes. Dishman et al. (Citation2010) reported a large effect of ηp2 = .36, while Brümmer et al. (Citation2011) also reported large effects across all conditions (treadmill: d = 1.16; bicycle: d = 2.12; arm crank: d = 1.49; isokinetic: d = 1.29; see ).
Beta activity
The second most observed frequency band activity was that of beta. Of the 18 studies examining brain activity during high-intensity exercise, 13 reported an increase in beta frequency across the bilateral frontal cortex (k = 5; e.g. Bailey et al., Citation2008), central cortex (k = 3; e.g. Moraes et al., Citation2007), parietal cortex (k = 3; e.g. Enders et al., Citation2016b), parieto-occipital regions (k = 2; Ciria et al., Citation2018, Citation2019), the limbic regions (k = 3; e.g. Schneider et al., Citation2009b), temporal cortex (k = 1; Schneider et al., Citation2009b), and in the whole brain (k = 4; e.g. Mechau et al., Citation1998). Five studies showed a decrease across the frontal cortex (k = 2; Brümmer et al., Citation2011; Schneider et al., Citation2010a), parietal cortex (k = 2; Brümmer et al., Citation2011; Schneider et al., Citation2009a), temporal cortex (k = 1; Schneider et al., Citation2010a), posterior brain regions (k = 1; Dishman et al., Citation2010), and occipital cortex (k = 1; Schneider et al., Citation2009a). With the exception of Wollseiffen et al. (Citation2016a), who reported a medium effect size for beta-1 (ηp2 = .07) and a small effect for beta-2 (ηp2 = .03), all studies exploring the effects of high-intensity exercise on beta activity reported large effect sizes. These ranged from d = 1.00 to d = 0.92 for beta-1 and beta-2, respectively, to d = 1.92 and d = 2.97 for beta-1 and beta-2, respectively (Moraes et al., Citation2011). Studies reporting partial eta squared indicated a range between ηp2 = .33 and ηp2 = .14 for beta-1 (Mechau et al., Citation1998) and beta-2 (Schneider et al., Citation2009a), respectively, to ηp2 = .76 and ηp2 = .75 for beta-1 and beta-2 respectively (Bailey et al., Citation2008).
Of the six studies examining brain activity during moderate-intensity exercise, five reported an increase in beta frequency across the bilateral frontal (k = 4; e.g. Nielsen et al., Citation2001), temporal cortex (k = 1; Kubitz & Mott, Citation1996), and in the whole brain (k = 1; Hottenrott et al., Citation2013). One study found a decrease in beta activity in the whole-brain (Hottenrott et al., Citation2013). Only one study (Wollseiffen et al., Citation2016a) that investigated the effects of moderate-intensity exercise on beta activity reported effect sizes; these were medium for beta-1 (ηp2 = .07) and small for beta-2 (ηp2 = .03). With reference to low-intensity exercise, only two studies observed an increase in beta activity across the frontal and parietal cortices (Brümmer et al., Citation2011), and in the whole-brain (Ciria et al., Citation2018). Only Brümmer et al. (Citation2011) reported effect sizes, which were large for all activity conditions (treadmill: d = 1.16; bicycle: d = 2.12; arm crank: d = 1.49; isokinetic: d = 1.29). Three studies reported no changes (e.g. Ohmatsu et al., Citation2014).
Delta activity
With reference to high-intensity exercise, five studies reported an increase in delta activity across the left prefrontal cortex (k = 1; Devilbiss et al., Citation2019), frontal cortex (k = 2; Schneider et al., Citation2010a, Citation2010b), the centro-occipital regions (k = 1; Ciria et al., Citation2018), limbic regions (k = 1; Schneider et al., Citation2010b), temporal cortex (k = 1; Schneider et al., Citation2010a), and in the whole brain (k = 2; Ciria et al., Citation2018; Mechau et al., Citation1998). Of the two studies exploring effects of high-intensity exercise on delta activity that reported effect sizes, both found large effect sizes. Schneider et al. (Citation2010a) reported d = 1.83 for the pre- vs. immediately post-exercise comparison, and d = 1.77 for the pre- vs. post-15-min exercise comparison, while Mechau et al. (Citation1998) reported ηp2 = .40 (see ). Moderate-intensity exercise was found to engender an increase in delta activity in one instance across the bilateral frontal region of the brain (Woo et al., Citation2009), and a decrease in another instance (Spring et al., Citation2017). Both studies reported large effect sizes for delta (ηp2 = .24; Spring et al., Citation2017; ηp2 = .41; Woo et al., Citation2009). Low-intensity exercise did not appear to have any meaningful effect on delta activity (Ohmatsu et al., Citation2014).
Gamma activity
Three effects pertaining to high-intensity exercise on gamma activity were observed. One study reported an increase across the frontal and parietal brain regions (Enders et al., Citation2016b), while the other two reported a decrease across the left prefrontal cortex (Devilbiss et al., Citation2019), and the left middle frontal gyrus and temporal cortex (Schneider et al., Citation2010a). Notably, gamma activity was not investigated in studies using moderate or low-intensity exercise. Of the studies that did investigate changes in gamma activity, only one reported effect sizes (Schneider et al., Citation2010a), and these were large for both the pre- vs. immediately post-exercise comparison (d = 0.97) and the pre- vs. post-15-min exercise comparison (d = 1.67; see ).
Theta activity
Theta activity was observed to increase with high-intensity exercise in seven studies across the frontal cortex (k = 3; e.g. Bailey et al., Citation2008), central regions (k = 1; Bailey et al., Citation2008), temporal cortex (k = 1; Schneider et al., Citation2010a), centro-occipital cortex (k = 2; Ciria et al., Citation2018, Citation2019), and in the whole brain (k = 3; Ciria et al., Citation2018; Citation2019; Mechau et al., Citation1998). A decrease was observed in two instances, across the left prefontal cortex (Devilbiss et al., Citation2019) and posterior brain region (Dishman et al., Citation2010). Two studies observed no changes in theta activity following high-intensity exercise. One of these took measures across the frontal area (Chaire et al., Citation2020), and another from the whole brain (Büchel et al., Citation2021). Studies that explored the effects of high-intensity exercise on theta activity and reported effect sizes, showed large effects in all instances. Moraes et al. (Citation2011) reported effect sizes of d = 1.66 and d = 2.47 for young and elderly groups, respectively, while Bailey et al. (Citation2008) reported an effect size of ηp2 = .72. Mechau et al. (Citation1998) reported an effect of ηp2 = .44 (see ).
Effects on theta activity pertaining to moderate-intensity exercise included a bilateral frontal cortex increase (Woo et al., Citation2009), a whole-brain decrease (Hottenrott et al., Citation2013) and, in one instance, no changes (Chaire et al., Citation2020). Only Woo et al. (Citation2009) reported an effect size, and this was large (ηp2 = .44). One study reported an increase in theta activity, due to low-intensity exercise, across the centro-occipital region and in the whole brain (Ciria et al., Citation2018), while the remaining studies observed no such changes (Chaire et al., Citation2020; Ohmatsu et al., Citation2014).
Study quality
The overall quality of studies exploring exercise intensity was rated as moderate (M = 2.01, SD = 0.20; see Supplemental Material 1).
Exercise vs. no exercise studies
Alpha activity
Among the six studies investigating changes in cortical activity for exercise vs. no-exercise group (see ), two found an increase in alpha activity for the exercise group in relation to the frontal cortex (Kao et al., Citation2020) and posterior regions (Dishman et al., Citation2010). Kubitz and Landers (Citation1993) reported lower alpha activity in the exercise group for the bilateral frontal cortex, as well as medium effect sizes for left alpha (d = 0.45) and small effects for right alpha (d = 0.18). Three studies did not find significant differences for exercise vs. no-exercise groups across the frontal and temporal cortices (Kubitz & Landers, Citation1993), and posterior and occipital cortices (Kao et al., Citation2020).
Beta activity
Two studies reported greater beta activity in the bilateral frontal cortex (Kubitz & Mott, Citation1996) and posterior regions of the brain (beta-2; Dishman et al., Citation2010) for the exercise group. One other study reported no significant differences across the bilateral frontal cortex (Kubitz & Landers, Citation1993), along with a medium effect size for left beta (d = 0.46) and a small effect for right beta (d = 0.02) in the exercise group, in comparison to small effect sizes for left beta (d = 0.16) and right beta (d < 0.01) in the non-exercise group. Notably, a further study did not report the brain region of interest (Severtsen & Bruya, Citation1986).
Theta activity
Dishman et al. (Citation2010) reported greater theta activity for the exercise group in relation to the posterior regions of the brain, while Kao et al. (Citation2020) found no significant differences.
Study quality
The overall quality of studies that compared exercise with no exercise was rated as moderate (M = 2.05, SD = 0.21; see Supplemental Material 1).
Discussion
This systematic review was conducted with the aim of investigating the effects of exercise on EEG-recorded neural oscillatory activity. After the screening and selection process, 47 studies met the inclusion criteria for the synthesis that ensued. The results showed that, in general, studies were conducted among young and healthy adults (Mage = 27.3), and employed an acute exercise intervention. It also emerged that studies were generally small in scope, as the average number of participants was only 24 (see ). The most commonly used EEG analysis methodology was frequency analysis (n = 35), followed by (s)LORETA (n = 8).
Changes in alpha frequency
Thirty-five studies (pre- vs. post-exercise = 24; pre-/post- vs. during-exercise = 11) found exercise to cause an increase in alpha-band activity, while four reported a decrease (see and ). The alpha activity pertaining to the effect of exercise was located mainly across the frontal areas (k = 18; e.g. Enders et al., Citation2016b; Gutmann et al., Citation2018a), with left lateralisation (k = 3; e.g. Petruzzello & Landers, Citation1994), and central regions of the brain (k = 12; e.g. Bailey et al., Citation2008; Fumoto et al., Citation2010). Over the last two decades, experimental evidence has suggested that physical exercise improves neuropsychological performance outcomes that include memory and executive functioning (Blomstrand & Engvall, Citation2021; Smith et al., Citation2010). Thus, the notable frontal alpha activity found in the present review might be reflective of the execution of cognitive functions (e.g. pace judgement, attentional focus, and recovery strategies) during and following exercise. It should be noted that such functions can also be enhanced by alpha activity (Escolano et al., Citation2014).
Enhanced alpha activity has been associated with a decrease in neuro-connectivity from somatosensory afferents (Gundlach et al., Citation2020). More specifically, alpha waves play a central role in regulating the timing of information processing within the cerebral cortex (see Klimesch et al., Citation2007). This can partially explain the increase in alpha activity observed in this review across the central sites of the brain, as these sites overlay regions of the sensorimotor cortex that receive afferent feedback during exercise (Crabbe & Dishman, Citation2004). Another possible reason for the increase in alpha activity concerns the cortical inhibition caused by brainstem and subcortical activation (Koriath et al., Citation1987; Lacey & Lacey, Citation1978). This might have a direct bearing on cardiovascular regulation during exercise. Nonetheless, only two studies reported enhanced alpha activity both during and post-exercise across the limbic lobe (Guimarães et al., Citation2015; Hilty et al., Citation2011).
Although an overall increase in alpha activity was observed in the selected studies, most focused on just one or two brain regions, meaning that oscillatory alpha activity in other regions would have been missed. Some studies (e.g. Hottenrott et al., Citation2013; Mierau et al., Citation2009) did not identify the cerebral regions of interest and examined the electrical activity of the brain as a whole. Such a generalised approach can lead to inconsistent results, given that detailed analysis of individual brain regions is not conducted. Nonetheless, the fact that most studies reported an increase in alpha activity may relate to the notion that such activity is present during processes that are germane to exercise, such as attention (Saalmann et al., Citation2012) and the delay of afferent feedback (Klimesch et al., Citation2007).
Among the seven studies reporting a decrease in alpha activity, two reported a decrease only in the alpha-2 range (Ohmatsu et al., Citation2014; Schneider et al., Citation2010a). Notably, five of these studies did not differentiate between alpha-1 and alpha-2 ranges (e.g. Hilty et al., Citation2011; Woo et al., Citation2010). Thus, it is possible that only alpha-2 frequency decreases during and after exercise, highlighting the importance associated with differentiating between alpha-1 and alpha-2 ranges. A decrease in alpha-2 would be consistent with the transient hypo-frontality hypothesis (Dietrich, Citation2006) and the dual-mode theory of exercise-related affect (Ekkekakis et al., Citation2005). These theoretical frameworks propose that acute exercise leads to a redistribution of brain activity – towards those areas responsible for preparing and performing motor commands (e.g. motor and sensory cortices) – due to limited cortical resources.
Changes in beta frequency
Oscillations in the beta frequency band were, primarily, found to increase due to exercise, as reported in 20 studies (pre- vs. post-exercise = 10; pre-/post- vs. during-exercise = 10). Only four studies reported a decrease (see and ). This activity was mainly identified across the central and parietal brain regions (k = 10; e.g. Brümmer et al., Citation2011), and limbic lobe (k = 3; e.g. Guimarães et al., Citation2015). Beta waves across the sensorimotor areas are usually associated with movement planning and production (Pogosyan et al., Citation2009). More specifically, the peripheral sensory sampling hypothesis posits that beta activity moderates the operation of sensory feedback loops to update the motor commands (Chung et al., Citation2017). In the present review, four studies reported a decrease in beta frequency pre- vs. post-exercise, while none that compared pre-/post- vs. during-exercise reported such a reduction. This finding supports the proposition that enhanced beta activity is associated with the production of movement per se and occurs only during task execution (Jenkinson & Brown, Citation2011).
EEG data have shown that emotional processing increases beta activity in the limbic structures (Cannon et al., Citation2005). Specifically, unpleasant audio-visual stimuli have been found to induce cerebral electrical activity in the beta frequency range (Hayashi et al., Citation2009). In the present review, the enhanced beta activity reported during and post-exercise across the limbic lobe might be reflective of affective responses to exercise (Hall et al., Citation2007). This is corroborated by the fact that the three studies reporting increased beta activity in this region employed a high-intensity exercise protocol (e.g. Guimarães et al., Citation2015).
There is much work in affective science to show marked affective decline at exercise intensities that exceed 70% V̇O2max (e.g. Box et al., Citation2020; Ekkekakis et al., Citation2005). A notable limitation of the present suite of studies is that affective responses to exercise were not measured. We would predict that high levels of beta activity would be associated with subjective displeasure during exercise (i.e. low scores for affective valence).
Similar to alpha activity, some studies calculated beta power as a mean across all electrodes, and reported any activity occurred within a range of 12.5–35 Hz as beta activity (e.g. Mierau et al., Citation2009; Schneider et al., Citation2009b). By considering beta activity to be any activity within such a large range, such studies fail to consider differences between beta-1 and beta-2 frequencies that could help explain between-group differences. While beta-1, or low beta waves (12–15 Hz), are mainly associated with focused concentration, beta-2, or mid-range beta waves (15–20 Hz), are linked to increases in energy and performance (Abhang et al., Citation2016). Accordingly, calculating beta power as a mean across all electrodes can lead to inaccurate results.
Inconsistent results for other frequency bands
It was observed in six studies that delta activity increased (pre- vs. post-exercise = 4; pre-/post- vs. during-exercise = 2; e.g. Ciria et al., Citation2019; Schneider et al., Citation2010b) and it decreased in one study pre- to post-exercise (Spring et al., Citation2017; see and ). There was no explanation offered by Spring et al. (Citation2017) for the decrease, which perhaps overlooks a hypothesis offered by Hatfield (Citation1991) that cortical activity reaches a point at which an inhibitory mechanism is activated in the brainstem. This mechanism is believed to then influence the reticular formation in a way that reduces the transmission of somatic afferents to the cortex, thereby reducing cortical activity. Another possible explanation for the post-exercise decreases in delta activity has been advanced by Lacey and Lacey (Citation1978). Specifically, the notion that brain activation decreases during exercise due to neuro-regulatory feedback mechanisms that pertain to communication between the heart and brain.
Schneider et al. (Citation2010a) asserted that the delta activity increases they observed were reflective of changes in cortical activity. The increases could be due to the oscillatory after-effects of running or artefacts stemming from the cardiovascular system. Conversely, Mechau et al. (Citation1998) suggested the increases in delta activity are associated with the predominant involvement of the motor cortex in the performance of running. The present discussion seeks to reflect the variety of reasons put forward in regard to increases in delta activity as a consequence of exercise engagement (e.g. Mechau et al., Citation1998; Schneider et al., Citation2010b).
Theta activity was seen to increase in seven studies (pre- vs. post-exercise = 4; pre-/post- vs. during-exercise = 3; e.g. Bailey et al., Citation2008; Spring et al., Citation2017) and to decrease pre- vs. post-exercise in three (e.g. Devilbiss et al., Citation2019). In addition, five studies reported no significant changes in theta activity (e.g. Ciria et al., Citation2018; Moraes et al., Citation2011; see and ). It is important to note, however, that some studies observed theta activity during the execution of cognitive tasks (e.g. Chaire et al., Citation2020). Cognitive activity may have influenced results, as elevated theta activity has been observed in executive cognitive function tasks, such as those that involve attention and working memory (Arski et al., Citation2021; Sirota et al., Citation2008).
Decreases in theta activity have been suggested to be indicative of impaired cognitive performance immediately after exercise, as well as the absence of relaxation (Devilbiss et al., Citation2019; Lambourne & Tomporowski, Citation2010). This means that contrasting findings may also be a consequence of the difference in timing of EEG recordings (i.e. during, immediately after exercise, or 15–30 min later). Thus, careful attention needs to be given to the methodological heterogeneities evident in the field of exercise science when interpreting the patterns of brain activity observed during exercise-related tasks.
To date, only the review of Gramkow et al. (Citation2020) has synthesised results pertaining to gamma activity in the domain of exercise and physical activity. In so doing, these authors exposed how limited the knowledge base is in regard to this frequency band. From among the 47 studies included in the present review, only five examined gamma activity (see and ). Gwin and Ferris (Citation2012) explored gamma-range coherence and the other three studies reported contrasting results; specifically, an increase in gamma activity during exercise (Devilbiss et al., Citation2019; Enders et al., Citation2016b), and both an increase (Contreras-Jordán et al., Citation2022) and a decrease (Schneider et al., Citation2010a) from pre- to post-exercise.
It might be argued that increases in gamma activity during exercise result from the increased arousal that is germane to exercise performance (Abhang et al., Citation2016; Lambourne & Tomporowski, Citation2010). Contrastingly, post-exercise decreases in gamma activity have been linked to a decrease in cortical arousal (Schneider et al., Citation2010a). However, due to the limited number of studies observing gamma activity, it is not possible to arrive at firm conclusions regarding the effects of exercise on oscillatory gamma frequency-band activity at the present time.
It is noteworthy that the average quality of the studies included in the present systematic review was rated as moderate (see Supplemental Material 1). Given that participants cannot be blinded to the presence or absence of an exercise intervention, the quality rating of studies used is limited (i.e. double-blind or placebo-controlled designs are not used in this literature; e.g. Renjith, Citation2017; Sedlmeier et al., Citation2012). Additionally, with the exception of three studies (Ciria et al., Citation2018; Dishman et al., Citation2010; Hilty et al., Citation2011), the articles included in the present review failed to control for volume conduction, thus limiting the interpretation of EEG-recorded regional effects produced by local neurons (Parr et al., Citation2021). This could provide a meaningful explanation for the inconsistent results found for some frequency bands (i.e. delta, theta, gamma). Moreover, the study quality could have impacted our results, as some of the trends are predicated on weak studies (see Supplemental Material 1).
Exercise intensity
We found that alpha, beta, and delta activity mostly increased as a consequence of high-intensity exercise, when compared to both moderate and low-intensity exercise. Previous research has found that heat stress caused by exhaustive exercise can lead to an increase in cortical activity (Nakata et al., Citation2016; Shibasaki et al., Citation2017), which might explain, in part, the relationship between exercise intensity and alpha, beta, and delta activity. Another possible explanation for the increase observed in beta activity, in particular at the fronto-central regions, is its association with higher levels of psychomotor arousal (Nielsen & Nybo, Citation2003) and increased cortical activation due to voluntary movements (Halliday et al., Citation1998; Moraes et al., Citation2007). An increase in theta activity has also been linked to the processing of novel information (Demiralp et al., Citation2001; Pesonen et al., Citation2007), thus the observed theta findings may relate to the control and regulation of attentional resources (Sauseng et al., Citation2010).
It is difficult to be certain of the exact cause of effects of high-intensity exercise on cortical activity due to the physiological effects that also manifest (e.g. elevated body temperature and blood circulation), which in turn may influence oscillatory activity. However, effects of moderate-intensity exercise are less likely to be affected by such factors, as, for example, body temperature is not elevated to the same degree (Takeda & Okazaki, Citation2018). Results from moderate-intensity exercise showed the most common finding to be an increase in alpha and beta activity across multiple brain regions (e.g. frontal, parietal, occipital, whole brain). This can be attributed to the fact that alpha-band activity is related to wakefulness (Cantero et al., Citation2002), while beta activity is essential to maintaining exercise at a steady state (Plattner et al., Citation2014).
When analysing the effects caused by low-intensity exercise on cortical activity, no meaningful patterns emerged, as the most common effect observed was that of no changes in oscillatory bands, except alpha. The effects of low-intensity exercise on alpha-band activity included increases and decreases as well as no changes. Nonetheless, no effect was seen to stand out, as there was an increase in five studies (e.g. Brümmer et al., Citation2011), a decrease in five studies (e.g. Kao et al., Citation2020), and no changes in five (e.g. Gutmann et al., Citation2018b). Similar to moderate-intensity exercise studies, those investigating low-intensity exercise focused primarily on alpha-band activity, thus limiting discussion in regard to other oscillatory bands.
Findings emanating from high-quality studies
Focusing exclusively on the high-quality studies based on QATQS ratings (i.e. M < 2.00), findings, on the whole, mirror those evident in the studies of moderate quality (i.e. M = 2.00–3.00; see Supplemental Material 1). There are, however, a few notable exceptions, albeit these are of a minor order. First, higher-quality studies tended to focus on the full range of brainwaves as well as differentiating between alpha-1 and alpha-2 (e.g. Büchel et al., Citation2021; Gutmann et al., Citation2018a; Citation2018b). Second, along similar lines, the higher-quality studies generally went beyond examining only frontal brain regions, thus providing a more holistic representation of brainwave activity (e.g. Hilty et al., Citation2011; Hottenrott et al., Citation2013). Third, with the exception of eight studies (e.g. Guimarães et al., Citation2015; Ludyga et al., Citation2016), the higher-quality studies provided sufficient statistical detail to facilitate calculation of effect sizes (e.g. Contreras-Jordán et al., Citation2022; Wollseiffen et al., Citation2016a; Citation2016b). More specifically, while eight out of 20 (40%) of the higher-quality studies did not provide sufficient information for such calculations, almost half of lower-quality studies (13 out of 27; 48.2%) lacked such statistical detail (e.g. Ciria et al., Citation2018; Citation2019).
From among the 20 high-quality studies, the most common effect of exercise on oscillatory activity was an increase in alpha activity from pre- to post-exercise (k = 15; e.g. Büchel et al., Citation2021; Contreras-Jordán et al., Citation2022) and from pre- to during exercise (k = 2; Hottenrott et al., Citation2013; Ludyga et al., Citation2016). Interestingly, this increase was observed across the frontal area of the brain in six studies (e.g. Contreras-Jordán et al., Citation2022; Hicks et al., Citation2018) and the whole brain in six studies (e.g. Gutmann et al., Citation2015; Hilty et al., Citation2011). In the five remaining studies, the increase in alpha frequency was reported across various brain areas without any clear trend (i.e. parietal and occipital cortices [k = 2], posterior cortex [k = 1], limbic lobe [k = 1], and anterior and posterior cingulate gyrus [k = 1]). It is noteworthy that only one high-quality study observed a decrease in the frontal brain region (Kubitz & Mott, Citation1996), which serves to question the veracity of the analogous decrease reported in six lower-quality studies (e.g. Hilty et al., Citation2011; Schneider et al., Citation2010a). With respect to other frequency bands, findings from high-quality studies were equivocal in nature; similar to those with a QAQTS mean rating of ≥ 2.0.
Considerations for use of EEG in exercise science
The inconsistent findings found in the present review for most of the EEG frequency ranges can also be attributed to the use of a variety of EEG devices. Given that the accuracy of EEG recordings increases in accord with the number of electrodes used (Lau et al., Citation2012), differences in this number can explain some of the differences reported in the present systematic review. While Engchuan et al. (Citation2019) used a commercially available lightweight EEG device that required the application of only one electrode, Schneider et al. (Citation2010a) used a 64-channel portable EEG device. Thus, researchers need to consider the EEG device that they use and the number of electrodes most suitable in testing their research hypotheses.
When selecting the most suitable technique for the measurement of brain activity, aspects such as temporal and spatial resolution must be considered (Liu et al., Citation2006). EEG may be a suitable tool in identifying cognitive processes that arise over brief time periods, such as observation and shifts in attention (e.g. Luck et al., Citation2000; Vecchiato et al., Citation2010). It is not, however, considered the most suitable technique for detecting activity in the deeper areas of the brain, such as the thalamus or the posterior cingulate cortex (Bigliassi et al., Citation2021). Instead, EEG is recommended for signals that originate from more peripheral areas of the brain such as the prefrontal cortex (Darvas et al., Citation2004; Dickter & Kieffaber, Citation2013).
EEG is considered to provide excellent temporal resolution but its spatial resolution is limited (Britton et al., Citation2016). This pertains to a mathematical issue known as the inverse problem, which entails the prediction of exact brain activity locations through surface electrodes placed on the scalp (Grech et al., Citation2008; Purves et al., Citation2008). Even if this limitation can be countered by the use of source reconstruction analysis that estimates the brain activation sources (see Luck, Citation2014), researchers might prefer brain-imaging methods that offer higher spatial resolution (e.g. functional near-infrared spectroscopy; Leff et al., Citation2011).
It is expected that different forms of physical exercise and different intensities will lead to different outcomes in terms of electrical activity in the brain (Gramkow et al., Citation2020). This is because each exercise type involves specific variances related to sensorimotor execution, cognition, and functional neural networks. These variances serve to activate different parts of the brain and different oscillatory bands. For instance, Brümmer et al. (Citation2011) found that exercise leads to a significant increase in alpha activity. While this change occurred under four exercise modes (i.e. treadmill running, indoor cycling, arm crank, and isokinetic exercises) during moderate-intensity exercise (50% maximum capacity), a significant increase in alpha activity was only observed in the isokinetic exercise protocol when participants worked at a high-intensity (80% maximum capacity). Furthermore, a significant increase in beta activity was only observed during the bicycle exercise when the intensity was moderate, and beta activity was seen to significantly decrease after high-intensity treadmill running (Brümmer et al., Citation2011). Notably, it has been suggested that the most significant alterations in cortical activity occur when the individual can self-select their exercise intensity, rather than use heart-rate limits to determine intensity (Schneider et al., Citation2009b). Future research should, therefore, take into consideration the variability that different exercise modes and intensities can elicit in brain activity; this will avoid the erroneous conglomeration of different outcomes.
Physically active young adults have shown higher activity of neural oscillations at 22–34 Hz when compared to physically inactive controls, thus implying that higher aerobic fitness levels are associated with enhanced top-down cognitive control regulation (Kamijo et al., Citation2010; Kamijo & Takeda, Citation2010). Additionally, participants with higher levels of aerobic fitness have been found to achieve faster reaction times and superior variation of oscillatory theta and beta power during a cognitive task. These findings further support the notion that superior aerobic fitness levels are associated with greater attentional control in regard to visuo-spatial processing, shown through greater motor preparation and upregulation of attentional processing (Wang et al., Citation2015). Thus, participants’ fitness level should be reported in future studies to facilitate the interpretation of findings.
Strengths and limitations
The present review is subject to a number of limitations and has served to illustrate some of the weaknesses in this body of literature. Firstly, the average number of participants in the studies included in this review was 22. Such a low sample size can detrimentally affect statistical analyses and interpretation of results; in particular, due to false-positives and over-estimation of the magnitude of an association (Hackshaw, Citation2008). Secondly, given that most studies conducting multiple tests failed to adjust for multiple comparisons (i.e. through Bonferroni adjustment), there is a risk of erroneous inferences, which calls into question the reliability of the findings presented in this review (Chen et al., Citation2017). Future research should, therefore, aim to design studies that are adequately powered and that make suitable adjustment for multiple comparisons.
This review contains a qualitative synthesis of results, given that we assumed that studies would exhibit large heterogeneity, based on previous reviews on the subject (Crabbe & Dishman, Citation2004; Gramkow et al., Citation2020). Although it could be argued that a quantitative synthesis might provide more exacting results, this would necessitate the exclusion of many studies that are incompatible with this approach. By extension, important data would be excluded and the generalisability of findings would be limited. Also, performing a meta-analysis when the differences among studies are so stark, as was the case in the present review, would mean that results are combined in a somewhat meaningless manner, as suggested by Gopalakrishnan and Ganeshkumar (Citation2013).
This review offers a quantitative synthesis to some extent, through the analysis of effect sizes that are displayed in . Although not all studies provided the detail necessary to facilitate calculation of effect sizes, the review showed that most studies observed oscillatory changes of practical significance, as illustrated through the majority of effect sizes being large (cf. Bhandari, Citation2021). Future researchers might consider a more complex approach that employs both quantitative and qualitative analytical methods in order to optimise reliability and generalisability (Harden, Citation2010). There is also a need to consider the relevance of items pertaining to blinding (e.g. item 4 in the QATQS) for experiments wherein blinding of conditions is very difficult or impossible.
This review aimed to include all studies that took EEG measurements before, during, and after exercise-related interventions. Previous reviews on the subject of exercise and brain activity have had other foci, such as the effects of exercise on mood states (Lattari et al., Citation2014), the use of the event-related potentials technique (Rahman et al., Citation2019), and the use of studies that could be evaluated by meta-analytic methods (Crabbe & Dishman, Citation2004). The review closest in aim to this systematic review is that of Gramkow et al. (Citation2020), yet they did not calculate effect sizes given that they adopted a qualitative approach. Moreover, they grouped healthy and clinical populations in part of their analysis. This makes it challenging to distinguish changes in cerebral electrical activity brought by facets of exercise and those that might be accounted for by psychological or physical pathologies.
It is important to note that although we have synthesised effects according to four main categories, there were various limitations to the categorisation of these studies due to the variability among them. For example, although some studies used biological parameters such as V̇O2max to measure exercise intensity, others used age predicted HRmax, which has been shown to have low accuracy (Shookster et al., Citation2020). Also, some studies used volitional exhaustion but failed to account for the physiological limits/fitness level of individuals, which can result in significant variance in terms of the actual intensity of exercise performed (Edvardsen et al., Citation2014). Future studies might assess relevant aspects of fitness (aerobic capacity) and report them.
Many of the studies explored in the present review failed to account for gender-dependent factors that can perpetuate differences in neural activity that would, ultimately, limit the accuracy and generalisability of findings (Cantillo-Negrete et al., Citation2016). For instance, Sheel et al. (Citation2016) found that, even when matched for lung size, women’s conducting airways are smaller than men’s, which could lead to a significant difference in ventilatory resistance and an associated difference in neural responses (Guyenet & Bayliss, Citation2015). Moreover, age-related differences such as reduced muscle mass and strength in older participants were not considered by many studies that included a broad age range (Suryadinata et al., Citation2020). It is also important to note that, while exercise took place indoors for most studies, three studies examined exercise in outdoor settings, which could have had a bearing on cardiovascular and metabolic parameters that, in turn, led to differences in oscillatory activity (Li et al., Citation2011). Accordingly, there are several factors that future studies should take into consideration.
The present conclusions do not concur with those reached by Crabbe and Dishman (Citation2004), who found an overall increase of activity in all frequency bands due to exercise. When analysing and considering all available data, the outcomes of the present review bear some similarity to those reported by Gramkow et al. (Citation2020). These authors also found a tendency for increases in alpha-band activity. Differences between the two studies may have resulted from differences in the process of study selection, as well as the inclusion of multiple studies that have been published over the intervening 2 years or were not included by Gramkow et al. (k = 6).
Conclusions
Research on the effects of exercise on cortical oscillatory activity recorded by EEG is growing rapidly. Today, EEG can be applied in settings wherein participants perform whole-body movements. This has greatly increased the number of EEG studies that explore brain activity in the field of exercise science. This systematic review was conducted with the aim to enhance knowledge regarding the effects of exercise on EEG-recorded cortical oscillatory activity. Following a review of 47 studies, results showed mostly inconsistent outcomes – even in the 20 high-quality studies – except for an increase in alpha and beta frequency-band activity due to exercise. Albeit this finding is supported by previous reviews, the qualitative synthesis showed that many of the included studies (27) were of moderate quality, therefore somewhat limiting the conclusions that can be drawn. In order to increase reliability and generalisability, future research should not only consider more robust designs, but also the recruitment of larger samples.
Disclosure statement
No potential conflict of interest was reported by the author(s).
Notes
1 One component was removed from Parr et al.’s (Citation2021) original 12 components, namely ‘secondary measures of verbal and conscious processing’ (p. 8), as this was not relevant to the present review.
2 This is a slight modification of Parr et al.’s (Citation2021) ‘alpha definition’ (p. 7) given that all EEG frequency bands are of interest in the present review.
3 The review included one study comparing healthy adults with hand cyclists (Brümmer et al., Citation2011), but otherwise excluded studies in which participants had medical conditions, diseases or disorders.
References
- References marked with an asterisk (*) indicate studies that are included in the systematic review.
- Abhang, P. A., Gawali, B. W., & Mehrotra, S. C. (2016). Introduction to EEG-and speech-based emotion recognition. Academic Press.
- American College of Sports Medicine. (2013). ACSM’s guidelines for exercise testing and prescription (11th ed.). Lippincott Williams & Wilkins.
- Arski, O. N., Young, J. M., Smith, M. L., & Ibrahim, G. M. (2021). The oscillatory basis of working memory function and dysfunction in epilepsy. Frontiers in Human Neuroscience, 14, Article 596. https://doi.org/https://doi.org/10.3389/fnhum.2020.612024
- Babiloni, C., Babiloni, F., Carducci, F., Cincotti, F., Cocozza, G., Del Percio, C., Moretti, D. V., & Rossini, P. M. (2002). Human cortical electroencephalography (EEG) rhythms during the observation of simple aimless movements: A high-resolution EEG study. NeuroImage, 17(2), 559–572. https://doi.org/https://doi.org/10.1006/nimg.2002.1192
- *Bailey, S. P., Hall, E. E., Folger, S. E., & Miller, P. C. (2008). Changes in EEG during graded exercise on a recumbent cycle ergometer. Journal of Sports Science & Medicine, 7(4), 505–511. https://www.ncbi.nlm.nih.gov/pmc/articles/PMC3761919/
- Bevilacqua, D., Davidesco, I., Wan, L., Chaloner, K., Rowland, J., Ding, M., Poeppel, D., & Dikker, S. (2019). Brain-to-brain synchrony and learning outcomes vary by student–teacher dynamics: Evidence from a real-world classroom electroencephalography study. Journal of Cognitive Neuroscience, 31(3), 401–411. https://doi.org/https://doi.org/10.1162/jocn_a_01274
- Bhandari, P. (2021, February 18). Effect size in statistics. Scribbr. https://www.scribbr.com/statistics/effect-size/
- Bigliassi, M., Karageorghis, C. I., Hoy, G. K., & Layne, G. S. (2019). The way you make Me feel: Psychological and cerebral responses to music during real-life physical activity. Psychology of Sport and Exercise, 41, 211–217. https://doi.org/https://doi.org/10.1016/j.psychsport.2018.01.010
- Bigliassi, M., Wright, M. J., Karageorghis, C. I., & Nowicky, A. V. (2021). Measuring electrical activity in the brain during exercise: A review of methods, challenges, and opportunities. Sport and Exercise Psychology Review, 16(1), 4–19. http://bura.brunel.ac.uk/handle/2438/22300
- *Bixby, W. R., Spalding, T. W., & Hatfield, B. D. (2001). Temporal dynamics and dimensional specificity of the affective response to exercise of varying intensity: Differing pathways to a common outcome. Journal of Sport & Exercise Psychology, 23(3), 171–190. https://doi.org/https://doi.org/10.1123/jsep.23.3.171
- Bland, B. H., & Oddie, S. D. (2001). Theta band oscillation and synchrony in the hippocampal formation and associated structures: The case for its role in sensorimotor integration. Behavioural Brain Research, 127(1–2), 119–136. https://doi.org/https://doi.org/10.1016/S0166-4328(01)00358-8
- Blomstrand, P., & Engvall, J. (2021). Effects of a single exercise workout on memory and learning functions in young adults—A systematic review. Translational Sports Medicine, 4(1), 115–127. https://doi.org/https://doi.org/10.1002/tsm2.190
- *Boutcher, S. H., & Landers, D. M. (1988). The effects of vigorous exercise on anxiety, heart rate, and alpha activity of runners and nonrunners. Psychophysiology, 25(6), 696–702. https://doi.org/https://doi.org/10.1111/j.1469-8986.1988.tb01911.x
- Box, A. G., Feito, Y., Zenko, Z., & Petruzzello, S. J. (2020). The affective interval: An investigation of the peaks and valleys during high-and moderate-intensity interval exercise in regular exercisers. Psychology of Sport and Exercise, 49, Article 101686. https://doi.org/https://doi.org/10.1016/j.psychsport.2020.101686
- Britton, J. W., Frey, L. C., Hopp, J. L., Korb, P., Koubeissi, M. Z., Lievens, W. E., Pestana-Knight, E. M., & St. Louis, E. K. (2016). Electroencephalography (EEG): An introductory text and atlas of normal and abnormal findings in adults, children, and infants. American Epilepsy Society.
- *Brümmer, V., Schneider, S., Abel, T., Vogt, T., & Strüder, H. K. (2011). Brain cortical activity is influenced by exercise mode and intensity. Medicine & Science in Sports & Exercise, 43(10), 1863–1872. https://doi.org/https://doi.org/10.1249/MSS.0b013e3182172a6f
- *Büchel, D., Sandbakk, Ø, & Baumeister, J. (2021). Exploring intensity-dependent modulations in EEG resting-state network efficiency induced by exercise. European Journal of Applied Physiology, 121(9), 2423–2435. https://doi.org/https://doi.org/10.1007/s00421-021-04712-6
- Buckley, J., Cohen, J. D., Kramer, A. F., McAuley, E., & Mullen, S. P. (2014). Cognitive control in the self-regulation of physical activity and sedentary behavior. Frontiers in Human Neuroscience, 8, Article 747. https://doi.org/https://doi.org/10.3389/fnhum.2014.00747
- Buzsáki, G., & Moser, E. I. (2013). Memory, navigation and theta rhythm in the hippocampal-entorhinal system. Nature Neuroscience, 16(2), 130–138. https://doi.org/https://doi.org/10.1038/nn.3304
- Cannon, R., Lubar, J., Thornton, K., Wilson, S., & Congedo, M. (2005). Limbic beta activation and LORETA: Can hippocampal and related limbic activity be recorded and changes visualized using LORETA in an affective memory condition? Journal of Neurotherapy, 8(4), 5–24. https://www.tandfonline.com/doi/abs/10.1300/J184v08n04_02
- Cantero, J. L., Atienza, M., & Salas, R. M. (2002). Human alpha oscillations in wakefulness, drowsiness period, and REM sleep: Different electroencephalographic phenomena within the alpha band. Neurophysiologie Clinique/Clinical Neurophysiology, 32(1), 54–71. https://doi.org/https://doi.org/10.1016/S0987-7053(01)00289-1
- Cantillo-Negrete, J., Carino-Escobar, R. I., Carrillo-Mora, P., Flores-Rodríguez, T. B., Elias-Vinas, D., & Gutierrez-Martinez, J. (2016). Gender differences in quantitative electroencephalogram during a simple hand movement task in young adults. Revista de Investigación Clinica, 68(5), 245–255. https://www.medigraphic.com/pdfs/revinvcli/nn-2016/nn165d.pdf
- Castermans, T., Duvinage, M., Cheron, G., & Dutoit, T. (2014). About the cortical origin of the low-delta and high-gamma rhythms observed in EEG signals during treadmill walking. Neuroscience Letters, 561, 16–70. https://doi.org/https://doi.org/10.1016/j.neulet.2013.12.059
- *Chaire, A., Becke, A., & Düzel, E. (2020). Effects of physical exercise on working memory and attention-related neural oscillations. Frontiers in Neuroscience, 14, Article 239. https://doi.org/https://doi.org/10.3389/fnins.2020.00239
- Chen, S. Y., Feng, Z., & Yi, X. (2017). A general introduction to adjustment for multiple comparisons. Journal of Thoracic Disease, 9(6), 1725–1729. https://doi.org/https://doi.org/10.21037/jtd.2017.05.34
- Cheval, B., Tipura, E., Burra, N., Frossard, J., Chanal, J., Orsholits, D., Radel, R., & Boisgontier, M. P. (2018). Avoiding sedentary behaviors requires more cortical resources than avoiding physical activity: An EEG study. Neuropsychologia, 119, 68–80. https://doi.org/https://doi.org/10.1016/j.neuropsychologia.2018.07.029
- Chung, J. W., Ofori, E., Misra, G., Hess, C. W., & Vaillancourt, D. E. (2017). Beta-band activity and connectivity in sensorimotor and parietal cortex are important for accurate motor performance. NeuroImage, 144, 164–173. https://doi.org/https://doi.org/10.1016/j.neuroimage.2016.10.008
- *Ciria, L. F., Luque-Casado, A., Sanabria, D., Holgado, D., Ivanov, P. C., & Perakakis, P. (2019). Oscillatory brain activity during acute exercise: Tonic and transient neural response to an oddball task. Psychophysiology, 56(5), Article 13326. https://doi.org/https://doi.org/10.1111/psyp.13326
- *Ciria, L. F., Perakakis, P., Luque-Casado, A., & Sanabria, D. (2018). Physical exercise increases overall brain oscillatory activity but does not influence inhibitory control in young adults. NeuroImage, 181(1), 203–210. https://doi.org/https://doi.org/10.1016/j.neuroimage.2018.07.009
- Cohen, J. (1988). Statistical power analysis for the behavioral sciences. Routledge.
- *Contreras-Jordán, O. R., Sánchez-Reolid, R., Infantes-Paniagua, Á, Fernández-Caballero, A., & González-Fernández, F. T. (2022). Physical exercise effects on university students’ attention: An EEG analysis approach. Electronics, 11(5), Article 770. https://doi.org/https://doi.org/10.3390/electronics11050770
- Cooke, A., Kavussanu, M., Gallicchio, G., Willoughby, A., McIntyre, D., & Ring, C. (2014). Preparation for action: Psychophysiological activity preceding a motor skill as a function of expertise, performance outcome, and psychological pressure. Psychophysiology, 51(4), 374–384. https://doi.org/https://doi.org/10.1111/psyp.12414
- Crabbe, J. B., & Dishman, R. K. (2004). Brain electrocortical activity during and after exercise: A quantitative synthesis. Psychophysiology, 41(4), 563–574. https://doi.org/https://doi.org/10.1111/j.1469-8986.2004.00176.x
- Dal Maso, F., Desormeau, B., Boudrias, M. H., & Roig, M. (2018). Acute cardiovascular exercise promotes functional changes in cortico-motor networks during the early stages of motor memory consolidation. NeuroImage, 174(1), 380–392. https://doi.org/https://doi.org/10.1016/j.neuroimage.2018.03.029
- Darvas, F., Pantazis, D., Kucukaltun-Yildirim, E., & Leahy, R. M. (2004). Mapping human brain function with MEG and EEG: Methods and validation. NeuroImage, 23, S289–S299. https://doi.org/https://doi.org/10.1016/j.neuroimage.2004.07.014
- De, A., & Mondal, S. (2020). Yoga and brain wave coherence: A systematic review for brain function improvement. Heart and Mind, 4(2), 33–39. https://doi.org/https://doi.org/10.4103/hm.hm_78_19
- Demiralp, T., Ademoglu, A., Istefanopulos, Y., Başar-Eroglu, C., & Başar, E. (2001). Wavelet analysis of oddball P300. International Journal of Psychophysiology, 39(2–3), 221–227. https://doi.org/https://doi.org/10.1016/S0167-8760(00)00143-4
- Desai, R., Tailor, A., & Bhatt, T. (2015). Effects of yoga on brain waves and structural activation: A review. Complementary Therapies in Clinical Practice, 21(2), 112–118. https://doi.org/https://doi.org/10.1016/j.ctcp.2015.02.002
- *Devilbiss, D. M., Etnoyer-Slaski, J. L., Dunn, E., Dussourd, C. R., Kothare, M. V., Martino, S. J., & Simon, A. J. (2019). Effects of exercise on EEG activity and standard tools used to assess concussion. Journal of Healthcare Engineering, 2019, Article 4794637. https://doi.org/https://doi.org/10.1155/2019/4794637
- Dickter, C. L., & Kieffaber, P. D. (2013). EEG methods for the psychological sciences. Sage Publications.
- Dietrich, A. (2006). Transient hypofrontality as a mechanism for the psychological effects of exercise. Psychiatry Research, 145(1), 79–83. https://doi.org/https://doi.org/10.1016/j.psychres.2005.07.033
- Di Flumeri, G., Aricò, P., Borghini, G., Sciaraffa, N., Di Florio, A., & Babiloni, F. (2019). The dry revolution: Evaluation of three different EEG dry electrode types in terms of signal spectral features, mental states classification and usability. Sensors, 19(6), Article 1365. https://doi.org/https://doi.org/10.3390/s19061365
- *Dishman, R. K., Thom, N. J., Puetz, T. W., O’Connor, P. J., & Clementz, B. A. (2010). Effects of cycling exercise on vigor, fatigue, and electroencephalographic activity among young adults who report persistent fatigue. Psychophysiology, 47(6), 1066–1074. https://doi.org/https://doi.org/10.1111/j.1469-8986.2010.01014.x
- Edvardsen, E., Hem, E., & Anderssen, S. A. (2014). End criteria for reaching maximal oxygen uptake must be strict and adjusted to sex and age: A cross-sectional study. PloS One, 9(1), e85276. https://doi.org/https://doi.org/10.1371/journal.pone.0085276
- Ekkekakis, P. (2009). The dual-mode theory of affective responses to exercise in metatheoretical context: II. Bodiless heads, ethereal cognitive schemata, and other improbable dualistic creatures, exercising. International Review of Sport and Exercise Psychology, 2(2), 139–160. https://doi.org/https://doi.org/10.1080/17509840902829323
- Ekkekakis, P., Hall, E. E., & Petruzzello, S. J. (2005). Variation and homogeneity in affective responses to physical activity of varying intensities: An alternative perspective on dose–response based on evolutionary considerations. Journal of Sports Sciences, 23(5), 477–500. https://doi.org/https://doi.org/10.1080/02640410400021492
- *Enders, H., Cortese, F., Maurer, C., Baltich, J., Protzner, A. B., & Nigg, B. M. (2016b). Changes in cortical activity measured with EEG during a high-intensity cycling exercise. Journal of Neurophysiology, 115(1), 379–388. https://doi.org/https://doi.org/10.1152/jn.00497.2015
- Enders, H., & Nigg, B. M. (2016a). Measuring human locomotor control using EMG and EEG: Current knowledge, limitations and future considerations. European Journal of Sport Science, 16(1), 416–426. https://doi.org/https://doi.org/10.1080/17461391.2015.1068869
- Engchuan, P., Wongsuphasawat, K., & Sittiprapaporn, P. (2019). Brain electrical activity during bench press weight training exercise. Asian Journal of Medical Sciences, 10(5), 80–85. https://doi.org/https://doi.org/10.3126/ajms.v10i5.21034
- Escolano, C., Navarro-Gil, M., Garcia-Campayo, J., Congedo, M., De Ridder, D., & Minguez, J. (2014). A controlled study on the cognitive effect of alpha neurofeedback training in patients with major depressive disorder. Frontiers in Behavioral Neuroscience, 8(296), Article 296. https://doi.org/https://doi.org/10.3389/fnbeh.2014.00296
- Fitzgibbon, S. P., Pope, K. J., Mackenzie, L., Clark, C. R., & Willoughby, J. O. (2004). Cognitive tasks augment gamma EEG power. Clinical Neurophysiology, 115(8), 1802–1809. https://doi.org/https://doi.org/10.1016/j.clinph.2004.03.009
- *Fumoto, M., Oshima, T., Kamiya, K., Kikuchi, H., Seki, Y., Nakatani, Y., Yu, X., Sekiyama, T., Sato-Suzuki, I., & Arita, H. (2010). Ventral prefrontal cortex and serotonergic system activation during pedalling exercise induces negative mood improvement and increased alpha band in EEG. Behavioural Brain Research, 213(1), 1–9. https://doi.org/https://doi.org/10.1016/j.bbr.2010.04.017
- Gallicchio, G., Cooke, A., & Ring, C. (2017). Practice makes efficient: Cortical alpha oscillations are associated with improved golf putting performance. Sport, Exercise, and Performance Psychology, 6(1), 89–102. https://doi.org/http://dx.doi.org/10.1037/spy0000077
- Gopalakrishnan, S., & Ganeshkumar, P. (2013). Systematic reviews and meta-analysis: Understanding the best evidence in primary healthcare. Journal of Family Medicine and Primary Care, 2(1), 9–l4. https://doi.org/https://doi.org/10.4103/2249-4863.109934
- Gramkow, M. H., Hasselbalch, S. G., Waldemar, G., & Frederiksen, K. S. (2020). Resting state EEG in exercise intervention studies: A systematic review of effects and methods. Frontiers in Human Neuroscience, 14, Article 155. https://doi.org/https://doi.org/10.3389/fnhum.2020.00155
- Grech, R., Cassar, T., Muscat, J., Camilleri, K. P., Fabri, S. G., Zervakis, M., Xanthopoulos, P., Sakkalis, V., & Vanrumste, B. (2008). Review on solving the inverse problem in EEG source analysis. Journal of Neuroengineering and Rehabilitation, 5(1), Article 25. https://doi.org/https://doi.org/10.1186/1743-0003-5-25
- *Guimarães, T., Macedo da Costa, B., Silva Cerqueira, L., de Carlo Andrade Serdeiro, A., Augusto Monteiro Saboia Pompeu, F., Sales de Moraes, H., Meireles dos Santos, T., & Camaz Deslandes, A. (2015). Acute effect of different patterns of exercise on mood, anxiety and cortical activity. Archives of Neuroscience, 2(1), Article e18780. https://doi.org/https://doi.org/10.5812/archneurosci.18781
- Gundlach, C., Müller, M. M., Hoff, M., Ragert, P., Nierhaus, T., Villringer, A., & Sehm, B. (2020). Reduction of somatosensory functional connectivity by transcranial alternating current stimulation at endogenous mu-frequency. NeuroImage, 221(1), 117–175. https://doi.org/https://doi.org/10.1016/j.neuroimage.2020.117175
- *Gutmann, B., Huelsduenker, T., Mierau, J., Strüder, H. K., & Mierau, A. (2018a). Exercise-induced changes in EEG alpha power depend on frequency band definition mode. Neuroscience Letters, 662(1), 271–275. https://doi.org/https://doi.org/10.1016/j.neulet.2017.10.033
- *Gutmann, B., Mierau, A., Hülsdünker, T., Hildebrand, C., Przyklenk, A., Hollmann, W., & Strüder, H. K. (2015). Effects of physical exercise on individual resting state EEG alpha peak frequency. Neural Plasticity, 2015, Article 717312. https://doi.org/https://doi.org/10.1155/2015/717312
- *Gutmann, B., Zimmer, P., Hülsdünker, T., Lefebvre, J., Binnebößel, S., Oberste, M., Bloch, W., Strüder, H. K., & Mierau, A. (2018b). The effects of exercise intensity and post-exercise recovery time on cortical activation as revealed by EEG alpha peak frequency. Neuroscience Letters, 668(1), 159–163. https://doi.org/https://doi.org/10.1016/j.neulet.2018.01.007
- Guyenet, P. G., & Bayliss, D. A. (2015). Neural control of breathing and CO2 homeostasis. Neuron, 87(5), 946–961. https://www.cell.com/neuron/fulltext/S0896-6273(15)00676-5?_returnURL = https%3A%2F%2Flinkinghub.elsevier.com%2Fretrieve%2Fpii%2FS0896627315006765%3Fshowall%3Dtrue https://doi.org/https://doi.org/10.1016/j.neuron.2015.08.001
- *Gwin, J. T., & Ferris, D. P. (2012). Beta- and gamma-range human lower limb corticomuscular coherence. Frontiers in Human Neuroscience, 6, Article 258. https://doi.org/https://doi.org/10.3389/fnhum.2012.00258
- Hackshaw, A. (2008). Small studies: Strengths and limitations. European Respiratory Journal, 32(1), 1141–1143. https://doi.org/https://doi.org/10.1183/09031936.00136408
- Hall, E. E., Ekkekakis, P., & Petruzzello, S. J. (2007). Regional brain activity and strenuous exercise: Predicting affective responses using EEG asymmetry. Biological Psychology, 75(2), 194–200. https://doi.org/https://doi.org/10.1016/j.biopsycho.2007.03.002
- Halliday, D. M., Conway, B. A., Farmer, S. F., & Rosenberg, J. R. (1998). Using electroencephalography to study functional coupling between cortical activity and electromyograms during voluntary contractions in humans. Neuroscience Letters, 241(1), 5–8. https://doi.org/https://doi.org/10.1016/S0304-3940(97)00964-6
- Harden, A. (2010). Mixed-methods systematic reviews: Integrating quantitative and qualitative findings (Technical brief No. 25). National Center for the Dissemination of Disability Research. https://ktdrr.org/ktlibrary/articles_pubs/ncddrwork/focus/focus25/Focus25.pdf
- Hatfield, B. D. (1991). Exercise and mental health: The mechanisms of exercise-induced psychological states. In L. Diamant (Ed.), Psychology of sports, exercise, and fitness: Social and personal issues (pp. 17–49). Hemisphere Publishing.
- Hayashi, T., Okamoto, E., Nishimura, H., Mizuno-Matsumoto, Y., Ishii, R., & Ukai, S. (2009). Beta activities in EEG associated with emotional stress. International Journal of Intelligent Computing in Medical Sciences & Image Processing, 3(1), 57–68. https://doi.org/https://doi.org/10.1080/1931308X.2009.10644171
- *Hicks, R. A., Hall, P. A., Staines, W. R., & McIlroy, W. E. (2018). Frontal alpha asymmetry and aerobic exercise: Are changes due to cardiovascular demand or bilateral rhythmic movement? Biological Psychology, 132, 9–16. https://doi.org/https://doi.org/10.1016/j.biopsycho.2017.10.011
- *Hilty, L., Langer, N., Pascual-Marqui, R., Boutellier, U., & Lutz, K. (2011). Fatigue-induced increase in intracortical communication between mid/anterior insular and motor cortex during cycling exercise. European Journal of Neuroscience, 34(12), 2035–2042. https://doi.org/https://doi.org/10.1111/j.1460-9568.2011.07909.x
- Hinrichs, H., Scholz, M., Baum, A. K., Kam, J. W., Knight, R. T., & Heinze, H. J. (2020). Comparison between a wireless dry electrode EEG system with a conventional wired wet electrode EEG system for clinical applications. Scientific Reports, 10(1), Article 5218. https://doi.org/https://doi.org/10.1038/s41598-020-62154-0
- *Hottenrott, K., Taubert, M., & Gronwald, T. (2013). Cortical brain activity is influenced by cadence in cyclists. The Open Sports Sciences Journal, 6(1), 9–14. https://pure.mpg.de/rest/items/item_1835316/component/file_2084660/content
- Jenkinson, N., & Brown, P. (2011). New insights into the relationship between dopamine, beta oscillations and motor function. Trends in Neurosciences, 34(12), 611–618. https://doi.org/https://doi.org/10.1016/j.tins.2011.09.003
- Kamijo, K., O'Leary, K. C., Pontifex, M. B., Themanson, J. R., & Hillman, C. H. (2010). The relation of aerobic fitness to neuroelectric indices of cognitive and motor task preparation. Psychophysiology, 47(5), 814–821. https://doi.org/https://doi.org/10.1111/j.1469-8986.2010.00992.x
- Kamijo, K., & Takeda, Y. (2010). Regular physical activity improves executive function during task switching in young adults. International Journal of Psychophysiology, 75(3), 304–311. https://doi.org/https://doi.org/10.1016/j.ijpsycho.2010.01.002
- *Kao, S. C., Wang, C. H., & Hillman, C. H. (2020). Acute effects of aerobic exercise on response variability and neuroelectric indices during a serial n-back task. Brain and Cognition, 138(1), 105–508. https://doi.org/https://doi.org/10.1016/j.bandc.2019.105508
- *Kao, S. C., Wang, C. H., Kamijo, K., Khan, N., & Hillman, C. (2021). Acute effects of highly intense interval and moderate continuous exercise on the modulation of neural oscillation during working memory. International Journal of Psychophysiology, 160(1), 10–17. https://doi.org/https://doi.org/10.1016/j.ijpsycho.2020.12.003
- Klimesch, W., Doppelmayr, M., Schimke, H., & Pachinger, T. (1996). Alpha frequency, reaction time, and the speed of processing information. Journal of Clinical Neurophysiology, 13(6), 511–518. https://journals.lww.com/clinicalneurophys/Abstract/1996/11000/Alpha_Frequency,_Reaction_Time,_and_the_Speed_of.6.aspx
- Klimesch, W., Sauseng, P., & Hanslmayr, S. (2007). EEG alpha oscillations: The inhibition–timing hypothesis. Brain Research Reviews, 53(1), 63–88. https://doi.org/https://doi.org/10.1016/j.brainresrev.2006.06.003
- Kochupillai, V. (2015). Quantitative analysis of EEG signal before and after sudharshana kriya yoga. International Journal Public Mental Health and Neurosciences, 2(2), 19–22. https://sarvasumana.in/files/24.pdf
- Koriath, J. J., Lindholm, E., & Landers, D. M. (1987). Cardiac-related cortical activity during variations in mean heart rate. International Journal of Psychophysiology, 5(4), 289–299. https://doi.org/https://doi.org/10.1016/0167-8760(87)90060-2
- Kropotov, J. D. (2016). Beta and gamma rhythms. In Elsevier (ed.), Functional Neuromarkers for Psychiatry: Applications for Diagnosis and Treatment. (pp. 107–119) Academic Press. https://doi.org/https://doi.org/10.1016/B978-0-12-410513-3.00009-7
- *Kubitz, K. A., & Landers, D. M. (1993). The effects of aerobic training on cardiovascular responses to mental stress: An examination of underlying mechanisms. Journal of Sport & Exercise Psychology, 15(3), 326–337. https://doi.org/https://doi.org/10.1123/jsep.15.3.326
- *Kubitz, K. A., & Mott, A. A. (1996). EEG power spectral densities during and after cycle ergometer exercise. Research Quarterly for Exercise and Sport, 67(1), 91–96. https://doi.org/https://doi.org/10.1080/02701367.1996.10607929
- Lacey, B. C., & Lacey, J. I. (1978). Two-way communication between the heart and the brain: Significance of time within the cardiac cycle. American Psychologist, 33(2), 99–113. https://doi.org/https://doi.org/10.1037/0003-066X.33.2.99
- Lambourne, K., & Tomporowski, P. (2010). The effect of exercise-induced arousal on cognitive task performance: A meta-regression analysis. Brain Research, 1341, 12–24. https://doi.org/https://doi.org/10.1016/j.brainres.2010.03.091
- Lattari, E., Portugal, E., Moraes, H., Machado, S., Santos, T. M., & Deslandes, A. C. (2014). Acute effects of exercise on mood and EEG activity in healthy young subjects: A systematic review. CNS & Neurological Disorders - Drug Targets, 13(6), 972–980. https://doi.org/https://doi.org/10.2174/1871527313666140612125418
- Lau, T. M., Gwin, J. T., & Ferris, D. P. (2012). How many electrodes are really needed for EEG-based mobile brain imaging? Journal of Behavioral and Brain Science, 2(3), Article 22107. https://doi.org/https://doi.org/10.4236/jbbs.2012.23044
- Leff, D. R., Orihuela-Espina, F., Elwell, C. E., Athanasiou, T., Delpy, D. T., Darzi, A. W., & Yang, G. Z. (2011). Assessment of the cerebral cortex during motor task behaviours in adults: A systematic review of functional near infrared spectroscopy (fNIRS) studies. NeuroImage, 54(4), 2922–2936. https://doi.org/https://doi.org/10.1016/j.neuroimage.2010.10.058
- Li, Q., Otsuka, T., Kobayashi, M., Wakayama, Y., Inagaki, H., Katsumata, M., … Kagawa, T. (2011). Acute effects of walking in forest environments on cardiovascular and metabolic parameters. European Journal of Applied Physiology, 111(11), 2845–2853. https://doi.org/https://doi.org/10.1007/s00421-011-1918-z
- Liu, Z., Ding, L., & He, B. (2006). Integration of EEG/MEG with MRI and fMRI. IEEE Engineering in Medicine and Biology Magazine, 25(4), 46–53. https://doi.org/https://doi.org/10.3389/fpsyg.2020.554915
- Luck, S. J. (2014). An introduction to the event-related potential technique (2nd ed.). MIT Press.
- Luck, S. J., Woodman, G. F., & Vogel, E. K. (2000). Event-related potential studies of attention. Trends in Cognitive Sciences, 4(11), 432–440. https://doi.org/https://doi.org/10.1016/s1364-6613(00)01545-x
- *Ludyga, S., Gronwald, T., & Hottenrott, K. (2016). The athlete’s brain: Cross-sectional evidence for neural efficiency during cycling exercise. Neural Plasticity, 2016, Article 4583674. https://doi.org/https://doi.org/10.1155/2016/4583674
- *Ludyga, S., Hottenrott, K., & Gronwald, T. (2017). Four weeks of high cadence training alter brain cortical activity in cyclists. Journal of Sports Sciences, 35(14), 1377–1382. https://doi.org/https://doi.org/10.1080/02640414.2016.1198045
- *Mechau, D., Mücke, S., Liesen, H., & Weiß, M. (1998). Effect of increasing running velocity on electroencephalogram in a field test. European Journal of Applied Physiology and Occupational Physiology, 78(4), 340–345. https://doi.org/https://doi.org/10.1007/s004210050429
- Menicucci, D., Gentili, C., Piarulli, A., Laurino, M., Pellegrini, S., Mastorci, F., Bedini, R., Montanaro, D., Sebastiani, L., & Gemignani, A. (2016). Brain connectivity is altered by extreme physical exercise during non-REM sleep and wakefulness: Indications from EEG and fMRI studies. Archives Italiennes de Biologie, 154(4), 103–117. https://doi.org/https://doi.org/10.12871/00039829201441
- *Mierau, A., Schneider, S., Abel, T., Askew, C., Werner, S., & Strüder, H. K. (2009). Improved sensorimotor adaptation after exhaustive exercise is accompanied by altered brain activity. Physiology and Behavior, 96(1), 115–121. https://doi.org/https://doi.org/10.1016/j.physbeh.2008.09.002
- Moher, D., Liberati, A., Tetzlaff, J., Altman, D. G., & PRISMA Group (2010). Preferred reporting items for systematic reviews and meta-analyses: The PRISMA statement. International Journal of Surgery, 8(5), 336–341. https://doi.org/https://doi.org/10.1016/j.ijsu.2010.02.007
- *Moraes, H., Deslandes, A., Silveira, H., Ribeiro, P., Cagy, M., Piedade, R., Pompeu, F., & Laks, J. (2011). The effect of acute effort on EEG in healthy young and elderly subjects. European Journal of Applied Physiology, 111(1), 67–75. https://doi.org/https://doi.org/10.1007/s00421-010-1627-z
- *Moraes, H., Ferreira, C., Deslandes, A., Cagy, M., Pompeu, F., Ribeiro, P., & Piedade, R. (2007). Beta and alpha electroencephalographic activity changes after acute exercise. Arquivos de Neuro-Psiquiatria, 65(3A), 637–641. https://doi.org/https://doi.org/10.1590/S0004-282X2007000400018
- *Mott, A. A., Dyer, R. A., & Kubitz, K. A. (1995). Effects of exercise on the coherence of human EEG signals at selected hemispheric sites. Biomedical Sciences Instrumentation, 31(1), 1–6. https://pubmed.ncbi.nlm.nih.gov/7654944/
- Nakata, H., Oshiro, M., Namba, M., & Shibasaki, M. (2016). Effects of aerobic exercise under different thermal conditions on human somatosensory processing. American Journal of Physiology-Regulatory Integrative and Comparative Physiology, 311(4), R629–R636. https://doi.org/https://doi.org/10.1152/ajpregu.00153.2016
- National Collaborating Center for Methods and Tools. (2008). Quality assessment tool for quantitative studies. National Collaborating Center for Methods and Tools. https://www.nccmt.ca/knowledge-repositories/search/14
- *Nielsen, B., Hyldig, T., Bidstrup, F., Gonzalez-Alonso, J., & Christoffersen, G. R. J. (2001). Brain activity and fatigue during prolonged exercise in the heat. Pflügers Archiv - European Journal of Physiology, 442(1), 41–48. https://doi.org/https://doi.org/10.1007/s004240100515
- Nielsen, B., & Nybo, L. (2003). Cerebral changes during exercise in the heat. Sports Medicine, 33(1), 1–11. https://doi.org/https://doi.org/10.1016/S0301-0511(96)05198-8
- *Ohmatsu, S., Nakano, H., Tominaga, T., Terakawa, Y., Murata, T., & Morioka, S. (2014). Activation of the serotonergic system by pedaling exercise changes anterior cingulate cortex activity and improves negative emotion. Behavioural Brain Research, 270, 112–117. https://doi.org/https://doi.org/10.1016/j.bbr.2014.04.017
- Oxford English Dictionary. (2021). Sport. https://www.oed.com/view/Entry/187476#eid21245788
- Parr, J. V. V., Gallicchio, G., & Wood, G. (2021). EEG correlates of verbal and conscious processing of motor control in sport and human movement: A systematic review. International Review of Sport and Exercise Psychology. Advance Online Publication, https://doi.org/https://doi.org/10.1080/1750984X.2021.1878548
- Pesonen, M., Hämäläinen, H., & Krause, C. M. (2007). Brain oscillatory 4–30 Hz responses during a visual n-back memory task with varying memory load. Brain Research, 1138, 171–177. https://doi.org/https://doi.org/10.1016/j.brainres.2006.12.076
- *Petruzzello, S. J., & Landers, D. M. (1994). State anxiety reduction and exercise: Does hemispheric activation reflect such changes? Medicine & Science in Sports & Exercise, 26(8), 1028–1035. https://doi.org/https://doi.org/10.1249/00005768-199408000-00015
- *Petruzzello, S. J., & Tate, A. K. (1997). Brain activation, affect, and aerobic exercise: An examination of both state-independent and state-dependent relationships. Psychophysiology, 34(5), 527–533. https://doi.org/https://doi.org/10.1111/j.14698986.1997.tb01739.x
- Plattner, K., Lambert, M. I., Tam, N., Lamberts, R. P., & Baumeister, J. (2014). Changes in cortical beta activity related to a biceps brachii movement task while experiencing exercise induced muscle damage. Physiology & Behavior, 123, 1–10. https://doi.org/https://doi.org/10.1016/j.physbeh.2013.08.022
- Pogosyan, A., Gaynor, L. D., Eusebio, A., & Brown, P. (2009). Boosting cortical activity at beta-band frequencies slows movement in humans. Current Biology, 19(19), 1637–1641. https://doi.org/https://doi.org/10.1016/j.cub.2009.07.074
- Pollack, M. L., Wilmore, H., & Fox, S. M. (1978). Health and fitness through physical activity. Wiley and Sons.
- Protzak, J., & Gramann, K. (2018). Investigating established EEG parameter during real-world driving. Frontiers in Psychology, 9, Article 2289. https://doi.org/https://doi.org/10.3389/fpsyg.2018.02289
- Purves, D., Brannon, E. M., Cabeza, R., Huettel, S. A., LaBar, K. S., Platt, M. L., & Woldorff, M. G. (2008). Principles of cognitive neuroscience. Sinauer Associates.
- Rahman, M., Karwowski, W., Fafrowicz, M., & Hancock, P. A. (2019). Neuroergonomics applications of electroencephalography in physical activities: A systematic review. Frontiers in Human Neuroscience, 13, Article 182. https://doi/org/10.3389/fnhum.2019.00182 https://doi.org/https://doi.org/10.3389/fnhum.2019.00182
- Renjith, V. (2017). Blinding in randomized controlled trials: What researchers need to know? Manipal Journal of Nursing and Health Sciences, 3(1), 45–50. https://ejournal.manipal.edu/mjnhs/docs/Volume%203_Issue%201/Full%20text/9.%20Vishnu.pdf
- Saalmann, Y. B., Pinsk, M. A., Wang, L., Li, X., & Kastner, S. (2012). The pulvinar regulates information transmission between cortical areas based on attention demands. Science, 337(6095), 753–756. https://doi.org/https://doi.org/10.1126/science.1223082
- Samaha, J., Bauer, P., Cimaroli, S., & Postle, B. R. (2015). Top-down control of the phase of alpha-band oscillations as a mechanism for temporal prediction. Proceedings of the National Academy of Sciences, 112(27), 8439–8444. https://doi.org/https://doi.org/10.1073/pnas.1503686112
- Sauseng, P., Griesmayr, B., Freunberger, R., & Klimesch, W. (2010). Control mechanisms in working memory: A possible function of EEG theta oscillations. Neuroscience & Biobehavioral Reviews, 34(7), 1015–1022. https://doi.org/https://doi.org/10.1016/j.neubiorev.2009.12.006
- *Schneider, S., Askew, C. D., Abel, T., Mierau, A., & Strüder, H. K. (2010a). Brain and exercise: A first approach using electrotomography. Medicine & Science in Sports & Exercise, 42(3), 600–607. https://doi.org/https://doi.org/10.1249/mss.0b013e3181b76ac8
- *Schneider, S., Askew, C. D., Abel, T., & Strüder, H. K. (2010b). Exercise, music, and the brain: Is there a central pattern generator? Journal of Sports Sciences, 28(12), 1337–1343. https://doi.org/https://doi.org/10.1080/02640414.2010.507252
- *Schneider, S., Askew, C. D., Diehl, J., Mierau, A., Kleinert, J., Abel, T., Carnahan, H., & Strüder, H. K. (2009a). EEG activity and mood in health orientated runners after different exercise intensities. Physiology & Behavior, 96(4–5), 709–716. https://doi.org/https://doi.org/10.1016/j.physbeh.2009.01.007
- *Schneider, S., Brümmer, V., Abel, T., Askew, C. D., & Strüder, H. K. (2009b). Changes in brain cortical activity measured by EEG are related to individual exercise preferences. Physiology & Behavior, 98(4), 447–452. https://doi.org/https://doi.org/10.1016/j.physbeh.2009.07.010
- Sedlmeier, P., Eberth, J., Schwarz, M., Zimmermann, D., Haarig, F., Jaeger, S., & Kunze, S. (2012). The psychological effects of meditation: A meta-analysis. Psychological Bulletin, 138(6), 1139–1171. https://doi.org/https://doi.org/10.1037/a0028168
- Sellami, M., Gasmi, M., Denham, J., Hayes, L. D., Stratton, D., Padulo, J., & Bragazzi, N. (2018). Effects of acute and chronic exercise on immunological parameters in the elderly aged: Can physical activity counteract the effects of aging? Frontiers in Immunology, 9(2187), 1–17. https://doi.org/https://doi.org/10.3389/fimmu.2018.02187
- *Severtsen, B., & Bruya, M. A. (1986). Effects of meditation and aerobic exercise on EEG patterns. Journal of Neuroscience Nursing, 18(4), 206–210. https://doi.org/https://doi.org/10.1097/01376517-198608000-00010
- Sheel, A. W., Dominelli, P. B., & Molgat-Seon, Y. (2016). Revisiting dysanapsis: Sex-based differences in airways and the mechanics of breathing during exercise. Experimental Physiology, 101(2), 213–218. https://doi.org/https://doi.org/10.1113/EP085366
- Shibasaki, M., Namba, M., Oshiro, M., Kakigi, R., & Nakata, H. (2017). Suppression of cognitive function in hyperthermia; from the viewpoint of executive and inhibitive cognitive processing. Scientific Reports, 7(1), 1–8. https://doi.org/https://doi.org/10.1038/srep43528
- Shookster, D., Lindsey, B., Cortes, N., & Martin, J. R. (2020). Accuracy of commonly used age-predicted maximal heart rate equations. International Journal of Exercise Science, 13(7), 1242–1250. https://www.ncbi.nlm.nih.gov/pmc/articles/PMC7523886/
- Sirota, A., Montgomery, S., Fujisawa, S., Isomura, Y., Zugaro, M., & Buzsáki, G. (2008). Entrainment of neocortical neurons and gamma oscillations by the hippocampal theta rhythm. Neuron, 60(4), 683–697. https://doi.org/https://doi.org/10.1016/j.neuron.2008.09.014
- Smith, P. J., Blumenthal, J. A., Hoffman, B. M., Cooper, H., Strauman, T. A., Welsh-Bohmer, K., Browndyke, J. N., & Sherwood, A. (2010). Aerobic exercise and neurocognitive performance: A meta-analytic review of randomized controlled trials. Psychosomatic Medicine, 72(3), 239–252. https://doi.org/https://doi.org/10.1097/PSY.0b013e3181d14633
- Sousa, A. F. M., Medeiros, A. R., Del Rosso, S., Stults-Kolehmainen, M., & Boullosa, D. A. (2019). The influence of exercise and physical fitness status on attention: A systematic review. International Review of Sport and Exercise Psychology, 12(1), 202–234. https://doi.org/https://doi.org/10.1080/1750984X.2018.1455889
- *Spring, J. N., Tomescu, M. I., & Barral, J. (2017). A single-bout of endurance exercise modulates EEG microstates temporal features. Brain Topography, 30(4), 461–472. https://doi.org/https://doi.org/10.1007/s10548-017-0570-2
- Srinivasan, R., & Nunez, P. L. (2017). Electroencephalography. In Elsevier (ed.), Neuroscience and Biobehavioral Psychology (pp. 1–10) Elsevier. https://doi.org/https://doi.org/10.1016/B978-0-12-809324-5.06408-7
- Steriade, M., Gloor, P. L. R. R., Llinas, R. R., Da Silva, F. L., & Mesulam, M. M. (1990). Basic mechanisms of cerebral rhythmic activities. Electroencephalography and Clinical Neurophysiology, 76(6), 481–508. https://doi.org/https://doi.org/10.1016/0013-4694(90)90001-Z
- Suryadinata, R. V., Wirjatmadi, B., Adriani, M., & Lorensia, A. (2020). Effect of age and weight on physical activity. Journal of Public Health Research, 9(2), 28–31. https://doi.org/https://doi.org/10.4081/jphr.2020.1840
- Swain, D. P., Abernathy, K. S., Smith, C. S., Lee, S. J., & Bunn, S. A. (1994). Target heart rates for the development of cardiorespiratory fitness. Medicine & Science in Sports & Exercise, 26(1), 112–116. https://pubmed.ncbi.nlm.nih.gov/8133731/
- Taheri, B. A., Knight, R. T., & Smith, R. L. (1994). A dry electrode for EEG recording. Electroencephalography and Clinical Neurophysiology, 90(5), 376–383. https://doi.org/https://doi.org/10.1016/0013-4694(94)90053-1
- Takeda, R., & Okazaki, K. (2018). Body Temperature Regulation During Exercise and Hyperthermia in Diabetics. In IntechOpen (Eds.), Diabetes and Its Complications. (pp. 88–109). https://doi.org/https://doi.org/10.5772/intechopen.74063
- Tilley, S., Neale, C., Patuano, A., & Cinderby, S. (2017). Older people’s experiences of mobility and mood in an urban environment: A mixed methods approach using electroencephalography (EEG) and interviews. International Journal of Environmental Research and Public Health, 14(2), Article 151. https://doi.org/https://doi.org/10.3390/ijerph14020151
- Vecchiato, G., Astolfi, L., Tabarrini, A., Salinari, S., Mattia, D., Cincotti, F., Bianchi, L., Sorrentiono, D., Aloise, F., Soranzo, R., & Babiloni, F. (2010). EEG analysis of the brain activity during the observation of commercial, political, or public service announcements. Computational Intelligence and Neuroscience, 2010, Article 985867. https://doi.org/https://doi.org/10.1155/2010/985867
- Wang, C. H., Liang, W. K., Tseng, P., Muggleton, N. G., Juan, C. H., & Tsai, C. L. (2015). The relationship between aerobic fitness and neural oscillations during visuo-spatial attention in young adults. Experimental Brain Research, 233(4), 1069–1078. https://doi.org/https://doi.org/10.1007/s00221-014-4182-8
- *Wollseiffen, P., Ghadiri, A., Scholz, A., Strüder, H. K., Herpers, R., Peters, T., & Schneider, S. (2016a). Short bouts of intensive exercise during the workday have a positive effect on neuro-cognitive performance. Stress and Health, 32(5), 514–523. https://doi.org/https://doi.org/10.1002/smi.2654
- *Wollseiffen, P., Schneider, S., Martin, L. A., Kerhervé, H. A., Klein, T., & Solomon, C. (2016b). The effect of 6 h of running on brain activity, mood, and cognitive performance. Experimental Brain Research, 234(7), 1829–1836. https://doi.org/https://doi.org/10.1007/s00221-016-4587-7
- *Woo, M., Kim, S., Kim, J., Petruzzello, S. J., & Hatfield, B. D. (2009). Examining the exercise-affect dose–response relationship: Does duration influence frontal EEG asymmetry? International Journal of Psychophysiology, 72(2), 166–172. https://doi.org/https://doi.org/10.1016/j.ijpsycho.2008.12.003
- *Woo, M., Kim, S., Kim, J., Petruzzello, S. J., & Hatfield, B. D. (2010). The influence of exercise intensity on frontal electroencephalographic asymmetry and self-reported affect. Research Quarterly for Exercise and Sport, 81(3), 349–359. https://doi.org/https://doi.org/10.1080/02701367.2010.10599683
- World Health Organization. . (2010). Global recommendations on physical activity for health. https://apps.who.int/iris/bitstream/handle/10665/44399/9789241599979_eng.pdf?%20sequence = 1
- Wriessnegger, S. C., Müller-Putz, G. R., Brunner, C., & Sburlea, A. I. (2020). Inter-and intra-individual variability in brain oscillations during sports motor imagery. Frontiers in Human Neuroscience, 14, Article 576241. https://doi.org/https://doi.org/10.3389/fnhum.2020.576241