ABSTRACT
In complex naturalistic sensorimotor behaviour, uncertainty arises from ambiguities and delays in sensory inputs as well as noise in sensory detection and motor execution. In sports, where human capacity reaches its limits, handling uncertainty is crucial. In fundamental motor-control research, five mechanisms for handling uncertainty – multisensory integration, prior-knowledge integration, risk optimisation, redundancy exploitation, and impedance control – have been proposed based on a rich body of evidence, mostly investigating simple arm and hand movement tasks. Here we review the literature investigating more complex tasks and examine to what extent these mechanisms explain handling uncertainty in sensorimotor control in sports. A systematic search following the PRISMA guidelines resulted in the consideration of 82 studies. These studies provide robust empirical evidence for the mechanisms of multisensory integration, prior-knowledge integration, and redundancy exploitation in complex naturalistic behaviour, whilst only a few publications focused on the other two mechanisms. Furthermore, only a few studies test model-based predictions that can be derived from the theoretical frameworks to a satisfactory extent. Finally, beyond discussing these explanatory mechanisms in isolation, we propose a unifying model that builds upon the theory of optimal feedback control, in which the mechanisms can be related to each other coherently.
1. Introduction
Our world is riddled with uncertainty. In sports, for instance, when a climber jumps to a hold she has never touched before, a number of – maybe vital – questions arise. How far is the jump and how big is the swing that has to be absorbed? How good is the hold in terms of shape and friction? Where exactly is the sweet spot of the hold? What is the optimal sequencing of subsequent actions and what is the optimal timing for this sequence? What are the consequences of a fall? In such cases of natural sensorimotor behaviour, uncertainties like these arise from different sources; particularly from ambiguities in the sensed environment (e.g. Kersten et al., Citation2004; Witt & Riley, Citation2014) as well as from noise in sensory and motor systems (e.g. Faisal et al., Citation2008; Körding & Wolpert, Citation2006). Moreover, we have to handle sensory-input delays and typically, the multiple possible solutions to solve a motor task (e.g. Franklin & Wolpert, Citation2011).
When relating these considerations for behavioural control to probabilities, uncertainty can be defined as ‘possible states or outcomes measured by assigning probabilities to each possible state or outcome’ (Sternad, Citation2018, p. 184). This definition can be further specified by the differentiation between expected and unexpected uncertainty and volatility (Bland & Schaefer, Citation2012). Expected uncertainty regards cases when the outcome probabilities are known and stable, like the outcome uncertainty of rolling a six from a dice. Unexpected uncertainty would occur if the dice was suddenly changed to a cheat dice with unusual probabilities, whose probabilities can no longer be predicted by past experience. Finally, volatility means a frequent change in probabilities, for example, due to a frequent change over several cheat dice.
In sports, where human capacities are characteristically brought to their limits, successfully handling different types of uncertainty is crucial. Thus, the question arises of how humans are able to master this challenge. In fundamental sensorimotor-control research, a rich body of evidence has been provided for five mechanisms contributing to the scientific explanation of how humans handle uncertainty in behavioural control (for reviews, e.g. Franklin & Wolpert, Citation2011; Gallivan et al., Citation2018; Körding & Wolpert, Citation2006; Todorov, Citation2004):
(1) | Multisensory integration |
(2) | Prior-knowledge integration |
(3) | Risk optimisation |
(4) | Redundancy exploitation |
(5) | Impedance control |
Since the five reported mechanisms are drawn from foundational motor-control research, most of which examines simple pointing or reaching movements, the question arises whether or to what extent the same mechanisms hold for the handling of sensorimotor uncertainty in more complex real-world situations, as are common in sports. The main goal of this review is therefore to investigate the external validity of these mechanisms. On the one hand, there is no reason to necessarily doubt this, but on the other hand, the external validity of basic research results cannot be taken for granted (see, e.g. Wolpert et al. (Citation2011, p. 748) in their review on computational mechanisms of human motor learning: ‘It is not clear whether the learning models that are developed will generalize to tasks such as tying shoelaces or learning to skateboard.’). Hence, it seems extremely valuable for both sports scientists and practitioners to take a closer look at exactly this question in order to gain a well-grounded knowledge base for handling uncertainty in complex, in particular sports-related tasks. Furthermore, the present review can claim relevance for contexts beyond sports, such as questions of complex sensorimotor control in professional fields or traffic.
In a recent narrative review, Gredin et al. (Citation2020b) propose a Bayesian framework to explain anticipatory behaviour in sports. They summarize multiple studies with overall good evidence in favour of the integration of contextual information into perception. The authors conclude that athletes reduce perceptual uncertainty by weighting different contextual and kinematic information sources according to their reliability and, by this means, enhance anticipatory behaviour. However, this conclusion on multisensory integration was drawn from foundational research on pointing and reaching-and-grasping tasks. Furthermore, by focusing on Bayesian inference in anticipation, Gredin et al. (Citation2020b) do not address additional mechanisms for handling uncertainty in complex motor behaviour; namely, risk optimisation, redundancy exploitation, and impedance control.
Therefore, in the present scoping review, we systematically list and discuss all original articles on handling uncertainty in natural sensorimotor, in particular sports-related tasks. This approach not only builds from a narrative to a scoping review, but also extends investigations on anticipatory behaviour to consider control mechanisms beyond Bayesian inference. Furthermore, we substantially broaden the current view by relating our findings to theoretical approaches rooted in either the ecological or the cognitive branch of motor coordination and control theory.
2. Method
This review followed the guidelines of Tricco et al. (Citation2018) for the Preferred Reporting Items for Systematic Reviews and Meta-Analyses extension for scoping reviews (PRISMA-ScR). The respective checklist can be found in Appendix A.
2.1. Inclusion and exclusion criteria
To be included in the review, the studies had to examine uncertainty aspects related to sensorimotor control in sports. No studies on sensorimotor learning or optimisation were included (e.g. focussing on the optimal degree of fluctuations in practice; Hossner et al., Citation2016). Moreover, studies on scattering in motion were excluded from the current review if there was no examination of task-relevant or -irrelevant variables (e.g. Den Hartigh et al., Citation2015). Studies on Fitts’ or Hick’s law were excluded as they do not address uncertainty at the core (e.g. Sanderson, Citation1983). Furthermore, no clinical or paediatric studies were considered as the focus was not on impairments or the development of the reviewed mechanisms.
The exclusion criterion for studies ‘not related to sensorimotor control in sports’ pertained to those which investigated decision-making behaviour with uncertainty, however, did not deploy a sensorimotor task (e.g. Adie et al., Citation2020). To be considered sports-related and included in the present review, the studies had to meet at least one of the following two conditions: Studies were included that used naturalistic sports-related stimuli (e.g. Gredin et al., Citation2018; Helm et al., Citation2020) or required a motor response beyond pointing or reaching-and-grasping movements, i.e. tasks involving whole-body movements such as throwing or catching (e.g. Stevenson et al., Citation2009). To clarify, if only a simple motor action was requested, but a natural sports-related stimulus was used, the study was still considered, because the complexity of reacting to, for instance, videos of moving persons with a high degree of dynamics in it (even with a button press) seems to be sufficiently close to real-world tasks like pulling the brake at the right moment in downhill biking. However, studies in which no motor response (i.e. exclusively perceptual tasks) was required at all were not included in the review.
Further, only original articles with empirical data published in peer-reviewed journals in English language were included. Finally, it should be noted that the paper of Scott et al. (Citation1997) summarizes the data of several other studies (Berg et al., Citation1994; Hay, Citation1988; Hay & Koh, Citation1988; Lee et al., Citation1982), which will not be considered separately in the results section.
2.2. Identification and screening
The following six academic databases were searched: PsycINFO, PubMed, ScienceDirect, Scopus, SPORTDiscus and Web of Science. The last search was conducted on July 15, 2023, using all searching fields with the following terms: (uncertainty OR noise) AND (sensor* OR motor) AND control AND sport AND movement. Moreover, the search was filtered (if possible) to English and original articles that have undergone a peer-review process. In the Scopus database, the subject area was further limited to social science or psychology due to many hits from other scientific disciplines (e.g. engineering or computer science). The exact search strategy applied to each database is documented in Appendix B.
As illustrated in , a total of 5,168 hits were exported to EndNote. Two raters independently screened all titles and abstracts after removing duplicates. The abstracts were then merged, meaning that the articles remained in the pool for full-text screening if at least one of the two raters judged the abstract as fulfilling the inclusion criteria. Furthermore, in an iterative process, the reference lists of all included studies were systematically screened such that 35 additional articles were included for review. Appendix C contains a table indicating which articles were found in the initial search and which were included from the reference lists. An additional five studies were found by forward citation searches and screening of the reference lists of theoretical articles. In the end, 82 articles were included in the review.
3. Results
The final 82 studies are listed in . The studies are subdivided into the five core mechanisms addressed: multisensory integration, prior-knowledge integration, risk optimisation, redundancy exploitation, and impedance control (including a sixth residual category of further studies). For each subdivision, the studies are sorted alphabetically and characterized by the researched sport, the deployed task, the study type, and the main findings.
Table 1. Studies on mechanisms for handling uncertainties in sensorimotor control in sports, sorted by mechanism and ordered alphabetically.
Overall, when considering the year of publication, it is clear that the popularity of the topic of uncertainty in sensorimotor control in sports has been growing. As illustrated in , the number of publications has rapidly increased over the last ten years.
Figure 2. Number of publications on mechanisms for handling uncertainty in sensorimotor control in sports as a function of year of publication.
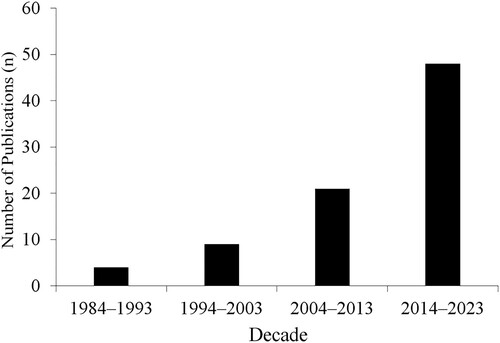
However, there are extensive differences in research interest when it comes to the five mechanisms (1)–(5) specified in the present paper. While a number of studies focus on the question of how multiple sensory inputs are integrated (16 studies), the largest portion of studies examine integrating prior knowledge (40 studies), either to reduce uncertainty in perception, action or to enhance performance. Aspects of risk optimisation have not been addressed to a notable degree thus far (1 study). Alternately, more attention has been attracted to redundancy exploitation (18 studies), relating to the question of which variables have to be controlled for superior performance and which variables are either not task-relevant or can be compensated. To date, the mechanism of impedance control appears to be under researched in sports science (3 studies). Finally, four studies remained with a focus on handling uncertainty in sensorimotor control in sports that cannot be related to the five mechanisms outlined above.
In regard to the type of the study, considerably more laboratory studies (54 studies) were included in the review than field studies (28 studies). However, the ratio of laboratory to field studies seems to depend on the investigated mechanism. While multisensory integration is rather equally studied in both laboratory (8 studies) and field (8 studies), more laboratory (33 studies) than field (7 studies) studies have been conducted on prior-knowledge integration, whereas there are even slightly more field studies (10 studies) investigating the redundancy exploitation than laboratory studies (8 studies). In general, the findings obtained in laboratory and field studies are in line with each other, so this distinction will not be considered further.
The researched sport, deployed tasks and main findings of the included studies are reported in more detail in the following paragraphs, subdivided by the mechanism assumed to be responsible for handling uncertainty in sensorimotor control in sport.
3.1. Studies on multisensory integration
Of the 16 studies focusing on multisensory integration (see ), almost the entirety reaches the conclusion that multiple sources of sensory information are taken into account in order to control complex sports behaviour. As a rare exception, distorted auditory information was found to have no effect on the performance of a table-tennis counter-hit (Petri et al., Citation2020). In the other two exceptions, the addition of visual information alongside auditory information did not improve the accuracy of ball-velocity estimations in soccer penalty shots and volleyball smashes (Sors et al., Citation2017; Sors et al., Citation2018). In contrast, there is considerable evidence that performance decreases when sensory information that is normally available is disturbed (Heinen et al., Citation2014; Kennel et al., Citation2015; Krabben et al., Citation2018; Santello et al., Citation2001; Schaffert et al., Citation2020; Sinnett & Kingstone, Citation2010; Sors et al., Citation2017; Takeuchi, Citation1993) and that performance can be improved by the provision of additional sensory information (Ankarali et al., Citation2014; Gray, Citation2009; O'Brien et al., Citation2020; O'Brien et al., Citation2021; Zelic et al., Citation2012). In line with these findings, it had been shown that providing misleading additional sensory information in a hurdling task (Kennel et al., Citation2015) or experimentally manipulating sound intensity when estimating stroke distances (Cañal-Bruland et al., Citation2018) decreases performance. However, athletes seem to be capable of compensating for misleading auditory signals over time (Kennel et al., Citation2015).
Five of the studies on multisensory integration explicitly reference Bayesian inference. However, apart from the fact that any evidence in favour of the integration of different input sources can be counted as generally fitting the Bayesian framework, according to Bayesian inference, multisensory integration should be based on a weighting procedure according to the inputs’ estimated reliabilities. In this more specific regard, different levels of reliability are considered in three studies. Petri et al. (Citation2020) reported no effects of auditory information in a table-tennis counter-hit regardless of information’s reliability. Kennel et al. (Citation2015) found that delayed – and thus misleading – additional auditory information initially impairs performance in a hurdling task, though this source is increasingly ignored with adaptation over time. In terms of weighting sensory inputs, the most compelling study was conducted by Gray (Citation2009). When only one source of information is available in a baseball-batting simulation, Gray (Citation2009) found that accuracy is best enhanced by visual information. Therefore, this input should be expected to deliver the most reliable information. As consequently predicted, visual information was found to be weighted most heavily when different sources of information are combined in a contradictory manner.
3.2. Studies on prior-knowledge integration
From the total of 82 studies, almost half of the studies (40) are conducted with an either explicit or at least implicit focus on the effects of prior knowledge on perception, action, or performance. There is considerable evidence that prior knowledge influences perception (Arthur & Harris, Citation2021; Gredin et al., Citation2018; Harris, Arthur, Vine, et al., Citation2022b; Nakamoto et al., Citation2022; Yamamoto et al., Citation2019) as well as action (Berg & Hughes, Citation2017, Citation2020; Eckerle et al., Citation2012; Gray, Citation2002; Gredin et al., Citation2018; Güldenpenning et al., Citation2023; Güldenpenning et al., Citation2018; Helm et al., Citation2020; Leukel et al., Citation2012; Loffing et al., Citation2015; Loffing et al., Citation2016; Magnaguagno et al., Citation2022; Magnaguagno & Hossner, Citation2020; McIntyre et al., Citation2001; Milazzo et al., Citation2016; Misirlisoy & Haggard, Citation2014; Sinn et al., Citation2023; Stevenson et al., Citation2009; Triolet et al., Citation2013; Whittier et al., Citation2022; Zago et al., Citation2004), and generally improves performance (Abernethy et al., Citation2001; Crognier & Féry, Citation2005; Farrow & Reid, Citation2012; Gray & Cañal-Bruland, Citation2018; Gredin et al., Citation2018; Gredin et al., Citation2019; Gredin et al., Citation2020c; Gredin et al., Citation2020a; Helm et al., Citation2020; Loffing et al., Citation2015; Loffing & Hagemann, Citation2014; Magnaguagno et al., Citation2022; Magnaguagno & Hossner, Citation2020; Mann et al., Citation2014; Milazzo et al., Citation2016; Murphy et al., Citation2016; Murphy et al., Citation2018; Navia et al., Citation2013; Runswick et al., Citation2018; Wang et al., Citation2019). Only a few incongruences can be reported; namely, considering the role of participants’ expertise levels and the either explicit or implicit provision of contextual information. While Gredin et al. (Citation2018) reported that experts only benefited from explicit (but not from implicit) prior knowledge other studies have shown that experts (but not novices) were able to use implicit prior knowledge (Abernethy et al., Citation2001; Loffing et al., Citation2016). Further specifying the findings that prior knowledge generally improves performance, it has been reported that the explicit provision of uncertain prior knowledge can also have an overall negative effect on performance, especially for expert athletes (Jackson et al., Citation2020; Magnaguagno et al., Citation2022), a finding that we will discuss in more depth below.
Half of the studies on prior-knowledge integration (20/40 studies) refer to principles of Bayesian inference, which provides a tool for identifying statistically optimal solutions and deriving predictions of human behaviour that can be put to empirical test (Griffiths et al., Citation2012). However, most of the studies included in the present review just refer to Bayesian inference as a theoretical framework; meaning that the examined behaviours are not compared to statistically optimal solutions and that the reported results are limited to the general conclusion that prior knowledge influences perception, action, or performance per se. Nevertheless, there is clear evidence of a simplified Bayesian model of reliability-weighted integration of prior knowledge and sensory information into perception, supported by 12 studies. However, only sparse evidence (4 studies) favours quantitative optimality derived from the Bayesian framework; particularly demonstrated by Arthur and Harris (Citation2021) and Harris, Arthur, Vine, et al. (Citation2022b) examining gaze strategies in returning bouncing balls. Notably, when it comes to the interactions of prior-knowledge integration with both the level of expertise and the distinction of explicitly instructed vs. implicitly self-generated knowledge as reported above, such calculations on information-gain estimates can also be found in the handball studies published by Magnaguagno et al. (Citation2022). In these studies, the gain estimates were calculated as a function of certainty of explicitly provided contextual knowledge. These calculations are based on the consideration that if explicit prior knowledge is weighted too heavily, the negative consequences in incongruent trials will outweigh the positive effect in congruent trials. Therefore, the congruence between prior knowledge and actual situational probabilities should also be considered as a crucial factor. Regarding the factor of expertise, it seems plausible that experts are better at estimating the reliability of implicit prior knowledge since they know from experience which information is important and trustworthy – as argued by Williams et al. (Citation2011). Taken together, the summarized findings support the call of Gredin et al. (Citation2020b) for a ‘Bayesian integration framework’ examining the combination of contextual priors and kinematic information in anticipation in sport. However, Bayesian predictions should not only be tested in a more quantitative manner but also be extended from the context of anticipation to the issue of handling noise and uncertainty in complex, naturalistic sensorimotor behaviour in general.
3.3. Studies on risk optimisation
The only included study that focused on risk optimisation was the one published by Bertucco et al. (Citation2020), which showed that inherent noise is taken into account in motor planning in order to optimize potential costs and rewards of the movement outcome. In this study, participants steered a virtual snowboard in a computer game by balancing on a rocker board. As participants passed through different acceleration zones on the slope, they received more financial rewards for reaching faster speeds. When the sensitivity of the rocker board was increased to induce higher inherent noise, the participants chose a larger safety distance to the off-piste penalty zone with the consequence of less acceleration in the middle of the slope. This implies that participants weighed the risk of a penalty with the amount of acceleration – according to different levels of noise – to maximize the financial outcome.
Bertucco et al. (Citation2020) discuss their findings with respect to the theoretical framework of risk optimisation as introduced by Trommershäuser et al. (Citation2008), but also in regards to optimal-control theory (Körding & Wolpert, Citation2006; Todorov, Citation2004), which posits that, motor control ultimately comes down to decision making with a focus on maximizing the utility of the movement outcome in the face of sensory, motor and task uncertainty (Wolpert & Landy, Citation2012).
3.4. Studies on redundancy exploitation
The redundancy exploitation has been introduced above as an alternative – or perhaps supplementary – strategy of weighting available information. Particularly, it provides an alternate approach to handling uncertainty such that sensorimotor noise is only minimized when the noise significantly concerns goal-relevant variables. All 18 studies within this category () confirmed that athletes do not try to reduce movement variance in its entirety, but only in certain task dimensions. This is observed in experts behaviour (Bardy & Laurent, Citation1998; Betzler et al., Citation2014; Bootsma & van Wieringen, Citation1990; Burgess-Limerick et al., Citation1991; Davids et al., Citation1999; Franks et al., Citation1985; Hiley et al., Citation2013; Horan et al., Citation2011; Iino et al., Citation2017; Morrison et al., Citation2014; Morrison et al., Citation2016; Nakano et al., Citation2020; Scott et al., Citation1997; Sheppard & Li, Citation2007; Tucker et al., Citation2013; van Soest et al., Citation2010) as well as in novices behaviour (Burgess-Limerick et al., Citation1991; Dupuy et al., Citation2000; Scholz et al., Citation2000; Scott et al., Citation1997; Sheppard & Li, Citation2007). This prioritisation particularly pertains to task-relevant variables in which superior athletes exhibit less movement variance (Bardy & Laurent, Citation1998; Betzler et al., Citation2014; Hiley et al., Citation2013).
In the more recent articles (Iino et al., Citation2017; Morrison et al., Citation2016; Nakano et al., Citation2020), the distinction between goal-relevant and goal-irrelevant variables is theoretically anchored in the uncontrolled-manifold hypothesis (Scholz et al., Citation2000; Scholz & Schöner, Citation1999). Accordingly, the variance in the control variables is split into a part that affects the task variables and a part that does not. The ratio of these variances then serves as a measure for the degree of redundancy exploitation, and thus also as a measurement of expertise (Iino et al., Citation2017; Morrison et al., Citation2016). To make the distinction between relevant and irrelevant variables for goal achievement, the majority of the reviewed studies draw from the behaviour of experts. In contrast to this purely empirically driven approach, five studies distinguish relevant from irrelevant variables based on functional arguments and compare optimal with actual human behaviour (Betzler et al., Citation2014; Dupuy et al., Citation2000; Hiley et al., Citation2013; Nakano et al., Citation2020; Scholz et al., Citation2000). In this regard, the study on underarm precision throwing by Dupuy et al. (Citation2000) demonstrated that people exploit the laws of physics and minimize the variability of the throwing distance adapting both the release angle and speed close to the predicted mechanical optimum.
3.5. Studies on impedance control
Three studies included in the present review focused on handling uncertainty and noise by adapting one’s impedance with optimal muscular co-contractions. Empirical findings supporting this strategy include performing a handstand with vs. without vibrations of the floor, where vibrations lead to higher muscle co-contractions and wrist torque stiffness (Blenkinsop et al., Citation2016). Furthermore, when the task of balancing a more or less inert stick on the hand became more difficult due to visual-focus instructions or mass distribution and the angular velocity of the stick increased, agonist and antagonist muscle activation increased. The increased joint stiffness due to increased activation of agonist and antagonist allowed the stick to be controlled at a higher frequency (Reeves et al., Citation2013; Reeves et al., Citation2016).
Although not precisely termed as such, motor control in these studies is interpreted as the optimisation of muscular activities as well as joint stiffness through co-activation (Blenkinsop et al., Citation2016; Reeves et al., Citation2013; Reeves et al., Citation2016). These studies can thus be taken as general empirical support for the notion of impedance control as a means for handling noise-related uncertainty by adapting one’s resistance against expected uncertainty. However, more specific predictions regarding the degree to which impedance should be exploited to optimize performance have not been tested so far; though would be a highly desirable exploration for the future.
3.6. Further studies
Due to distinctly different foci, the three remaining studies cannot be assigned to the defined categories. Bar-Eli et al. (Citation2007) show that in penalty situations, soccer goalkeepers tend to jump more often than optimal to the side rather than staying in the middle of the goal. This implies cost functions of the highest relevance are not so obvious, since in the present example, the spectator-related social costs of not acting at all may play a co-decisive role. Goodman et al. (Citation2009) found that rifle shooters wait in a certain range of random movement until the aiming sight happens to point perfectly at the target. The authors explain this strategy by the higher accuracy of the visual than the motor system as a potential further strategy to deal with uncertainty in complex sensorimotor tasks. On the basis of a simple perceptual model with intrinsic positional uncertainty in order to explain challenges in tennis, Mather (Citation2008) recommends, due to perceptual uncertainties, that tennis players should generally take advantage of all the challenges available to them. Finally, Mazyn et al. (Citation2007) tested participants in a trade-off situation where they had to catch a ball and the light was switched of immediately after movement initiation. In this situation participants accepted increased signal-dependent noise of a faster catching movement to gather more ball-flight information.
Although not explicitly relating to one of the mechanisms (1)–(5) identified, these studies should be considered as valuable additions to further our understanding of how humans handle uncertainty in complex motor behaviour.
4. Discussion
This scoping review aimed to examine how humans handle uncertainty in sensorimotor control in naturalistic tasks such as sports and to determine to what extent the well-investigated underlying mechanisms (1)–(5) can be transferred from more fundamental to more complex tasks. The importance of testing the external validity of these mechanisms from fundamental research can be seen in the rapidly growing research interest in uncertainty in sensorimotor control in sports over the last ten years (illustrated in ). However, there are pronounced differences in research interest when it comes to the five mechanisms (1)–(5).
Multisensory integration
(2) | Prior-knowledge integration |
(3) | Risk optimisation |
(4) | Redundancy exploitation |
(5) | Impedance control |
Taken together, these findings – from a broad range of study types and investigated tasks – show that the mechanisms (1), (2), and (4) have been convincingly empirically proven in naturalistic complex sensorimotor tasks. The mechanisms (3) and (5) remain under-researched with some empirical evidence for their external validity in a naturalistic environment. Moreover, regarding the (frequently) self-imposed theoretical framework, the empirical tests of the mechanisms did not always match to a satisfactory extent. Future research should thus more specifically focus on how these mechanisms are utilized by testing clear predictions derived from theoretical models rather than just scratching the surface to show that, for instance, prior knowledge is considered per se. In this respect, the study of Arthur and Harris (Citation2021) could be regarded as a landmark for future research. In this study, not only empirically quantitative predictions of an optimal Bayesian observer were made and compared with actual behaviour, but also eye movements as the first movements in anticipatory behaviour were investigated. Examining eye movements may, therefore, provide conclusive insights into the process of prior-knowledge integration. Further, Magnaguagno et al. (Citation2022) presented quantitative predictions about expected anticipatory behaviour, broadening the empirical focus by considering the effects of further variables, such as the level of expertise or implicit prior-knowledge accumulation versus explicit prior-knowledge provision can be expected to provide additional depth to understand the mechanisms in even more detail. Finally, while considerable evidence for mechanisms (1), (2), and (4) has been provided, the mechanisms of (3) risk optimisation and (5) impedance control are still poorly researched in sports science so far. Therefore, further research is urgently required to determine these mechanisms’ additional or interacting contributions to handling uncertainty in sensorimotor control in naturalistic complex, in particular sports-related tasks. Furthermore, it becomes clear that for future research, different emphases should be set for each mechanism.
Having discussed five explanatory mechanisms largely in isolation, the question arises as to whether and, if so, how these mechanisms might be placed in a single theoretical framework. In seeking such a framework, it should first be noted that not all of the studies included in this scoping review are based on a cognitive approach. In contrast to the Bayesian idea of an accentuated cognitive weighting of probabilities, Gray (Citation2002) applies an alternative model with high predictive power; namely a non-Bayesian two-state Markov model with a fairly simple win-stay, loose-switch heuristic. Moreover, dynamical-systems theory and the ecological approach to movement coordination can undoubtedly contribute to the examination of handling uncertainty. As illustrated in this review by juxtaposing the cognitive principle of minimal intervention (Todorov & Jordan, Citation2002) and the uncontrolled-manifold hypothesis (Scholz & Schöner, Citation1999) rooted in dynamical-systems theory – cognitive and ecological explanations of handling uncertainty in human behaviour may arrive at similar conclusions (e.g. in comparison, Davids et al., Citation1999, p. 439; Franklin & Wolpert, Citation2011, p. 429). On this basis, a unifying framework considering the empirical evidence from fundamental research (for reviews, e.g. Franklin & Wolpert, Citation2011; Gallivan et al., Citation2018; Körding & Wolpert, Citation2006; Todorov, Citation2004) as well as the empirical evidence in more complex, in particular sports-related tasks seems even more highly desirable. To this end, the assumption that coordinated behaviour requires sufficiently reliable online predictions of changes in the world might provide a guiding direction. This idea is presented in more detail in , where the framework of optimal feedback control (according to Körding & Wolpert, Citation2006; and Todorov, Citation2004) is used to integrate the highlighted mechanisms (1)–(5).
Figure 3. Optimal feedback-control loops (Körding & Wolpert, Citation2006, p. 323; Todorov, Citation2004, p. 910; combined and modified by the authors) with indication of mechanisms (1)–(5) for handling uncertainty in sensorimotor control: (1) multisensory integration, (2) prior-knowledge integration,(3) risk optimisation, (4) redundancy exploitation, and (5) impedance control.
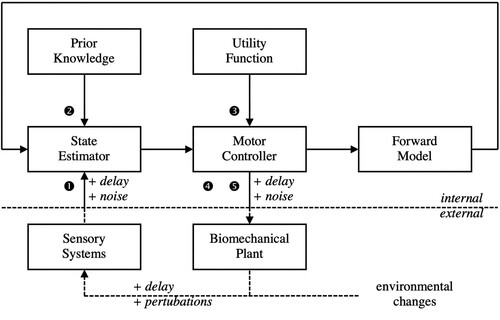
This proposed model is based on the assumption of a close interaction between an internal and an external control loop. The motor controller generates efferent signals dependent on both the estimation of the current state and the specifics of the motor goal according to the utility function. In the internal loop, a forward model predicts how the current state would change based on an internal copy of emitted efferences. In the external loop, the actual changes produced by the biomechanical plant under altering conditions in the external world are observed by the sensory systems. This observation is carried out with a considerable time delay and is corrupted by noise and ambiguities. To reduce uncertainty about the current state, the mechanisms of (1) multisensory integration and (2) prior-knowledge integration come into play. These mechanisms form a continuous integration of all available information sources – perhaps either by Bayesian or alternative procedures – including internal state predictions derived from the forward model. Risk optimisation as mechanism (3) becomes relevant when, on the basis of the estimated state, details of the current motor goal are specified. This regards the intended effect as well as a utility function, where the costs of all possible movement outcomes are considered – especially due to noise and delays in movement execution. Based on internal predictions of the resulting effects, the motor controller determines which manipulations of control variables are required to achieve the desired effect whilst preferably staying within or entering the subspace of optimal task-solutions – thereby instantiating mechanism (4) on redundancy exploitation. Finally, as an alternative to actively handling noise and delays, the motor controller may also adapt the impedance of parts of the biomechanical plant as an optimal solution to the given motor task in terms of mechanism (5) of impedance control such that external perturbations are immediately dampened.
In , we chose optimal feedback control as our theoretical framework because it has become a highly influential theory within the engineering approach to understanding sensorimotor behaviour over the past few decades and, moreover, has been widely confirmed empirically (for reviews, e.g. Franklin & Wolpert, Citation2011; Gallivan et al., Citation2018; Körding & Wolpert, Citation2006; Todorov, Citation2004). In the present context, however, the model serves only as a framework to systematically place and illustrate basic mechanisms for dealing with uncertainty in complex sensorimotor behaviour. This being said it is obvious that alternative models could have been chosen as a framework as well. In our view, this is particularly true for the idea of active inference (Friston, Citation2010; in regards to prior-knowledge integration, see Harris, Arthur, Broadbent, et al., Citation2022a), which operates more at a neuro-implementational rather than an engineering level of explanation. While certain differences in specific theory elements are discussed in the scientific community (for details, see Friston, Citation2011), the two approaches share the theoretical core of viewing perception and action as fundamentally probabilistic based on online predictions and the integration of information according to Bayesian principles. More specifically, and referring to , the approaches share key theoretical concepts by asserting the need for an internal forward/generative model, Bayesian integration of sensory information and prior knowledge/beliefs, and behavioural control optimisation according to costs/surprise. This convergence at the level of key concepts is, in our view, good news for joint and coherent progress in this area.
In precisely this sense, the present review and proposed integrative framework should primarily be understood as a heuristic for stimulating future research on handling uncertainty in complex sensorimotor behaviour, including interactions between multiple mechanisms; for example, how prior knowledge affects impedance control. It is expected that such empirical work will advance our understanding at a theoretical level, hopefully moving us from a general framework to a concise theory enabling the derivation of novel and testable predictions. We would be grateful if readers felt invited to participate in this scientific endeavour.
Acknowledgements
The author would like to thank Catherine Haber for providing manuscript proofreading, Manuel Burger and Maurin Franck for their diverse assistance, particularly in the screening process, and Ralf Kredel for valuable comments on a previous version of the present paper.
Disclosure statement
No potential conflict of interest was reported by the author(s).
Data availability statement
Data available within the article or its supplementary materials.
References
- Abernethy, B., Gill, D. P., Parks, S. L., & Packer, S. T. (2001). Expertise and the perception of kinematic and situational probability information. Perception, 30(2), 233–252. https://doi.org/10.1068/p2872
- Adie, J. M., Renshaw, I., Polman, R., Thompson, M. B., & Mann, D. L. (2020). When in doubt, it’s not out: Match format is associated with differences in elite-level cricket umpires’ leg-before-wicket decisions. Psychology of Sport and Exercise, 51. https://doi.org/10.1016/j.psychsport.2020.101760
- Ankarali, M. M., Sen, H. T., De, A., Okamura, A. M., & Cowan, N. J. (2014). Haptic feedback enhances rhythmic motor control by reducing variability, not improving convergence rate. Journal of Neurophysiology, 111(6), 1286–1299. https://doi.org/10.1152/jn.00140.2013
- Arthur, T., & Harris, D. J. (2021). Predictive eye movements are adjusted in a Bayes-optimal fashion in response to unexpectedly changing environmental probabilities. Cortex, 145, 212–225. https://doi.org/10.1016/j.cortex.2021.09.017
- Bardy, B. G., & Laurent, M. (1998). How is body orientation controlled during somersaulting? Journal of Experimental Psychology: Human Perception and Performance, 24(3), 963–977. https://doi.org/10.1037/0096-1523.24.3.963
- Bar-Eli, M., Azar, O. H., Ritov, I., Keidar-Levin, Y., & Schein, G. (2007). Action bias among elite soccer goalkeepers: The case of penalty kicks. Journal of Economic Psychology, 28(5), 606–621. https://doi.org/10.1016/j.joep.2006.12.001
- Berg, W. P., & Hughes, M. R. (2017). The effect of load uncertainty and foreperiod regularity on anticipatory and compensatory neuromotor control in catching. Motor Control, 21(1), 1–25. https://doi.org/10.1123/mc.2015-0003
- Berg, W. P., & Hughes, M. R. (2020). The effect of load uncertainty on neuromotor control in catching: Gender differences and short foreperiods. Journal of Motor Behavior, 52(3), 318–332. https://doi.org/10.1080/00222895.2019.1622503
- Berg, W. P., Wade, M. G., & Greer, N. L. (1994). Visual regulation of gait in bipedal locomotion: Revisiting Lee, Lishman, and Thomson (1982). Journal of Experimental Psychology: Human Perception and Performance, 20(4), 854–863. https://doi.org/10.1037/0096-1523.20.4.854
- Bernstein, N. A. (1987). Bewegungsphysiologie. J. A. Barth. First publications 1935-1965.
- Bertucco, M., Dunning, A., & Sanger, T. D. (2020). Tuning of standing postural responses to instability and cost function. Neuroscience, 428, 100–110. https://doi.org/10.1016/j.neuroscience.2019.12.043
- Betzler, N. F., Monk, S. A., Wallace, E. S., & Otto, S. R. (2014). The relationships between driver clubhead presentation characteristics, ball launch conditions and golf shot outcomes. Proceedings of the Institution of Mechanical Engineers, Part P: Journal of Sports Engineering and Technology, 228(4), 242–249. https://doi.org/10.1177/1754337114541884
- Bland, A. R., & Schaefer, A. (2012). Different varieties of uncertainty in human decision-making. Frontiers in Neuroscience, 6, 85. https://doi.org/10.3389/fnins.2012.00085
- Blenkinsop, G. M., Pain, M. T. G., & Hiley, M. J. (2016). Evaluating feedback time delay during perturbed and unperturbed balance in handstand. Human Movement Science, 48, 112–120. https://doi.org/10.1016/j.humov.2016.04.011
- Bootsma, R. J., & van Wieringen, P. C. W. (1990). Timing an attacking forehand drive in table tennis. Journal of Experimental Psychology: Human Perception and Performance, 16(1), 21–29. https://doi.org/10.1037/0096-1523.16.1.21
- Burdet, E., Osu, R., Franklin, D. W., Milner, T. E., & Kawato, M. (2001). The central nervous system stabilizes unstable dynamics by learning optimal impedance. Nature, 414(6862), 446–449. https://doi.org/10.1038/35106566
- Burgess-Limerick, R., Abernethy, B., & Neal, R. J. (1991). Experience and backswing movement time variability. Human Movement Science, 10(6), 621–627. https://doi.org/10.1016/0167-9457(91)90019-T
- Cañal-Bruland, R., Müller, F., Lach, B., & Spence, C. (2018). Auditory contributions to visual anticipation in tennis. Psychology of Sport and Exercise, 36, 100–103. https://doi.org/10.1016/j.psychsport.2018.02.001
- Crognier, L., & Féry, Y.-A. (2005). Effect of tactical initiative on predicting passing shots in tennis. Applied Cognitive Psychology, 19(5), 637–649. https://doi.org/10.1002/acp.1100
- Davids, K., Bennett, S., Handford, C., & Jones, B. (1999). Acquiring coordination in self-paced, extrinsic timing tasks: A constraints-led perspective. International Journal of Sport Psychology, 30(4), 437–461.
- Den Hartigh, R. J. R., Cox, R. F. A., Gernigon, C., Van Yperen, N. W., & Van Geert, P. L. C. (2015). Pink noise in rowing ergometer performance and the role of skill level. Motor Control, 19(4), 355–369. https://doi.org/10.1123/mc.2014-0071
- Dupuy, M. A., Mottet, D., & Ripoll, H. (2000). The regulation of release parameters in underarm precision throwing. Journal of Sports Sciences, 18(6), 375–382. https://doi.org/10.1080/02640410050074304
- Eckerle, J. J., Berg, W. P., & Ward, R. M. (2012). The effect of load uncertainty on anticipatory muscle activity in catching. Experimental Brain Research, 220, 311–318. https://doi.org/10.1007/s00221-012-3139-z
- Ernst, M. O., & Banks, M. S. (2002). Humans integrate visual and haptic information in a statistically optimal fashion. Nature, 415(6870), 429–433. https://doi.org/10.1038/415429a
- Faisal, A. A., Selen, L. P., & Wolpert, D. M. (2008). Noise in the nervous system. Nature reviews neuroscience, 9(4), 292–303. https://www.ncbi.nlm.nih.gov/pubmed/18319728
- Farrow, D., & Reid, M. (2012). The contribution of situational probability information to anticipatory skill. Journal of Science and Medicine in Sport, 15(4), 368–373. https://doi.org/10.1016/j.jsams.2011.12.007
- Franklin, D. W., & Wolpert, D. M. (2011). Computational mechanisms of sensorimotor control. Neuron, 72(3), 425–442. https://doi.org/10.1016/j.neuron.2011.10.006
- Franks, I. M., Weicker, D., & Robertson, D. G. E. (1985). The kinematics, movement phasing and timing of a skilled action in response to varying conditions of uncertainty. Human Movement Science, 4(2), 91–105. https://doi.org/10.1016/0167-9457(85)90005-3
- Friston, K. (2010). The free-energy principle: a unified brain theory? Nature Reviews Neuroscience, 11(2), 127–138. https://doi.org/10.1038/nrn2787
- Friston, K. (2011). What is optimal about motor control? Neuron, 72(3), 488–498. https://doi.org/10.1016/j.neuron.2011.10.018
- Gallivan, J. P., Chapman, C. S., Wolpert, D. M., & Flanagan, J. R. (2018). Decision-making in sensorimotor control. Nature Reviews Neuroscience, 19(9), 519–534. https://doi.org/10.1038/s41583-018-0045-9
- Goodman, S., Haufler, A., Shim, J., & Hatfieldd, B. (2009). Regular and random components in aiming-point trajectory during rifle aiming and shooting. Journal of Motor Behavior, 41(4), 367–384. https://doi.org/10.3200/JMBR.41.4.367-384
- Gray, R. (2002). “Markov at the Bat”: A model of cognitive processing in baseball batters. Psychological Science, 13(6), 542–547. https://doi.org/10.1111/1467-9280.00495
- Gray, R. (2009). How do batters use visual, auditory, and tactile information about the success of a baseball swing? Research Quarterly for Exercise and Sport, 80(3), 491–501. https://doi.org/10.1080/02701367.2009.10599587
- Gray, R., & Cañal-Bruland, R. (2018). Integrating visual trajectory and probabilistic information in baseball batting. Psychology of Sport and Exercise, 36, 123–131. https://doi.org/10.1016/j.psychsport.2018.02.009
- Gredin, N. V., Bishop, D. T., Broadbent, D. P., Tucker, A., & Williams, A. M. (2018). Experts integrate explicit contextual priors and environmental information to improve anticipation efficiency. Journal of Experimental Psychology: Applied, 24(4), 509–520. https://doi.org/10.1037/xap0000174
- Gredin, N. V., Bishop, D. T., Williams, A. M., & Broadbent, D. P. (2020a). Integrating explicit contextual priors and kinematic information during anticipation. Journal of Sports Sciences, 39(7), 783–791. https://doi.org/10.1080/02640414.2020.1845494
- Gredin, N. V., Bishop, D. T., Williams, A. M., & Broadbent, D. P. (2020b). The use of contextual priors and kinematic information during anticipation in sport: Toward a Bayesian integration framework. International Review of Sport and Exercise Psychology, 1–25. https://doi.org/10.1080/1750984X.2020.1855667
- Gredin, N. V., Broadbent, D. P., Findon, J. L., Williams, A. M., & Bishop, D. T. (2020c). The impact of task load on the integration of explicit contextual priors and visual information during anticipation. Psychophysiology, 57(6), e13578. https://doi.org/10.1111/psyp.13578
- Gredin, N. V., Broadbent, D. P., Williams, A. M., & Bishop, D. T. (2019). Judgement utility modulates the use of explicit contextual priors and visual information during anticipation. Psychology of Sport and Exercise, 45, 101578. https://doi.org/10.1016/j.psychsport.2019.101578
- Griffiths, T. L., Chater, N., Norris, D., & Pouget, A. (2012). How the Bayesians got their beliefs (and what those beliefs actually are): comment on Bowers and Davis (2012). Psychological Bulletin, 138(3), 415–422. https://doi.org/10.1037/a0026884
- Güldenpenning, I., Jackson, R. C., & Weigelt, M. (2023). Action outcome probability influences the size of the head-fake effect in basketball. Psychology of Sport and Exercise, 68, 102467. https://doi.org/10.1016/j.psychsport.2023.102467
- Güldenpenning, I., Alaboud, M. A. A., Kunde, W., & Weigelt, M. (2018). The impact of global and local context information on the processing of deceptive actions in game sports. German Journal of Exercise and Sport Research, 48(3), 366–375. https://doi.org/10.1007/s12662-018-0493-4
- Harris, D. J., Arthur, T., Broadbent, D. P., Wilson, M. R., Vine, S. J., & Runswick, O. R. (2022a). An active inference account of skilled anticipation in sport: Using computational models to formalise theory and generate new hypotheses. Sports Medicine, 52(9), 2023–2038. https://doi.org/10.1007/s40279-022-01689-w
- Harris, D. J., Arthur, T., Vine, S. J., Liu, J., Abd Rahman, H. R., Han, F., & Wilson, M. R. (2022b). Task-evoked pupillary responses track precision-weighted prediction errors and learning rate during interceptive visuomotor actions. Scientific Reports, 12, Article 22098. https://doi.org/10.1038/s41598-022-26544-w
- Hay, J. G. (1988). Approach strategies in the long jump. International Journal of Sport Biomechanics, 4(2), 114–129. https://doi.org/10.1123/ijsb.4.2.114
- Hay, J. G., & Koh, T. J. (1988). Evaluating the approach in the horizontal jumps. International Journal of Sport Biomechanics, 4(4), 372–392. https://doi.org/10.1123/ijsb.4.4.372
- Heinen, T., Koschnick, J., Schmidt-Maass, D., & Vinken, P. M. (2014). Gymnasts utilize visual and auditory information for behavioural synchronization in trampolining. Biology of Sport, 31(3), 223–226. https://doi.org/10.5604/20831862.1111850
- Helm, F., Cañal-Bruland, R., Mann, D. L., Troje, N. F., & Munzert, J. (2020). Integrating situational probability and kinematic information when anticipating disguised movements. Psychology of Sport and Exercise, 46, 101607. https://doi.org/10.1016/j.psychsport.2019.101607
- Hiley, M. J., Zuevsky, V. V., & Yeadon, M. R. (2013). Is skilled technique characterized by high or low variability? An analysis of high bar giant circles. Human Movement Science, 32(1), 171–180. https://doi.org/10.1016/j.humov.2012.11.007
- Hogan, N. (1984). Adaptive control of mechanical impedance by coactivation of antagonist muscles. IEEE Transactions on Automatic Control, 29(8), 681–690. https://doi.org/10.1109/TAC.1984.1103644
- Horan, S. A., Evans, K., & Kavanagh, J. J. (2011). Movement variability in the golf swing of male and female skilled golfers. Medicine & Science in Sports & Exercise, 43(8), 1474–1483. https://doi.org/10.1249/MSS.0b013e318210fe03
- Hossner, E. J., Käch, B., & Enz, J. (2016). On the optimal degree of fluctuations in practice for motor learning. Human Movement Science, 47, 231–239. https://doi.org/10.1016/j.humov.2015.06.007
- Iino, Y., Yoshioka, S., & Fukashiro, S. (2017). Uncontrolled manifold analysis of joint angle variability during table tennis forehand. Human Movement Science, 56, 98–108. https://doi.org/10.1016/j.humov.2017.10.021
- Jackson, R. C., Barton, H., & Bishop, D. T. (2020). Knowledge is power? Outcome probability information impairs detection of deceptive intent. Psychology of Sport and Exercise, 50), https://doi.org/10.1016/j.psychsport.2020.101744
- Kennel, C., Streese, L., Pizzera, A., Justen, C., Hohmann, T., & Raab, M. (2015). Auditory reafferences: The influence of real-time feedback on movement control. Frontiers in Psychology, 6, 1–6. https://doi.org/10.3389/fpsyg.2015.00069
- Kersten, D., Mamassian, P., & Yuille, A. (2004). Object perception as Bayesian inference. Annual Review of Psychology, 55(1), 271–304. https://doi.org/10.1146/annurev.psych.55.090902.142005
- Körding, K. P., & Wolpert, D. M. (2004). Bayesian integration in sensorimotor learning. Nature, 427(6971), 244–247. https://doi.org/10.1038/nature02169
- Körding, K. P., & Wolpert, D. M. (2006). Bayesian decision theory in sensorimotor control. Trends in Cognitive Sciences, 10(7), 319–326. https://doi.org/10.1016/j.tics.2006.05.003
- Krabben, K. J., van der Kamp, J., & Mann, D. L. (2018). Fight without sight: The contribution of vision to judo performance. Psychology of Sport and Exercise, 37, 157–163. https://doi.org/10.1016/j.psychsport.2017.08.004
- Lee, D. N., Lishman, J. R., & Thomson, J. A. (1982). Regulation of gait in long jumping. Journal of Experimental Psychology: Human Perception and Performance, 8(3), 448–459. https://doi.org/10.1037/0096-1523.8.3.448
- Leukel, C., Taube, W., Lorch, M., & Gollhofer, A. (2012). Changes in predictive motor control in drop-jumps based on uncertainties in task execution. Human Movement Science, 31(1), 152–160. https://doi.org/10.1016/j.humov.2011.04.006
- Loffing, F., & Hagemann, N. (2014). On-court position influences skilled tennis players’ anticipation of shot outcome. Journal of Sport and Exercise Psychology, 36(1), 14–26. https://doi.org/10.1123/jsep.2013-0082
- Loffing, F., Sölter, F., Hagemann, N., & Strauss, B. (2016). On-court position and handedness in visual anticipation of stroke direction in tennis. Psychology of Sport and Exercise, 27, 195–204. https://doi.org/10.1016/j.psychsport.2016.08.014
- Loffing, F., Stern, R., & Hagemann, N. (2015). Pattern-induced expectation bias in visual anticipation of action outcomes. Acta Psychologica, 161, 45–53. https://doi.org/10.1016/j.actpsy.2015.08.007
- Magnaguagno, L., & Hossner, E. J. (2020). The impact of self-generated and explicitly acquired contextual knowledge on anticipatory performance. Journal of Sports Sciences, 38(18), 2108–2117. https://doi.org/10.1080/02640414.2020.1774142
- Magnaguagno, L., Zahno, S., Kredel, R., & Hossner, E.-J. (2022). Contextual information in situations of uncertainty: The value of explicit-information provision depends on expertise level, knowledge acquisition and prior-action congruency. Psychology of Sport and Exercise, 59, 102109. https://doi.org/10.1016/j.psychsport.2021.102109
- Mann, D. L., Schaefers, T., & Cañal-Bruland, R. (2014). Action preferences and the anticipation of action outcomes. Acta Psychologica, 152, 1–9. https://doi.org/10.1016/j.actpsy.2014.07.004
- Mather, G. (2008). Perceptual uncertainty and line-call challenges in professional tennis. Proceedings of the Royal Society B: Biological Sciences, 275, 1645–1651. https://doi.org/10.1098/rspb.2008.0211
- Mazyn, L. I. N., Savelsbergh, G. J. P., Montagne, G., & Lenoir, M. (2007). Planning and on-line control of catching as a function of perceptual-motor constraints. Acta Psychologica, 126(1), 59–78. https://doi.org/10.1016/j.actpsy.2006.10.001
- McIntyre, J., Zago, M., Berthoz, A., & Lacquaniti, F. (2001). Does the brain model Newton's laws? Nature Neuroscience, 4(7), 693–694. https://doi.org/10.1038/89477
- Milazzo, N., Farrow, D., Ruffault, A., & Fournier, J. F. (2016). Do karate fighters use situational probability information to improve decision-making performance during on-mat tasks? Journal of Sports Sciences, 34(16), 1547–1556. https://doi.org/10.1080/02640414.2015.1122824
- Misirlisoy, E., & Haggard, P. (2014). Asymmetric predictability and cognitive competition in football penalty shootouts. Current Biology, 24(16), 1918–1922. https://doi.org/10.1016/j.cub.2014.07.013
- Morrison, A., McGrath, D., & Wallace, E. (2014). Changes in club head trajectory and planarity throughout the golf swing. Procedia Engineering, 72, 144–149. https://doi.org/10.1016/j.proeng.2014.06.083
- Morrison, A., McGrath, D., & Wallace, E. S. (2016). Motor abundance and control structure in the golf swing. Human Movement Science, 46, 129–147. https://doi.org/10.1016/j.humov.2016.01.009
- Murphy, C. P., Jackson, R. C., Cooke, K., Roca, A., Benguigui, N., & Williams, A. M. (2016). Contextual information and perceptual-cognitive expertise in a dynamic, temporally-constrained task. Journal of Experimental Psychology: Applied, 22(4), 455–470. https://doi.org/10.1037/xap0000094
- Murphy, C. P., Jackson, R. C., & Williams, A. M. (2018). The role of contextual information during skilled anticipation. Quarterly Journal of Experimental Psychology, 71(10), 2070–2087. https://doi.org/10.1177/1747021817739201
- Nakamoto, H., Fukuhara, K., Torii, T., Takamido, R., & Mann, D. L. (2022). Optimal integration of kinematic and ball-flight information when perceiving the speed of a moving ball. Frontiers in Sports and Active Living, 4, Article 930295. https://doi.org/10.3389/fspor.2022.930295
- Nakano, N., Inaba, Y., Fukashiro, S., & Yoshioka, S. (2020). Basketball players minimize the effect of motor noise by using near-minimum release speed in free-throw shooting. Human Movement Science, 70, 102583. https://doi.org/10.1016/j.humov.2020.102583
- Navia, J. A., Van der Kamp, J., & Ruiz, L. M. (2013). On the use of situation and body information in goalkeeper actions during a soccer penalty kick. International Journal of Sport Psychology, 44(3), 243–251. https://doi.org/10.7352/IJSP
- O'Brien, B., Juhas, B., Bienkiewicz, M., Buloup, F., Bringoux, L., & Bourdin, C. (2020). Online sonification for golf putting gesture: Reduced variability of motor behaviour and perceptual judgement. Experimental Brain Research, 238(4), 883–895. https://doi.org/10.1007/s00221-020-05757-3
- O'Brien, B., Juhas, B., Bienkiewicz, M., Buloup, F., Bringoux, L., & Bourdin, C. (2021). Sonification of golf putting gesture reduces swing movement variability in novices. Research Quarterly for Exercise and Sport, 92(3), 301–310. https://doi.org/10.1080/02701367.2020.1726859
- Petri, K., Schmidt, T., & Witte, K. (2020). The influence of auditory information on performance in table tennis. European Journal of Human Movement, 45. https://doi.org/10.21134/eurjhm.2020.45.7
- Reeves, N. P., Pathak, P., Popovich, Jr., J. M., & Vijayanagar, V. (2013). Limits in motor control bandwidth during stick balancing. Journal of Neurophysiology, 109(10), 2523–2527. https://doi.org/10.1152/jn.00429.2012
- Reeves, N. P., Popovich, J. M., Vijayanagar, V., & Pathak, P. K. (2016). Less precise motor control leads to increased agonist-antagonist muscle activation during stick balancing. Human Movement Science, 47, 166–174. https://doi.org/10.1016/j.humov.2016.03.006
- Runswick, O. R., Roca, A., Williams, A. M., McRobert, A. P., & North, J. S. (2018). The temporal integration of information during anticipation. Psychology of Sport and Exercise, 37, 100–108. https://doi.org/10.1016/j.psychsport.2018.05.001
- Sanderson, F. H. (1983). The effect of directional uncertainty on reaction time and movement time in a fencing task. Journal of Sports Sciences, 1(2), 105–110. https://doi.org/10.1080/02640418308729667
- Santello, M., McDonagh, M. J., & Challis, J. H. (2001). Visual and non-visual control of landing movements in humans. The Journal of Physiology, 537(1), 313–327. https://doi.org/10.1111/j.1469-7793.2001.0313k.x
- Schaffert, N., Oldag, B., & Cesari, P. (2020). Sound matters: The impact of auditory deprivation on movement precision in rowing. European Journal of Sport Science, 20(10), 1299–1306. https://doi.org/10.1080/17461391.2019.1710265
- Scholz, J. P., & Schöner, G. (1999). The uncontrolled manifold concept: Identifying control variables for a functional task. Experimental Brain Research, 126(3), 289–306. https://doi.org/10.1007/s002210050738
- Scholz, J. P., Schoner, G., & Latash, M. L. (2000). Identifying the control structure of multijoint coordination during pistol shooting. Experimental Brain Research, 135(3), 382–404. https://doi.org/10.1007/s002210000540
- Scott, M. A., Li, F. X., & Davids, K. (1997). Expertise and the regulation of gait in the approach phase of the long jump. Journal of Sports Sciences, 15(6), 597–605. https://doi.org/10.1080/026404197367038
- Sheppard, A., & Li, F.-X. (2007). Expertise and the control of interception in table tennis. European Journal of Sport Science, 7(4), 213–222. https://doi.org/10.1080/17461390701718505
- Sinn, S. R., Berg, W. P., Vachon, G. E., & Hughes, M. R. (2023). The role of anticipatory and reflexive compensatory muscle activation in catching errors under load uncertainty. Experimental Brain Research, 241(5), 1411–1419. https://doi.org/10.1007/s00221-023-06614-9
- Sinnett, S., & Kingstone, A. (2010). A preliminary investigation regarding the effect of tennis grunting: Does white noise during a tennis shot have a negative impact on shot perception? PLoS One, 5(10), e13148. https://doi.org/10.1371/journal.pone.0013148
- Sors, F., Lath, F., Bader, A., Santoro, I., Galmonte, A., Agostini, T., & Murgia, M. (2018). Predicting the length of volleyball serves: The role of early auditory and visual information. PLoS One, 13(12), e0208174. https://doi.org/10.1371/journal.pone.0208174
- Sors, F., Murgia, M., Santoro, I., Prpic, V., Galmonte, A., & Agostini, T. (2017). The contribution of early auditory and visual information to the discrimination of shot power in ball sports. Psychology of Sport and Exercise, 31, 44–51. https://doi.org/10.1016/j.psychsport.2017.04.005
- Sternad, D. (2018). It’s not (only) the mean that matters: Variability, noise and exploration in skill learning. Current Opinion in Behavioral Sciences, 20, 183–195. https://doi.org/10.1016/j.cobeha.2018.01.004
- Stevenson, I. H., Fernandes, H. L., Vilares, I., Wei, K., & Kording, K. P. (2009). Bayesian integration and non-linear feedback control in a full-body motor task. PLoS Computational Biology, 5(12), e1000629. https://doi.org/10.1371/journal.pcbi.1000629
- Takeuchi, T. (1993). Auditory information in playing tennis. Perceptual and Motor Skills, 76(3_suppl), 1323–1328. https://doi.org/10.2466/pms.1993.76.3c.1323
- Todorov, E. (2004). Optimality principles in sensorimotor control. Nature Neuroscience, 7(9), 907–915. https://doi.org/10.1038/nn1309
- Todorov, E., & Jordan, M. I. (2002). Optimal feedback control as a theory of motor coordination. Nature Neuroscience, 5(11), 1226–1235. https://doi.org/10.1038/nn963
- Tricco, A. C., Lillie, E., Zarin, W., O'Brien, K. K., Colquhoun, H., Levac, D., Moher, D., Peters, M. D. J., Horsley, T., Weeks, L., Hempel, S., Akl, E. A., Chang, C., McGowan, J., Stewart, L., Hartling, L., Aldcroft, A., Wilson, M. G., Garritty, C., … Straus, S. E. (2018). PRISMA Extension for Scoping Reviews (PRISMA-ScR): Checklist and Explanation. Annals of Internal Medicine, 169, 467–473. https://doi.org/10.7326/M18-0850
- Triolet, C., Benguigui, N., Le Runigo, C., & Williams, M. (2013). Quantifying the nature of anticipation in professional tennis. Journal of Sports Sciences, 31(8), 820–830. https://doi.org/10.1080/02640414.2012.759658
- Trommershäuser, J., Maloney, L. T., & Landy, M. S. (2003). Statistical decision theory and the selection of rapid, goal-directed movements. Journal of the Optical Society of America A, 20(7), 1419–1433. https://doi.org/10.1364/JOSAA.20.001419
- Trommershäuser, J., Maloney, L. T., & Landy, M. S. (2008). Decision making, movement planning and statistical decision theory. Trends in Cognitive Sciences, 12(8), 291–297. https://doi.org/10.1016/j.tics.2008.04.010
- Tucker, C. B., Anderson, R., & Kenny, I. C. (2013). Is outcome related to movement variability in golf? Sports Biomechanics, 12(4), 343–354. https://doi.org/10.1080/14763141.2013.784350
- van Soest, A. J., Casius, L. J., de Kok, W., Krijger, M., Meeder, M., & Beek, P. J. (2010). Are fast interceptive actions continuously guided by vision? Revisiting Bootsma and van Wieringen (1990). Journal of Experimental Psychology: Human Perception and Performance, 36(4), 1040–1055. https://doi.org/10.1037/a0016890
- Wang, Y., Ji, Q., & Zhou, C. (2019). Effect of prior cues on action anticipation in soccer goalkeepers. Psychology of Sport and Exercise, 43, 137–143. https://doi.org/10.1016/j.psychsport.2019.02.001
- Whittier, T. T., Weller, Z. D., & Fling, B. W. (2022). Novel applications of Bayesian inference clarify sensorimotor uncertainty during stepping movements. Neuropsychologia, 173, 108310–8. https://doi.org/10.1016/j.neuropsychologia.2022.108310
- Williams, A. M., Ford, P. R., Eccles, D. W., & Ward, P. (2011). Perceptual-cognitive expertise in sport and its acquisition: Implications for applied cognitive psychology. Applied Cognitive Psychology, 25(3), 432–442. https://doi.org/10.1002/acp.1710
- Witt, J. K., & Riley, M. A. (2014). Discovering your inner Gibson: Reconciling action-specific and ecological approaches to perception-action. Psychonomic Bulletin & Review, 21(6), 1353–1370. https://doi.org/10.3758/s13423-014-0623-4
- Wolpert, D. M., Diedrichsen, J., & Flanagan, J. R. (2011). Principles of sensorimotor learning. Nature Reviews Neuroscience, 12(12), 739–751. https://doi.org/10.1038/nrn3112
- Wolpert, D. M., & Landy, M. S. (2012). Motor control is decision-making. Current Opinion in Neurobiology, 22(6), 996–1003. https://doi.org/10.1016/j.conb.2012.05.003
- Yamamoto, H., Shinya, M., & Kudo, K. (2019). Cognitive bias for the distribution of ball landing positions in amateur tennis players (Cognitive bias for the motor variance in tennis). Journal of Motor Behavior, 51(2), 141–150. https://doi.org/10.1080/00222895.2018.1440523
- Zago, M., Bosco, G., Maffei, V., Iosa, M., Ivanenko, Y. P., & Lacquaniti, F. (2004). Internal models of target motion: Expected dynamics overrides measured kinematics in timing manual interceptions. Journal of Neurophysiology, 91(4), 1620–1634. https://doi.org/10.1152/jn.00862.2003
- Zelic, G., Mottet, D., & Lagarde, J. (2012). Behavioral impact of unisensory and multisensory audio-tactile events: Pros and cons for interlimb coordination in juggling. PLoS ONE, 7(2), e32308. doi:10.1371/journal.pone.0032308
Appendices
Appendix A. PRISMA-ScR checklist
Table A1. Preferred reporting items for systematic reviews and meta-analyses extension for scoping reviews (PRISMA-ScR) checklist.
Comments
*Item 5, protocol and registration: No protocol was preregistered. However, specific objectives of the review and methods were defined a priori:
Objectives: To provide a complete overview of peer-reviewed research on the topic of handling uncertainty in sensorimotor control in sports, focusing on collating all empirical evidence and contrasting different theoretical approaches.
Methods: Based on the review’s objective, (a) eligibility criteria (pp. 4–6), (b) information sources (pp. 5–6), and (c) search strategy (pp. 5–6) were specified before conducting the review.
**Item 9, study selection: Two raters independently screened all titles and abstracts. The abstracts were then merged, meaning that the record remained in the pool for full-text screening if at least one of the two raters judged the abstract as fulfilling the inclusion criteria. Furthermore, in an iterative process, the reference lists of all included studies were systematically screened, and in appendix C is documented which article is referred to by the other articles. The full-text screening was conducted by the first author. Potentially ambiguous cases were discussed with the other authors and resolved by consensus after referring to the inclusion and exclusion criteria.
***Item 12 / 16, critical appraisal: Only studies published in peer-reviewed journals were considered. Based on the current review’s objective, no quality assessment of individual studies was sought. However, regarding a critical appraisal, theoretical assumptions and their empirical foundation were critically discussed.
****Item 13 synthesis of results: Based on the current review’s objective and the inherent diversity in methodologies and different mechanisms to handle uncertainty, the synthesis of the evidence was made by comparing the number of studies supporting a mechanism and how powerful the theoretical prediction was made (e.g. normative models).
Appendix B. Electronic search strategy
Last search:
15 July, 2023, via Campus Network …
PsycINFO (via OvidSP)
((uncertainty or noise) and (sensor* or motor) and control and movement and sport).af.
limit 1 to (peer reviewed journal and english language and ‘0110 peer-reviewed journal’ and english)
PubMed pubmed.gov
((((uncertainty OR noise)) AND (sensor* OR motor)) AND control) AND sport) AND movement
ScienceDirect
(uncertainty OR noise) and (sensory OR motor) and control and sport and movement
Scopus
(uncertainty OR noise) AND (sensory OR motor) AND control AND sport AND movement AND (LIMIT-TO (PUBSTAGE, ‘final’)) AND (LIMIT-TO (DOCTYPE, ‘ar’)) AND (LIMIT-TO (LANGUAGE, ‘English’)) AND (LIMIT-TO (SUBJAREA, ‘PSYC’) OR LIMIT-TO (SUBJAREA, ‘SOCI’))
SportDiscus (via EBSCOhost)
(uncertainty OR noise) AND (sensor* OR motor) AND control AND sport AND movement
Web of Science
uncertainty OR noise (All Fields) and sensor* OR motor* (All Fields) and control (All Fields) and sport (All Fields) and movement (All Fields) and Articles (Document Types) and English (Languages)