ABSTRACT
Background
The objective of this study was to identify and determine factors associated with patients’ ophthalmic adherence in common ocular conditions from randomized clinical trials (RCT).
Research design methods
A univariate analysis with proportions, a bivariate analysis using polychoric correlations, and logistic regression (LR) models were used. The collected dataset was made up of records from RCT. Using three validated LR models, factors were identified and ranked based on their adjusted odds ratio and their statistical significance to adherence.
Results
A total of 1,087 valid patients were included in this analysis, of which 88.96% presented adherence. All models were calibrated, had a good performance, were well specified and cost-effective using the Hosmer–Lemeshow test, metrics for class imbalance, link test approach and Akaike’s criteriums, respectively.
Conclusion
We identified as determinants for encouraging good ophthalmic adherence the adverse events presented, duration of the study, female sex, and older age; other determinants such as medical condition, protocol treatment, type of treatment and disease are all risk factors for adherence. Improvements in ophthalmic adherence may be achieved by focused attention to young male patients with chronic degenerative diseases such as glaucoma or ocular hypertension (especially those who need combination therapy) and developing medications with reduced side effects.
1. Introduction
Ocular diseases are very common in humans, mainly due to high heritability and an increased use of technology to perform daily tasks and work requirements. They negatively affect the economy, education-related tasks, and work activities of those who suffer from them, reducing their sight and therefore quality of life [Citation1,Citation2]. Increase in life expectancy has been accompanied by a rise in the incidence of chronic diseases, including ophthalmic conditions, leading to a growing number of people with visual impairments or blindness across the world, as well as an increased demand for visual health services [Citation3–6]. For example, the number of people with glaucoma worldwide is expected to increase from 64.3 million in 2013 to 111.8 million in 2040 [Citation4].
Chronic visual diseases accounted for more than 19 million cases of moderate to severe visual impairment in adults aged 50 years and over in 2020, transforming these illnesses into significant targets for prevention and treatment management [Citation3].
At present, multiple causes of visual impairments can be prevented or treated; for example, the use of maximum medical therapy (MMT) in glaucoma [Citation2,Citation7]. MMT is used to determine the association between intraocular pressure (IOP) lowering medications and concomitant-fixed combination (FC) regimens, such as Timolol-Brimonidine-Dorzolamide FC (TBD, Krytantek Ofteno, Laboratorios Sophia, S.A. de C.V., Zapopan, Mexico), in which case MMT is expanded into three types of medications [Citation8]. The use of adequate ophthalmic drugs for long-term treatment can improve quality of life and reduce the probability of developing visual impairment or irreversible blindness [Citation9,Citation10].
Several studies have confirmed that good adherence to a treatment, defined as compliance to a medication regimen, is important to improve disease control, avoid complications, and reduce health care-related costs, especially in older people taking multiple treatments [Citation11–14]. However, between 30 and 50% of adults in the United States of America who suffer from a chronic disease do not adhere to their treatments, producing more than 100 billion dollars in avoidable annual costs and an estimated 125,000 deaths [Citation15,Citation16]. Constant adherence to long-term treatments is essential for disease control, therefore the objective of a treatment therapy is to obtain 100% adherence from the patients’ side [Citation17]. In real life practice however, lower thresholds are accepted as allowable adherence. Currently, the most used percentage to define ‘good’ adherence is 80% [Citation17,Citation18]. Nevertheless, the clinically significant threshold may vary depending on disease, type of treatment, patient characteristics, and expected clinical results, among other factors. As an example, patients with glaucoma or ocular hypertension (OHT) need ‘aggressive’ treatments to lower their IOP, and in some cases at least 90% adherence to ocular antihypertensives is required to reach an IOP within the target range [Citation19]. Meanwhile, in patients with dry eye disease (DED), adherence of 70% to ophthalmic lubricants is sufficient to observe a significant clinical improvement [Citation20].
Despite efforts to improve adherence rates in chronic diseases such as glaucoma, diabetes or arterial hypertension, these values remain considerably low [Citation11]. Adherence can be affected by multiple factors, such as forgetting treatment at home, regimen complexity, failing filling out the prescription, incidence of adverse events (AE), or lack of access to public health services or health insurance [Citation11,Citation21]. In addition, it has been described that the psychiatric and neurological state of mind of the patient, as well as their mood or cognitive impairment, can also affect their adherence to drug regimens [Citation21,Citation22]. Other factors associated with chronic disease adherence are patient income, educational level, cultural characteristics, lifestyle, and regimen length, among others [Citation22–25]. Therefore, the spectrum of variables that can modulate a patient’s adherence to a pharmacological therapy range from the characteristics of the drug, type of pathology, and even more complex situations dealing with intrinsic factors associated with the patient.
The development and improvement of predictive models of adherence can help to understand the compliance to medical regimens and facilitate educating the patient about its importance, as well as personalize their treatment management [Citation22]. The use of machine learning (ML) algorithms, such as logistic regression, are useful to construct predictive models that aim to identify and explain the medication adherence factors in ophthalmic diseases, such as current adherence of the patient as a predictor of their future adherence [Citation26–29].
Data collected from randomized clinical trials (RCTs) are important sources of measurements whose collective characteristics allow them to be used reliably and to make inferences. However, accurately measuring adherence to medication is difficult to evaluate, and the variety of adherence measures or calculation methods has led to divergent outcomes [Citation30]. Traditionally, patient self-reporting, health insurance data and the weight of the dropper bottle are common methods for monitoring ophthalmic medications adherence [Citation31].
This study aims to identify the determinants associated with adherence in patients receiving ophthalmic drugs, who present common conditions such as acute medical conditions (AMC), chronic medical conditions (CMC), and chronic degenerative diseases (CDD), obtained from RCTs in Mexico and Colombia. The results will help to identify risk factors for patients with ophthalmologic treatments.
2. Patients and methods
Properly designed and conducted RCTs are essential in evidence-based medical practice, they are used for drug regulatory approval and are included in clinical practice guidelines [Citation32]. Adherence may also be an indicator of research quality and treatment acceptance by participants. This was a meta-analysis of data collected from a cohort study using data from patients’ records at nine previously conducted RCTs, registered on clinicaltrials.gov with the following registration IDs: NCT02801617, NCT03257813, NCT03223909, NCT03521791, NCT03693989, NCT03696342, NCT04111965, NCT04702802, and NCT0704531. The studies included were randomized, parallel, prospective, and controlled trials. They were conducted in 57 sites of Mexico and Colombia after an ethics committee for each RCT reviewed and approved each study’s protocol and its corresponding informed consent form (See Table S1). All studies were conducted in compliance with Good Clinical Practices Standards. Every patient included in our database provided written and signed informed consent and was recruited between October 2015 and June 2022. For more information about the main RCTs see the Supplementary Material (Table S2).
2.1. Data source and description
Clinical data recollection was retrieved electronically and hosted by Laboratorios Sophia, S.A. de C.V. Data included subject protocol information, date of study, patient first visit, patient last visit, and adherence to their respective protocol arm. Total adherence was measured by the records of the subject’s study diary and corroborated by the weight of each eye dropper bottle.
This study focused on factors measured during the conduction of RCTs. The following variables were included in the dataset: subject ID, country, study time (days), age, sex, study diagnosis (refers to the participant’s clinical condition or disease i.e., conjunctivitis, DED, primary open-angle glaucoma [POAG] or OHT, post-cataract surgery, and pterygium), severity of medical condition (AMC, CMC, CDD), treatment group (dry eye drops, hypotensive drops, antibiotic drops, corticosteroid drops and non-steroid anti-inflammatory drug [NSAID] drops), protocol arm-treatment (refers to intervention to which the patients were randomized in their respective study, i.e. single dose unit [SDU], FC, preservative-free [PF] drug, see Table S3), dosage, number of days of treatment (dose length), co-morbidity diagnoses within the main diagnosis in the study period, incidence of AE, if the patient complete the entire protocol without major deviations, and total adherence. For more details about each study see Tables S2 and S4.
Total adherence was calculated for each pharmaceutical drug that each patient was taking. Adherence was defined as ≥ 80% of total prescribed dose over the duration of treatment and non-adherence as a patient with less than 80% of it.
2.2. Statistical analysis
The statistical analysis was carried out based on the intention to treat model, which is a widely accepted method implemented in RCTs. For continuous variables, we used the mean with its standard deviation (SD) and percentages for categorical variables (). This analysis was made to explore factors that might support an explanation related to adherence and the potential associations between them.
Figure 1. Distribution of factors for patients who were adherent and non-adherent to ophthalmic drugs.
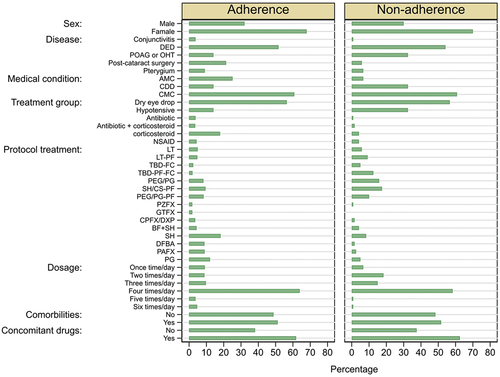
Firstly, we performed an exploratory analysis using polychoric correlations to investigate the intensity of the relationship between predictors for adherence, this helps to avoid the multicollinearity problem in LR models. Secondly, using three LR models we identified the determinants associated with adherence to ophthalmic drugs. Lastly, we adjusted the odds ratios (ORs) and their 95% confidence intervals (95% CI) for adherence so we could explain adherence to ophthalmic drugs. A p-value <0.05 was considered statistically significant. This methodology is useful because of its power of interpretation using ORs, mathematical support, and simple computational requirements.
To assess the performance of LR models, calibration, discrimination, specification, and parsimonies were evaluated. Calibration involved comparing expected episodes to observed episodes across the entire risk range and was evaluated using the Hosmer–Lemeshow (H-L) goodness of fit test. Discrimination refers to the ability of a model to identify patients who present adherence or no adherence, and it was evaluated using metrics for class imbalance-handling such as: precision, recall, F1 score and Precision-Recall curve (PR-AUC) based on previously published works, where TP: True Positives; TN: True Negatives; FP: False Positives; and FN: False Negatives [Citation21,Citation27,Citation33,Citation34]. The precision of the model is the probability that it predicts a TP for an observation when the outcome is indeed positive, and recall refers to the probability that the model predicts a TN for an observation when the outcome is indeed negative. The PR-AUC was used to show the relationship between the TP rate and FP rate at certain thresholds of a binary classifier [Citation35]. To investigate the model specification, we used the link test approach, which is based on the idea that if a regression model is properly specified, it should be able to find no additional independent variables (such as the square of predicted value) that are significant, except by chance. Finally, the parsimonies of models were assessed with the Akaike Information Criterion (AIC) and Bayesian Information Criterion (BIC) metrics.
All statistical and graphic analysis were performed in Stata version 16.1 (Stata Corporation, College station, Texas, U.S.A.).
3. Results
3.1. Data source and description
The database analyzed contained information of 1,144 patients prescribed with least once a day ophthalmic drug treatment on 66,361 days of dosing history. It seems reasonable to assume that the data reflects a representative view of patterns of ophthalmic drug adherence in a similar patient population.
A total of 1,144 patients were included in this study, from which nine were excluded because of them being younger than 18 and older than 90 years old (0.8%, 9/1,144). One patient was discarded for no reported protocol treatment, another for staying only one day in the RCT, two patients weren’t included due to their adherence being reported as a zero and a one (data entry errors), the adherence total of 43 patients was not available, and finally one patient was excluded due to study time (less one week), resulting in a total of 1,087. Demographic and baseline characteristics of the patients are shown in . The mean age ± SD was 54.6 ± 17.2 years old, and 68.2% of the patients were female. A total of 557 patients (51.2%) had co-morbidities, from which the most common class of reported diseases was hypertension (24.7%), followed by diabetes mellitus (12.5%). Remarkably, 673 patients (61.9%) were users of at least one concomitant drug, without necessarily presenting a diagnosis of comorbidity. In the exploratory analysis, 36.9% of patients reported at least one adverse event, of which, 85.4% were considered mild, 13.8% moderate and 0.8% severe. The most common class of reported AE was burning sensation in eye (30.7%), followed by ocular itching (8.5%), and foreign body sensation in eye (7.3%). A total of 1,030 patients (90.8%) completed their respective protocol without deviations up to their last visit (patient last visit day). The distribution of factors for adherence and non-adherence are presented in .
Table 1. Baseline characteristics.
3.2. Correlation among factors and adherence
We used a polychoric correlation analysis to quantify the strength, statistical significance, and direction of factors associated to adherence and non-adherence. This helped us to construct LR models considering the high correlation between the event of interest (adherence) and factors, as well as the correlation between predictors, that avoid multicollinearity problems and inconsistent of estimators in LR models.
We identified a high positive correlation between adherence and the patient completing the study protocol (Rho = 0.90, S.E = 0.02), the protocol treatment showed a medium positive correlation with adherence (Rho = 0.34, S.E = 0.04), dosage showed a low and positive correlation (Rho = 0.16, S.E = 0.05) and adverse events count also presented a low and positive correlation (Rho = 0.13, S.E = 0.06). Other low strength correlations were treatment group and study time (days). Furthermore, correlation analysis allowed us to identify that age is correlated with medical condition, treatment group, incidence of adverse events, and the use of concomitant drugs. Study condition (disease) showed a high correlated with dosage, treatment group, protocol treatment (study arm-treatment) and medical condition. Medical condition is highly correlated with treatment group, use of concomitant drugs, comorbidities, and study time. The correlation analysis of all factors associated with adherence are summarized and shown in .
3.3. Determinants of adherence to ophthalmic treatment
After this exploratory and correlation analysis, data from 1,087 patients were included to adjust three LR models, but only 1,069 were considered valid. Model one (M1) included as factors medical condition and other predictors to investigate the adherence, but not the type of treatment and disease. Model two (M2) included the type of treatment but not the medical condition and disease. Finally, model three (M3) included disease type but not the medical condition and type of treatment.
The percentage of patients with non-adherence to their treatment was 11.0% (imbalanced problem), thus, variables correlated with adherence were included in the LR models. Ten variables were used to build these three LR models: age, sex, severity of medical condition, study arm-treatment, duration of study, number of adverse events, uses of concomitant drugs, type of treatment, disease and as control variables, if the patient completed the entire protocol and country. Seven determinants showed statistical significance (p < 0.05) to treatment adherence in patients with ophthalmic conditions, these factors are shown in . When the factors were contained in our three LR models we determined the following averages of estimated odds ratios and confidence intervals: age OR = 1.02 (95% CI [1.001–1.040]), female gender OR = 2.211 (95% CI, [1.177–4.137]) with respect to male, medical condition (CDD) OR = 0.028 (95% CI [0.004–0.204]), Hypotensive (TBD-PF-FC) OR = 0.118 (95% CI [0.038–0.367]), dry eye drop (PEG/PG) OR = 0.01 (95% CI [0.001–0.078]), dry eye drop (SH/CS-PF) OR = 0.014 (95% CI [0.002–0.117]), dry eye drop (PEG/PG-PF) OR = 0.023 (95% CI [0.002–0.236]), duration of study in days OR = 1.044 (95% CI [1.014–1.074]), number of adverse events presented OR = 1.37 (95% CI [1.026–1.828]), treatment hypotensive OR = 0.020 (95% CI [0.003–0.113]), and disease POAG or OHT OR = 0.030 (95% CI [0.001–0.488]). Additionally, we included the factor of presence of concomitant drugs, which was not significative (p = 0.057) but acceptable for p < 0.06.
Figure 3. Factors significantly associated with adherence to ophthalmic drugs. *p < 0.05, **p < 0.01, ***p < 0.001; horizontal lines: 95% CI; vertical line: OR = 1 (no effect).
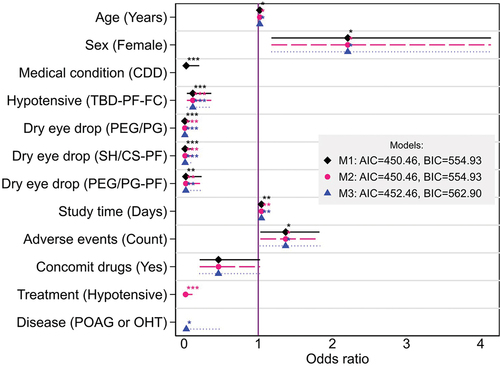
A good model has a best fit, is parsimonious (small or most efficient), and is biologically reasonable to describe the relationship between an outcome and a set of predictors [Citation36]. This study demonstrates the effectiveness of an LR model approach to identify determinants in patients which ophthalmic conditions who are at risk of future non-adherence.
We determined the probability cutoff point of M1, M2, and M3 as 0.906 using Youden’s J statistic, also called Youden’s index [Citation37], this value is where precision (TP) and recall (TN) have an optimum classification for adherence (see Figure S1). The cutoff point was used for evaluating the precision, recall, F1 score, and PR-AUC metrics, that showed good discrimination considering the class imbalance problem ().
Table 2. Metrics for evaluate the LR models performance considering class imbalanced.
We found that H-L for M1, M2, and M3, showed good calibration. A link test also confirmed correct specification of the three models. The AIC and BIC were similar for all models, showing adequate parsimonies (). We present ROC-AUC only as a reference, although it’s not a recommended metric for the class imbalance-problem.
The observed and expected adherence/non-adherence values with their respective thresholds used for metrics limits are shown in , where a very well adjustment for M1 can be observed. The other models achieved a similar fitting of adjusted parameters, as shown in and .
4. Discussion
Failure to take medication as prescribed is a main concern globally. The World Health Organization (WHO) has claimed that up to 50% of drugs prescribed for long-term diseases are not taken as intended [Citation38]. Even in RCTs, 50% of the patients discontinue within the first year of follow-up [Citation39]. One of the major strategies to improve adherence is to educate patients about their disease, as well as to simplify their therapeutic regiment (i.e. the use of FC drugs) [Citation11].
Previous studies have identified factors affecting adherence, calculating the degree of impact by univariate analysis of predictors, and designing a model using LR. A few of the risk factors associated with the adherence of targeted knee osteoarthritis patients were gender, age, educational level, height, NSAID treatment, body mass index, and disease duration [Citation26]. Meanwhile, in patients with type 2 diabetes, the associated factors were age, gender, whether the prior fasting blood glucose was under control, duration of the treatment regimen, diet, hyperlipoidemia, and body mass index [Citation22]. Sociodemographic variables (age, sex, urbanicity, among others) and health-related variables (diagnostics, use of antidepressant, polypharmacy, etc.) have been determined as robust indicators for the clinician to identify non-adherent patients for monitoring, especially in patients with mental disorders [Citation40]. Therefore, to maximize the benefit of pharmacological interventions, the identification of patient populations susceptible to low medication adherence values would make it possible to focus efforts on that target population using strategies aimed at improving their adherence.
To manage this issue, our study assessed determinant factors associated with adherence in patients receiving ophthalmic drugs using data from patient records of previously conducted RCTs in Mexico and Colombia in the past 7 years.
The limitation inherent to this study design is related to the source of the data. Researchers have used systematic reviews to investigate the association between medication regime complexity and adherence using data from RCTs, cross-sectional, cohort, or case-control studies with conclusive results [Citation41–43]. Identifying the risks that can negatively impact the success of the study population, treatment pattern, outcome measurement, or motivations for patients to adhere to study drugs is necessary. Adherence data can influence the interpretation of trial results, affecting the magnitude of an observed treatment efficacy [Citation44]. While poor adherence may mask a true treatment difference among therapies; the RCTs included in this study are heterogeneous and representative of an array of common ophthalmic diseases, drugs used, and lengths of follow-up [Citation36]. The factors included in our models were like significant variables found in previous studies of other health areas [Citation26,Citation27,Citation45]. Age, sex, severity of medical condition, study arm-treatment, duration of study time, if the patient completes their respective protocol, uses of concomitant drugs, and number of AE presented by each patient, were included.
The LR models identified female gender, older age, number of AE presented by each patient, and duration of study as variables with the highest OR for adherence. Patients with chronic degenerative diseases (glaucoma and OHT), and users of MMT therapy and ocular lubricants points to an association too but given significant lows ORs.
Contrary to popular belief, medication adherence is often higher among patients aged 65 to 80 years old compared to younger adults under 50 [Citation46]. In fact, older age can be a determining factor of good adherence, rather than poor adherence. As shown in our results, adherence is better in elderly patients compared to younger patients. Age is a protective factor, and in those aged 65 years and older, the probability of the patient having a good adherence increase by a factor of 1.02. Older people usually have multiple chronic health problems that require ongoing healthcare interventions [Citation14,Citation41]. The use of concomitant drugs could be considered a risk factor for adherence. A limited number of studies have evaluated the number the medications as a risk factor [Citation14,Citation47], while in our study, this factor is accepted for p < 0.06 as indicative of risk for adherence. The relationship between age and adherence can be influenced by environment, sex, and comorbidities, as stated before. Ultimately, it is important to consider the unique needs of patients when it comes to medication adherence to ensure that appropriate actions are in place to promote successful management of their health [Citation46].
Studies have reported an association between gender and medication adherence; suggesting that women may have a higher adherence rate for a specific set of conditions. Hormones such as estrogens and androgens may affect immune activation differently, with estrogens enhancing and androgens suppressing it [Citation48]. The correlation of women being more likely to adhere to medical treatments than men is a subject of interest in the medical field, and as of now, we can say it has been proven true in this study related to ophthalmic diseases. For sex, the odds that a patient has a good adherence is 2.2 times higher in women than if the patient was a male. These results differ from those of Rolnick et al., who evaluated the patients’ characteristics associated with medication adherence, and found that it was most often men who were more adherent. The authors hypothesized it may be due to women, who are frequently the primary caregivers, spending less time and energy taking care of themselves [Citation49].
Adverse side effects can be a concern for patients who are prescribed medication regardless of their underlying disease. These effects may range from mild to moderate, but understanding the treatment plan and practicing effective communication between healthcare providers and patients is a must [Citation50]. Non-adherence has been associated with medication side effects, length of treatment, and pill burden, all which impact treatment failure. In our study, the percentage of patients that presented at least one AE was 36.9% and the number of AE reported for each patient was identified as a determinant of adherence, opposite to previously reported findings [Citation51]. However, in these studies, the incidence of AE was higher, and their findings focused on patients that had experienced side effects such as risk factors and didn’t contrast the number of AE presented by each patient or their severity.
Patients in RCTs generally report side effects earlier and more frequently than clinicians or common patients. Another important aspect to take into consideration is that the patients under RCT data belonged to a Latino population. Culturally, the AE self-reported by common Latin patients are barely frequent, reporting only severe or lethal AE. Treatments with worse AE profiles may falsely prove to be of similar safety if patients are non-adherent due to these AE. For this reason, adherence is a relevant indicator of the acceptance of treatment by patients [Citation51].
It is probably assumed that our results overestimated this factor. The number of AE was in our case a protective factor, increasing 1.4 times the probability of presenting good adherence in those patients that presented more AEs, relevant in the context of the data source. This could be associated with the fact that enrolled patients are well-trained and have been provided enough knowledge about the study and side effects of the drugs, which differ from common patients, where a good adherence is more likely to occur as the number of AE presented is low.
Regardless of the ophthalmic conditions evaluated, the most common way to treat glaucoma is through topical medical therapy. According to American Academy of Ophthalmology guidelines, one of the goals of glaucoma therapy is to control IOP within a target range. As mentioned, when patients are in use of a monotherapy for glaucoma treatment and an elevation of their IOP is recognized; this indicates that it is time to introduce a different management, i.e., a triple therapy. If used correctly and persistently, the treatment outcome can be considerable good regarding of the patient’s own benefit [Citation10].
Most of the medication used currently in glaucoma treatment therapy come either in a bottle or an SDU. Some people find it harder to use SDUs compared to bottles for their eyedrops. However, using a simple therapeutic regimen, administering drops less frequently can help make this process easier. It is particularly important to engage in treatment during the first 6 months of using topical therapy, when adherence is likely to be at its best.
In terms of the capacity to reduce IOP, PF formulations are as effective as preserved formulations while also having lesser ocular AE [Citation8]. In our model, patients with CDD (POAG and OHT) and users of MMT therapy (like TBD) point to an association, but give significant low ORs, which indicate that a good adherence is less likely another simple therapeutic regimen. Often timolol, brimonidine, and dorzolamide are used as the famously known triple therapy; these ocular drugs work together to further lower IOP. Previous research has shown that the use of this combination helps patients reduce the number of times they need to apply the product, therefore reducing the risk of forgetting to administer the drug, leading to knowledge that this effective medical therapy requires a low level of maintenance to achieve a high-level adherence [Citation8,Citation19].
Similar findings were observed in our model for patients’ use of ophthalmic lubricants. DED is a condition that affects millions of lives around the world, regardless of the etiology of DED, ocular lubricants such as SH/CS (Humylub Ofteno, Laboratorios Sophia, S.A. de C.V., Zapopan, Mexico) or PEG/PG (Systane Ultra, Alcon Research. Ltd, Fort Worth, Texas, U.S.A.) are traditionally the therapeutic tool most frequently used, even in mild cases of the condition. These lubricants have a low significant OR in our model (0.012, 0.015, and 0.025, respectively), which can be associated with the lack of adherence of DED patients. However, it has been demonstrated that QID dosing scheme of either lubricant was not superior when compared to non-adherence (uses of < 80% of indicate treatment) in terms of reducing symptoms and signs of DED [Citation20,Citation52]. A related consideration for the clinician to identify non-adherent patients’ users of ocular lubricants, especially in those that require normalizing the parameters of the ocular surface and downgrading of squamous metaplasia.
Lastly, as previously reported, a short-term follow-up of 6 weeks revealed that attending more doctor appointments indirectly made patients more likely to adhere to their medical regimen, resulting in a positive outcome toward their own health benefits. In long-term studies, the odds that a patient had a good adherence increased versus short-term follow-up. This can be explained because those patients under treatment for acute diseases (such as a conjunctivitis or an inflammatory process after a surgery) can suspend the use of the treatment when starting to ‘feel better,’ in contrast to patients who suffer from chronic disease, where a constant adherence to a long-term treatment is essential for the control of the illness [Citation29,Citation45]. At least 60% of cases of suspected or culture-positive acute bacterial conjunctivitis are self-limiting within 1 to 2 weeks of presentation [Citation53]. We did not find effects related to adherence in any acute condition or significant ORs for antibiotic users. Several studies have found no significant differences in clinical cure rate when a less frequent antibiotic dosage is used regardless of better adherence [Citation53,Citation54]. It appears reasonable to assume that the use of antibiotics or anti-inflammatory drugs in acute conditions does not represent an ‘adherence issue.’
We have clear evidence of the determinants shown to be associated with adherence to ophthalmic drugs in patients with ophthalmic conditions using data from RCTs. However, the study’s limitation includes the lack of classification of the non-adherent patients. It is possible to assume that with a dataset from a real-world evidence study or using online tools, the results could have been more conclusive for non-adherent patients [Citation55,Citation56]. Another option for a study on those patients who do not follow good adherence, is to collect a larger sample to build a predictive model. It is recommended to carry out a future case study that allows the integration of an ad-hoc sample to extrapolate it.
A disadvantage of using RCT patients is that subjects may fail to reflect actual clinical performance of patients sufficiently to represent the entire population, given the set of inclusion and exclusion criteria. Another disadvantage is that it would be difficult to apply our findings to several patients selected by set criteria in RCTs to diversified situations that could happen in the real world [Citation57].
A problem with adherence studies is the inability to measure adherence accurately and reliability, making it harder to find true differences, which could have contributed to the variable effects observed in RCTs. In this sense, several studies used patient self-report measures, which are known to overestimated adherence [Citation58]. To minimizing this problem, in our study adherence was measured by the records of the subject’s study diary and corroborated by the weight of each eye dropper bottle.
Nonetheless, our results are clinically important in several ways. The relevance of this study is that health care professionals can identify the determinant factors of patients who do follow the recommended treatment, which allows for more personalized attention to those with poor adherence, improving the control of these diseases, avoiding complications, and reducing healthcare costs [Citation59]. Patients who are at risk of poor adherence need reinforcement and motivation to continue with their treatment properly, especially young adult men with CDD treated with MMT.
5. Conclusions
In conclusion, we identified as determinants for adherence the number of adverse events presented, duration of the study, female sex, and older age, as well as medical condition, protocol treatment, type of treatment, and disease were risk factors for adherence. These findings may further underscore the need for enhancements in ocular care services. One strategy to improve adherence is to educate the patient about their disease and treatment. Improvements in ophthalmic adherence may also be achieved by focused attention to young males and patients with chronic degenerative diseases such as glaucoma or ocular hypertension, especially in those who needs combination therapy, and developing medication drug with reduced side effects.
Abbreviations
AE | = | adverse events |
AIC | = | acute medical conditions |
BIC | = | Bayesian Information Criterion |
CDD | = | chronic degenerative diseases |
CMC | = | chronic medical conditions |
DED | = | dry eye disease |
FC | = | fixed combination |
IOP | = | intraocular pressure |
LR | = | logistic regression |
ML | = | machine learning |
MMT | = | maximum medical therapy |
OHT | = | ocular hypertension |
PEG/PG | = | Systane Ultra |
PF | = | preservative-free |
POAG | = | primary open-angle glaucoma |
RCT | = | randomized clinical trials |
SDU | = | single dose unit |
SH/CS | = | Humylub Ofteno PF |
TBD | = | Timolol-Brimonidine-Dorzolamide |
WHO | = | The World Health Organization |
Declaration of interest
P Muñoz-Villegas and O Olvera-Montaño are employed by Laboratorios Sophia, S.A. de C.V. This commercial affiliation did not have any additional role in the data collection. The authors have no other relevant affiliations or financial involvement with any organization or entity with a financial interest in or financial conflict with the subject matter or materials discussed in the manuscript apart from those disclosed.
Reviewer disclosures
Peer reviewers on this manuscript have no relevant financial or other relationships to disclose.
Author contributions
All authors substantially contributed to the conception and design, data acquisition, or analysis and interpretation of data; took part in drafting the article or revising it critically for important intellectual content; gave final approval of the version to be published; and agreed to be accountable for all aspects of the work. All authors helped to draft the article, and all authors have read and approved the final article.
Ethics statement
All study protocols and their respective informed consent form were approved by their respective Institutional Review Boards (see Table S1).
Supplemental Material
Download MS Word (124.5 KB)Acknowledgments
The authors thank Dr. José María Torres Arellano, PhD for his assistance on data curation and Dr. Sarahí del Carmen Gómez Macías, MD for her assistance writing the original manuscript.
Data availability statement
The dataset analyzed for this study can be found in the Open Science Framework repository (https://osf.io) as DOI 10.17605/OSF.IO/2WJKV.
Supplementary material
Supplemental data for this article can be accessed online at https://doi.org/10.1080/17512433.2023.2279740
Additional information
Funding
References
- Stevens GA, White RA, Flaxman SR, et al. Global prevalence of vision impairment and blindness. Ophthalmol. 2013;120:2377–2384. doi: 10.1016/j.ophtha.2013.05.025
- Pezzullo L, Streatfeild J, Simkiss P, et al. The economic impact of sight loss and blindness in the UK adult population. BMC Health Serv Res. 2018;18:63. doi: 10.1186/s12913-018-2836-0
- Steinmetz JD, Bourne RRA, Briant PS, et al. Causes of blindness and vision impairment in 2020 and trends over 30 years, and prevalence of avoidable blindness in relation to VISION 2020: the right to sight: an analysis for the Global burden of disease study. Lancet Glob Health. 2021;9:e144–60. doi: 10.1016/S2214-109X(20)30489-7
- Tham Y-C, Li X, Wong TY, et al. Global prevalence of glaucoma and projections of glaucoma burden through 2040. Ophthalmol. 2014;121:2081–2090. doi: 10.1016/j.ophtha.2014.05.013
- Hashemi H, Khabazkhoob M, Nabovati P, et al. The prevalence of age-related eye disease in an Elderly population. Ophthalmic Epidemiol. 2017;24:222–228. doi: 10.1080/09286586.2016.1270335
- Nguyen DD, Luo L-J, Yang C-J, et al. Highly retina-permeating and long-acting resveratrol/metformin nanotherapeutics for enhanced treatment of macular degeneration. ACS Nano. 2023;17:168–183. doi: 10.1021/acsnano.2c05824
- Luo L-J, Nguyen DD, Lai J-Y. Benzoic acid derivative-modified chitosan-g-poly(N-isopropylacrylamide): methoxylation effects and pharmacological treatments of glaucoma-related neurodegeneration. JControlled Release. 2020;317:246–258. doi: 10.1016/j.jconrel.2019.11.038
- Gómez-Aguayo F, Paczka JA, Leñero-Córdova R, et al. A phase III randomized clinical Trial of a 0.5% Timolol + 0.2% Brimonidine + 2.0% Dorzolamide fixed combination, preservative-free ophthalmic solution vs. 0.5% Timolol + 0.2% Brimonidine + 2.0% Dorzolamide fixed combination in patients with controlled primary Open-angle glaucoma. Ophthalmol Ther. 2018;7:145–156. doi: 10.1007/s40123-018-0128-8
- Luo L-J, Nguyen DD, Lai J-Y. Dually functional hollow ceria nanoparticle platform for intraocular drug delivery: A push beyond the limits of static and dynamic ocular barriers toward glaucoma therapy. Biomaterials. 2020;243:119961. doi: 10.1016/j.biomaterials.2020.119961
- Cate H, Bhattacharya D, Clark A, et al. Patterns of adherence behaviour for patients with glaucoma. Eye. 2013;27:545–553. doi: 10.1038/eye.2012.294
- Meier-Gibbons F, Töteberg-Harms M. Influence of cost of care and adherence in glaucoma management: an update. J Ophthalmol. 2020;2020:1–5. doi: 10.1155/2020/5901537
- Miura T, Kojima R, Mizutani M, et al. Effect of digoxin noncompliance on hospitalization and mortality in patients with heart failure in long-term therapy: a prospective cohort study. Eur J Clin Pharmacol. 2001;57:77–83. doi: 10.1007/s002280100272
- Cramer JA, Roy A, Burrell A, et al. Medication compliance and persistence: terminology and definitions. Value Health. 2008;11:44–47. doi: 10.1111/j.1524-4733.2007.00213.x
- Cross AJ, Elliott RA, Petrie K, et al. Interventions for improving medication-taking ability and adherence in older adults prescribed multiple medications. Cochrane Database Syst Rev. 2020;2020. doi: 10.1002/14651858.CD012419.pub2
- Bilger M, Wong TT, Lee JY, et al. Using adherence-contingent rebates on chronic disease treatment costs to promote medication adherence: results from a randomized controlled Trial. Appl Health Econ Health Policy. 2019;17:841–855. doi: 10.1007/s40258-019-00497-0
- Kumamaru H, Lee MP, Choudhry NK, et al. Using Previous medication adherence to predict future adherence. J Manag Care Spec Pharm. 2018;24:1146–1155. doi: 10.18553/jmcp.2018.24.11.1146
- Lo-Ciganic W-H, Donohue JM, Thorpe JM, et al. Using machine learning to examine medication adherence thresholds and risk of hospitalization. Med care. 2015;53:720–728. doi: 10.1097/MLR.0000000000000394
- Sackett D, Gibson E, Taylor DW, et al. RANDOMISED CLINICAL TRIAL of STRATEGIES for IMPROVING MEDICATION COMPLIANCE in PRIMARY HYPERTENSION. Lancet. 1975;305:1205–1207. doi: 10.1016/S0140-6736(75)92192-3
- Costa VP, Aung T, Konstas AG. Evolution of the treatment paradigm for maximum medical therapy in glaucoma. Expert Rev Ophthalmol. 2019;14:33–42. doi: 10.1080/17469899.2019.1570138
- Belalcázar-Rey S, Sánchez Huerta V, Ochoa-Tabares JC, et al. Efficacy and safety of sodium hyaluronate/chondroitin sulfate preservative-free ophthalmic solution in the treatment of dry eye: a clinical Trial. Curr Eye Res. 2021;46:919–929. doi: 10.1080/02713683.2020.1849733
- Koesmahargyo V, Abbas A, Zhang L, et al. Accuracy of machine learning-based prediction of medication adherence in clinical research. Psychiatry Res. 2020;294:113558. doi: 10.1016/j.psychres.2020.113558
- Wu X-W, Yang H-B, Yuan R, et al. Predictive models of medication non-adherence risks of patients with T2D based on multiple machine learning algorithms. BMJ Open Diabetes Res Care. 2020;8:e001055. doi: 10.1136/bmjdrc-2019-001055
- Krueger KP, Berger BA, Felkey B. Medication adherence and persistence: a comprehensive review. Adv Ther. 2005;22:313–356. doi: 10.1007/BF02850081
- Otón T, Carmona L, Urruticoechea-Arana A, et al. Discordance between doctor and patient assessments and non-adherence to subcutaneous biological drugs. Rheumatol Int. 2019;39:1077–1082. doi: 10.1007/s00296-019-04304-w
- Westberg A, Sjölander M, Glader E-L, et al. Primary non-adherence to preventive drugs and associations with beliefs about medicines in stroke survivors. Patient Prefer Adherence. 2022;16:343–352. doi: 10.2147/PPA.S351001
- Park KK, Choi CH, Ha C-W, et al. The effects of adherence to non-steroidal anti-inflammatory drugs and factors influencing drug adherence in patients with knee osteoarthritis. J Korean Med Sci. 2016;31:795. doi: 10.3346/jkms.2016.31.5.795
- Marras C, Monteagudo I, Salvador G, et al. Identification of patients at risk of non-adherence to oral antirheumatic drugs in rheumatoid arthritis using the compliance questionnaire in rheumatology: an ARCO sub-study. Rheumatol Int. 2017;37:1195–1202. doi: 10.1007/s00296-017-3737-y
- Schmid-Mohler G, Thut MP, Wüthrich RP, et al. Non-adherence to immunosuppressive medication in renal transplant recipients within the scope of the integrative model of behavioral prediction: a cross-sectional study. Clin Transplant. 2010;24:213–222. doi: 10.1111/j.1399-0012.2009.01056.x
- Zongo A, Simpson S, Johnson JA, et al. Change in trajectories of adherence to lipid‐lowering drugs following non‐fatal acute coronary syndrome or stroke. J Am Heart Assoc. 2019 8;8(23). doi: https://doi.org/10.1161/JAHA.119.013857
- Cate H, Bhattacharya D, Clark A, et al. A comparison of measures used to describe adherence to glaucoma medication in a randomised controlled trial. Clin Trial. 2015;12:608–617. doi: 10.1177/1740774515592636
- Erras A, Shahrvini B, Weinreb RN, et al. Review of glaucoma medication adherence monitoring in the digital health era. Br J Ophthalmol. 2023;107:153–159. doi: 10.1136/bjophthalmol-2020-317918
- Harrington RA. Trials and tribulations of randomized clinical trials. Circulation. 2022;146:380–382. doi: 10.1161/CIRCULATIONAHA.122.060649
- Brooks KM, Castillo-Mancilla JR, Morrow M, et al. Adherence to direct-acting antiviral therapy in people actively using drugs and alcohol: the INCLUD study. Open Forum Infect Dis. 2021 8;8(1). doi: https://doi.org/10.1093/ofid/ofaa564
- Mino-León D, Reyes-Morales H, Flores-Hernández S. Effectiveness of involving pharmacists in the process of ambulatory health care to improve drug treatment adherence and disease control. J Eval Clin Pract. 2015;21:7–12. doi: 10.1111/jep.12207
- Cook J, Ramadas V. When to consult precision-recall curves. Stata J. 2020;20:131–148. doi: 10.1177/1536867X20909693
- Brunner HI, Giannini EH. Trial design, measurement, and analysis of clinical investigations. In Textbook of pediatric rheumatology. Elsevier; 2011. p. 127–156. doi: 10.1016/B978-1-4160-6581-4.10007-X.
- Youden WJ. Index for rating diagnostic tests. Cancer. 1950;3:32–35. doi: 10.1002/1097-0142(1950)3:1<32:AID-CNCR2820030106>3.0.CO;2-3
- Gu Y, Zalkikar A, Liu M, et al. Predicting medication adherence using ensemble learning and deep learning models with large scale healthcare data. Sci Rep. 2021;11:18961. doi: 10.1038/s41598-021-98387-w
- Vrijens B, Vincze G, Kristanto P, et al. Adherence to prescribed antihypertensive drug treatments: longitudinal study of electronically compiled dosing histories. BMJ. 2008;336:1114–1117. doi: 10.1136/bmj.39553.670231.25
- Pedrosa-Naudín MA, Gutiérrez-Abejón E, Herrera-Gómez F, et al. Non-adherence to antidepressant treatment and related factors in a region of Spain: a population-based registry study. Pharmaceutics. 2022;14:2696. doi: 10.3390/pharmaceutics14122696
- Pantuzza LL, Ceccato MDG, Silveira MR, et al. Association between medication regimen complexity and pharmacotherapy adherence: a systematic review. Eur J Clin Pharmacol. 2017;73:1475–1489. doi: 10.1007/s00228-017-2315-2
- Alves-Conceição V, Rocha KSS, Silva FVN, et al. Are clinical outcomes associated with medication regimen complexity? A systematic review and meta-analysis. Ann Pharmacother. 2020;54:301–313. doi: 10.1177/1060028019886846
- Nieuwlaat R, Wilczynski N, Navarro T, et al. Interventions for enhancing medication adherence. Cochrane Database Syst Rev. 2014;2014. doi: 10.1002/14651858.CD000011.pub4
- Zhang Z, Peluso MJ, Gross CP, et al. Adherence reporting in randomized controlled trials. Clin Trial. 2014;11:195–204. doi: 10.1177/1740774513512565
- Benedict C, Wang J, Reppucci M, et al. Cost of survivorship care and adherence to screening—aligning the priorities of health care systems and survivors. Transl Behav Med. 2021;11:132–142. doi: 10.1093/tbm/ibz182
- Burnier M, Polychronopoulou E, Wuerzner G. Hypertension and drug adherence in the elderly. Front Cardiovasc Med. 2020;7. doi: 10.3389/fcvm.2020.00049
- Gray SL, Mahoney JE, Blough DK. Medication adherence in elderly patients receiving home health services following hospital discharge. Ann Pharmacother. 2001;35:539–545. doi: 10.1345/aph.10295
- Boucquemont J, Pai ALH, Dharnidharka VR, et al. Gender differences in medication adherence among adolescent and young adult kidney transplant recipients. Transplantation. 2019;103:798–806. doi: 10.1097/TP.0000000000002359
- Rolnick SJ, Pawloski PA, Hedblom BD, et al. Patient characteristics associated with medication adherence. Clin Med Res. 2013;11:54–65. doi: 10.3121/cmr.2013.1113
- Allen CF, Simon Y, Edwards J, et al. Adherence to antiretroviral therapy by people accessing services from non-governmental HIV support organisations in three Caribbean countries. West Indian Med J. 2011;60:269–275.
- López-Campos JL, Quintana Gallego E, Carrasco Hernández L. Status of and strategies for improving adherence to COPD treatment. Int J Chron Obstruct Pulmon Dis. 2019;14:1503–1515. doi: 10.2147/COPD.S170848
- Baiza-Durán LM, Muñoz-Villegas P, Sánchez-Ríos A, et al. Efficacy and safety of an ophthalmic DMPC-Based nanoemulsion in patients with dry eye disease: a phase I/II randomized clinical Trial. J Ophthalmol. 2023;2023:1–9. doi: 10.1155/2023/1431473
- Azari AA, Barney NP. Conjunctivitis. JAMA. 2013;310:1721. doi: 10.1001/jama.2013.280318
- Szaflik J, Szaflik JP, Kamińska A, et al. Clinical and microbiological Efficacy of levofloxacin administered three times a day for the treatment of bacterial conjunctivitis. Eur J Ophthalmol. 2009;19:1–9. doi: 10.1177/112067210901900101
- Tsioufis K, Castellano Vázquez JM, Sykara G, et al. Real-world evidence for adherence and persistence with atorvastatin therapy. Cardiol Ther. 2021;10:445–464. doi: 10.1007/s40119-021-00240-8
- Chen C, Li X, Su Y, et al. Adherence with cardiovascular medications and the outcomes in patients with coronary arterial disease: “Real‐world” evidence. Clin Cardiol. 2022;45:1220–1228. doi: 10.1002/clc.23898
- Kim H-S, Lee S, Kim JH. Real-world evidence versus randomized controlled Trial: clinical research based on electronic medical records. J Korean Med Sci. 2018;33(34):33. doi: https://doi.org/10.3346/jkms.2018.33.e213
- Stephenson BJ. Is this patient taking the treatment as prescribed? JAMA. 1993;269:2779. doi: 10.1001/jama.1993.03500210079036
- Tannenbaum SL, McClure LA, Zheng DD, et al. Ocular screening adherence across Hispanic/Latino heritage groups with diabetes: results from the ocular SOL ancillary to the Miami site of the hispanic community health study/study of latinos (HCHS/SOL). BMJ Open Diabetes Res Care. 2016;4:e000236. doi: 10.1136/bmjdrc-2016-000236