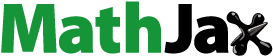
ABSTRACT
Urban densification, a response to population growth, poses adverse impacts on built environments. Limited access to sunlight is one of these consequences. Solar radiation and daylight affect urban canyons in different ways, including thermal comfort and illuminance levels. A balance between summer and solar heat gain can alleviate solar thermal considerations. This becomes even more important in an urban context like Western Sydney with an air temperature of around 6 to 10 degrees hotter than Inner Sydney (during extreme heat events). Additionally, Western Sydney is predicted to absorb two-thirds of the population growth of the Sydney region. Nevertheless, the urban development trend in this region doesn’t seem to meet the requirements of an environmentally sustainable scheme covering solar geometry-based design principles. Therefore, this study aims to develop a general urban model based on the spatial analysis of the western Sydney suburbs and propose a novel integrated methodology to provide the optimal solar-based urban design solution. This process uses an evolutionary algorithm as a computational tool to optimize solar radiation and daylight levels in extreme weather conditions. Results offer the optimum value for a wide range of design parameters regarding solar geometry.
1. Introduction
Rapid urbanization has exerted excessive pressure on contemporary urban development processes to address population growth and migration trends, leading to rampant densification of the built environment (Cheng et al., Citation2006a). It is generally assumed that urban densification poses negative impacts on the environment, such as an increase in the urban heat island effect (UHI), thermal discomfort (Giridharan et al., Citation2004), inadequate solar radiation, natural ventilation, and the potential for harnessing utmost capabilities of renewable energy sources (Cheng et al., Citation2006a). Studies have shown that more than 70% of occupants within dense urban environments are dissatisfied with the lighting conditions of indoor spaces (Chan et al., Citation2008) due to the inadequate amount of daylight exposure (Ng, Citation2003). Daylight quality is an essential aspect of Indoor Environmental Quality that can directly affect a user's mental and physical health (Chan et al., Citation2008). Studies suggest that the adequacy of daylight in interior spaces improves workers’ comfort and productivity levels (Andersen et al., Citation2012). Adequate daylight also results in potential energy-saving by reducing electrical lighting energy demand and associated sensible cooling load (Li & Wong, Citation2007). However, solar radiation is also a significant source of heat (D.Colton, Citation2013) and the exposure to direct sunlight can cause occupants to become uncomfortable and dissatisfied. Heat stress exerted from the external ground and buildings’ facades (Chatzipoulka et al., Citation2016) further reduces the thermal comfort of occupants in both indoor and outdoor spaces. This also holds true for Sydney, Australia, especially during hot summers, with temperature ranges often exceeding the comfort threshold (Reardon & Downton, Citation2008).
Although daylight availability has a crucial role in office building design, solar heat gain remains the most important environmental factor to be considered in housing-oriented developments (Strømann-Andersen and Sattrup, Citation2011). Solar heat gains directly influence the amount of energy consumed for a building’s cooling and heating demand during the summer and winter seasons, respectively. Direct solar radiation can greatly affect human thermal comfort, especially in outdoor urban environments (Van Esch et al., Citation2012).
Solar radiation can significantly be restricted in dense urban environments facing obstructions such as neighboring building blocks (Van Esch et al., Citation2012). Several studies have investigated the amount of solar radiation falling on a building’s facade with the intent of improving either thermal conditions or energy use (Chatzipoulka et al., Citation2016; Van Esch et al., Citation2012). There is an excellent potential for harvesting solar energy using building-integrated PV (BIPV) systems (Biloria et al., Citation2023). Buildings’ facades and roofs offer an excellent context for PV installation and electricity generation to reduce the peak-time pressure on the grid. Different urban studies have focused on solar energy saving (Bouyer et al., Citation2011) or generation potentials (Compagnon, Citation2004; Hofierka & Kaňuk, Citation2009; Kämpf et al., Citation2010). Urban morphology itself can vary thermal load in a hot, humid climate (Lima et al., Citation2019) and energy consumption in an arid climate (Ratti et al., Citation2005) by 16-18% and 10%, respectively. The amount of solar radiation is the most commonly investigated indicator for energy potential estimation, and it can be increased in an optimized urban layout (in Greater London) by 45% and 9% on buildings’ facades and roofs, respectively (Sarralde et al., Citation2015).
Recent studies utilize parametric or sensitivity analysis to assess an urban block's performance, in which block settings are considered hypothetically in both uniform and non-uniform frames. For example, the generic model of Cheng et al. in 2006 consisted of both uniform and random blocks of 100*100 m, in which the correlation between urban form, density, and solar potential was investigated (Cheng et al., Citation2006b). In another study, a 3*3 uniform urban block was chosen to investigate the correlation between the solar potential at an urban scale using optimization processes (Vermeulen et al., Citation2018). Martins et al. used sensitivity analysis in 5 typical configurations to conduct multi-objective research on the solar potential for energy generation. Maintaining an acceptable indoor daylight level was one of the objectives of this research and was considered a design constraint (Martins et al., Citation2014). Reinhart et al. conducted a daylight-based urban zoning analysis in 2017, which investigated the optimum floor area ratio in 50 different block typologies using the SDAFootnote1 daylight metric (Saratsis et al., Citation2017). In a performance-based assessment, a generic base-case model of 35m*35 m office building was selected to evaluate the impact of nearby buildings’ shading effect on daylight and energy use objectives. Results indicate that adjacent obstructions strongly affect the amount of daylight received and the resultant energy demand of buildings. These parameters should be critically considered while developing a design process as a vital urban environmental factor (Li & Wong, Citation2007).
Among other design parameters, floor area ratio and orientation were the most commonly used indicators, as they elaborate the typology and height of buildings (Natanian et al., Citation2019). Vatholomaios utilized sensitivity analysis and proved that the shape factor and orientation impact heating and cooling energy use among geometrical parameters of urban forms (Vartholomaios, Citation2017). In another study by Martins et al., the urban aspect ratio parameterFootnote2 has proven to be the most influential factor affecting solar irradiation (Martins et al., Citation2016). In all these studies, various design parameters, including shape factor, floor area ratio, site coverage, orientation, aspect ratio, sky view factor, distance between buildings, and average building height, are considered to evaluate the performance of the built environment (Natanian et al., Citation2019).
However, a shortcoming in the design process is that even if an initial performance-based evaluation is run during the project, buildings are usually considered as a single object, and the complexities within the urban neighborhood, including the shadowing effect of other buildings are typically neglected. This shortcoming inspired the aims of the presented research to establish a correlation between building facades and urban-scale design parameters for evaluating solar parameters of daylight sufficiency, solar heat gain on buildings’ facades, and energy generation potentials (on roofs) using an evolutionary computing-based workflow.
2. Study context
Greater Western Sydney (GWS), NSWFootnote3, Australia, is selected as the study’s urban context due to its unique and critical climatic conditions. Western Sydney is now developing to accommodate a population increase of 3 million by 2036. Additionally, it is expected to absorb two-thirds of the population growth of the Sydney region (Sokaris, Citation2018). Therefore, urban development in this region requires a sustainable vision and planning process that recognizes challenges at a microclimatic scale. Moreover, the region is 6 to 10 °C hotter than Sydney CBDFootnote4 during extreme heat events (Ogge & Hughes, Citation2018) and the average air temperature has risen more drastically in this region (with around 3.3°C difference) over the past thirty years (Rachwani, Citation2021). This is while uncontrolled sunlight and solar radiation can further exacerbate urban heat within this region. Thus, it is necessary to consider solar geometry in the Western Sydney development scheme.
According to the Western Sydney district plan, Camden, Liverpool, Campbelltown, and Penrith regions are predicted to have the highest housing supply target till 2021, each with 11.800, 8.250, 6.800, and 6.600 housing units, respectively. Moreover, 31,553 new dwellings were completed from 2012 to 2017, of which 28% were located in Camden, 25% in Liverpool, and 20% in Penrith. This is while 77% of these projects were detached dwellings and 23% were multi-unit dwellings. Based on the Greater Sydney Commission report, existing housing typologies in the western city consist of independent houses (81%), medium density housing (11%), and apartments (8%) (Greater Sydney Commission, Citation2018). Therefore, development scenarios based on the urban characteristics of these four suburbs present potential models for solar radiation investigation in the urban context of Western Sydney (). Accordingly, 30% to 53% of the land area is typically dedicated to housing, while commercial use varies between 7% to 37% in the four selected suburbs of Camden, Liverpool, Penrith, and Campbelltown. 3% to 9% of the area is dedicated to open space and greenery, while 10% to 70% of the land is undeveloped. Most of the residential buildings comprise one to two-story housing. However, newly built apartments rise up to 4 to 8 floors. Commercial building height vary between one to two floors (mostly in Camden, Campbelltown, and Penrith) and three in Liverpool. Urban grid orientation also changes between 10 to 15 degrees (from main axis directions) in Liverpool and Penrith and −10 to −35 degrees in Camden and Campbelltown while incorporating 15 m (primary), 10 m (secondary), and 5 m (residential) street widths.
Based on these extracted morphological characteristics, a simplified model is developed to simulate solar conditions in Western Sydney regions. The model incorporates an average of 50%, 30%, and 10% of the land use reserved for the residential sector, commercial uses, and open green spaces, respectively (figures 3 and 4).
3. Methodology
In this study, an optimization driven modeling approach used in similar studies (Jayaweera et al., Citation2021; Shi et al., Citation2017) is employed. This includes the following three steps:
Collecting data (constant and variable design factors),
Generating urban forms (based on the case studies),
Running the optimization process.
The workflow incorporates a generative urban design process that investigates the influential factors affecting daylight level and solar radiation at building facades and urban scales. illustrates this process.
A genetic algorithm-based evolutionary computing approach is thus used to run a multi-objective optimization process that leads to a comprehensive solar-based urban design strategy. The optimum urban configuration introduced in this step is further analyzed through a parametric pilot study to find the impact of individual design factors on different aspects of solar geometry.
Accordingly, models are developed in the Grasshopper plugin, a graphical algorithm editor in Rhinoceros. Grasshopper plugin integrated with Rhinoceros provides the platform to link different plugins to simulate various conditions and parameters (). In this study, the Honeybee tool is employed to calculate the four main objectives: Daylight sufficiency, efficient solar heat gain in summer and winter, and solar energy potentials. This plugin uses validated engines of Radiance (Ward Larson et al., Citation1998), Daysim (Jakubiec & Reinhart, Citation2012), and EnergyPlus for dynamic and static daylight and energy simulations and imports EnergyPlus weather data (EPW) to incorporate the models’ geometry and material features to perform the simulations (Ahmadi, Citation2019).
A five-step process defines the urban model developed to represent a general morphology for western Sydney suburbs. A scaled urban lot (1;8), embraces a 30% mixed-use zone, which is determined by a center point on the lot to consider all the possible locations for this zone (blue colored in ). Main streets will be automatically extracted from the four ends of this zone. The other zones, which are supposed to be dedicated to the residential sector, are divided into four other sub-streets, each by a point on the plots to provide access to the buildings. Further, residential blocks are divided by urban morphology to shape the housing patterns, including four green open spaces. Steps () forming an urban model for computational assessment can be summarized as follows:
The commercial zone is designated using a central point,
Main streets are formed by continuing the four sides of the commercial urban block,
Sub-streets are defined by four selected points on the residential blocks,
Urban blocks are divided by four to provide access to all the housing blocks, resulting in 16 blocks surrounded by residential streets (12 housing blocks are designed, 6 of which are combined in pairs to form larger blocks to consider a wider range of building typologies),
Four green spaces are left open in each block as the house yards.
Subsequently, building heights, ranging from 3 m to 30 m for residential zones and 9 m to 90 m for mixed-use zones, are allocated to the urban model to form the vertical distribution (, right). In other words, the designed urban model embraces mid-rise residential-detached housing and high-rise mixed-use buildings in Western Sydney.
Figure 5. Simplified urban model (phenotypes) and the associated evaluation grid sensors (colored in red).
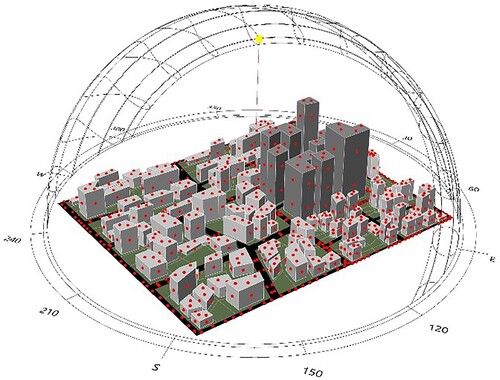
A vertical mesh on the buildings’ facade is defined to calculate both illuminance levels (VDIFootnote5) and solar radiation (kWh) to ensure indoor daylight sufficiency and efficient solar heat gain during extreme weather conditions, respectively. A typical summer and winter day is regarded as the simulation time to consider annual weather conditions. An additional evaluation grid on the street level (at 1.5 m above, equivalent to the height of a pedestrian) is also established to estimate the thermal condition of urban environments. The same indicator of solar radiation (kWh) measures solar energy potentials using an analysis grid on the buildings’ roofs. The other simulation inputs and the optical properties of the construction material are described in .
Table 1. Simulation settings.
Finally, a comprehensive set of Design Factors (DFs) is selected to be explored based on the optimum solar access in both indoor and outdoor built environments. Urban Morphology (UM), floor area ratio (FAR), mean building height (MBH), Urban Grid Rotation (UGR), and Urban surfaces material, including buildings’ facades (BFM) and roofs (BRM) are selected to run a multi-objective solar-based study. defines these influential parameters.
Table 2. Influential design factors.
Ultimately, defined algorithms () trigger Wallacei (Makki et al., Citation2018), an evolutionary analytic engine, to run a multi-objective optimization process to find the most efficient urban configurations with the highest solar performance.
In this study, indoor daylight availability, outdoor thermal condition, and energy generation potential in terms of solar radiation are set as fitness objectives. These goals can be conflicting as lower solar radiation in summer means a lower level of illuminance, which contradicts our objective of maximizing daylight access. A multi-objective optimization is thus required. A Pareto-front problem-solving (Gossard et al., Citation2013; Jayaweera Citation2021; Machairas et al., Citation2014; Shi, Citation2011) method to find the trade-offs between Pareto-optimal (non-dominated) sets of illuminance (VDI) and solar radiation metrics is thus considered during the analysis. The Pareto-front solutions are non-dominant solutions that do not include any other solutions in their subset, and the other dominated solutions are their trade-offs ().
Figure 6. An example of Pareto-frontier based optimization (Image source: (Pilechiha et al., Citation2020)).
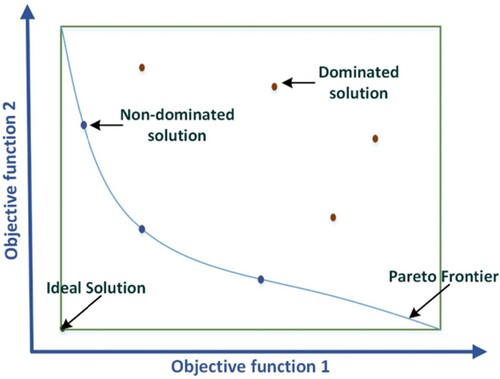
‘The Pareto solutions are accompanied with a vector of an ideal solution and a vector of dominated solutions, determining the upper and the lower bounds of optimal solutions. An ideal or utopia point is a hypothetical concept concerning a perfect target in which each objective is optimized without paying attention to the satisfaction of the others. Multi-objective optimization algorithms attempt to generate solutions close to the Pareto optimal front with a possible uniform distribution. When the non-dominated solutions are identified, decision-makers choose from this set a final resolution according to the particular problem and personal preferences’ (Pilechiha et al., Citation2020).
Wallacei plugin uses NSGA-II (Deb et al., Citation2000), which is known as the most efficient MOEA-based method (Compagnon, Citation2000). This method is also used in similar studies (Martins et al., Citation2014; Navarro-Mateu et al., Citation2018; Pilechiha et al., Citation2020). In the optimization process, feasible (non-dominated) solutions () are generated within a set of circumstances defined by the fitness criteria, followed by optimum solutions representing either the maximum or minimum value of the set objectives (Pilechiha et al., Citation2020).
In this study, phenotypes refer to urban scenarios governed by a gene pool of design factors (DFs) that alter the configurations. The evolutionary algorithm includes 100 generations, each with a population size of 20 individuals, a mutation probability of 25% (equal to 1/n suggested by Deb et al. s. (Deb et al., Citation2000), where n is the number of variables), a crossover rate of 90%, and Elitism size of 50% (fixed by the plugin). Mutation probability is ‘the percentage of mutations taking place in the generation,’ while crossover rate represents ‘the percentage of solutions in the generation that will reproduce for the next generation’ (Makki et al., Citation2018). The last generation is selected to represent the optimal urban configurations as it can provide optimum solutions with multiple design factors rather than offering a singular option. Populationists highlight the significance of variation between design solutions rather than a single average solution since no single solution represents all the characteristics included in the precise mean value for the whole population (Mayr, Citation1994). Accordingly, the last generation represents optimal urban design solutions.
4. Results workflow
The solution selection process is an important step within the evolutionary computing workflow, typically conducted by specialists, designers, and planners. Because, unlike the other optimization approaches, this method offers multiple solutions with respect to different objectives and their priorities for users as illustrated by Wallacei’s analysis graphs (). The three presented standard deviation (SD) graphs (left) are dedicated to the set fitness objectives of FO1. Daylight sufficiency, FO2. Solar energy generation potentials, FO3. Max. solar heat gain in winter, and FO4. Min. Solar heat gain in summer. The red lines indicate the first generations, while the blue lines represent the latter ones. More importantly, the narrower curves indicate less variation in each objective, thus indicating that variables are able to change results slightly. This results in a smoother slope in the mean value trendline ( right), demonstrating a higher average fitness decrease per generation. On the contrary, fitness objectives of indoor and outdoor illuminance comprise higher variations and can be changed by altering urban design factors to a greater extent, including urban pattern, floor area ratio, urban grid rotation, mean buildings’ height, facade, and roof material of residential and mixed-use blocks. As can be seen in , the convergence has been limited in this model owing to conflicting nature of the selected objectives.
To find solutions satisfying the chosen fitness objectives and different aspects of solar geometry, two methods namely:
Average of fitness ranks (FA)
(1)
(1)
Relative Difference (RD) between fitness ranks
(2)
(2)
Xn: Solution’s ranking for specific fitness objective are used to analyze the Pareto front individuals (design solutions with the highest performance) through Parallel Coordinate Plots (PCP) (). Using a line-based representation for each solution, this chart shows the performance of all design alternatives (for the four fitness objectives of daylight sufficiency, solar energy potentials, and solar heat gain in winter and summer). The black line indicates the optimum solution found using FA (top) and RD (bottom) methods. The first strategy (FA) provides the possibility to find the extreme individuals that are specialized based on one fitness objective, while in the other strategy (RD) individuals tend to find an equilibrium between all fitness objectives (Navarro-Mateu et al., Citation2018). Accordingly, the best design solutions (top-ranked individuals) for both FA (Gen.87, Indv.15) and RD (Gen.21, Indv.19) are shown in (indicated by black lines).
Figure 9. Parallel Coordinate Plots of the top-ranked individual for Fitness Average (top) and Relative Difference (bottom).
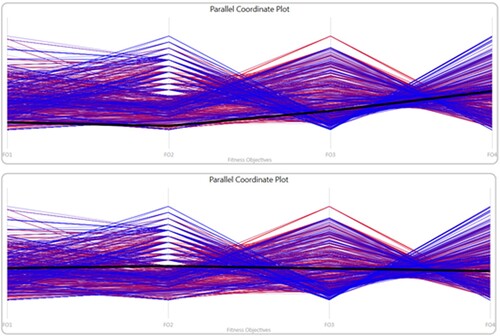
shows the urban layouts that are selected based on these two methods of RD and FA, based on which the UGRs of 172.8 and 21.67 degrees, FARs of 0.88 and 0.41, MBH(rz) of 15.88 and 16.94 m, MBH(muz) of 41.78 and 52.28 m, FM(rz) of 0.80 and 0.56, FM(muz) of 0.61 and 0.89, and RM of 0.50 and 0.37 are suggested. Additionally, a difference of 50.58, 50.53, 19.51, and 9.18% is reported between the results obtained from objectives of FO1, FO2, FO3, and FO4 using the two methods of RD and FA, respectively. Therefore, FA suggests a more efficient solution with higher solar performance in winter while only worsening thermal conditions by 9.18%, compared to the model selected by RD, which seems to be neglectable due to other solar benefits it offers.
Table 3. Multi-responsive design scenarios using RD and FA methods and the utopia solution with their related genes, layouts, values, and chromosomes (from top to bottom).
The other important individual solution that needs to be analyzed is the Utopia solution, which has the nearest proximity to the Ideal Solution (that doesn’t exist) () with high performance rating for all fitness objectives. Solution Gen.16, Indv.19 represents the Utopia solution and embraces the chromosome feature stated above (). ()
Figure 10. Parallel coordinate plot (top) and unsupervised machine learning algorithm (bottom) of the Pareto Front solutions.
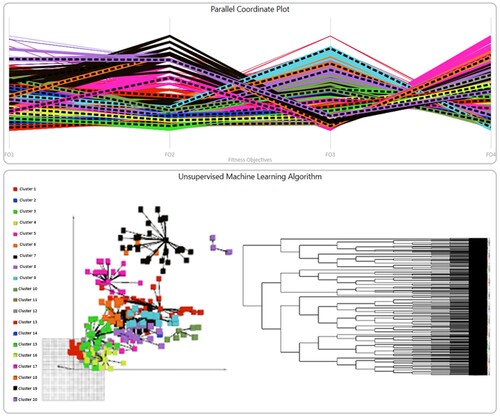
According to the results of the evolutionary computing, 293 Pareto front solutions are found among the selected population of 2000 individuals. Hence, these individuals are clustered into 20 groups to find the Utopia solutions. The centre of each cluster is further selected as a representative of the whole cluster. Thus, the 293 individuals are shrunk into 20 solutions. illustrates the clustering process of Pareto Front solutions.
indicates that cluster 11 (C11) includes the closest individuals to the Utopia point. Comparing the fitness objective values of the selected solution through FA and clustering analysis methods, a lower difference between solar heat gain in summer and winter is observed in the prior method (FA), and thus this solution (Gen.21, Inv.19), included in the cluster 15, offers a more balanced SHG. This is while a relatively slight difference exists between the other objectives of daylight sufficiency and solar energy potentials.
The outliers, which are solutions with the highest value in only one of the objectives, can also be seen and extracted from the clusters in .
As the three objectives of daylight availability (FO1), solar access on buildings’ roof (FO2), and maximum solar heat gain in winter (FO3) outweigh the FO4 of minimum solar heat gain in summer, the solution chosen by the FA method (Gen.21 Indv.19) is considered as the optimum solution ().
4.1. Parametric study
In this section, a pilot parametric study, as an addition and a benefit to the employed GA method, is conducted to allow the solution to improve, which could have occure if more time and generation allocation were given to the evolutionary algorithm. As in the evolutionary computation all the design variables change simultaniously to form different generation, it is difficult to extract the impact size of different variables (design factors here). Therefore, this part of the study tries to improve the performance of the final solution extracted from the evolutionary computing process and, more specifically, to find the extent to which each design factor can change different solar performance-related results. Accordingly, in the parametric study, the minimum and maximum thresholds of the range considered for design parameters in the EAFootnote6 process are selected as the variables () to see to what extent altering these values to the extreme ones can change the objectives. In this way, the impact size can be extracted by comparing the solar performance of the best case and the worst case scenario.
Table 4. Variable range of design parameters.
Covering all the solar objectives (FO1, FO2, FO3, FO4) an average value is extracted to show case the importance of different design factors. Accordingly, among the selected urban design parameters, urban morphology plays the most crucial role, with an average effect size of 43.26% (regarding all the solar aspects). This means that if the location of the mixed-use zone (defining the urban morphology here) changes from the optimum place (UP(x):0.21, UP(y):0.38) to the worst location (UP(x):0.01, UP(y):0.01) solar performance can reduce by up to 43.26%. The floor area ratio assumes the second priority with a value of 37.94%. illustrates the impact size of different design alternatives on all four objectives of daylight sufficiency (FO1), solar energy potentials (FO2), and solar heat gain in winter (FO3) and summer (FO4). Accordingly, urban morphology (defined by the location of the mixed-use zone) has the highest impact, followed by FAR, UGR (15.58%), MBH (8.20%), FM (3.74%), and RM (0.21%).
Table 5. Impact size of design parameters on different objectives
The performance of the final solution obtained from the previous step (GA method) can be improved taking the simulations further and narrowing the range considered for design factors. shows the improved solution and its associated design factors and objective values. Results indicate that FAR and MBH(mixed-use) values should be further reduced, while MBH(residential), facade and roof material reflectivity should be increased.
5. Discussion
This study sought to optimize urban scenarios for enhanced daylight access and solar radiation utilization through the application of evolutionary computation. Due to the time limitation and to make sure if the GA algorithm had enough chance to converge fully and provide the most optimum solution, a parametric approach was employed. This method also helped to extract the impact size of different design factors. Since parametric approach allows designer and analysts to control variables manually, compared to the automatically alteration in the evolutionary computation. In fact, parametric experience used in this study is a benefit to the GA method, which provides the opportunity for architects, stackholders, and designers to opt between the solar objectives and choose which one is in the priority to select the optimum value for design parameters. In ther words, if daylight (FO1) is a more important factor compared to the other three solar objectives, the most important design factor to consider would be Floor Area Ratio with an impact size of 49.38% (using ). This approch gives the designer the freedom to prioritize design factors along with other parameters.
The conducted study examines a hypothetical design model derived after studying the grain of urban fabric in Western Sydney suburbs. This included simplification of building geometry to decrease the computational simulation time. However, the inclusion of realistic urban block conditions, incorporating elements such as tree canopies and other urban obstructions into the simulation process, will certainly prove crucial for developing an accurate representation of the urban environment. Besides this, a key recommendation for simulating solar access inside buildings should involve the inclusion of detailed building-scale factors, such as room width and material properties. Furthermore, to ensure the validity and applicability of the simulation results, the study advocates for the use of real-time on-site measurements (of solar-related parameters icluding, daylight level, thermal comfort, energy generation potentials), which will bridge the gap between theoretical models and practical applications, enhancing the reliability of the findings.
The presented methodology can be integrated into the workflows of designers, architects, and urban planners alike, thus aiding in multiscalar analysis and refinement of the urban and architectural fabric. By doing so, it will be possible to create urban environments that maintain acceptable levels of daylight and solar radiation throughout the year, thereby improving thermal comfort and energy efficiency in urban settings. This framework offers a robust foundation for future urban design strategies aimed at sustainability and resilience in rapidly growing regions like Western Sydney.
6. Conclusion
Western Sydney is increasingly absorbing more population, and the climatic crisis it is experiencing needs special consideration. One of the attempts could be to improve its urban contexts by employing the optimum design scenarios on both urban and building scales. One of the important aspects of an environmentally concentrated design is solar geometry, whether it's about solar thermal comfort or daylight availability. Therefore, this study tries to provide suggestions on design factors affecting this parameter (solar geometry) to make the most of its benefit and eliminate its adverse impacts on occupants of both indoor (building) and outdoor (urban) spaces. This study uses two processes, evolutionary computing and parametric optimization, to investigate urban layouts that are driven by solar conditions, including daylight availability, energy generation potentials, and heat gain at extreme times of the year.
Accordingly, a multi-objective model is developed, and the solar performance of different alternatives is evaluated (using evolutionary computation) and improved further by running a manually controlled parametric optimization. The results of the analysis indicate that a lower floor area ratio (FAR) and mean building height (MBH) and a higher reflectivity for both the facade and roof are preferable in terms of solar access. Although the design scenario presented by the GA method is an optimum solution addressing all the set objectives, it has the potential to be improved based on the designer’s decision to modify one or two objectives of low priority depending on contextual, economic, or speed of actual implementation needs. It is thus suggested that the extraction of the impact factor of each design parameter (as opposed to only running a multi-objective optimization process such as what is suggested in ) will aid in further refinement and fine-tuning of the optimized solar geometry driven design.
Disclosure statement
No potential conflict of interest was reported by the author(s).
Notes
1 Spatial daylight autonomy
2 "the distance between buildings and the surface equivalent albedo"
3 New South Wales
4 Central Business District
5 Vertical Daylight Illuminance
6 Evolutionary Algorithm
7 Light Reflectance Value
8 Visula Light Transmittance
9 Boundry box of the building blocks is considered for the simulation and the study does not go further into details due to the scale of the research, which is more focused on urban analysis.
References
- Ahmadi, S. (2019). An analysis of urban form and canyon for performative daylighting design [Doctoral Dissertation]. University of Washington.
- Andersen, M., Mardaljevic, J., & Lockley, S. W. (2012). A framework for predicting the non-visual effects of daylight–Part I: photobiology-based model. Lighting Research & Technology, 44(1), 37–53. https://doi.org/10.1177/1477153511435961
- Biloria, N., Makki, M., & Abdollahzadeh, N. (2023). Multi-performative façade systems: The case of real-time adaptive BIPV shading systems to enhance energy generation potential and visual comfort. Frontiers in Built Environment, 9, 1–14. https://doi.org/10.3389/fbuil.2023.1119696
- Bouyer, J., Inard, C., & Musy, M. (2011). Microclimatic coupling as a solution to improve building energy simulation in an urban context. Energy and Buildings, 43(7), 1549–1559. https://doi.org/10.1016/j.enbuild.2011.02.010
- Chan, E. H. W., Lam, K. S., & Wong, W. S. (2008). Evaluation on indoor environment quality of dense urban residential buildings. Journal of Facilities Management, 6(4), 245–265. https://doi.org/10.1108/14725960810908127
- Chatzipoulka, C., Compagnon, R., & Nikolopoulou, M. (2016). Urban geometry and solar availability on façades and ground of real urban forms: using London as a case study. Solar Energy, 138, 53–66. https://doi.org/10.1016/j.solener.2016.09.005
- Cheng, V., Steemers, K., Montavon, M., & Compagnon, R. (2006a). Compact cities in a sustainable manner, 44, 1–11.
- Cheng, V., Steemers, K., Montavon, M., & Compagnon, R. (2006b). Urban form, density and solar potential.
- Compagnon, R. (2000). Precis: assessing the potential for renewable energy in cities, annexe3: Solar and daylight availability in urban areas. Univ. Appl. Sci. West. Switz. (HES-SO). Fribg.
- Compagnon, R. (2004). Solar and daylight availability in the urban fabric. Energy and Buildings, 36(4), 321–328. https://doi.org/10.1016/j.enbuild.2004.01.009
- D.Colton. (2013). Solarindustrymag Corporation.
- Deb, K., Agrawal, S., Pratap, A., & Meyarivan, T. (2000). A fast elitist non-dominated sorting genetic algorithm for multi-objective optimization: NSGA-II. In International Conference on Parallel Problem Solving from Nature (pp. 849–858). Springer.
- Giridharan, R., Ganesan, S., & Lau, S. S. Y. (2004). Daytime urban heat island effect in high-rise and high-density residential developments in Hong Kong. Energy and Buildings, 36(6), 525–534. https://doi.org/10.1016/j.enbuild.2003.12.016
- Gossard, D., Lartigue, B., & Thellier, F. (2013). Multi-objective optimization of a building envelope for thermal performance using genetic algorithms and artificial neural network. Energy and Buildings, 67, 253–260. https://doi.org/10.1016/j.enbuild.2013.08.026
- Greater Sydney Commission. (2018). Western City District Plan 135.
- Hofierka, J., & Kaňuk, J. (2009). Assessment of photovoltaic potential in urban areas using open-source solar radiation tools. Renewable Energy, 34(10), 2206–2214. https://doi.org/10.1016/j.renene.2009.02.021
- Jakubiec, J. A., & Reinhart, C. F. (2012). “Overview and Introduction to DAYSIM and Current Research Developments,” [Online].
- Jayaweera, N., Rajapaksha, U., & Manthilake, I. (2021). A parametric approach to optimize solar access for energy efficiency in high-rise residential buildings in dense urban tropics. Solar Energy, 220, 187–203. https://doi.org/10.1016/j.solener.2021.02.054
- Kämpf, J. H., Montavon, M., Bunyesc, J., Bolliger, R., & Robinson, D. (2010). Optimisation of buildings’ solar irradiation availability. Solar Energy, 84(4), 596–603. https://doi.org/10.1016/j.solener.2009.07.013
- Li, D. H. W., & Wong, S. L. (2007). Daylighting and energy implications due to shading effects from nearby buildings. Applied Energy, 84(12), 1199–1209. https://doi.org/10.1016/j.apenergy.2007.04.005
- Lima, I., Scalco, V., & Lamberts, R. (2019). Estimating the impact of urban densification on high-rise office building cooling loads in a hot and humid climate. Energy and Buildings, 182, 30–44. https://doi.org/10.1016/j.enbuild.2018.10.019
- Machairas, V., Tsangrassoulis, A., & Axarli, K. (2014). Algorithms for optimization of building design: A review. Renewable and Sustainable Energy Reviews, 31, 101–112.
- Makki, M., Showkatbakhsh, M., & Song, Y. (2018). Wallacei, An evolutionary and analytic engine for Grasshopper 3D, Primer 2.0,[Online].
- Martins, T. A. d. L., Adolphe, L., Bastos, L. E. G., & Martins, M. A. d. L. (2016). Sensitivity analysis of urban morphology factors regarding solar energy potential of buildings in a Brazilian tropical context. Solar Energy, 137, 11–24. https://doi.org/10.1016/j.solener.2016.07.053
- Martins, T. A. L., Adolphe, L., & E.g. Bastos, L. (2014). From solar constraints to urban design opportunities: Optimization of built form typologies in a Brazilian tropical city. Energy and Buildings, 76, 43–56. https://doi.org/10.1016/j.enbuild.2014.02.056
- Mayr, E. (1994). Typological versus population thinking. In E. Sober (Ed.), Conceptual issues in evolutionary biology (pp. 157–160). The Mit Press.
- Natanian, J., Aleksandrowicz, O., & Auer, T. (2019). A parametric approach to optimizing urban form, energy balance and environmental quality: The case of Mediterranean districts. Applied Energy, 254, 113637. https://doi.org/10.1016/j.apenergy.2019.113637
- Navarro-Mateu, D., Makki, M., & Cocho-Bermejo, A. (2018). Urban-tissue optimization through evolutionary computation. Mathematics, 6(10), 189. https://doi.org/10.3390/math6100189
- Ng, E. (2003). Studies on daylight design and regulation of high-density residential housing in Hong Kong. Lighting Research & Technology, 35, 127–139.
- Ogge, M., & Hughes, T. (2018). Heatwatch: extreme heat in Western Sydney. Aust. Inst, 1–19.
- Pilechiha, P., Mahdavinejad, M., Pour Rahimian, F., Carnemolla, P., & Seyedzadeh, S. (2020). Multi-objective optimisation framework for designing office windows: quality of view, daylight and energy efficiency. Applied Energy, 261, 114356. https://doi.org/10.1016/j.apenergy.2019.114356
- Rachwani, M. (2021). “Extremely dangerous”: how much of the heat can western Sydney bear? [WWW Document]. Guard. URL https://www.theguardian.com/australia-news/2021/mar/08/extremely-dangerous-how-much-of-the-heat-can-western-sydney-bear (accessed 4.11.21)
- Ratti, C., Baker, N., & Steemers, K. (2005). Energy consumption and urban texture. Energy and Buildings, 37(7), 762–776. https://doi.org/10.1016/j.enbuild.2004.10.010
- Reardon, C., & Downton, P. (2008). Passive Design: 4.2 Design for climate. Your Home Tech. Manual, Dep. Environ. Water, Herit. Arts. Aust. Gov.
- Saratsis, E., Dogan, T., & Reinhart, C. F. (2017). Simulation-based daylighting analysis procedure for developing urban zoning rules. Building Research & Information, 45(5), 478–491. https://doi.org/10.1080/09613218.2016.1159850
- Sarralde, J. J., Quinn, D. J., Wiesmann, D., & Steemers, K. (2015). Solar energy and urban morphology: Scenarios for increasing the renewable energy potential of neighbourhoods in London. Renewable Energy, 73, 10–17. https://doi.org/10.1016/j.renene.2014.06.028
- Shi, X. (2011). Design optimization of insulation usage and space conditioning load using energy simulation and genetic algorithm. Energy, 36(3), 1659–1667. https://doi.org/10.1016/j.energy.2010.12.064
- Shi, Z., Fonseca, J. A., & Schlueter, A. (2017). A review of simulation-based urban form generation and optimization for energy-driven urban design. Building and Environment, 121, 119–129. https://doi.org/10.1016/j.buildenv.2017.05.006
- Sokaris, C. (2018). 2019 Outlook, Creating opportunities, WESTERN SYDNEY, AUSTRALIA’S THIRD LARGEST ECONOMY [WWW Document]. Natl. Aust. Bank. URL https://business.nab.com.au/western-sydney-australias-third-largest-economy-32773/ (accessed 4.11.21)
- Strømann-Andersen, J., & Sattrup, P. A. (2011). The urban canyon and building energy use: Urban density versus daylight and passive solar gains. Energy and Buildings, 43(8), 2011–2020.
- Van Esch, M. M. E., Looman, R. H. J., & de Bruin-Hordijk, G. J. (2012). The effects of urban and building design parameters on solar access to the urban canyon and the potential for direct passive solar heating strategies. Energy and Buildings, 47, 189–200. https://doi.org/10.1016/j.enbuild.2011.11.042
- Vartholomaios, A. (2017). A parametric sensitivity analysis of the influence of urban form on domestic energy consumption for heating and cooling in a Mediterranean city. Sustainable Cities and Society, 28, 135–145. https://doi.org/10.1016/j.scs.2016.09.006
- Vermeulen, T., Merino, L., Knopf-Lenoir, C., Villon, P., & Beckers, B. (2018). Periodic urban models for optimization of passive solar irradiation. Solar Energy, 162, 67–77. https://doi.org/10.1016/j.solener.2018.01.014
- Ward Larson, G., Shakespeare, R., Mardaljevic, J., & Ehrlich, C. (1998). Rendering with Radiance : A Practical Tool for Global Illumination. p. 129.