Abstract
The demographic dynamics are known to drive the disease dynamics in constant environments. In periodic environments, we prove that the demographic dynamics do not always drive the disease dynamics. We exhibit a chaotic attractor in an SIS epidemic model, where the demograhic dynamics are asymptotically cyclic. Periodically forced SIS epidemic models are known to exhibit multiple attractors. We prove that the basins of attraction of these coexisting attractors have infinitely many components.
1. Introduction
The role of periodic environments in determining the long-term dynamics of populations has become an area of intensive study in both ecological and epidemiological research Citation1–27. In a recent paper, Franke and Yakubu studied the impact of seasonal factors on a discrete-time SIS (susceptible–infected–susceptible) epidemic model Citation13. For the periodically forced SIS model, Franke and Yakubu, computed the epidemic threshold parameter, ℛ0, and used it to prove that if then the disease goes extinct whereas if
then the disease is endemic and may even be cyclic. In addition, Franke and Yakubu, used simulations to show that in periodic environments, it is possible for the infective population to be on a chaotic attractor while the demographic dynamics is non-chaotic Citation13. For certain parameter values, the SIS model of Franke and Yakubu, a periodically forced hierarchical model, has multiple attractors when
. What is the nature and structure of the basins of attraction of these coexisting attractors?
In this paper, we focus on deriving verifiable conditions that guarantee the existence of cyclic or chaotic attractors in periodically forced hierarchical models. When the periodically forced SIS model exhibits multiple compact attractors, we prove that at least one of the basins of attraction of the coexisting attractors has infinitely many components. That is, it is almost impossible to accurately specify all the initial conditions that lead to each of the coexisting attractors. This ‘uncertainty’ phenomenon is known to occur in deterministic models that exhibit sensitive dependence on initial conditions Citation28–34.
The paper is organized as follows. In section 2, we introduce the periodically forced SIS model of Franke and Yakubu. We review, in section 3, the results of Franke and Selgrade on ‘time-dependent’ versus ‘time-independent’ dynamical systems. In section 4, we use a general non-autonomous hierarchical model to derive conditions for the existence of cyclic or chaotic attractors. The periodically forced SIS model of Franke and Yakubu fits into our hierarchical framework. Illustrative examples of cyclic and chaotic dynamics in SIS models are provided in section 5. In these examples, the SIS epidemic model is under asymptotically cyclic demographic dynamics and infections are modeled as Poisson processes Citation13, Citation28–30, 35, 36. Section 6 is on the basins of attraction of multiple (coexisting) compact attractors. Illustrative examples of cyclic attractors with basins that have infinitely many components are demonstrated in section 7, and concluding remarks are presented in section 8.
2. SIS epidemic model in periodic environments
In this section, we introduce the main model, the periodically forced SIS epidemic model of Franke and Yakubu Citation13. To do this, we first assume that the dynamics of the total population size in generation t, denoted by N(t), are governed by the p-periodic demographic equation
Theorem 1
[Citation12,Citation13] Model
Equation(1)
with
has a globally attracting positive s-periodic cycle that starts at
Theorem 2
[Citation12
Citation13] Model
Equation(1)
with
and μ>1 has a globally attracting positive s-cycle, where s divides p.
By these two results, the total population is asymptotically periodic (bounded) and lives on a cyclic attractor, denoted by , when the recruitment function is either a periodic constant or the Beverton–Holt model.
Next, we build a simple SIS epidemic process on ‘top’ of the periodic demographic equation, equation Equation(1). As in Citation13, Citation28–30, we let S(t) denote the population of susceptibles; I(t) denote the population of the infected, assumed infectious;
denote the total population size at generation t, N
∞ denote the demographic steady state or attracting population and
the initial point on a globally attracting cycle, when they exist. We assume that individuals survive with constant probability γ each generation, and infected individuals recover with constant probability (1−σ).
Let be a monotone convex probability function with
and
for all
We assume that the susceptible individuals become infected with nonlinear probability
per generation, where the transmission constant α>0. When infections are modeled as Poisson processes, then
Citation13, Citation28–30, 35, 36.
Our assumptions and notation lead to the following SIS epidemic model in p-periodic environments:
Using the substitution , the I-equation in Model Equation(2)
becomes
Franke and Yakubu, used the map F
N
to study disease dynamics in the periodic SIS epidemic model, Model Equation(2). In particular, they obtained the basic reproduction number,
Lemma 3
-
(a)
and
-
(b) F N (I) is concave down on [0, N].
-
(c)
on [0, N].
-
(d) If
then F N has a unique positive fixed point I N in [0, N].
-
(e) Let
Then
. That is, Ψ N is a topological conjugacy between F 1 and F N .
-
(f) If N 0<N 1 and
, then
where
is the positive fixed point of
in [0, N i ].
-
(g) If C 1 exists, then C N =NC 1.
-
(h) If N 0<N 1, then
for all I∈(0, N 0].
3. Review of time-periodic dynamical systems
Our periodically forced SIS epidemic model is a time-periodic dynamical system. To study the attractors generated by the model when , we use a very general time-independent discrete-time dynamical system to motivate definitions of attractors for a time-periodic dynamical system. In Citation9, Franke and Selgrade showed that the classical definitions from time-independent discrete dynamical systems theory applied to autonomous systems lead to important new concepts for the corresponding time-periodic dynamical system.
As in Citation9, let (X, d) be a metric space (usually an open subset of ℝ
n
). A discrete
\ dynamical system is a finite sequence
of maps where
for
. Extend this sequence to a periodic infinite sequence by defining
for i≥p. The trajectory {x(t)} of a point x∈X is given by the t-fold composition of these p maps. That is,
Let
𝒳 consists of p copies of X referred to as
fibers (see ). Open sets in 𝒳 are open sets in each copy of X. For each
Figure 1. The fibered cylinder 𝒳 and the cylinder map ℱ corresponding to the dynamical system {F 0, F 1, …, F p−1}.
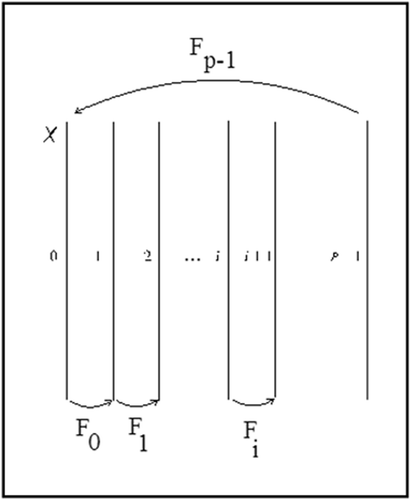
ℱ is an autonomous dynamical system on 𝒳, and the standard definitions for an invariant set, attractor and ω -limits apply. In Citation9, Franke and Selgrade introduced similar concepts for time-periodic dynamical systems.
As in Citation9, define the projection by
Definition
A set is invariant under the time-periodic dynamical system if there is a set
with
and
Citation9.
Trapping regions play an important role in understanding the long-term dynamics of many systems.
Definition
A set U⊂X is a trapping region for the time-periodic dynamical system if there is an open set with compact closure
so that
and
Citation9. 𝒰 is called a corresponding trapping region to U.
a nonempty compact invariant set, is an attractor for ℱ whenever 𝒰 is a trapping region. We capture this in the following precise definition.
Definition
A set is an attractor for the time-periodic dynamical system if it has a trapping region U, with corresponding trapping region
, such that
where
Citation17.
By these definitions, an attractor Γ in 𝒳 produces an attractor Λ in X for the time-periodic dynamical system.
4. Compact attractors
To study the nature and structure of compact attractors in our SIS epidemic model, we assume that the p-periodic demographic equation (equation Equation(1)) has a globally attracting positive cycle
. Recall that, when the recruitment function is either periodically constant or periodic Beverton–Holt, the demographic equation is asymptotically cyclic (Theorems 1 and 2). If in addition
Franke and Yakubu showed that it is possible for the uniformly persistent epidemic to live on a cyclic or chaotic attractor.
To understand compact attractors for our epidemic process, we consider the following general hierarchical system.
In our SIS epidemic model, let
Assume throughout this section that is a globally attracting p-periodic orbit for the p-periodic dynamical system g(t,_), where each x
i
is unique. Let
Let
Next, we obtain that the p-periodic dynamical system, G, has an attractor whenever the one dimensional map H has one, and vice versa.
Theorem 4
The p-periodic dynamical system
on V has a compact attractor if and only if
has a compact attractor.
Proof
Let A be a compact attractor for the p-periodic dynamical system on V and
be the globally attracting p-periodic orbit for the g(t,_) p-periodic dynamical system. Then, in the fiber cylinder there is a compact attractor ˜A which projects onto A. Let U be a compact trapping neighborhood of ˜A whose image under the fiber map 𝒢 is in its interior. 𝒢
p
maps the 0th fiber into itself. In the x variable, this mapping has x
0 as a globally attracting fixed point. The projection of the part of U in the 0th fiber onto the first coordinate produces a compact neighborhood U
x
of x
0. Since x
0 is a globally attracting fixed point,
The above proof gives a relationship between the structure of attractors for G and H. We capture this relationship in the following two corollaries.
Corollary 5
If the p-periodic dynamical system
on V has a compact attractor A, then
has a compact attractor B and A=
Corollary 6
If
has a compact attractor B, then the p-periodic dynamical system
on V has A=
The cardinality of attractor A is p times the cardinality of attractor B. Hence, by Theorem 4 the following result is immediate.
Corollary 7
The one parameter family of p-periodic dynamical systems
Chaotic attractors have-positive Lyapunov exponents Citation39. Next, we obtain that the attractor for the p-periodic dynamical system, G, is chaotic whenever that of the one-dimensional map H is chaotic, and vice versa.
Theorem 8
If
has a compact attractor B with a positive Lyapunov exponent then G has a compact attractor A with a positive Lyapunov exponent.
Proof
By Corollary 6, the compact attractor B for H corresponds to a compact attractor A for G. Under G iterations, the first coordinate has a globally attracting periodic orbit. Hence, the first coordinate cannot produce a positive Lyapunov exponent. Under G iterations, the second coordinate on A corresponds exactly to that of H on B. Thus, if has a compact attractor B with a positive Lyapunov exponent then G has a compact attractor with a positive Lyapunov exponent. ▪
5. Illustrative examples: cyclic and chaotic attractors
In this section, we use a specific example to illustrate the predicted cyclic and chaotic attractors in our SIS epidemic model by Corollary 6 and Theorem 7, where the demographic dynamics is cyclic and non-chaotic. In this example, we consider our epidemic model with periodic constant recruitment function, where infections are modeled as Poisson processes.
Example 9
Consider Model Equation(4) with 2-periodic constant recruitment function
With our choice of parameters, the 2-periodic demographic equation has a globally attracting 2-cycle (Theorem 1). shows period-doubling bifurcation route to chaos in the infective population (H dynamics) as the transmission constant α is varied between 0 and 400. By Corollary 6, the corresponding SIS epidemic model undergoes period doubling bifurcation route to chaos, where the demographic dynamics is non-chaotic.
Figure 2. Period-doubling bifurcation route to chaos in the infective population. On the horizontal axis, 0≤α≤400 and on the vertical axis, 0≤I≤160.
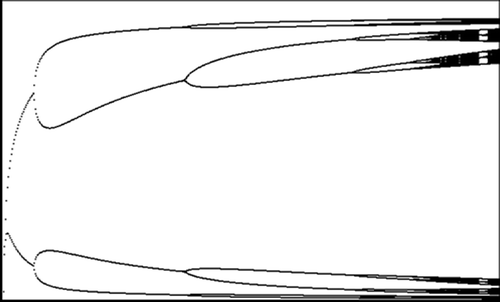
shows an attracting 24-cycle in the infective population (H dynamics). For this choice of parameters, Corollary 7 and Sharkovskii's Theorem guarantee a chaotic attractor (Li–Yorke type Citation39) in the corresponding epidemic model.
Figure 3. A period-24 cycle in the infective population. On the horizontal axis, 300≤α≤400 and on the vertical axis, 120≤I≤160.
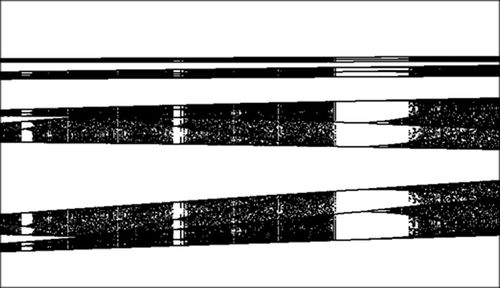
By Corollary 7 and Theorem 8, the general pattern illustrated in and are not restricted to our choice of the periodic constant recruitment function, but also follows when the periodic Beverton–Holt and Ricker models are used Citation2, 12, 13, 24, Citation27–34, 40.
6. Multiple attractors
Franke and Yakubu showed that Model Equation(2) is capable of exhibiting multiple (coexisting) compact attractors when the critical point of F
1, C
1, is less than the fixed point of F
1 (
). In this section, we study the structure of the basins of attraction of these coexisting attractors.
Throughout this section,
Next, we obtain a closed interval on which our composition map,
Theorem 10
If C
1
is less than the positive fixed point of F
1
and
is an orbit of the p-periodic dynamical system
Proof
Since is an orbit of the p-periodic dynamical system
In Corollary 11 and Lemma 12, we obtain regions on which the composition map
Corollary 11
If C 1 is less than the positive fixed point of F 1, then
The proof of Corollary 11 is contained in that of Theorem 10.
Lemma 12
If C 1 is less than the positive fixed point of F 1, then there is an L>0 such that
Proof
From the proof of Theorem 10, so
moves the origin to a point of the positive x-axis. Since this axis is invariant,
Next, we establish that in our epidemic model, Model Equation(4), the point
is not in the basin of attraction of any set of coexisting attractors.
Lemma 13
If Model
Equation(4)
has multiple disjoint compact attractors and C
1
is less than the positive fixed point of F
1, then the point
is not in the basin of attraction of these multiple attractors.
Proof
{0} is a repelling fixed point of . By Theorem 10, any neighborhood of {0} eventually gets mapped onto the entire range. Hence, every neighborhood of {0} contains points of all basins of attraction. Thus
cannot be in any basin of attraction of Model Equation(4)
. ▪
In Citation11, Franke and Yakubu obtained that periodically forced models can exhibit multiple (coexisting) attractors via cusp bifurcations, where the corresponding unforced models exhibit no multiple attractors. When our SIS epidemic model has coexisting attractors, then at least one of the basins of these attractors has infinitely many components. That is, the basins of attraction are in the cylinder space and a component of the basins is a subset of one of the fibers. We capture this in the following result.
Theorem 14
If Model\/
Equation(4)
has multiple disjoint compact attractors and C
1
is less than the positive fixed point of F
1, then at least one of the basins of attraction has infinitely many components.
Proof
We will show that the 0th fiber contains infinitely many components. By Corollary 5, to each attractor A
i
of Model Equation(4) there is a corresponding attractor B
i
of the composition map
7. Illustrative examples: multiple attractors
Here, we use a specific example to demonstrate coexisting attractors with basins of attraction having infinitely many components. As in Example 9, we consider Model Equation(4) with periodic constant recruitment function, where infections are modeled as Poisson processes.
Example 15
Consider Model Equation(4) with 4 -
constant recruitment function
With our choice of parameters, the 4-periodic demographic equation has a globally attracting 4-cycle (Theorem 1). shows that Example 15 has two coexisting 4-cycle attractors (multiple attractors) at
Figure 4. Two coexisting 4-cycle attractors. On the horizaontal axis, 0≤N≤175, and on the vertical axis, 0≤I≤75.
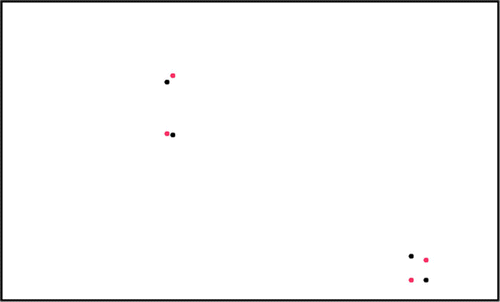
show the basins of attraction of the two coexisting 4-cycle attractors in Example 15 (or ), where the red and blue regions are, respectively, the basins of attraction of the 4-cycle attractors R and B.
Figure 5. Basins of the two coexisting 4-cycle attractors in Example 15, where the red and blue regions are, respectively, the basins of attraction of attractors R and B. On the horizaontal axis, 0≤N≤100, and on the vertical axis, 0≤I≤100.
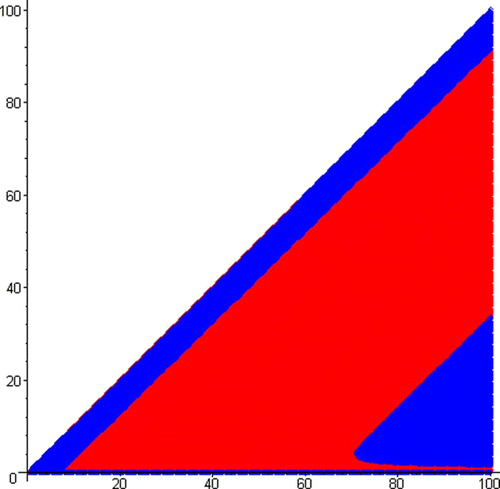
Figure 6. Zoom of around the origin by a factor of 1000, where the red and blue regions are, respectively, the basins of attraction of attractors R and B. On the horizaontal axis, 0≤N≤0.1, and on the vertical axis, 0≤I≤0.1.
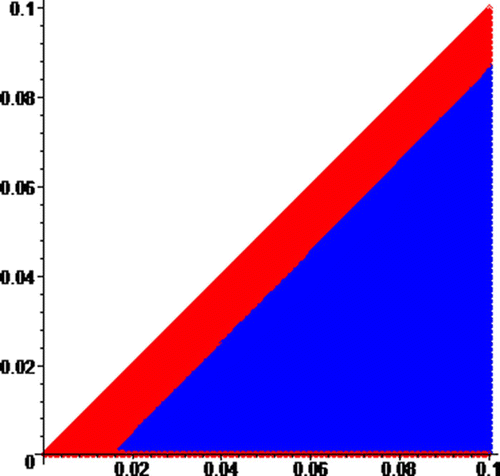
To demonstrate that the basins of the attractors have infinitely many components, we zoom into the origin of by a factor 1000 to obtain . Similarly, we obtain by zooming into the origin of by a factor of 1000. As predicted by Theorem 14, our sequence of zooms produces pictures with the colors switching back and forth. The edge of the diagonal changes color back and forth as you zoom into the origin.
Figure 7. Zoom of around the origin by a factor of 1000, where the red and blue regions are, respectively, the basins of attraction of attractors R and B. On the horizaontal axis, 0≤N≤0.0001, and on the vertical axis, 0≤I≤0.0001.
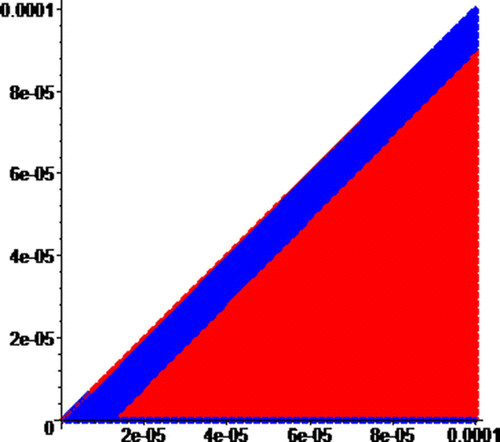
, a zoom of away from the origin, shows that the blue basin of attraction has an end; making the red basin a connected set.
Figure 8. Blue basin ends and red basin is connected. On the horizaontal axis, 2000≤N≤2600, and on the vertical axis, 2000≤I≤2600.
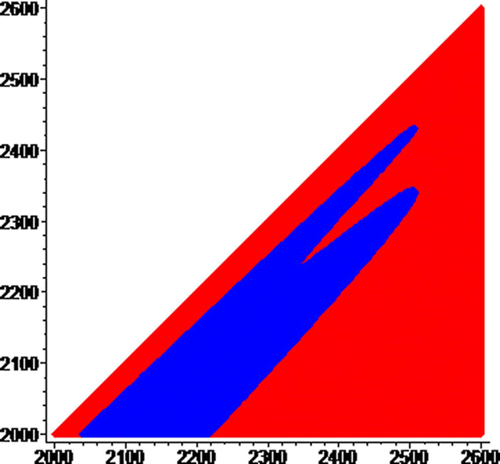
As illustrated by Theorem 14, the general pattern illustrated in are not restricted to our choice of the periodic constant recruitment function, but also follows when the periodic Beverton–Holt model is used, and certainly hold for any increasing homeomorphism with a globally attracting positive periodic orbit.
8. Conclusion
The periodically forced SIS model of Franke and Yakubu has illustrated several important principles, both concerning the role of periodic environments, and the complexity of the interaction between infectives and susceptibles in discrete-time models Citation2, Citation12–14, 25, 26, Citation41–43.
Castillo-Chavez and Yakubu obtained that in constant environments the demographic equation drives the disease dynamics Citation28–30. That is, when the demographic dynamics are cyclic and non-chaotic, then the disease dynamics are cycle and non-chaotic. Similarly, when the demographic dynamics are chaotic, then the disease dynamics are chaotic. In the current paper, we prove that in periodic environments it is possible for the infective population to be on a chaotic attractor while the demographic dynamics are cyclic and nonchaotic. That is, in periodic environments, the demographic dynamics do not drive the disease dynamics Citation13.
In constant environments, simple SIS models do not exhibit multiple attractors Citation1, 2, 12, 13, Citation28–30, 35, 36, 39. However, in periodic environments the corresponding simple models can have multiple attractors with basins of attraction having infinitely many components. In this situation, it is impossible to make accurate predictions of the final outcome of all initial population sizes despite the fact that and the disease is endemic Citation13. This extreme dependence of the long-term behavior on initial population sizes may have serious implications on the persistence and control of infectious diseases.
References
- Anderson , R. M. and May , R. M. 1992 . Infectious Diseases of Humans: Dynamics and Control , Oxford : Oxford University Press .
- Bailey , N. T.J. 1975 . The Mathematical Theory of Infectious Diseases and Its Applications , London : Griffin .
- Costantino , R. F. , Cushing , J. M. , Dennis , B. , Desharnais , R. A. and Henson , S. M. 1998 . Resonant population cycles in temporarily fluctuating habitats . Bulletin of Mathematical Biology , 60 : 247 – 273 .
- Cushing , J. M. and Henson , S. M. 2001 . Global dynamics of some periodically forced, monotone difference equations . Journal of Difference Equations and Applications , 7 : 859 – 872 .
- Elaydi , S. N. and Sacker , R. J. 2005 . Global stability of periodic orbits of nonautonomous difference equations and population biology . Journal of Difference Equations , 208 : 258 – 273 .
- Elaydi , S. N. and Sacker , R. J. Global stability of periodic orbits of nonautonomous difference equations in population biology and Cushing–Henson conjectures . Proceedings of the 8th International Conference on Difference Equations and Applications . 2005 . pp. 113 – 126 . Boca Raton, FL : Chapman & Hall/CRC .
- Elaydi , S. N. and Sacker , R. J. 2005 . Nonautonomous Beverton–Holt equations and the Cushing-Henson conjectures . Journal of Difference Equations and Applications , 11 : 336 – 346 .
- Elaydi , S. N. and Sacker , R. J. 2006 . Periodic difference equations, populations biology and the Cushing–Henson conjectures . Mathematical Biosciences , 201 : 195 – 207 .
- Franke , J. E. and Selgrade , J. F. 2003 . Attractor for periodic dynamical systems . Journal of Mathematics Analysis and Applications , 286 : 64 – 79 .
- Franke , J. E. and Yakubu , A.-A. 2005 . Periodic dynamical systems in unidirectional metapopulation models . Journal of Difference Equations and Applications , 11 : 687 – 700 .
- Franke , J. E. and Yakubu , A.-A. 2005 . Multiple attractors via cusp bifurcation in periodically varying environments . Journal of Difference Equations and Applications , 11 : 365 – 377 .
- Franke , J. E. and Yakubu , A.-A. 2005 . Population models with periodic recruitment functions and survival rates . Journal of Difference Equations and Applications , 11 : 1169 – 1184 .
- Franke , J. E. and Yakubu , A.-A. 2006 . Discrete-time SIS Epidemic Model In a Seasonal Environment . SIAM Journal on Applied Mathematics , 66 : 1563 – 1587 .
- Fretwell , S. D. 1972 . Populations in a Seasonal Environment , Princeton, NJ : Princeton University Press .
- Henson , S. M. 2000 . Multiple attractors and resonance in periodically forced population models . Physica D , 140 : 33 – 49 .
- Henson , S. M. 1999 . The effect of periodicity in maps . Journal of Difference Equations and Applications , 5 : 31 – 56 .
- Henson , S. M. , Costantino , R. F. , Cushing , J. M. , Dennis , B. and Desharnais , R. A. 1999 . Multiple attractors, saddles, and population dynamics in periodic habitats . Bulletin of Mathematical Biology , 61 : 1121 – 1149 .
- Henson , S. M. and Cushing , J. M. 1997 . The effect of periodic habitat fluctuations on a nonlinear insect population model . Journal of Mathematical Biology , 36 : 201 – 226 .
- Jillson , D. 1980 . Insect populations respond to fluctuating environments . Nature , 288 : 699 – 700 .
- Kocic , V. L. 2005 . A note on nonautonomous Beverton–Holt model . Journal of Difference Equations and Applications , 11 : 415 – 422 .
- Kon , R. 2004 . A note on attenuant cycles of population models with periodic carrying capacity . Journal of Difference Equations and Applications , 10 : 791 – 793 .
- Kon , R. 2005 . Attenuant cycles of population models with periodic carrying capacity . Journal of Difference Equations and Applications , 11 : 423 – 430 .
- Li , J. 1992 . Periodic solutions of population models in a periodically fluctuating environment . Mathematics and Bioscience , 110 : 17 – 25 .
- Nisbet , R. M. and Gurney , W. S.C. 1982 . Modelling Fluctuating Populations , New York : Wiley & Sons .
- Rosenblat , S. 1980 . Population models in a periodically fluctuating environment . Journal of Mathematical Biology , 9 : 23 – 36 .
- Selgrade , J. F. and Roberds , H. D. 2001 . On the structure of attractors for discrete, periodically forced systems with applications to population models . Physica D , 158 : 69 – 82 .
- Yakubu , A. -A. Periodically forced nonlinear difference equations with delay . Difference Equations and Discrete Dynamical Systems, Proceedings of the 9th International Conferenc . Edited by: Allen , L. , Aulbach , B. , Elaydi , S. and Sacker , R. pp. 217 – 231 . River Edge, NJ : World Scientific . University of Southern California
- Castillo-Chavez , C. and Yakubu , A. 2001 . Dispersal, disease and life-history evolution . Mathematics and Bioscience , 173 : 35 – 53 .
- Castillo-Chavez , C. and Yakubu , A. 2001 . Discrete-time S-I-S models with complex dynamics . Nonlinear Analysis , 47 : 4753 – 4762 .
- Castillo-Chavez , C. and Yakubu , A. A. 2002 . “ Intraspecific competition, dispersal and disease dynamics in discrete-time patchy environments ” . In Mathematical Approaches for Emerging and Reemerging Infectious Diseases: An Introduction to Models, Methods and Theory , Edited by: Blower , S , Castillo-Chavez , C. , van den Driessche , P. , Kirschner , D. and Yakubu , A.-A. 165 – 181 . New York : Springer-Verlag .
- May , R. M. and Oster , G. F. 1976 . Bifurcations and dynamic complexity in simple ecological models . American Naturalist , 110 : 573 – 579 .
- May , R. M. 1977 . Simple mathematical models with very complicated dynamics . Nature , 261 : 459 – 469 .
- May , R. M. 1974 . Stability and Complexity in Model Ecosystems , Princeton, NJ : Princeton University Press .
- Nicholson , A. J. 1954 . Compensatory reactions of populations to stresses, and their evolutionary significance . Australian Journal of Zoology , 2 : 1 – 65 .
- Allen , L. J.S. and Burgin , A. M. 2000 . Comparison of deterministic and stochastic SIS and SIR models in discrete-time . Mathematics and Bioscience , 163 : 1 – 33 .
- Allen , L. J.S. 1994 . Some discrete-time SI, SIR and SIS epidemic models . Mathematics and Bioscience , 124 : 83 – 105 .
- Beverton , R. J.H. and Holt , S. J. 1957 . On the Dynamics of Exploited Fish Populations, Fish. Invest. Ser. II , London : H. M. Stationery Office .
- Elaydi , S. N. and Yakubu , A.-A. 2002 . Global stability of cycles: Lotka–Volterra competition model with stocking . Journal of Difference Equations and Applications , 8 : 537 – 549 .
- Alligood , K. , Sauer , T. and Yorke , J. A. 1996 . Chaos: An Introduction to Dynamical Systems , New York : Springer-Verlag .
- Yakubu , A.-A. and Fogarty , M. 2006 . Spatially discrete metapopulation models with directional dispersal . Mathematical Bioscience , 204 : 68 – 101 .
- Hadeler , K. P. and van den Driessche , P. 1997 . Backward bifurcation in epidemic control . Mathematics and Bioscience , 146 : 15 – 35 .
- Hassell , M. P. , Lawton , J. H. and May , R. M. 1976 . Patterns of dynamical behavior in single species populations . Journal of Animal Ecology , 45 : 471 – 486 .
- van den Driessche , P. and Watmough , J. 2000 . A simple SIS epidemic model with a backward bifurcation . Journal of Mathematical Biology , 40 : 525 – 540 .