Abstract
Stochastic partial differential equations (SPDEs) for size-structured and age- and size-structured populations are derived from basic principles, i.e. from the changes that occur in a small time interval. Discrete stochastic models of size-structured and age-structured populations are constructed, carefully taking into account the inherent randomness in births, deaths, and size changes. As the time interval decreases, the discrete stochastic models lead to systems of Itô stochastic differential equations. As the size and age intervals decrease, SPDEs are derived for size-structured and age- and size-structured populations. Comparisons between numerical solutions of the SPDEs and independently formulated Monte Carlo calculations support the accuracy of the derivations.
1. Introduction
In this paper, a stochastic partial differential equation (SPDE) is derived for a population whose individuals randomly experience births, deaths, and size changes. An SPDE is also derived for an age-structured population undergoing births, deaths, and size changes. The SPDEs are derived from basic principles, i.e. from the changes in the system that occur in a small time interval. Each dynamical system is carefully studied to determine the different independent random changes that occur. Appropriate terms are identified for these changes in developing a discrete-time stochastic model, which then infers a certain stochastic differential equation (SDE) system, see, e.g. Citation2 Citation4 Citation6. The SDE system then leads to an SPDE Citation3. As the techniques used to derive SPDEs have recently been developed Citation3, it is likely that this paper presents the first derivations of SPDEs for problems in mathematical biology.
In constructing certain population dynamics models, the age and/or size of the individuals in the population can be an important consideration. Any factor, such as age or size, that has a significant influence on reproduction or survival in a population must be considered in developing an accurate mathematical model. There has been much work deriving, developing, and analyzing deterministic size-structured and age- and size-structured models, e.g. Citation1 Citation13 Citation20 Citation22 Citation23 Citation25. Size- and/or age-structured population models have been widely employed to study such diverse processes as cell population growth, harvesting, plant evolution, and cannibalism, e.g. Citation9 Citation11 Citation12 Citation17 Citation18. Such structured models are of fundamental importance in mathematical biology. In the past few years, SDEs have provided additional insight into the effects of random influences on the population dynamics, e.g. Citation2 Citation4 Citation5 Citation7. For example, random influences have a strong effect on the persistence time of a population. Recently developed techniques are applied in the present investigation to derive SPDE versions of the deterministic size-structured and age- and size-structured population models. The SPDEs derived here account for demographic variability due to the random occurrence of births, deaths, and size changes and generalize the deterministic partial differential equation models. Environmental variability in age- and size-structured population dynamics is not studied in the present investigation, although environmental changes may produce independent random changes in the birth, death, and growth rates as studied in Citation7 for discrete and continuous stochastic population models. Assuming that the environment produces independent random changes in the birth, death, and growth rates, the SPDEs derived in the present investigation can be extended to systems of SPDEs to account for environmental as well as demographic variability.
In the next section, a SPDE is derived for a size-structured population. The changes in size, births, and deaths are assumed to occur randomly with probability proportional to the population level and to the time interval. In the following section, an SPDE is derived for a size- and age-structured population. Changes in births, deaths, and sizes are again assumed to occur randomly for this population. However, age changes are assumed to occur deterministically, i.e. age depends only on time since birth and so age, for example, is not considered a physical condition. The two stochastic models are then solved computationally and compared with Monte Carlo calculations. The Monte Carlo calculational procedure differs from the numerical method for solving the SPDEs. In the Monte Carlo calculations, the populations are checked at each small interval of time to see if a birth, death, or size change occurs. Comparisons between the two different computational methods are in close agreement indicating that the SPDEs accurately model the random behaviour of size- and age-structured populations.
2. Derivation of a size-structured population SPDE
A standard partial differential equation for a size-structured population has the form Citation1 Citation12 Citation13 Citation20 Citation25:
System (1) is deterministic and random variations in the population level due to the inherent randomness in births, deaths, and size changes cannot be studied using this system. To derive a SPDE generalization of EquationEquation (1), the changes that occur in the population for a small time interval are tabulated taking into account the randomness in births, deaths, and size changes. A discrete stochastic model of a size-structured population is then constructed. As the time interval decreases, the discrete stochastic model leads to a system of Itô SDEs. As the size interval decreases, a SPDE is derived for a size-structured population.
2.1. Some properties of Brownian sheets
Before deriving these stochastic equations, it is useful to consider several properties of Brownian sheets Citation8 Citation10 Citation24. A Brownian sheet on [0, 5]×[0, 5] is illustrated in . The Brownian sheet W(x, t) satisfies:
2.2. An SPDE for a size-structured population
Consider now the changes which occur in the size-structured population for a small time interval Δ t. To find these changes, it is assumed that the population is divided into K size intervals [x
k−1, x
k
], for k = 1, 2, …, K, where ,
, and u
k
(t) is the population level at time t of size x
k−1 to size x
k
. The changes possible for u
k
= u
k
(t) are tabulated in for k > 1. Births are added to the first size class and the possible changes are given in for k = 1. In and ,
is the growth rate for individuals of size class k,
is the death rate for individuals of size class k, and
is the birth rate. In particular, for Δ t small, the probability of an individual increasing in size from size class k−1 to size class k in time Δ t is
, the probability of a death in size class k is
, and the probability of a birth into size class 1 is
.
Table 1. Possible changes in population level u k (t) for k > 1 for time Δ t.
Table 2. Possible changes in population level u 1(t) for time Δ t.
and define a discrete stochastic model for the system of K sub-populations. The mean and mean square of the change are important for constructing a system of Itô SDEs. These values are readily calculated using and to obtain:
Introduced now is a one-dimensional Wiener process W*(t; x) parameterized by size x such that W*(t; x) is an independent Wiener process for each value of x. In addition, a Brownian sheet W(x, t) is applied. Then, the preceding equations can be written as:
The size interval Δ x is now allowed approach zero, resulting in a SPDE system for the size-structured population. Letting and decreasing Δ x, then
Notice that EquationEquations (10) and Equation(11)
generalize EquationEquation (1)
. If the stochastic terms are set equal to zero, then EquationEquations (10)
and Equation(11)
are identical to EquationEquation (1)
. Of course, for the deterministic or stochastic version, the initial condition and number of individuals are given, respectively, by
3. Derivation of a size- and age-structured population SPDE
In this section, the population's age structure as well as size structure is also considered. The size-structured EquationEquation (1) can be extended to the size- and age-structured partial differential equation Citation22:
Similar to EquationEquation (1), EquationEquation (12)
is deterministic and random variations in the population level due to the inherent randomness in births, deaths, and size changes cannot be studied using this equation. To derive a SPDE generalization of EquationEquation (1)
, the changes which occur in the population for a small time interval are tabulated carefully taking into account the randomness in births, deaths, and size changes. Age changes are assumed here to occur deterministically, i.e. the age of an individual is merely the time since birth and is not considered a condition or state of an individual although such a consideration would be interesting. A discrete stochastic model of the size- and age-structured population is next constructed. As the time interval decreases, the discrete stochastic model leads to a system of Itô SDEs. As the size and age intervals decrease, a SPDE is derived for the size- and age-structured population.
Consider now the changes which occur in the population for a small time interval Δ t. To find these changes, it is assumed that the population is divided into J age intervals [y
j−1, y
j
], for j = 1, 2, …, J where ,
, and K size intervals [x
k−1, x
k
], for k = 1, 2, …, K where
,
, and u
j, k
(t) is the population level at time t of individuals with age from y
j−1 to age y
j
and of size x
k−1 to size x
k
. The changes possible for
are tabulated in for j > 1. Births are added to the first age class so
includes the rate of births into the kth size class. The possible changes are given in for j = 1. In and ,
is the growth rate for individuals of age class j and size class k,
is the death rate for individuals of age class j and size class k, and
is the birth rate for individuals of size class k′ and age class j′ with newborns in size class k. In particular, in small time Δ t, there is a probability of 1 for
individuals to change age from age-size class j−1, k to age-size class j, k, there is a probability of
for an individual to change size from age-size class j, k−1 to age-size class j, k, there is a probability of
of a death in time Δ t in age-size class j, k, and there is a probability of
of a birth to age-size class 1, k.
Table 3. Possible changes in population level u j, k (t) for j > 1 in time Δ t.
Table 4. Possible changes in population level u 1, k (t) in time Δ t.
and define a discrete stochastic model for the system of K sub-populations. The mean and mean square of the change are important for constructing a system of Itô SDEs and can be readily calculated using these tables to obtain for j ≥ 2:
Introduced now is a two-dimensional Brownian sheet W*(t, y; x) parameterized by size x such that W*(t, y; x) is an independent Brownian sheet for each value of x. In addition, a three-dimensional Brownian sheet W(x, y, t) is applied. Then, the preceding equations can be written as:
The size and age intervals Δ x and Δ y are now allowed to approach zero, resulting in a SPDE for the size-structured population. Letting and decreasing Δ x and Δ y, then
4. Comparison with Monte Carlo calculations
In this section, the SPDEs derived in the previous two sections for the structured populations are numerically solved and compared with independent Monte Carlo computations. First, the SPDEs Equation(10) and Equation(11)
are considered. To define a numerical method, the population is divided into K size intervals [x
k−1, x
k
], for k = 1, 2, …, K where
,
and time is discretized where
Integrating EquationEquation (10)
over a size interval and using an explicit approximation in time t suggests the numerical procedure
Results obtained by solution of EquationEquations (23) and Equation(24)
were compared with results obtained using an independent Monte Carlo computational procedure for estimating size-structured population levels. In the Monte Carlo procedure, the population was divided into 100 size classes and time was divided into 1000 intervals where the total time was taken, for illustrative purposes, as 0.5. For each time step, each sub-population was checked for a death, birth, or size change using the probabilities given in and .
In the calculational comparisons, the growth rate, death rate, birth rate, and initial population were assumed to be ,
,
,
, respectively, with x
min = 0 and x
max = 1. For these parameters, the growth rate declines with size x and the death and birth rates are assumed constant. With these parameter selections, the exact solution to the deterministic size-structured models Equation(1)
and Equation(2)
is
with the total number of individuals
. Thus, the deterministic population level increases exponentially with an average size of the individuals of 0.25. Of interest here is the random behaviour of the population.
Summarized in are the results for 500 sample paths (500 different runs) for the SPDE calculation and for the Monte Carlo procedure. The calculational results are in close agreement. The population levels (total number of individuals) and average individual size at time t = 0.5 for 500 sample paths are shown in and , respectively. It is clear that the SPDE accurately models the dynamics of the size-structured populations.
Figure 2. Calculated distribution of populations levels at time t = 0.5 for 500 sample paths using Monte Carlo (MC) and the SPDE Equation(10) and Equation(11)
.
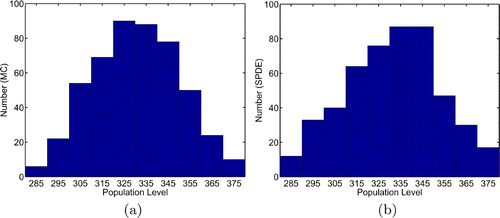
Figure 3. Calculated distribution of average size at time t = 0.5 for 500 sample paths using Monte Carlo (MC) and the SPDE Equation(10) and Equation(11)
.
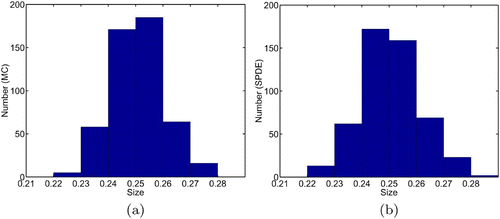
Table 5. Monte Carlo (MC) and SPDEs Equation(10)
and Equation(11)
calculational results at time t = 0.5.
The SPDEs Equation(21) and Equation(22)
are numerically investigated in a similar way. To obtain a numerical procedure, the population is divided into K size intervals [x
k−1, x
k
], for k = 1, 2, …, K where
,
and J age intervals [y
j−1, y
j
] where
and
. Integrating EquationEquation (21)
over a size interval and over an age interval and using an explicit approximation in time t suggests the numerical procedure
Numerical solution of EquationEquations (25) and Equation(26)
were compared with results obtained using an independent Monte Carlo computational procedure for approximating the age- and size-structured population levels. In the Monte Carlo procedure, the population was divided into 200 size-age classes and time was divided into 4000 steps where the final time was taken, for illustrative purposes, as t = 1. For each time step, each sub-population was checked for a death, birth, or size change using the probabilities given in and . To compare the two independent computational approaches, the growth rate, death rate, birth rate, and initial population were assumed to be
,
,
,
, respectively, with x
min = 0, x
max = 1, y
min = 0, and y
max = 1. For these parameters, the growth rate declines with size x and age y, the death rate increases rapidly as the maximum age y = 1 is reached, and the birth rate size distribution peaks at size x = 1/2. With these parameter selections, the exact solution to the deterministic age- and size-structured model Equation(12)
is
with the total number of individuals
. Thus, the deterministic population level increases exponentially with an average size of the individuals of 0.5 and with an average age of 0.25. Of interest here is the random behaviour of the population.
Summarized in and are the results for the 500 sample paths (500 different runs) for the SPDE numerical method and for the Monte Carlo procedure. Notice that the results for the two different computational procedures are close. The population levels (total number of individuals), average individual size, and the average individual age at time t = 1 for 500 sample paths are shown in , and . It is clear that the SPDE accurately models the dynamics of the age- and size-structured populations.
Figure 4. Calculated distribution of populations levels at time t = 1.0 for 500 sample paths using Monte Carlo (MC) and the SPDE Equation(21) and Equation(22)
.
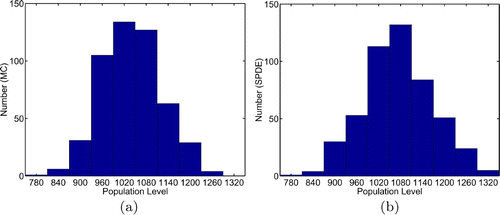
Figure 5. Calculated distribution of average size at time t = 1.0 for 500 sample paths using Monte Carlo (MC) and the SPDE Equation(21) and Equation(22)
.
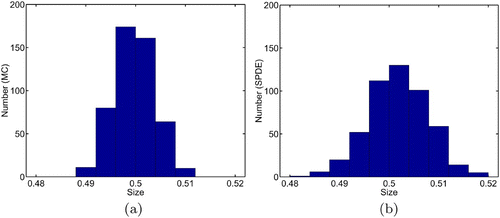
Figure 6. Calculated distribution of average age at time t = 1.0 for 500 sample paths using Monte Carlo (MC) and the SPDE Equation(21) and Equation(22)
.
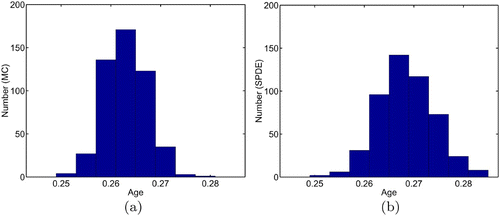
Table 6. Monte Carlo (MC) and SPDE Equation(21)
and Equation(22)
calculational results at time t = 1.0.
Table 7. Monte Carlo (MC) and SPDE Equation(21)
and Equation(22)
calculational results at time t = 1.0.
5. Summary and conclusions
SPDEs are becoming increasingly important in applied mathematics Citation14–16,Citation21. Recently developed techniques Citation3
Citation6 are applied in the present investigation to derive SPDEs for age- and size-structured populations. In the derivation procedure, the deterministic and stochastic terms in the differential equation system are simultaneously derived. First, a discrete stochastic model is constructed. Next, an SDE system is derived. Finally, a particular SPDE follows from the SDE system. The SPDE for a size-structured population is given by EquationEquations (10) and Equation(11)
. The SPDE for an age- and size-structured population is given by EquationEquations (21)
and Equation(22)
. The stochastic equations generalize the deterministic size- and age-structured models and include the demographic influences of randomly varying births, deaths, and size changes. Hence, random influences on certain properties of a structured population, such as on persistence time or on size variability, can be studied using these SPDEs. The SPDEs derived for age- and size-structured population models are solved numerically and compared with an independently formulated Monte Carlo method. The computational results between the two different numerical methods are in good agreement supporting the accuracy of the SPDE derivation procedure.
Acknowledgements
The author acknowledges partial support from the National Science Foundation grant NSF-DMS 0718302.
References
- Ackleh , A. S. , Deng , K. and Thibodeaux , J. J. 2007 . A monotone approximation for a size-structured population model with a generalized environment . J. Biol. Dyn. , 1 : 291 – 304 .
- Allen , E. J. 2007 . Modeling With Itô Stochastic Differential Equations , Dordrecht : Springer .
- Allen , E. J. 2008 . Derivation of stochastic partial differential equations . Stoch. Anal. Appl. , 26 : 357 – 378 .
- Allen , L. J.S. 2003 . An Introduction to Stochastic Processes with Applications to Biology , Upper Saddle River, New Jersey : Pearson Education Inc .
- Allen , L. J.S. and Allen , E. J. 2003 . A comparison of three different stochastic population models with regard to persistence time . Theor. Popul. Biol. , 68 : 439 – 449 .
- Allen , E. J. , Allen , L. J.S. , Arciniega , A. and Greenwood , P. E. 2008 . Construction of equivalent stochastic differential equation models . Stoch. Anal. Appl. , 26 : 274 – 297 .
- Allen , E. J. , Allen , L. J.S. and Schurz , H. 2005 . A comparison of persistence-time estimation for discrete and continuous stochastic population models that include demographic and environmental variability . Math. Biosci. , 196 : 14 – 38 .
- Allen , E. J. , Novosel , S. J. and Zhang , Z. 1998 . Finite element and difference approximation of some linear stochastic partial differential equations . Stoch. Stoch. Rep. , 64 : 117 – 142 .
- Arino , J. , Gouzé , J.-L. and Sciandra , A. 2002 . A discrete, size-structured model of phytoplankton growth in the chemostat . J. Math. Biol. , 45 : 313 – 336 .
- Cabaña , E. M. 1970 . The vibrating string forced by white noise . Zeitschrift für Wahrscheinlichkeitstheorie und verwandte Gebiete , 15 : 111 – 130 .
- Childs , D. Z. , Rees , M. , Rose , K. E. , Grubb , P. J. and Ellner , S. P. 2003 . Evolution of complex flowering strategies: an age- and size-structured integral projection model . Proc. Biol. Sci. , 270 : 1829 – 1838 .
- Cushing , J. M. 1992 . A size-structured model for cannibalism . Theor. Popul. Biol. , 42 : 347 – 361 .
- Cushing , J. M. 1998 . An Introduction to Structured Population Dynamics , Philadelphia : SIAM .
- Da Prato , G. and Tubaro , L. 2006 . Stochastic Partial Differential Equations and Applications – VII , Edited by: Da Prato , G. and Tubaro , L. Boca Raton, Florida : CRC Press, Taylor & Francis Group .
- Gunzburger , M. 2007 . Numerical methods for stochastic PDEs . SIAM News , 40 : 3
- Holden , H. , Øksendal , B. , Ubøe , J. and Zhang , T. 1996 . Stochastic Partial Differential Equations: A Modeling, White Noise Functional Approach , Boston, Massachusetts : Birhäuser .
- Kato , N. 2006 . Linear size-structured population models and optimal harvesting problems . Int. J. Ecol. Dev. , 5 : 6 – 19 .
- Kato , N. , Oharu , S. and Shitaoka , K. 2007 . Size-structured population models and harvesting problems . J. Comput. Appl. Math. , 204 : 114 – 123 .
- Kloeden , P. E. , Platen , E. and Schurz , H. 1994 . Numerical Solution of SDE Through Computer Experiments , Berlin : Springer .
- Kostova , T. 2003 . An explicit third-order numerical method for size-strucured population equations . Numer. Meth. Partial Diff. Equ. , 19 : 1 – 21 .
- Schurz , H. 2007 . Nonlinear stochastic wave equations in R 1 with power-law nonlinearity and additive space-time noise . Contemp. Math. , 440 : 223 – 242 .
- Sinko , J. W. and Streifer , W. 1967 . A new model for age-size structure of a population . Ecology , 48 : 910 – 918 .
- Thieme , H. R. 1991 . Analysis of age-structured population models with an additional structure . Lect. Notes Pure Appl. Math. , 131 : 115 – 126 .
- Walsh , J. B. 1986 . “ An introduction to stochastic partial differential equations ” . In Lect. Notes in Mathematics – Volume 1180 , Edited by: Dold , A. and Eckmann , B. 265 – 439 . Berlin : Springer-Verlag .
- Webb , G. F. 1985 . Theory of Age-Dependent Population Dynamics , New York : Marcel-Dekker .