Abstract
The problem of who is mixing with whom is of great theoretical importance in the context of heterosexual mixing. In this article, we publish for the first time, data from a study carried out in 1989 that had the goal of estimating who is mixing with whom, in heterosexually active college populations in the presence of co-factors like drinking. The gathering of these data and the challenges involved in modelling the interaction between and among heterosexually active populations of individuals are highlighted in this manuscript. The modelling is based on the assumptions that at least two processes are involved: individual affinities or preferences determine ‘what we want’ while mixing patterns describe ‘what we get’. We revisit past results on the role of affinity/preference on observed mixing patterns in one- and two-sex mixing populations. Some new results for homosexually active populations are presented. The study of mixing is but the means to an end and consequently, we also look at the role of affinity on epidemics as filtered by observed mixing patterns. It would not be surprising to observe that highly distinct preference or mixing structures may actually lead to quite similar epidemic patterns.
1. Introduction
The question of who interacts with whom and the reasons behind recorded or observed preferential patterns of interactions among or between individuals is of interest in population dynamics, demography, epidemiology, and social dynamics. Formulating suitable frameworks that incorporate preferential interactions between or within groups or subpopulations and scaling them up to the level of the population or community is important in the study of processes where heterogeneity may be relevant to the time evolution of a population or to the selective pressures that shape its structure Citation9 Citation10 Citation12 Citation14 Citation15 Citation24 Citation25 Citation27 Citation30 Citation31 Citation37 Citation39 Citation72. Identifying precise mechanisms responsible for observed mixing patterns is riddled with difficulties because a multitude of behavioural patterns can lead to ‘identical’ population mixing patterns. There is an inherent lack of uniqueness in the identification of the individual-level mechanisms that shape community patterns; that is, similar mixing population-level patterns may result from distinct individually driven contact structures. Not surprisingly, the study of the role of individuals’ preferences or affinities on population dynamics has been studied under rather simplified settings or avoided all together. The modus operandi (classical mathematical demographic models) has been to focus on the study of role of vital rates (birth and death processes) on population dynamics while ignoring the contact structures or mating systems responsible for the reproduction process. This effort has been carried out quite effectively in the context of single-sex (female) models Citation18 Citation56 Citation57 Citation61.
While mating structures have been extensively studied in population genetics Citation23, they have not received the same level of attention at the population level partly because the resulting system of equations are not easy to analyse. Nevertheless, the desire to develop a framework that naturally incorporates diverse mating processes has always been of interest to biologists and demographers alike. Here is some history on the mixing problem.
The study of population-level mating structures often called marriage functions in demography was pioneered over three decades ago Citation27 Citation50 Citation51 Citation62 Citation65 Citation66. A large number of solid extensions Citation16 Citation17 Citation18 Citation38 Citation72 have been carried out, but unfortunately, they have not been implemented by other behavioural epidemiologists, sociologists, or relevant policy-makers. The HIV/AIDS epidemic attracted the attention of an increasing number of theoreticians to the study of mating systems, including the role of preference on two-sex dynamics. Hence, the study of questions such as who mixes with whom and their consequences accelerated with the emergence of the HIV/AIDS pandemic Citation10 Citation24 Citation25 Citation30 Citation31 Citation33 Citation34 Citation40 Citation41 Citation42 Citation43 Citation44 Citation45 Citation47 Citation48 Citation52 Citation53 Citation54 Citation69 Citation70 Citation71 Citation73. Furthermore, emphasis has been put on the study of the dynamics of social networks and their applications to the study of the transmission dynamics of infectious or sexually transmitted diseases Citation4 Citation5 Citation7 Citation21 Citation28 Citation29 Citation32 Citation49 Citation55 Citation64 Citation68. This is an area of extensive active research.
Our own efforts have involved the development of theoretical models as well as empirical studies aimed at identifying and quantifying mixing patterns. A survey instrument Citation37 Citation22 was developed with the goal of confronting the complications associated with the estimation of contact structures (who is mixing with whom) in populations living in ‘controlled’ environments. Our focus has been on residential college student populations. The college survey focused on identifying who mixes with whom in the context of sexual or ‘dating’ activities. The role of co-factors like alcohol, which plays a critical role in college-drinking dynamics Citation63, was explored as well. Our approach is flexible and quantifiable and can be applied to the study of a multitude of processes that include disease dynamics, the transmission dynamics of cultural traits, general demographic processes, and even frequency-dependent predation Citation39 Citation59 Citation8 Citation9 Citation5 Citation7 Citation4 Citation10 Citation14 Citation15. Serious efforts to connect these models to data are found in Citation38 Citation37 Citation68 Citation6. Recent theoretical results and applications include those in Citation26 Citation20 Citation72.
This paper provides an incomplete overview of the problem of mixing, focusing on questions involving the structure described in Citation8 Citation9 Citation5. Section 2 revisits a two-sex mixing framework expansion developed in Citation11 Citation12 Citation5 Citation8 Citation37. Our theoretical efforts in the two-sex case Citation8 Citation9 Citation4 Citation5 Citation6 Citation68 Citation38 Citation37 drove us not only to the development of a survey instrument but to its implementation Citation37 Citation22. Two-sex, P−Q, mixing matrices directly computed from survey college mixing data are presented, for the first time, to illustrate the challenges involved in connecting data with mixing. Our two-sex formulation is a natural expansion of the single-sex version that first appeared in Citation8 Citation9 Citation4 Citation5. Its presentation serves as a prelude for the introduction of new results (via simulations) on one-sex mixing, the object of Sections 3 and 4. Section 3 highlights the theoretical results associated with the discrete version of the single-sex mixing group framework developed in Citation8 Citation9 Citation4 Citation5 Citation37 Citation14 Citation38 Citation12. In addition to the prior results, we analytically and computationally explore the effects of stochastic fluctuations within the affinity component of the mixing matrix on the role of preference, e.g. the patterns of who mixes with whom in the one-sex framework. Section 4 explores the impact of mixing in homosexually active populations on disease dynamics. A two-group susceptible–infectious–susceptible (SIS) model is used to explore how the level of variation inherent in the simplest form of heterogenous mixing (proportionate mixing) filters over an epidemic process. Section 5 discusses mixing in the context of past, current, and future applications.
2. Two-sex mathematical formulation
Marriage functions have been studied extensively in demography Citation50 Citation51 Citation27 Citation62 Citation65 Citation66. However, the lack of useful results (theoretical results that can be connected to data) managed to reduce interest in the study of the dynamics of ‘mating’ at the population level for some time. The start of the HIV/AIDS pandemic in developed nations regenerated theoretical and practical interest in the study of marriage functions, given the importance naturally placed in the study of the dynamics of heterosexually transmitted diseases. Questions like who mixes with whom and subsequent studies on the consequences of social dynamics became increasingly important again as HIV/AIDS grew Citation24 Citation25 Citation30 Citation31 Citation33 Citation73 Citation10 Citation34. The study of heterosexually transmitted diseases have also contributed to the growing interest in the study of epidemics on social networks. Recent advances in the network theory have in fact increased the expectation that the theory may finally provide useful insights on the dynamics (outcomes) of ‘two-body’ problems in biology Citation49 Citation28 Citation55 Citation21 Citation29 Citation32 Citation64.
The two-sex framework presented was first introduced in Citation11 as a natural extension of the modelling work carried out by Sir Ronald Ross on the dynamics of vector-transmitted diseases Citation67. Ross’ solutions, found in a primitive fashion in Ross's Citation67 article, are used to describe more general forms of mixing. The extensions and results on two-sex mixing have been taken from the detailed descriptions provided in Citation12 Citation38 Citation37.
In order to present the two-sex framework, the following additional notation and definitions are required: p
ij
(t) is the probability that a male in group i mixed with a female in group j at time t given that he mixed with somebody; q
ji
(t) is the probability that a female in group j mixed with a male in group i at time t given that she mixed with somebody; is the number of males in group i at time t,
is the number of females in group j at time t;
the average (not necessarily constant) number of female partners per group-i male per time unit, which is per capita pair formation rate for group-i males,
is the average (not necessarily constant) number of male partners per group-j female per time unit, which is per capita pair formation rate for group-j females.
Definition
The matrices
Q=(q
ji
(t)) are called a mixing/pair formation matrix if and only if they satisfy the following properties at all times:
-
(A1)
.
-
(A2)
for all i, and
for all j.
-
(A3)
for all i, j.
-
(A4) If for some i and/or some j, we have
for some t, then we define
.
It is important to point out that in general, it would be impossible to maintain the above constraints (first put forward in terms of constant contact rates by Ross) under the assumption that the per capita rates of activity for males and females are constant. In fact, in general, the and
must be functions of both the availability of male and females. This point is evident from the single-group two-sex formulations found in Citation50
Citation51
Citation27
Citation62
Citation65
Citation66 and in the relatively recent extension of Citation39.
The only separable solutions to (A1)–(A4) are known as the Ross’ solutions. They are denoted by (p¯ j (t), q¯ i (t)), where
Moreover, all solutions to (A1)–(A4) can be represented as multiplicative perturbations to Ross’ solutions. Explicitly,
Also as a consequence of this relationship among the φ values, we have the so-called it takes two to tango theorem Citation37.
Theorem 2.1
(T
3) The non-negative affinities of two genders,
and
, obey the following explicit relationship at all times, letting
and
This was also generalized to include age structure in Citation37 and role of preference explored in this last context in Citation13.
2.1. Estimating p ij and q ij from survey data
The spread of sexually transmitted diseases may depend on a few small groups of individuals (core groups) Citation35. Estimation of the sizes and behaviours of the core groups is thus very relevant for a correct prediction of the epidemics, especially for HIV/AIDS. For this purpose, a survey was conducted in 1989 in Cornell University to investigate social and sexual patterns among undergraduate students Citation22. Two versions of a questionnaire with identical appearance were prepared: the indirect and the direct versions. The common questions in both versions included frequencies of attending lectures and social activities, availability and consumption of alcohol at different social activities, number and gender of pair-off partners at different social activities, study year and demographics of the partners, as well as personal information of the respondent, such as study year, gender, living in campus or not, marital status, age, family gross annual income, religion, and ethnicity. The indirect version included further questions about frequency of intimate behaviour and the association between pair-off and consumption of alcohol/drugs. The direct version included questions regarding explicit sexual intercourse, including frequency, use of condoms, and the association of these behaviours with the consumption of alcohol/drugs. Two thousand names were randomly drawn from the registrars list of all undergraduates and invited by a letter to participate in the survey. The participants received either questionnaire version by random choice with equal chance and answered the questions in an anonymous way. The indirect questionnaire was filled out by 493 students, while the direct questionnaire by 502 students. Students received a small reward for participating or had a chance to participate in a lottery that offered a larger reward.
Based on data of sexually active respondents, the sizes of active population and the mixing patterns were estimated Citation38. In heterosexually dating active respondents, the proportion of alcohol drinker among females is highest among freshmen (33%) and lowest among seniors (10%), while among males the proportion of alcohol drinker is similarly high in the first three study years (28–32%) and reduced by about half for seniors (14%). Even the size of the non-targeted population, graduate/staff/faculty and non-Cornell, was estimated using the mark–recapture method, as shown in Citation68. Specifically, a modified mark–recapture approach was used to estimate total size of these active populations from a single survey and then a sort of minimized least squares approach was used to estimate the and the
contact rates.
The data were placed into two larger categories, mixing with or without alcohol along with stratifications by year, as shown in and . In heterosexually dating active female respondents, the mean, standard deviation, and median of the number of partners are, respectively, 1.86, 1.35, and 2 for non-alcohol drinkers and 1.68, 1.04, and 1 for drinkers; the difference between the two alcohol groups is statistically significant (Wilcoxon two-sample test p=0.0019). In male respondents, the corresponding mean, standard deviation, and median are 3.28, 2.42, and 3 for non-alcohol drinkers and 3.19, 4.50, and 2 for drinkers; the difference between the two alcohol groups is statistically non-significant (Wilcoxon two-sample test p=0.086). Results of these two tests suggest that the effect of alcohol on number of partners is different between female and male respondents. Without alcohol, we arrive at mixing matrices
Figure 1. Distribution of number of pair-off partners in female respondents with heterosexual dating activity. (a) Stratified by status of alcohol consumption; (b) stratified by study year of respondent.
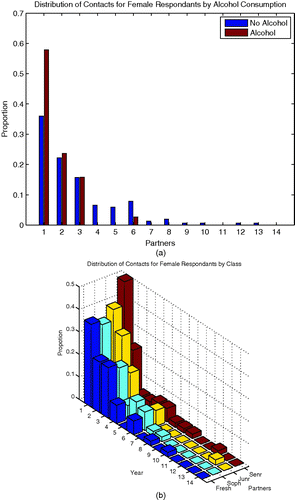
Figure 2. Distribution of number of pair-off partners in male respondents with heterosexual dating activity. (a) Stratified by status of alcohol consumption; (b) stratified by study year of respondent.
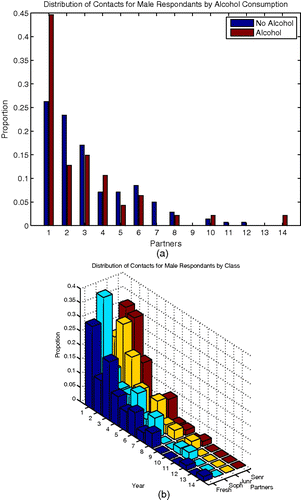
Figure 3. Pair-off mixing matrix of female respondents with heterosexual dating activity. (a) In respondents without alcohol consumption; (b) in respondents with alcohol consumption.
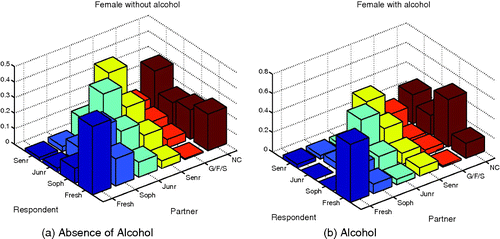
Figure 4. Pair-off mixing matrix of male respondents with heterosexual dating activity. (a) In respondents without alcohol consumption; (b) in respondents with alcohol consumption.
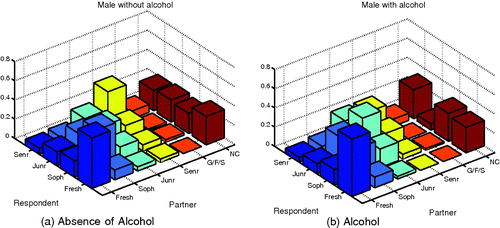
The presence of alcohol dramatically increases the likelihood of sophomores mixing with non-Cornell individuals for females and freshmen with freshmen interactions for both groups. Males experience a large increase in their mixing with juniors in the presence of alcohol with most at the junior mixing with junior level. It should be noted, however, that the total increase in reinforcement due to alcohol is about 17% larger in females than in males. Also for each strata we have data on, except for sophomores, increases in the like-with-like mixing patterns are observed in the presence of alcohol.
In and , it is shown that for both genders sophomores experienced the most aversion from other groups when alcohol is present. Females experience a higher tendency to mix with juniors in the absence of alcohol second only to their tendency to mix with sophomores. There are also large differences in the interactions of freshmen with non-Cornell and sophomores with freshman when alcohol is present with such interactions more likely to take place in the absence of alcohol.
Figure 5. Shown here is the difference of the female and male mixing matrices without alcohol from that with alcohol. Reinforced behaviours are those with positive difference and may be interpreted as mixings more likely when drinking.
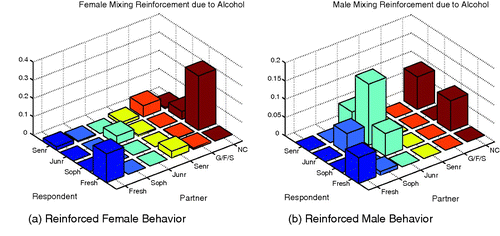
Figure 6. Shown here is the difference of the female and male mixing matrices without alcohol from that with alcohol. Weakened behaviours are those with negative difference and may be interpreted as mixings more likely to occur when sober.
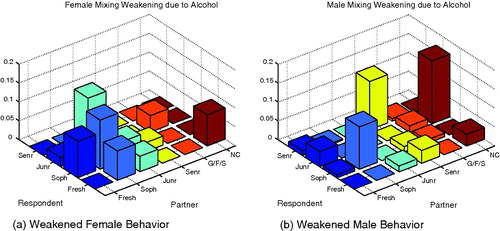
The role of heterogeneity on disease dynamics depends strongly on preferences and mixing. Studying STD (Sexually Transmitted Disease) dynamics in the context of highly heterogenous populations that include heterosexually, homosexually, and bisexually active populations as well as sex workers males and females is a rather complex problem but progress can be made Citation49.
3. Single sex mixing distributions
Work done in Citation8
Citation9
Citation5 sets forth the axiomatic approach used to formulate the process of mixing among heterogenous groups. We describe this setting in the context of a fixed number of interacting subpopulations, each constituted or defined by behaviourally equivalent individuals in a single-sex setting. The mixing is described in terms of a conditional probability matrix P with entries P
ij
, each denoting the fraction of contacts that individuals from group or subpopulation i have with individuals in group or subpopulation j given that a member from group i has made a contact. It is assumed that the subpopulations involved are large (each with size N
i
; i=1, 2, …, L), and that the per capita average contact rates (C
i
, i=1, 2, …, L) are constant and large enough so that all groups are connected. The mixing matrix (P
ij
; i, j in ) is assumed to satisfy three properties or mixing axioms:
The first two make P a stochastic matrix while the third asserts that the average total contact rates between pairs of groups must be balanced. Or in other words, shared contacts per unit of time between two groups must be maintained. In Citation8
Citation9
Citation5
Citation6 it was shown that the only separable solution to the mixing axioms is proportionate mixing, . In fact, it was shown that all matrices P
ij
satisfying the above mixing axioms can be generated as multiplicative perturbations of proportionate mixing via the ‘preference’ symmetric matrix Φ (for alternative, recent result, see Citation26):
Theorem 3.1
[Citation5
Citation6
Citation8
Citation9] The unique separable solution to the mixing axioms is given by
where
,
and
is an L×L symmetric matrix, that is
∀ i, j
.
Efforts to interpret the matrix as group affinities have been carried out but several questions remain. Do the relation between the φ-entries of the Φ-matrix provide a useful relative measure of the ‘desire’ of individuals of group i to mix with those in group j? The work in Citation4
Citation7
Citation8
Citation14
Citation37 strongly supports this perspective, but there are no conclusive analytical results. Here, we continue to explore the relationship between the φ-entries of the Φ-matrix and the P-matrix through simulations. We explore this question in the context of single sex for simplicity and understanding (similar work in the two-sex case is quite complicated). We refer to Φ as the affinity matrix and P as the mixing matrix in the remainder of this manuscript. Further, our baseline perspective starts from the observation that when
with α a constant, the matrix P
ij
reduces to proportionate mixing, that is,
for all
. In other words, in the absence of pronounced differences in the preferences, or affinities, proportionate mixing drives population-level mixing under this framework.
3.1. Analytical results
We explore the role that variation in the Φ-matrix has on the matrix P starting from the case proportionate mixing case, that is, when with α a constant (
for all
). Specifically, it is first assumed that each
for appropriately chosen Φ
ij
values (positive and less than one). It is further assumed that the entries of the Φ-matrix are independent, identically distributed random variables with support on
. Next, a prior distribution for the elements of the affinity matrix Φ is selected. Samples from the Φ distribution are used to explore its role on the distribution of the P-matrix.
In summary, it is assumed that {Φ
ij
} is a collection of i.i.d. random variables on for i≤j each with density function
. The assumed symmetry of Φ-matrix means that
for all j>i. We use Φ
ij
to stress the fact that we are dealing with a random variable and φ
ij
to refer to particular values of the random variable. Φ denotes the random matrix itself.
Now, we are ready to consider the conditional mixing matrix P whose entries, P ij , are defined in Theorem 3.1. The goal would be to calculate the distribution function of P ij but how to do it is not obvious in general. Here we look at the case L=2 for simplicity and proceed to condition on the assumption that values of the diagonal elements of the Φ-matrix are known. Hence, we have that
3.2. Numerical results
In this section, we carry out a few computational experiments based on the above results and formulae. Letting P
ij
, V, and R
i
be defined as before with denoting a symmetric random matrix whose entries are continuous random variables supported on a given subset of (0, 1). We proceed to analyse several cases. For simplicity, we only include the situation where there are two subpopulations, L=2 when C
i
=C and N
i
=N all constants, in fact removing generality from the distribution of P
ij
. However, this is not critical, since the focus here is not in the study of general P-matrices but rather on isolating the role/effect of the distribution of Φ
ij
on the matrix P. Hence, we consider the case where φ
ij
are i.i.d. uniform on
. Thus, this family of random variables has mean 0.5 and variance δ2/3. We also consider the case where the distribution for the φ
ij
’s is given by a beta distribution with the parameters chosen so that these beta distributions have the same mean and variance as the pre-selected family of uniform distributions. The expressions for the beta parameters are thus
For the very tight distributions the mean of P ij is 0.5, with slight deviations like 0.4999, regardless of the distribution on Φ ij and the variance is on the order of 10−6, again with deviations at the 10−8 spot. This represents a slight loss of variance in the transformation from Φ ij to P ij . Important to note is that too, from the perspective of machine precision, 10−15, the distributions are also not dependent on i or j. For tight parameters, the mean of P ij for i≠j was approximately 0.498 and that of P ii was 0.502 with little difference regardless of the family used uniform and beta for the Φ-matrix. Variance for all terms was about 0.0012 with differences between φ distributions in the order of 10−5. This marks yet again a loss of significant value in the variance between Φ ij and P ij . For the very loose parameter regime, we see a mean of 0.5118 for diagonal elements and 0.48818 for off-diagonal elements when uniform distributions were used for the Φ-entries and 0.5116 and 0.4884 when beta distributions were in place. The variance was essentially constant when beta or uniform distributions were used regardless of i and j. In fact, they were 0.0076 and 0.00758 for uniform and beta, respectively. In other words, when the differences in affinity or preference as modelled by the Φ-matrix are small, the P-matrix essentially corresponds to proportionate mixing, but otherwise there seemed to be a drift towards like-with-like mixing as the support grew, as seen in .
Figure 7. Histograms of the P ij values top to bottom with the very tight, tight, and very loose parameter choices. We can observe a definite difference in shape between the uniform and beta shapes but the first and second moments are roughly equivalent.
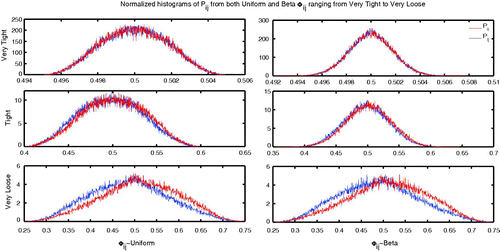
Certainly, from the observation that the relationship between limited variability in affinities and proportionate mixing is strong, we cannot distil the exact role of these preferences on the mixing patterns. Hence, in our preliminary efforts to gain further understanding, we explore, as a source of comparison, the role the following like-with-like affinity matrix on P. We repeat the above procedure with Φ
ii
having means 0.9 and Φ
ij
means 0.1, taking care not to exceed (0, 1) on either end (albeit this is probably not that relevant). Here very tight shall be
, tight
and very loose
. This represents a population group's desire to mix within their own group rather than with another.
In the case of like-with-like mixing (), we can see that the process from seems to represent, in the case of uniform φ distributions, a smoothing of the P distribution. Regardless of the uniform distribution the means of each hump, P
ii
and P
ij
, all seem to shift closer to 0.5, from their values at 0.9 and 0.1, respectively. Also the fact that
seems to be preserved with P. The shape of the P
ij
distribution seems to preserve affinity differences.
Figure 8. Histograms of the P ij under like-with-like affinity assumptions values top to bottom with the very tight, tight, and very loose parameter choices. We can observe a definite difference in shape between the uniform and beta shapes, but the first and second moments are roughly equivalent.
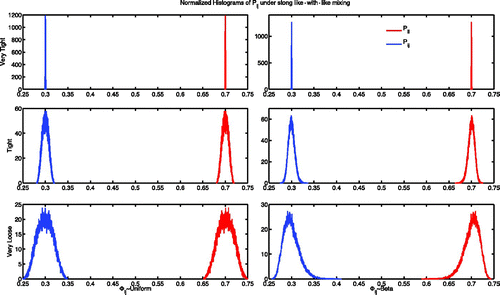
Given an affinity matrix Φ and thus a resulting mixing matrix P, we define the random matrix
Figure 9. Pictured here are the distributions of Δ ij . It is easily seen in (a) that the P formed via Φ being a deviation from a constant matrix have a nearness to proportionate mixing and that the variance on Φ weakens this nearness. The like-with-like mixing matrices, as seen in (b), have more or less a constant ‘distance’ from proportionate mixing.
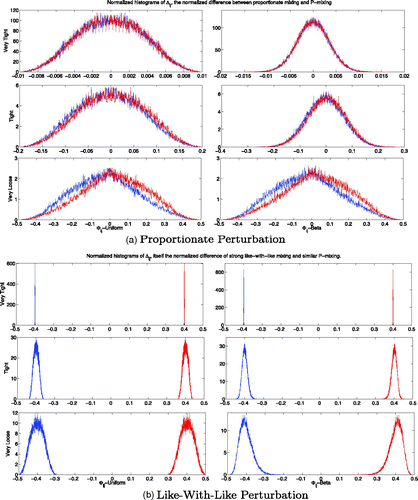
4. Application in epidemiology
The structure (including its contact structure) of a population plays a central role in the transmission dynamics of diseases, cultural traits, genetic traits, etc. Citation1 Citation2 Citation3 Citation8 Citation9 Citation10 Citation37 Citation38 Citation58. Extensive applications of the role of mixing in the context of HIV/AIDS have been carried out in the past. Some significant efforts include those found in Citation10 Citation24 Citation25 Citation30 Citation31 Citation33 Citation34 Citation40 Citation41 Citation42 Citation43 Citation44 Citation45 Citation47 Citation48 Citation52 Citation53 Citation54 Citation69 Citation70 Citation71 Citation73.
Here, we focus on the role of mixing in the simplest possible two-group SIS population. That is, affinity-based mixing is incorporated in a setting that can be easily used to carry out test-bed experiments that explore the role of preference and mixing on higher level processes, here an epidemic. We carry out numerical solutions of this model, a system of ODEs (Ordinary Differential Equation), over a large number of Φ realizations, and thus P realizations, in order to assess informally the impact of Φ-variability on the dynamics of S i (t) and I i (t) via the mixing matrix P.
4.1. System equations
Consider a two-group SIS system, with just enough recruitment in the susceptible class so as to maintain constant population size. In the absence of disease-induced mortality, we arrive at the following two-group, single-sex SIS epidemic model:
Here the parameter λ
i
models differential susceptibility; μ
i
, i=1, 2 denote the per capita death and recruitment rates; and denote the per capita recovery rates. The matrix P with entries P
ij
is the general solution of the mixing conditions and
is a symmetric matrix with
.
If we let C i =C and N i =N for i=1, 2 we arrive the simplified model that will be used in our simulations, namely
4.2. Epidemic calculations
It is assumed that disease contraction is difficult for subpopulation 1, recovery is a short process, and that the entry and removal into this subpopulation is moderate. Thus we assign , and
. We pair this subpopulation with a second subpopulation for which disease contraction is easier, recovery takes longer, and the entry and removal of new individuals are faster. Thus, we let
, and
. Finally, we set
which defines our baseline formulation which results in the proportionate mixing curves displayed in .
Figure 10. Plot of S 1, I 1, S 2, and I 2 with each patch having a total population of 1000 and each infectious class initialized with a single individual.
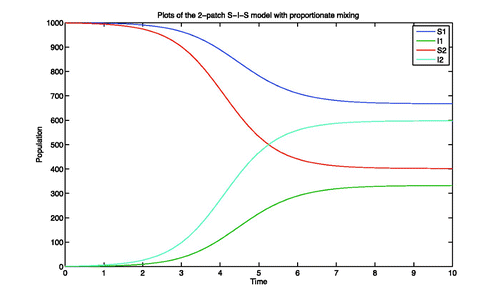
The perturbations of proportionate mixing via δ are explored. Hence, we let each φ
ij
be an i.i.d. uniform random variable on for i≤j and for i>j let
. Each solution is an instantiation of what effect the difference from proportionate mixing could have. collects the results of 1000 such repetitions of this idea under the tight parameter regime of (0.3,0.7). From this figure, we can see non-constant, and non-monotonic, variance along the time series for each system state variable.
4.3. Some results
We now explored how the variation in Φ impacts the model outcome and hence, arbitrarily, we subtract the trajectory of the S 1 state variable under proportionate mixing from each trajectory of S 1 under the effects of an instantiation of P, and thus Φ. All such trajectories in have the property that if by mid-epidemic they have values above those of proportionate mixing, then they will have lower endemic levels and vice versa. Of interest would be to classify this behaviour via Φ or P.
Figure 12. 1000 instantiations with α=0.5 and δ=0.2 represented via the difference from the mean of S 1.
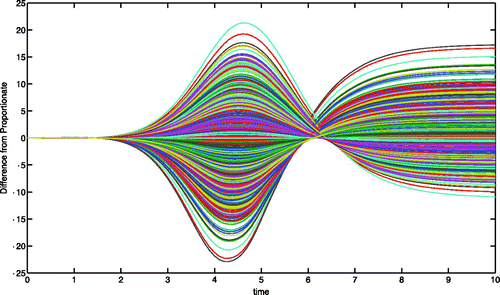
We proceed to collect all of the Φ’s that correspond to an endemic equilibrium below the mean (the ones that are above average at first) and call that Φ
b
and similarly define Φ
a
to be the collection of the matrices Φ such that endemic levels are above average. Now consider the determinant of a matrix . From our simulations, this determinant is always less than zero (i.e.
). This may imply that the mixing between unlike groups is greater than same-group mixing. For a great deal of the results, we have that
for
(i.e.
. That is, same-group mixing seems more likely than unlike-group mixing. It turns out that if the endemic susceptible population under some non-proportionate mixing, call it
, is less than the one corresponding to proportionate mixing, S
∞, there are a few cases, about 8% out of 10,000 in our preliminary simulations, where the determinant of the affinity matrix is negative but
as shown in .
Figure 13. This plot collects the eigenvalues of 10,000 Φ-affinity matrices coloured blue if the limiting size of S 1 under affinity-based mixing, [Stilde]1, was greater than that of S 1 under proportionate mixing and green otherwise. The red highlight those Φ matrices that have [Stilde]1>S 1 but det (Φ)<0. These matrices had a negative minimal eigenvalue, a characteristic dominated by those Φ such that [Stilde]1<S 1.
![Figure 13. This plot collects the eigenvalues of 10,000 Φ-affinity matrices coloured blue if the limiting size of S 1 under affinity-based mixing, [Stilde]1, was greater than that of S 1 under proportionate mixing and green otherwise. The red highlight those Φ matrices that have [Stilde]1>S 1 but det (Φ)<0. These matrices had a negative minimal eigenvalue, a characteristic dominated by those Φ such that [Stilde]1<S 1.](/cms/asset/5cd2d8a3-c85d-4f20-aec6-d5143a076e8f/tjbd_a_510212_o_f0013g.jpg)
If we instead consider the mixing matrices that result from each of these groups of affinity matrices, that is, P
a
and P
b
, it turns out that and
for every realization. This was verified over 200,000 realizations of the process without a single exception. This would seem to imply that systems under like-with-like dynamics, i.e. P
ii
≫P
ij
, are probably more likely to experience a lower infected population and hence, proportionate mixing (the narrow version used here may indeed correspond to the worst case scenario Citation26
Citation19). Thus we put forward the following hypothesis in the form of a proposition.
Proposition 4.1
Given a system of equations as defined in Equation (24) with the mixing matrix P=(P ij ), let [Stilde] i be the limiting susceptible population in group i under this mixing and S i be that under proportionate mixing. We have that
5. Discussion
This work revisits classical and relatively recent work on the problem of who mixes with whom, a problem of importance to those involved in the modelling and the study of the dynamics of interacting populations. We have conducted further numerical explorations on the role of affinity (what individuals want) in order to gain additional understanding of its role on mixing patterns (what individuals get). It is our hope that further theoretical and empirical research will be carried out on the questions raised in this manuscript (see Citation26, for recent contributions).
The focus here has been primarily on exploring the role of affinity or preference (what we want, Φ-matrix) via simulations on resulting mixing patterns (P-matrix). We have also proceeded to investigate numerically and in a rather simple setting, the role of affinity/mixing processes on disease dynamics. Several issues are highlighted including a hypothesis (presented in the form of a proposition) on the role of affinity at the epidemic level that we are exploring numerically in the context of an arbitrary number of interacting subpopulations. We are also interested in finding out what the eigenvalues of P tell us about processes utilizing P Citation26. The study of the role of selection on mixing patterns and consequently, on the evolution of affinities is also a question of interest that we may be able to pursue in this setting. However, in order to carry this out, the mixing must connect interacting subpopulations with differential vital rates due, for example, to disease-induced deaths.
We have also revisited some of our earlier work on two-sex mixing. In this manuscript, we have included mixing data that includes alcohol as a co-factor and have shown its effect on affinity-based mixing. It is known that alcohol is a key driver of the dynamics of sexually transmitted diseases, but not much modelling work has been done in this direction Citation63. Expanding the work outlined here in the context of a two-sex model involving two subpopulations, as in the one-sex SIS model, presents quite interesting challenges that we hope to pursue.
Acknowledgements
This project have been partially supported by grants from the National Science Foundation (NSF – Grant DMS – 0502349), the National Security Agency (NSA – Grant H98230 – 06-1-0097), the Alfred T. Sloan Foundation, and the Office of the Provost of Arizona State University. A special thank you to the editors and reviewers who have helped us craft a much better paper.
References
- Anderson , R. M. 1982 . Population Dynamics of Infectious Diseases: Theory and Applications , Edited by: Anderson , R. M. London, New York : Chapman and Hall .
- Anderson , R. M. and May , R. M. 1991 . Infectious Diseases of Humans , Oxford, New York : Oxford Science Publications .
- Bailey , N. T.J. 1975 . The Mathematical Theory of Infectious Diseases and Its Applications , London : Griffin .
- Blythe , S. P. and Castillo-Chavez , C. 1989 . Like-with-like preference and sexual mixing models . Math. Biosci. , 96 : 221 – 238 .
- Blythe , S. P. , Castillo-Chavez , C. , Palmer , J. and Cheng , M. 1991 . Towards a unified theory of mixing and pair formation . Math. Biosci. , 107 : 379 – 405 .
- Blythe , S. P. , Castillo-Chavez , C. and Casella , G. 1992 . Empirical methods for the estimation of the mixing probabilities for socially structured populations from a single survey sample . Math. Popul. Stud. , 3 ( 3 ) : 199 – 225 .
- Blythe , S. P. , Busenberg , S. and Castillo-Chavez , C. 1995 . Affinity and paired-event probability . Math. Biosci. , 128 : 265 – 284 .
- Busenberg , S. and Castillo-Chavez , C. 1989 . Interaction, Pair Formation and Force of Infection Terms in Sexually Transmitted Diseases , New York : Springer-Verlag . (Lecture Notes in Biomathematics 83)
- Busenberg , S. and Castillo-Chavez , C. 1991 . A general solution of the problem of mixing subpopulations, and its application to risk- and age-structured epidemic models for the spread of AIDS . IMA J. Math. Appl. Med. Biol. , 8 : 1 – 29 .
- Castillo-Chavez , C. 1989 . Mathematical and Statistical Approaches to AIDS Epidemiology (Lecture Notes in Biomathematics 83) , Edited by: Castillo-Chavez , C. New York : Springer-Verlag .
- Castillo-Chavez , C. and Busenberg , S. On the solution of the two-sex mixing problem . Proceedings of the International Conference on Differential Equations and Applications to Biology and Population Dynamics . Edited by: Busenberg , S. and Martelli , M. pp. 80 – 98 . Berlin-Heidelberg, New York : Springer-Verlag . Lecture Notes in Biomathematics 92
- Castillo-Chavez , C. and Hsu Schmitz , S.-F. 1997 . “ The evolution of age-structured marriage functions: It takes two to tango ” . In Structured-Population Models Marine, Terrestrial, and Freshwater Systems , Edited by: Tuljapurkar , S. and Caswell , H. 533 – 550 . New York : Chapman & Hall .
- Castillo-Chavez , C. , Busenberg , S. and Gerow , K. 1990 . “ Pair formation in structured populations ” . In Differential Equations with Applications in Biology, Physics and Engineering , Edited by: Goldstein , J. , Kappel , F. and Schappacher , W. 4765 New York : Marcel Dekker .
- Castillo-Chavez , C. , Fridman , S. and Luo , X. Stochastic and deterministic models in epidemiology . Proceedings of the First World Congress on Nonlinear Analysts . August 19–26 1992 , Tampa, Florida.
- Castillo-Chavez , C. , Velasco-Hernandez , J. X. and Fridman , S. 1993 . Modeling Contact Structures in Biology (Lecture Notes in Biomathematics 100) , New York : Springer-Varlag .
- Castillo-Chavez , C. , Huang , W. and Li , J. 1996 . On the existence of stable pair distributions . J. Math. Biol. , 34 : 413 – 441 .
- Castillo-Chavez , C. , Yakubu , A.-A. , Thieme , H. and Martcheva , M. 2002 . “ Nonlinear mating models for populations with discrete generations ” . In Mathematical Approaches for Emerging and Reemerging Infectious Diseases: An Introduction , Edited by: Castillo-Chavez , C. , van den Driessche , P. , Kirschner , D. and Yakubu , A.-A. Vol. 125 , 251 – 268 . Berlin–Heidelberg–New York : Springer-Verlag . IMA
- Caswell , H. September 2000 . Matrix Population Models: Construction, Analysis and Interpretation , 2 , September , Sunderland, Massachusetts : Sinauer Associates .
- Chowell , G. and Castillo-Chavez , C. 2003 . Worst Case Scenarios and Epidemics. In (Banks model building and evaluation . Can. J. Vet. Res. , 67 : 307 – 311 .
- Chowell , G. , Cintron-Arias , A. , Del Valle , S. , Sanchez , F. , Song , B. , Hyman , M. and Castillo-Chavez , C. 2006 . “ Mathematical applications associated to the deliberate release of infectious agents ” . In Mathematical Studies on Human Disease Dynamics: Emerging Paradigms and Challenges , Edited by: Gumel , A. , Castillo-Chavez , C. , Clemence , D. P. and Mickens , R. E. Vol. 410 , 51 – 72 . Rhode Island, Providence : American Mathematical Society .
- Chowell , G. , Hyman , J. M. , Bettencourt , L. M.A. and Castillo-Chavez , C. 2009 . Mathematical and Statistical Estimation Approaches in Epidemiology , Edited by: Chowell , G. , Hyman , J. M. , Bettencourt , L. M.A. and Castillo-Chavez , C. 370 Springer .
- Crawford , C. M. , Schwager , S. J. and Castillo-Chavez , C. 1990 . A methodology for asking sensitive questions among college undergraduates , Ithaca, NY : Cornell University . Tech. Rep. # BU-1105-M in the Biometrics Unit series
- Crow , J. F. and Kimura , M. 1970 . An Introduction to Population Genetics Theory , New York : Harper & Row .
- Dietz , K. 1988 . On the transmission dynamics of HIV . Math. Biosci. , 90 : 397 – 414 .
- Dietz , K. and Hadeler , K. P. 1988 . Epidemiological models for sexually transmitted diseases . J. Math. Biol. , 26 : 1 – 25 .
- Elsner , L. and Hadeler , K. P. October 1 2009 . The Spectral Radius and the Largest Basic Reproduction Number in Multi-type Epidemic Models with Mixing October 1 , Unpublished Manuscript
- Fredrickson , A. G. 1971 . A mathematical theory of age structure in sexual populations: Random mating and monogamous marriage models . Math. Biosci. , 20 : 117 – 143 .
- Goodreau , S. , Kitts , J. and Morris , M. 2009 . Birds of a feather, or friend of a friend? Using statistical network analysis to investigate adolescent social networks . Demography , 46 ( 1 ) : 103 – 125 .
- Gumel , A. , Castillo-Chavez , C. , Clemence , D. P. and Mickens , R. E. 2006 . Modeling the dynamics of human diseases: Emerging paradigms and challenges . Am. Math. Soc. , 410 : 389
- Hadeler , K. P. 1989 . Pair formation in age-structured populations . Acta Appl. Math. , 14 : 91 – 102 .
- Hadeler , K. P. 47th Session of the International Statistical Institute . August/September , Paris. Modeling AIDS in structured populations , pp. 83 – 99 . Conf. Proc., C1–2
- Hethcote , H. W. 2000 . The mathematics of infectious diseases . SIAM Rev. , 42 ( 4 ) : 599 – 653 .
- Hadeler , K. P. and Nagoma , K. 1990 . Homogeneous models for sexually transmitted diseases . Rocky Mountain J. Math. , 20 : 967 – 986 .
- Hethcote , H. W. and Van Ark , J. W. 1992 . Modeling HIV Transmission and AIDS in the United States (Lecture Notes in Biomathematics 95) , New York : Springer-Verlag .
- Hethcote , H. W. and Yorke , J. A. 1984 . Gonorrhea Transmission Dynamics and Control , Berlin, Heiderlberg, New York, London, Paris, Tokyo, , Hon Kong : Springer-Verlag .
- Hethcote , H. W. and Yorke , J. A. 1984 . Gonorrhea Transmission Dynamics and Control , New York : Springer-Verlag . (Lecture Notes in Biomathematics 56
- Hsu Schmitz , S.-F. 1993 . Some theories, estimation methods and applications of marriage functions and two-sex mixing functions in demography and epidemiology Unpublished doctoral dissertation, Cornell University, Ithaca, NY, USA
- Hsu Schmitz , S.-F. and Castillo-Chavez , C. 1994 . “ Parameter estimation in nonclosed social networks related to dynamics of sexually transmitted diseases ” . In Modelling the AIDS Epidemic: Planning, Policy, and Prediction , Edited by: Kaplan , E. H. and Brandeau , M. L. 533 – 559 . New York : Raven Press Ltd. .
- Hsu Schmitz , S.-F. and Castillo-Chavez , C. 2000 . A note on pair-formation functions . Math. Comput. Model. , 31 : 83 – 91 .
- Hyman , J. M. and Stanley , E. A. 1988 . Using mathematical models to understand the AIDS epidemic . Math. Biosci. , 90
- Hyman , J. M. and Stanley , E. A. 1989 . “ The effects of social mixing patterns on the spread of AIDS ” . In Mathematical Approaches to Problems in Resource Management and Epidemiology (Ithaca, NY, 1987) , Edited by: Castillo-Chavez , C. , Levin , S. A. and Shoemaker , C. A. 190 – 219 . Berlin : Springer . Lecture notes in Biomathematics, 81
- Hyman , J. M. and Stanley , E. A. 1994 . “ A risk-based heterosexual model for the AIDS epidemic with biased sexual partner selection ” . In Modeling the AIDS Epidemic , Edited by: Kaplan , E. and Brandeau , M. 331 – 364 . New York : Raven Press .
- Hyman , J. M. , Li , J. and Stanley , E. A. 2001 . The initialization and sensitivity of multigroup models for the transmission of HIV . J. Theor. Bio. , 208 : 227 – 249 .
- Hyman , J. M. , Li , J. and Stanley , E. A. 1999 . The Differential Infectivity and Staged Progression Models for the Transmission of HIV . Math. Biosci. , 155 ( 2 ) : 77 – 109 .
- Jacquez , J. A. , Simon , C. P. , Koopman , J. , Sattenspiel , L. and Perry , T. 1988 . Modeling and the analysis of HIV transmission: The effect of contact patterns . Math. Biosci. , 92 : 119 – 199 .
- Jewell , N. P. , Dietz , K. and Farewell , V. T. 1991 . AIDS Epidemiology: Methodological Issues , Boston, Basel, Berlin : Birkhäuser .
- Kaplan , E. H. 1989 . What Are the Risks of Risky Sex? . Oper. Res. , 37 : 198 – 209 .
- Kaplan , E. H. and Brookmeyer , R. 1999 . Snapshot estimators of recent HIV incidence rates . Oper. Res. , 47 : 29 – 37 .
- Kasseem , G. T. , Roudenko , S. , Tennenbaum , S. and Castillo-Chavez , C. 2006 . “ The role of transactional sex in spreading HIV/AIDS in Nigeria: A modeling perspective ” . In Mathematical Studies on Human Disease Dynamics: Emerging Paradigms and Challenges , Edited by: Gumel , A. , Castillo-Chavez , C. , Clemence , D. P. and Mickens , R. E. Vol. 410 , 367 – 389 . Rhode Island, Providence : American Mathematical Society .
- Kendall , D. G. 1949 . Stochastic processes and population growth . R. Statist. Soc. Ser. B , 2 : 230 – 264 .
- Keyfitz , N. The Mathematics of Sex and Marriage . Proceedings of the Sixth Berkeley Symposium on Mathematical Statistics and Probability . Vol. 4 , pp. 89 – 108 . Berkeley: California : University of California Press .
- Kirschner , D. 1996 . A diffusion model for AIDS in a closed, heterosexual populations: Estimating rates of infection . SIAM J. Appl. Math. , 56 : 143 – 166 .
- Koopman , J. , Simon , C. P. , Jacquez , J. A. , Joseph , J. , Sattenspiel , L. and Park , T. 1988 . Sexual partner selectiveness effects on homosexual HIV transmission dynamics . J. AIDS , 1 : 486 – 504 .
- Koopman , J. S. , Jacquez , J. A. , Welch , G. W. , Simon , C. P. , Foxman , B. , Pollock , S. M. , Barth-Jones , D. , Adams , A. L. and Lange , K. 1997 . The role of primary HIV infection in the spread of HIV . J. AIDS , 14 : 249 – 258 .
- Kretzschmar , M. 1995 . Deterministic and stochastic pair formation models for the spread of sexually transmitted diseases . J. Biol. Syst. , 3 : 789 – 801 .
- Leslie , P. H. 1945 . On the use of matrices in certain population mathematics . Biometrika , 33 : 183 – 212 .
- Lotka , A. J. 1922 . The stability of the normal age distribution . Proc. Natl Acad. Sci. , 8 : 339 – 345 .
- Lubkin , S. and Castillo-Chavez , C. A Pair Formation Approach to Modeling Inheritance of Social Traits . Proceedings of the First World Congress on Nonlinear Analysts . August , Tampa, FL. pp. 19 – 26 . Hawthorne, NJ : Walter de Gruyter & Co .
- Luo , X. and Castillo-Chavez , C. 1991 . Limit behavior of pair formation for a large dissolution rate . J. Math. Syst. Estimation Control , 3 ( 2 ) : 247 – 264 .
- Luo , X. , Castillo-Chavez , C. and Cheng , M. 1991 . A probabilistic model of pair formation , Ithaca, NY : Cornell University . Biometrics Unit Tech. Rep., BU-1145-M
- MacKendrick , A. G. 1926 . Applications of mathematics to medical problems . Proc. Edinb. Math. Soc. , 44 : 98 – 130 .
- McFarland , D. D. 1972 . “ Comparison of alternative marriage models ” . In Population Dynamics , Edited by: Greville , T. N.E. 89 – 106 . New York, London : Academic Press .
- Mubayi , A. , Greenwood , P. E. , Castillo-Chavez , C. , Gruenewald , P. and Gorman , D. M. 2010 . Impact of Relative Residence Times on the Distribution of Heavy Drinkers in Highly Distinct Environments . Socio-Econ. Plann. Sci. , 43 ( 1 ) : 1 – 12 .
- Newman , M. E.J. 2003 . The structure and function of complex networks . SIAM Rev. , 45 : 167 – 256 .
- Parlett , B. 1972 . “ Can there be a marriage function? ” . In Population Dynamics , Edited by: Greville , T. N.E. 107 – 135 . New York, London : Academic Press .
- Pollard , J. H. 1973 . Mathematical Models for the Growth of Human Populations , Cambridge : Cambridge University Press .
- Ross , R. 1911 . The Prevention of Malaria , 2 , London : John Murray . with addendum
- Rubin , G. , Umbauch , D. , Shyu , S.-F. and Castillo-Chavez , C. 1992 . Application of capture-recapture methodology to estimation of size of population at risk of AIDS and/or other sexually-transmitted diseases . Stat. Med. , 11 : 1533 – 1549 .
- Sattenspiel , L. 1989 . “ The structure and context of social interactions and the spread of HIV ” . In Mathematical and Statistical Approaches to AIDS Epidemiology , Edited by: Castillo-Chavez , C. 242 – 259 . Berlin : Springer-Verlag . Lecture Notes in Biomathematics 83
- Sattenspiel , L. , Koopman , J. , Simon , C. P. and Jacquez , J. A. 1990 . The effects of population subdivision on the spread of the HIV infection . Am. J. Phys. Anthropol. , 82 : 421 – 429 .
- Sattenspiel , L. and Castillo-Chavez , C. 1990 . Environmental context, social interactions, and the spread of HIV . Am. J. Hum. Biol. , 2 : 397 – 417 .
- Tennenbaum , S. 2006 . A two-sex, age-structured population model in discrete time , Ph.D. thesis, Cornell University
- Waldstätter , R. 1989 . Pair Formation in Sexually Transmitted Diseases (Lecture Notes in Biomathematics) , Vol. 83 , 260 – 274 . New York : Springer-Verlag .