Abstract
We postulate that the large difference in infection prevalence, 24% versus 5%, in R. norvegicus and R. rattus, respectively, between these two co-occurring host species may be due to differences in ectoparasite and potential vector infestation rates. A compartmental model, representative of an infectious system containing these two Rattus species and two ectoparasite vectors, was constructed and the coefficients of the forces of infection determined mathematically. The maximum difference obtained by the model in the prevalence of Bartonella in the two Rattus species amounts to 4.6%, compared to the observed mean difference of 19%. Results suggest the observed higher Bartonella infection prevalence in Rattus norvegicus compared to Rattus rattus, cannot be explained solely by higher ectoparasite load. The model also highlights the need for more detailed biological research on Bartonella infections in Rattus and the importance of the flea vector in the spread of this disease.
1. Introduction
More than 20 diseases caused by bacterial, viral and protozoal infections are spread to humans by rodents, either directly or indirectly Citation21. Due to their frequent association with human settlements, and status as natural reservoir of infections, they are often implicated in outbreaks of bacterial diseases, including plague (caused by Yersinia pestis), Lyme disease (Borrelia burgdorferi), Typhus (Rickettsia typhi) and the spotted fevers (Rickettsia spp.) Citation16 Citation51. The gram-negative bacterium, Bartonella, is no exception and rodents have been found to host several species, including Bartonella elizabethae, Bartonella grahamii, Bartonella tribocorum, Bartonella rattimassiliensis, Bartonella rattiaustraliani, Bartonella taylorii, Bartonella doshiae, Bartonella cooperplainensis, Bartonella queenslandensis and Bartonella phoceensis Citation2 Citation22 Citation32 Citation33. Although not all of these Bartonella species are associated with human illnesses, it has been suggested that most Bartonella species are capable of infecting humans and that lack of exposure to current reservoirs is the only obstacle preventing this Citation11. This is highlighted by the observation that some Bartonella infections in humans only came to light after their initial detection in rodents (e.g. B. grahamii, B. elizabethae and B. vinsonii arupensis) Citation27.
Invasive members of the Rattus genus, viz. Rattus rattus and Rattus norvegicus, are known to harbour Bartonella species with documented zoonotic potential Citation32. These two Rattus species, which, essentially have a worldwide distribution due to increased trade and favourable living conditions created by human presence, occur in South Africa Citation9. Hsieh et al. Citation26 report a 43% higher Bartonella prevalence in R. norvegicus than in R. rattus, while Ellis et al. Citation19 similarly show a 7.4% higher prevalence in the former species. Data on Bartonella in Rattus from South Africa concur with that of Northern hemisphere data, indicating that bacterial prevalence differs markedly in the two species Citation13 Citation42.
These differences in infection rates between R. norvegicus and R. rattus suggest a constantly higher infection prevalence in the former species despite the co-occurrence of both species in the countries under study. This is of interest as one would anticipate that related species with similar biologies that occur in the same area, would have comparable susceptibility to infection. This suggests some extrinsic factor is likely to be responsible for the observed differences in Bartonella prevalence in these two species. Therefore, an investigation into the dynamics of Bartonella infections in these rodents, linked to a mathematical epidemiological model for Bartonella infections in R. rattus and R. norvegicus was initiated.
More detailed investigation of the factors mediating Bartonella infections, revealed a lack of biological information on this infectious system. The available peer-reviewed studies on rodent hosts did, however, confirm the following: Equation(1)
Bartonella is a vector-borne bacterial infection transmitted by haematophagous arthropod vectors such as fleas and ticks Citation25
Citation35
Citation41
Citation45, Equation(2)
natural Bartonella infections seem to have little effect on the mortality of rodents (Citation19, Equation(3)
vertical transmission of Bartonella occurs in some rodent species Citation11
Citation19
Citation28, Equation(4)
individual rodents can display super-infection with different Bartonella species Citation53, Equation(5)
rodent hosts acquire immunity following exposure to a Bartonella species, but are not protected from subsequent infection by a different species Citation11 and Equation(6)
Bartonella can exhibit host-specificity although this has not been shown for Rattus specifically Citation1
Citation8
Citation25
Citation46.
Seasonal variation in Bartonella infections which could possibly be attributed to seasonal fluctuation of rodent and ectoparasite population numbers has been demonstrated for the Tunisian fat sand rat (Psammomys obesus, Citation20). Studies investigating the known and possible vectors of Bartonella (mostly ticks and fleas) show large variation in Bartonella infection rates Citation25 Citation35 Citation41 Citation45. These typically range from 5% to 40% in ticks (mainly Ixodidae) and between 13% and 60% for fleas (mainly Xenopsylla) Citation5 Citation12 Citation15 Citation23 Citation29 Citation41 Citation43 Citation45. Studies also suggest an age, sex and seasonal bias in the Bartonella infection rates of these blood-sucking arthropods Citation23 Citation25. Additionally, co-infection of different Bartonella species at a rate of 30% in ticks and a sex-biased co-infection rate in adult ticks of 44% in females compared to 11% in males has also been reported Citation23 Citation25.
To this end, the aim of this work is firstly, to study mathematically the relationships between the contributing factors of Bartonella infections in a commensal rat population in South Africa, in an effort to explain the differences in infections rates between two different species of Rattus with respect to their ectoparasite infestation levels. Secondly, this work aims to assess the most crucial factors and biological parameters for which information is presently lacking, that may prove vital in the understanding of Bartonella infections in carrier rodents and their consequent zoonotic threat to humans. To this end, this work will lay the foundation for directing future biological and epidemiological research.
2. The Bartonella model
Mathematical modelling has become a major tool for studying the evolution of infectious diseases in general Citation4 Citation17, and vector-borne diseases in particular Citation14 Citation18 Citation36 Citation37. The mathematical model developed for this study is in the form of a system of ordinary differential equations involving two hosts (R. rattus and R. norvegicus) and two vectors (Ixodid ticks (potential vector) and Xenopsylid fleas (known vector)). Although ticks have not been proven vectors of Bartonella, their ability to pick up the bacterium from the host has been confirmed Citation13 and this, along with available biological data (as opposed to other known vectors) justified the inclusion of this ectoparasite in the model. Considering this, both ectoparasites will be considered as vectors of the disease for the remainder of this paper. In constructing the model, we use the well-known SEI compartmental approach with three compartments for each population, namely susceptible (S), exposed (E) and infective (I); 12 compartments in total. The mathematical model, therefore, comprises 12 ordinary differential equations defining a dynamical system. The parameters of the model are related to the transfer rates to and from compartments as determined by the vital dynamics (birth and deaths) of the populations as well as the course of the infection. Numerical simulations confirmed the stability of final states of the model in the case of stable population sizes for both hosts and vectors and also in the case of periodic changes in the populations of the ectoparasite vectors. Furthermore, we computed sensitivity indices of the prevalence rates with respect to all parameters of the model which will aid in directing future research. Extended mathematical details for modelling of host and vector populations are detailed in Citation6.
2.1. Host
The host animal is specified to be a commensal population of co-occurring R. rattus and R. norvegicus in South Africa. Both species are invasive rodents in South Africa. R. norvegicus is typically larger, heavier and more aggressive than R. rattus Citation21 Citation49. They are found in out-buildings, stores and houses, occasionally nesting in well-covered natural habitats Citation7. They are nocturnal omnivorous pests that can live for up to two years. They show year-round reproductive ability in human populated areas, and are capable of producing litters of 5–10 pups every two months Citation7, corresponding to between 30 and 60 pups per female per year, under natural conditions. Differences between the two species mainly relate to their body size, their general behaviour and ectoparasite load. R. norvegicus spends most of its time on the ground, foraging and also nesting in self-constructed burrows in the soil Citation21 Citation49 Citation52. Possibly due to this ground-dwelling behaviour and their larger size, they are infected with up to 1.4 times more ectoparasites than R. rattus Citation21 Citation40 Citation49. R. rattus on the other hand, spends most of its time in trees and raised surfaces, especially in the presence of R. norvegicus, due to the aggressive nature of the latter larger rat. It is presumed this evasive behaviour limits the exposure to ectoparasites (especially ticks) which often search for their host on the ground where they hatch from eggs and molt Citation21.
If the numbers of the rodents are not controlled via natural predators or pest control measures, the rat population will be expected to increase exponentially. As this is generally not the case, we assume that the populations of the two species are in equilibrium. This means that the death rate and the birth rate, μ, are equal. We calculate μ by using the well-known Partial Differential Equation (PDE) population model Citation24:
Figure 1. Determining values, μ, for the birth and death rates of invasive Rattus from South Africa.
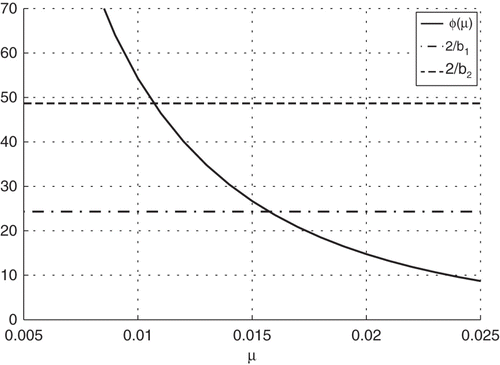
However, the change is small and within the round-off error to four significant figures. Therefore, we assume that the recruitment/death rate for each of the Rattus species is . Additionally, the assumption was made that rats can transmit Bartonella to their unborn offspring through vertical transmission Citation30. Experimental infection of rodents with low and high concentrations of Bartonella
Citation31 showed rodents to recover from Bartonella after about 6–9 weeks. This study also shows that the rodents become immune to challenge with the same species of Bartonella after recovery, but that they are susceptible to alternate Bartonella species, suggesting full recovery and potential re-infection. Added to this is evidence from the literature Citation1
Citation25
Citation46 of co-infections with different species of Bartonella in individual rodents – suggesting that a full recovery is not needed for super-infection by another Bartonella species. Given the above background, we considered the available data from South Africa on Bartonella diversity in Rattus
Citation13
Citation42, which suggests that the diversity of bacterial strains to which rats are potentially exposed is probably quite limited, with only three species of Bartonella documented in rats thus far Citation13
Citation42 and one of the three pre-dominating with a prevalence of 85% of infected rats. Therefore, we propose that if a rat in South Africa recovers from a Bartonella infection, although it is likely to be exposed to Bartonella again, it is very unlikely that it would be exposed to a different species which it would be susceptible to. We, therefore, modelled the reduced probability of being re-infected as shown in Section 2.4.
2.2. Flea vector
Rodent fleas mostly belong to the genus Xenopsylla that will hatch from eggs within 2–12 days and undergo three moults before pupating into adults Citation44. The larvae of fleas are rarely parasitic and feed on organic debris in the burrow/nest of the host Citation34, and are therefore, not considered vectors of Bartonella. After taking a blood meal, females can lay up to 50 eggs per day Citation44 Citation48. On average, these fleas will live up to one year, which may or may not include an extended pupal phase, depending on environmental factors Citation48. Due to temperature and humidity constraints on development, adult fleas exhibit a defined periodic abundance, which falls within the spring and summer months that run from September to February in South Africa Citation44 Citation48. As adult females produce an enormous number of eggs, it can safely be assumed that the amount of new recruits in the epidemiologically relevant population does not depend on its current population size, but only on the prevailing environmental conditions at the given time which we model through the carrying capacity of the environment. The epidemiologically relevant population consists of all adult fleas that feed on the population of rats that we consider. From this, we can make the inevitable assumption that this population is to a large extent separated from the rest of the fleas in the world. Therefore, the maximum carrying capacity is determined by the maximum (on time) average (over the modelled rat population) number of fleas and/or ticks per rat. Here, we assume that about 10% of adult fleas can be between hosts at any time. The transition between maximum abundance during spring and summer and low abundance in autumn and winter (set at about 20%) is modelled by using a periodic environmental carrying capacity of the form
The size N F of the flea population is obtained from the differential equation
We should remark that with seasonal change of the environment, the average lifespan is much shorter than one year since the death rate can be significantly higher than in adverse conditions (over-capacity). Irrespective of its initial size, the population of fleas reaches a stable periodical cycle within a year, as shown in , where the seasonal amount of fleas on a 1000-strong population of each of the Rattus species is plotted. Here and in the sequel, we use
.
2.3. Tick vector
Ticks of the family Ixodidae are known carriers of Bartonella and considered potential vectors in most areas Citation10 Citation15 Citation23 Citation29 Citation41 Citation45 Citation50. The Ixodid tick population exhibits a three-host life cycle in which the immatures only feed on murid rodents mainly during the dry or winter months Citation38 Citation55. The adults of these ticks parasitize larger hosts such as cattle, ungulates, goats and domestic cats and dogs Citation38 Citation55. In South Africa, these fall within the genera Rhipicephalus, Haemaphysalis, Hyalomma and Ixodes of the family Ixodidae (hard ticks) Citation38 Citation55. They commonly display adult activity in the spring and summer months of the year, while larvae and nymphs are active during the autumn and winter months Citation38 Citation55. In this three-host life cycle, six-legged larvae will hatch on the ground from eggs, find a suitable host (typically a rodent) and attach for about a week. Once engorged, the larvae will drop from the host, moult on the ground and after a few days, will find a second host to attach to Citation38 Citation55. They will remain on this host for about a week, and once engorged, drop to the ground to moult to adults Citation38 Citation55. These adults will locate a larger, non-rodent host after a couple of days, attach, mate and once the female is engorged, it will drop to the ground to lay a single batch of about 2000–5000 eggs Citation38 Citation55. The female will die after oviposition, while males can remain on the host for several months, sometimes switching between individuals of the same host species Citation38 Citation55.
Under natural conditions, ticks show a very pronounced periodic cycle of abundance due to their alternate adult host preference. In this case, where the nymphs specifically are being modelled, there is a marked increase of larvae and nymphs in the dry cold months in South Africa Citation38
Citation55. These stages decrease dramatically during the wet warm months of the year, which represents the dominant time for adult ticks Citation38
Citation55. Thus, we should observe a high abundance of larvae and nymphs on rodents during winter, and a low abundance in summer when adult ticks can be found in high numbers on larger mammals Citation38
Citation55. The epidemiologically relevant part of the population, namely the immature nymphs, is modelled in the same way as the fleas, but the cycle is shifted six months due to their high abundance in the dry cold months in South Africa Citation38
Citation55 rather than in the summer months as for fleas. Furthermore, individuals are transferred out of the population not only due to death but also due to maturation. Hence, the rate of removal from the population is the sum of the death rate μT and maturation rate , the latter reflecting a duration of the larval stage of the tick of about 42 days Citation38
Citation55. In the absence of any precise data, we take rather arbitrarily
. However, due to its relatively smaller contribution to the removal rate, it is expected that the possible error in the value of μT does not have a significant impact.
2.4. The model
We assumed no significant difference between the level of ectoparasite infection on male and female rats Citation39. Although there is evidence of increased ectoparasite infection on juvenile rats, this was assumed to be constant for the sake of simplicity Citation39. Although it has been suggested that there is an age, sex and seasonal bias in the infection rates of blood-sucking arthropods with Bartonella Citation23 Citation25, we also assumed no such bias, again, for the sake of simplicity. The assumption was made that vertical transmission of Bartonella occurs in rats, but not in the tick or flea vector Citation30 and that Bartonella does not significantly alter the death rate of either the rats or their ectoparasites Citation11 Citation19 Citation28. The four populations are compartmentalized using the usual SEI approach with S, E and I denoting the number of susceptible, exposed (carriers not yet infective) and infective individuals, respectively. The indices denote the species (r for R. rattus, n for R. norvegicus, T for ticks and F for fleas). The compartmental structure with the epidemiological flow between the compartments is presented in . A key issue in the modelling of diseases are the transfer rates from susceptible compartments to exposed compartments, also known as the forces of infection. In order to model these transfer rates, we apply the method of standard incidence Citation24. Suppose, for simplicity that there is one host, for example, R. rattus and one vector, for example, flea, then the force of infection acting on the host population should be proportional to the number of fleas per rat and the prevalence of the infection among the fleas resulting in a transfer from S r to E r of the form
Figure 4. Compartmental structure and epidemiological flow between the compartments of Bartonella infections in Rattus.
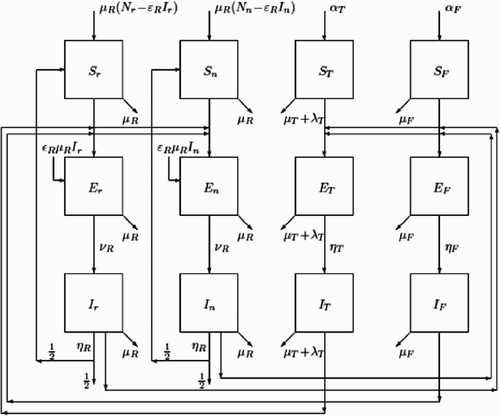
In the more complicated situation of two host species, we apply the same approach as in EquationEquations (4) and Equation(5)
, but taking into account that the flea population is split between the two hosts in the ratio
. We have
The forces of infection related to the tick population are modelled in the same way using constants σT and θT. The transfer rates from exposed to infective reflects the latent period of the infection for the respective species. The removal from each population is proportionally applied to all compartments. The recruitment in a population is placed in the respective susceptible compartment except for the host species where, due to vertical transmission, a certain proportion is placed in the exposed compartment. Once infective, the fleas and ticks remain so for the rest of their lives.
The rats, however, recover in 42 days and are no longer infective Citation30. Rodents also acquire life-long immunity against the particular strain of Bartonella they have been infected with. Since there are two dominant types of Bartonella in South African Rattus (a third type has only a marginal distribution) Citation13
Citation42, a rat which is recovered from one infection can be infected again with the other Bartonella species. Instead of complicating the model, we assume that on the recovered, there is a force of infection which is 1/2 of the original one or alternatively that 1/2 of the recovered return to the susceptible population. A compartment for the recovered is not explicitly included since it does not interact with any other compartment. However, the number of recovered can always be obtained as or
for each one of the host species. A complete list of the parameters, short description and values are given in .
Table 1. Model parameters and baseline values for Bartonella in Rattus.
In our search for biological data, we aimed for values obtained from peer-reviewed studies where there was evidence of co-habitation of R. rattus and R. norvegicus. Flea infestation data were obtained from Citation21
Citation49 where R. rattus and R. norvegicus co-occur. Values in these studies ranged from –12.6 and
–10.42 fleas per rat. The average values were used to determine the difference in infestation rates between the two Rattus species, which amounted to a factor of 1.4. The average number of ticks found on R. rattus, was obtained from Citation3
Citation29
Citation54 and corresponds to 0.2 ticks for every rat collected. As no biological data are available for tick infestation rates in R. norvegicus, we therefore extrapolated the number of ticks per R. norvegicus based on the factor of difference between flea infestation numbers in R. rattus and R. norvegicus. On this basis, the calculated tick infestation data for R. norvegicus was calculated to be 0.28
.
Preliminary prevalence estimates of Rattus–Bartonella infections in South Africa concur with prevalence estimates for these two species found in the literature, when one considers those studies that investigated both species using the same methodology and for which 10 or more animals were screened, viz. Citation13 Citation19 Citation26 Citation42. Together, the preliminary South African studies yield prevalence estimates of 24% for R. norvegicus and 5% for R. rattus (i.e. nearly a five-fold difference in prevalence between the two host species). These values were used in this model and were obtained for co-occurring species in South Africa Citation13 Citation42. Infection rates of ticks and fleas were obtained as far possible from studies that removed these ectoparasites from rats, and where possible, were limited to studies where the two Rattus species co-occur. This produces an infection estimate of 4.4% Citation29 and 61% for Xenopsylid fleas Citation10.
Bartonella seems to have little effect on the mortality rates of rodents and several authors have suggested infections may be maintained in the population by vertical transmission from mother to offspring in the womb Citation11
Citation19
Citation28. Vertical transmission was, therefore, included in our model, and the parameter value based on the study by Kosoy et al.
Citation31, where 48% of rodent neonates were shown to be infected with Bartonella through their mother before birth. We assume that at birth, the infection is at the start of its progression. Hence, the affected neonates are place in the exposed compartment of infected but not yet infective individuals. Experimental infection done by Kosoy et al.
Citation31 shows that bartonellae can be detected from the blood of rodents after seven days of incubation. Using this value, we obtain a probability of for a rat becoming infective. The probability of an exposed tick becoming infective is calculated based on the same principle as the corresponding parameter for rats. The incubation period of the bacteria in ticks, unfortunately, has no experimental substantiation.
For the purpose of this model however, we have considered the life cycle of these ticks. These ticks feed on a single host for a week at a time, and only after molting will attach to a new host and have the ability to spread the disease Citation38
Citation55. Although the incubation period is likely to be much shorter than that in rats (due to lack of immune response), the tick can only transmit the pathogen after about two weeks due to its method of feeding. From this, the actual incubation time in the ticks is not important for our model, and we can assume an ‘incubation period’ of 14 days before a tick becomes ‘infective’. This results in a probability of . There is also no scientific data available for the time, it takes for Bartonella to incubate in a flea, that is, the time it takes for an exposed flea to become infective. This parameter was, therefore, estimated and tested with a sensitivity analysis.
The mathematical model describing the flow in the diagram on is the following system of 12 differential equations defining a dynamical system on the non-negative cone of ℝ12:
3. Numerical simulations
A numerical solution of the system (9)–(20) can be obtained with any prescribed accuracy through a large variety of methods. We use the Matlab procedure which implements a fourth-order Runge–Kutta method with automatic step-size adjustment. Note that at this stage, we do not know the coefficients σT, σF, θT and θF of the forces of infection. Nevertheless, numerical simulations indicate that for all values of these coefficient, in some realistic range, the solution of Equations (9)–(20) approaches a limit cycle (periodic solution) as time increases. Typical results are shown in which are plotted from the numerical solutions in two extreme cases:
In both cases, we consider a 1000-strong population of each of the Rattus hosts and the values of the coefficients of the forces of infection are arbitrarily set to 0.01. We observe that in 3–4 years, the solution sets into a periodic pattern which is eventually the same in both cases.
Due to the arbitrary values of σT, σF, θT and θF in this simulation, one cannot claim any strong biological relevance of the concrete values of the solution. However, the important point here is their qualitative behaviour. The empirical evidence suggests that for any set of values of σT, σF, θT,θF and initial population sizes, the model (9)–(20) admits a stable limit cycle so that for any non-zero initial infection prevalence, the respective solution of Equations (9)–(20) approaches this limit cycle.
4. Determining the unknown parameters
The parameters σT, σF, θT and θF reflect unknown characteristics of the infection transmission such as the probability of transmission to or from the host over a single blood meal. Since currently there are no data from which the values of these parameters can be calculated directly, we use other observable data, namely the average infection prevalence in the two host species, R. rattus and R. norvegicus and two vectors, Ixodid ticks and Xenopsylid fleas (see ).
Table 2. Observed average infection prevalence for two Rattus host species and two ectoparasite vectors.
For simplicity in the notation, let q be a vector of the unknown parameters and let y be the vector of the state variables, that is and
. Then the system (9)–(20) can be written in the form
Then, the most suitable values of the unknown parameters are determined via an optimization problem: find a value of q which minimizes the function
Figure 8. Prevalence rates over time for optimal value of parameters estimated for Bartonella infections in Rattus and two vectors (Ixodid ticks and Xenopsylid fleas).
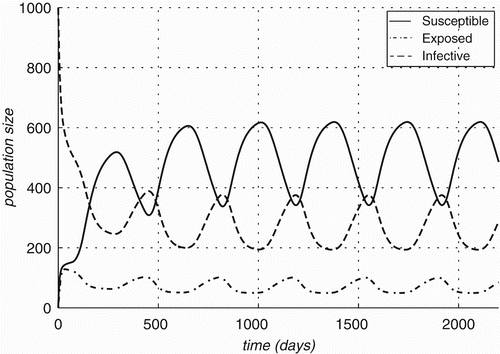
Table 3. Optimal coefficients and model prevalence rates.
One can observe the high oscillations in the infection prevalence in the flea population, oscillations of lesser amplitude in the infection prevalence of the rodent populations and almost no oscillation in the infection prevalence of the ticks (see ). This leads to the conclusion that the fleas are the primary vector in the sylvatic cycle of Bartonella. Similar conclusions can also be obtained by calculating the forces of infection for the obtained values of the parameters. However, it should be noted that this model does not explain the large difference in the observed infection prevalence between R. rattus and R. norvegicus. While the average infection prevalence of the two host species combined is captured in the model (14.5% average in data versus 15% average for model), the difference in the infection prevalence between the two host species captured by the models is only 3.3% compared to the observed difference between the two of nearly 19%. Some of the difference can be explained when we assume that the populations of the ectoparasites living on the two host species are to some extent separated. When modelled so, this leads to higher prevalence rates of the ectoparasite in R. norvegicus than the corresponding rates of those on R. rattus. The exact values of prevalence rates for the same values of the parameters are given in with the respective plots against time presented in . The difference of 4.6% shown by this version of the model is still much smaller than the observed difference in infection rates.
Figure 9. Bartonella infection prevalence rates for (a) R. rattus and (b) R. norvegicus and their respective ectoparasite populations as simulated by the mathematical epidemiological model.
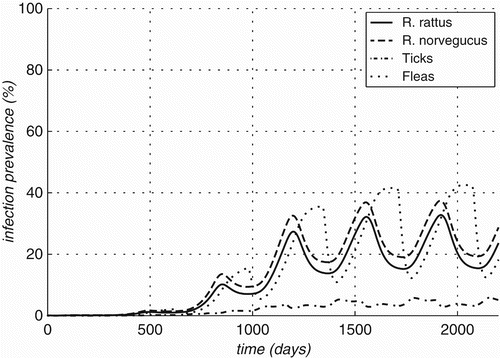
Table 4. Prevalence rates in the model of two Rattus hosts with disjunct vector populations.
This raises the question of the validity of the assumption in the model that the two rodent species are similar enough physiologically and behaviourally, that the probability of infection and the durations of exposure and recovery are the same. While indeed this is a fundamental assumption in the model, we need to note that its construction is based on little biological data. Verifying the optimal values of the coefficients obtained here would be a difficult task but the model gives predictions which may be easier to check experimentally. These include, for example, the periodicity of the infection prevalence in all host and vector species but more importantly in the fleas. If this periodicity is confirmed, then the relevant data will provide more complete information for refining the model and thus increasing the reliability of predictions.
Further research can also be guided by the sensitivity of the observable variables, namely the infection prevalences, on the parameters of the model. We characterize the sensitivity of the prevalence rates in terms of sensitivity indices. Let us recall that the sensitivity index of a variable u on a parameter q is defined as the relative change of u over the relative change of q, that is, . Naturally, the change of q is assumed to be small. If u is a differentiable function of q then letting
the index is simply
. The sensitivity indices of the four infection prevalence rates with respect to the parameters of the model at their baseline values used thus far, are given in .
Table 5. Sensitivity of the infection prevalence of Bartonella in Rattus on the model parameters at their baseline values.
Values with the largest impact on the parameters of the model are highlighted as: Equation(1) the recovery rate of the rats from Bartonella infection, Equation(2)
the flea infestation levels on each rat species and Equation(3)
the force of infection from fleas to rats (). These should be investigated biologically to confirm their importance.
5. Discussion
Understanding the dynamics of zoonotic diseases provides the ability to put appropriate control measures in effect that will limit the potential for transmission at the rodent–human interface. Therefore, the large difference in Bartonella infection rates between two co-occurring commensal Rattus species in South Africa is an interesting phenomenon that is of veterinary and medical interest. Differences in the ectoparasite infestation rates (mainly fleas) between R. rattus and R. norvegicus has been proposed and observed for many years Citation21, and we would assume higher ectoparasite (vector) infestation rates would logically lead to higher Bartonella infection rates in R. norvegicus, as is observed biologically. Due to the ease of mathematical versus biological experimentation, this model was developed in order to assess if the proposed ectoparasite variation could fully explain the higher Bartonella infection prevalence in South African R. norvegicus. Construction of the model was based on two invasive Rattus species occurring in South Africa, and available and relevant peer-reviewed literature on the pathogen and vertebrate host.
The model revealed a striking need for more detailed biological research on Bartonella infections in Rattus and their role as carriers of this bacteria. Available data on the tick indices of rats, incubation periods of Bartonella in both vector and host as well as the forces of infection are for the most part not known. The likelihood of acquiring Bartonella per vector bite for the vector or the host has also not been investigated, as has the probability of recovering from Bartonella infection for the host. As mentioned before, data concerning the forces of infection (the likelihood of transmitting Bartonella per tick bite/feeding episode) is also needed. The model, however, allowed several interesting conclusions to be drawn regarding the epidemiological cycle of Bartonella in Rattus.
From the model, one can observe the importance of the flea vector of Bartonella due to the high oscillations in the infection prevalence in this ectoparasite population (see ) and from the results of the sensitivity analysis (). The sensitivity analysis indicates that the number of fleas per rat as well as the force of infection from these fleas are important in determining the infection rates of the rats (). This leads to the conclusion that the fleas are the primary vector in the infectious cycle of Bartonella within the setting of this model, possibly due to their high mobility. The contribution of fleas to the infection of Rattus with Bartonella should, therefore, be investigated further, especially since fleas have been implicated in the zoonotic spread of other diseases such as plague. Furthermore, the sensitivity analysis also indicated the importance of the rate of recovery of the rats from Bartonella infection. Logically, the faster the recovery rate, the more resistant the population will be to high infection levels of Bartonella, since individuals recover relatively quickly. In our model, however, the rates of recovery were assumed to be constant for the two rat species due to their assumed similar biologies. Whether they in fact recover at different rates will have to be investigated and may provide a better understanding of the differences in infection prevalences between the two rat species. As expected, the model strongly depends on the coefficients of the forces of infection. However, our result is that by varying these coefficients alone, the model prevalence in the Rattus species does not approach the observed biological values. also reveals strong dependence of the prevalence rates on the recovery rate of Rattus. This suggests further investigation into the infectiveness period for each species because any difference between the two species in this regard is likely to explain easily the difference in the prevalence rate. Differential susceptibility of rodent hosts to Bartonella infection can also not be overlooked.
Difference in ectoparasite infestation rates between the two Rattus species did not explain the observed difference in Bartonella infection prevalence between R. rattus and R. norvegicus. With the assumed shared ectoparasite population between the two rat populations, the maximal difference in infection prevalence that could be obtained with the model was about 3% compared to the observed 19%. When the ectoparasite populations are assumed to be disjunct, due to R. rattus avoiding R. norvegicus, the maximal difference in infection prevalence that could be obtained was almost 5%, which does explain all the variation we see in nature (see and ).
There are thus additional factors involved in the infectious cycle of Bartonella in rodents that are not obvious. These may include a variety of factors such as the physiology and behaviour of the host and vector or presence of other competing bacteria Citation47. Most importantly, this emphasizes the need for more detailed and extensive research on this, and other emerging zoonotic pathogens, and sets the foundation for directing future research.
Acknowledgements
We gratefully acknowledge the National Research Foundation (NRF), for funding the biological work for this study through a Focus Area grant awarded to ADSB. Support of individual researchers through their associated institutions is gratefully acknowledged.
References
- Abbot , P. , Aviles , A. E. , Eller , L. and Durden , L. A. 2001 . Mixed infections, cryptic diversity, and the host . J. Parasitol. , 87 : 545 – 553 .
- Agan , B. K.D. and Dolan , M. J. 2002 . Laboratory diagnosis of Bartonella infections . Clin. Lab. Med. , 22 : 937 – 962 .
- Ajayi , O. O. , Ogwurike , B. A. , Ajayi , J. A. , Ogo , N. I. and Oluwadare , A. T. 2006 . Ectoparasites of rodents caught around human habitats n Jos, Plateau State, Nigeria . Nig. Ann. Nat. Sci. , 6 : 11 – 19 .
- Anderson , R. M. and May , R. M. 1991 . Infectious Diseases of Humans: Dynamics and Control , Oxford : Oxford University Press .
- Angelakis , E. , Billeter , S. A. , Breitschwerdt , E. B. , Chomel , B. B. and Raoult , D. 2010 . Potential for tick-borne Bartonelloses . Emerg. Infect. Dis. , 16 : 385 – 391 .
- Anguelov , R. , Brettschneider , H. and Bastos , A. D.S. 2011 . A case of multi-vector and multi-host epidemiological model: Bartonella infection . AIP Conf. Proc. , 1301 : 175 – 187 .
- Apps , P. 2000 . Smithers’ Mammals of Southern Africa: A Field Guide , Cape Town , , South Africa : Struik .
- Bastos , A. D.S. 2007 . Bartonella incidence and diversity in endemic South African Murid rodents occurring commensally with humans , 1 – 7 . The Southern African Society for Veterinary Epidemiology and Preventive Medicine .
- Bastos , A. D.S. , Chimimba , C. T.C. , von Matltitz , E. , Kerstin , F. and Belmain , S. 2005 . Identification of rodents species that play a role in disease transmission to humans in South Africa , 78 – 83 . The Southern African Society for Veterinary Epidemiology and Preventive Medicine .
- Beati , L. , Ellis , B. A. , Rood , M. , Eldaieef , S. and Regnery , R. L. First International Conference – Bartonella as Emerging Pathogens , pp. 34 abstract 13
- Birtles , R. J. , Hazel , S. M. , Bennet , M. , Bown , K. , Raoult , D. and Begon , M. 2001 . Longitudinal monitoring of the dynamics of infection due to Bartonella species in UK woodland rodents . Epidemiol. Infect. , 126 : 323 – 329 .
- Bitam , I. , Dittmar , K. , Parola , P. , Whiting , M. F. and Raoult , D. 2010 . Fleas and flea-borne diseases . Int. J. Infect. Dis. , 14 : 667 – 676 .
- Brettschneider , H. 2010 . “ Bartonella dynamics in indigenous and invasive murid rodents from South Africa: Prevalence, diversity and mathematical modeling ” . University of Pretoria, South Africa . Ph.D. diss.
- Chitnis , N. and Cushin , J. M. 2006 . Hyman bifurcation analysis of a mathematical model for Malaria transmission . SIAM J. Appl. Math. , 67 : 24 – 45 .
- Chomel , B. B. , Boulouis , H. J. , Maruyama , S. and Breitschwerdt , E. B. 2006 . Bartonella spp. in pets and effect on human health, . Emerg. Infect. Dis. , 12 : 389 – 394 .
- Davis , S. , Calvet , E. and Leirs , H. 2005 . Fluctuating rodent populations and risk to humans from rodent-borne diseases . Vector Borne Zoonotic Dis. , 5 : 305 – 314 .
- Diekmann , O. and Heesterbeek , J. A. 2000 . Mathematical Epidemiology of Infectious Diseases , Chichester : Wiley .
- Dumont , Y. , Chiroleu , F. and Domerg , C. 2008 . On a temporal model for the Chikungunya disease: Modeling, theory and numerics . Math. Biosci. , 213 : 70 – 81 .
- Ellis , B. A. , Regnery , R. L. , Beati , L. , Bacellar , F. , Rood , M. , Glass , G. G. , Marston , E. , Ksiazek , T. G. , Jones , D. and Childs , J. E. 1999 . Rats of the genus Rattus are reservoir hosts for pathogenic Bartonella species: An Old World origin for a New World disease? . J. Infect. Dis. , 180 : 220 – 224 .
- Fichet-Calvet , E. , Jomaa , I. , Ismail , B. and Ashford , R. W. 2000 . Patterns of infection of haemoparasites in the fat sand rat, Psammomys obesus, in Tunisia, and effect on the host . Ann. Trop. Med. Parasitol. , 94 : 55 – 68 .
- Gratz , N. G. 1997 . The burden of rodent-borne diseases in Africa south of the Sahara . Belgian J. Zool. , 127 : 71 – 84 .
- Gundi , V. A.K.B. , Davoust , B. , Khamis , A. , Boni , M. , Raoult , D. and La Scola , B. 2009 . Isolation of Bartonella rattimassiliensis sp. Nov. and Bartonella phoceensis sp. nov. From European Rattus norvegicus . J. Clin. Microbiol. , 42 : 3816 – 3818 .
- Halos , L. , Jamal , T. , Maillard , R. , Girard , B. , Guillot , J. , Chomel , B. , Vayssier-Taussat , M. and Boulouis , H. 2004 . Role of Hippoboscidae flies as potential vectors of Bartonella spp. infecting wild and domestic ruminants . Appl. Environ. Microbiol. , 70 : 6302 – 6305 .
- Hethcote , H. W. 2000 . The mathematics of infectious disease . SIAM Rev. , 42 : 599 – 653 .
- Holden , K. , Boothby , J. T. , Kasten , R. W. and Chomel , B. B. 2006 . Co-detection of Bartonella henselae, Borrelia burgdorferi, and Anaplasma phagocytophilum in Ixodes pacificus ticks from California . Vector Borne Zoonotic Dis. , 6 : 99 – 102 .
- Hsieh , J. W. , Tung , K. C. , Chen , W. C. , Lin , J. W. , Chien , L. J. , Hsu , Y. M. , Wang , H. C. , Chomel , B. B. and Chang , C. C. 2009 . Epidemiology of Bartonella infections in rodents and shrews in Taiwan , Zoonoses and Public Health .
- Jardine , C. , Appleyard , G. , Kosoy , M. Y. , McColl , D. , Chirino-Trejo , M. , Wobeser , G. and Leighton , F. A. 2005 . Rodent-associated Bartonella in Saskatchewan, Canada . Vector Borne Zoonotic Dis. , 5 : 402 – 409 .
- Jones , R. T. , McCormick , K. F. and Martin , A. P. 2008 . Bacterial communities of Bartonella-positive fleas: Diversity and community assembly patterns . Appl. Environ. Microbiol. , 74 ( 5 ) : 1667 – 1670 .
- Kim , C. M. , Kim , J. Y. , Yi , Y. H. , Lee , M. J. , Cho , M. R. , Shah , D. H. , Klein , T. A. , Kim , H. C. , Song , J. W. , Chong , S. T. , O'Guinn , M. L. , Lee , J. S. , Lee , I. Y. , Park , J. H. and Chae , J. S. 2005 . Detection of Bartonella species from ticks, mites and small mammals in Korea . J. Vet. Sci. , 6 : 327 – 334 .
- Kosoy , M. Y. , Regnery , R. L. , Kosaya , O. I. , Jones , D. C. , Marston , E. L. and Chllds , J. E. 1998 . Isolation of Bartonella spp. from embryos and neonates of naturally infected rodents . J. Wildl. Dis. , 34 : 305 – 309 .
- Kosoy , M. Y. , Regnery , R. L. , Kosaya , O. I. and Childs , J. E. 1999 . Experimental infection of cotton rats with three naturally occurring Bartonella species . Journal of Wildl. Dis. , 35 : 275 – 284 .
- Kosoy , M. , Mandel , E. , Green , D. , Marston , E. and Childs , J. 2004 . Prospective studies of Bartonella of rodents. Part I. Demographic and temporal patterns in population dynamics . Vector Borne Zoonotic Dis. , 4 : 285 – 295 .
- Kosoy , M. , Mandel , E. , Green , D. , Marston , E. and Childs , J. 2004 . Prospective Studies of Bartonella of Rodents. Part II. Diverse infections in a single rodent community . Vector Borne Zoonotic dis. , 4 : 296 – 305 .
- Krasnov , B. R. , Khokhlova , I. S. and Shenbrot , G. I. 2004 . Sampling fleas: The reliability of host infestation data . Med. Vet. Entomol. , 18 : 232 – 240 .
- Loftis , A. D. , Reeves , W. K. , Szumlas , D. E. , Abbassy , M. M. , Helmy , I. M. , Moriarity , J. R. and Dasch , G. A. 2006 . Population survey of Egyptian arthropods for Rickettsial agents . Ann. N. Y. Acad. Sci. , 1078 : 364 – 367 .
- Lotka , A. J. 1923 . Contribution of the analysis of malaria epidemiology . Am. J. Hyg. , 3 ( Suppl. 1 ) : 1 – 21 .
- Macdonald , G. 1957 . The Epidemiology and Control of Malaria , London : Oxford University Press .
- Matthee , S. and Krasnov , B. R. 2008 . Searching for generality in the patterns of parasite abundance and distribution: Ectoparasites of a South African rodent, Rhabdomys pumilio . Int. J. Parasitol. , 39 : 781 – 788 .
- Milazzo , A. , Gouy De Bellocq , J. , Cagnin , M. , Casanova , J. , Di Bella , C. , Feliu , C. , Fons , R. , Morand , S. and Santalla , F. 2003 . Research note, helminths and ectoparasites of Rattus rattus and Mus musculus from Sicily Italy . Comp. Parasitol. , 70 : 199 – 204 .
- Mohr , C. O. 1961 . Relation of ectoparasite load to host size and standard range . J. Parasitol. , 47 : 978 – 984 .
- Morozova , O. V. , Cabello , F. C. and Dobrotvorsky , A. K. 2004 . Semi-nested PCR detection of Bartonella henselae in Ixodes persulcatus ticks from Western Siberia, Russia . Vector Borne Zoonotic Dis. , 4 : 306 – 309 .
- Mostert , M. E. 2010 . Molecular and morphological assessment of invasive inland Rattus (Rodentia: Muridae) congenetics in South Africa and their reservoir host potential with respect to Helicobacter and Bartonella , South Africa : University of Pretoria . MSc diss.
- Parola , P. , Sanogo , O. Y. , Lerdthusnee , B. K. , Zeaiter , D. Z. , Chauvancy , B. G. , Gonzalez , J. P. , Miller , R. S. , Telford , S. R. , Wongsrichanalai , A. and Raoult , D. 2003 . Identification of Rickettsia spp. and Bartonella spp. in fleas from the Thai–Myanmar border . Ann. N.Y. Acad. Sci. , 990 : 173 – 181 .
- Picker , M. , Griffiths , C. and Weaving , A. 2004 . Field guide to insects of southern Africa , Cape Town , , South Africa : Struik .
- Podsaidly , E. , Chmielewski , T. , Sochon , E. and Tylewska-Wierzbanowska , S. 2007 . Bartonella henselae in Ixodes ricinus ticks removed from dogs . Vector Borne Zoonotic Dis. , 7 : 189 – 192 .
- Pretorius , A. , Beati , L. and Birtles , R. J. 2004 . Diversity of Bartonellae associated with small mammals inhabiting Free State province, South Africa . Int. J. Syst. Evol. Microbiol. , 54 : 1959 – 1967 .
- Reid , G. , Howard , J. and Gan , B. S. 2001 . Can bacterial interference prevent infection? . Trends Microbiol. , 9 : 424 – 428 .
- Shrewsbury , J. F.D. 2005 . A History of Bubonic Plague in the British Isles , Cambridge : Cambridge University Press .
- Soliman , S. , Main , A. J. , Marzouk , A. S. and Montasser , A. A. 2001 . Seasonal studies on commensal rats and their ectoparasites in a rural area of Egypt: The relationship of ectoparasites to the species, locality, and relative abundance of the host . J. Parasitol. , 87 : 545 – 553 .
- Stevenson , H. L. , Bai , Y. , Kosoy , M. Y. , Montenieri , J. A. , Lowell , J. L. , Chu , M. C. and Gage , K. L. 2003 . Detection of novel Bartonella strains and Yersinia pestis in prairie dogs and their fleas (Siphonaptera: Ceratophyllidae and Pulicidae) using multiplex polymerase chain reaction . J. Med. Entomol. , 40 : 329 – 337 .
- Taylor , P. J. , Arntzen , L. , Hayter , M. , Iles , M. , Frean , J. and Belmain , S. 2008 . Understanding and managing sanitary risks due to rodent zoonoses in an African city: Beyond the Boston model . Integr. Zool. , 3 : 38 – 50 .
- Traweger , D. , Travnitzky , A. E.R. , Walzer , C. and Guenther , A. E. 2006 . Habitat preferences and distribution of the brown rat (Rattus norvegicus, Berk.) in the city of Salzburg (Austria): Implications for an urban rat management . J. Pest Sci. , 79 : 113 – 125 .
- Vayssier-Taussat , M. , Le Ruhn , D. , Bonnet , S. and Cotte , V. 2009 . Insights in Bartonella host specificity. Rickettsiology nad Rickettsial diseases-fifth international conference . Ann. N. Y. Acad. Sci. , 1166 : 127 – 132 .
- Vijay , K. S. 1997 . Ixodid ticks infecting rodents and sheep in diverse biotopes of southern India . J. Parasitol. , 84 : 766 – 767 .
- Walker , J. B. , Keirans , J. E. and Horak , I. 2000 . The Genus Rhipicephalus (Acari, Ixodidae) A Guide to the Brown Ticks of the World , Cambridge : Cambridge University Press .