Abstract
In this paper, we conduct a careful global stability analysis for a generalized cholera epidemiological model originally proposed in [J. Wang and S. Liao, A generalized cholera model and epidemic/endemic analysis, J. Biol. Dyn. 6 (2012), pp. 568–589]. Cholera is a water- and food-borne infectious disease whose dynamics are complicated by the multiple interactions between the human host, the pathogen, and the environment. Using the geometric approach, we rigorously prove the endemic global stability for the cholera model in three-dimensional (when the pathogen component is a scalar) and four-dimensional (when the pathogen component is a vector) systems. This work unifies the study of global dynamics for several existing deterministic cholera models. The analytical predictions are verified by numerical simulation results.
1. Introduction
Cholera, a severe water- and food-borne infectious disease caused by the gram negative bacterium Vibrio cholerae, remains a significant public health burden in the developing world, despite a large body of clinical and theoretical studies Citation1 Citation10 Citation12 Citation20 Citation33,Citation36–38,Citation45 and tremendous efforts in prevention and intervention Citation48. This is partly due to the limited understanding at present on the complex infection dynamics of cholera, which involve both direct human-to-human and indirect environment-to-human transmission pathways.Footnote
Over the last decade, quite a few mathematical models have been published to investigate the transmission dynamics of cholera. For example, Codeço in 2001 proposed a model Citation5 that explicitly accounted for the environmental component, i.e. the V. cholerae concentration in the water supply, into a regular SIR epidemiological model. The incidence (or the infection force) was modelled by a saturating function aS(B/(K+B)) to represent the effect of saturation, where S is the susceptible population, B is the pathogen concentration, a is the contact rate with contaminated water, and K is the half saturation rate (i.e. the infectious dose in water sufficient to produce disease in 50% of those exposed). Hartley et al.
Citation13 in 2006 extended Codeço's work to include a hyperinfectious (HI) state of the pathogen, representing the ‘explosive’ infectivity of freshly shed V. cholerae, based on the laboratory observations Citation1
Citation33. They modelled the incidence factor by where βH and β
L
are the HI and less-infectious (LI) ingestion rates, and κH and κ
L
are the HI and LI half saturation rates. This model was rigorously analysed in Citation30. Joh et al.
Citation18 in 2009 modified Codeço's model by a threshold pathogen density for infection, with a careful discussion on human–environment contact and in-reservoir pathogen dynamics. More recently, Mukandavire et al.
Citation34 proposed a model to study the 2008–2009 cholera outbreak in Zimbabwe. The model explicitly considered both human-to-human and environment-to-human transmission pathways. The incidence was represented by
with I denoting the infected population and βe and βh being the rates of vibrio ingestion from the environment and the human–human interaction, respectively. The results in this work demonstrated the importance of the human-to-human transmission in cholera epidemics, especially in such places as Zimbabwe, a land-locked country in the middle of Africa. Moreover, Tien and Earn Citation44 in 2010 published a water-borne disease model which also included the dual transmission pathways, with bilinear incidence rates employed for both the environment-to-human and human-to-human infection routes. No saturation effect was considered in Tien and Earn's work.
The afore-mentioned work has certainly made important contribution to the understanding of cholera dynamics. Some limitations of these models, however, are that they only considered bilinear or saturating incidence functions, and they all assumed that the bacterial growth outside of human hosts follows linear dynamics. Practically, the multiple interactions between human population, cholera pathogen, and the environment could be much more complicated. For example, in a cholera model published by Jensen et al.
Citation17, the incidence was represented as , a highly nonlinear function, and the growth of V. cholerae was also nonlinear (a quadratic function in B). Also, in a recent paper by Shuai and van den Driessche Citation39, the incidence function is a summation of the form
with nonlinear functions f
j
and g
j
for different infection stages and pathogen concentrations, and the bacterial growth is determined by a nonlinear shedding rate from the infectious human population.
Building on these studies, Wang and Liao Citation47 in 2011 proposed a generalized cholera epidemiological model which incorporates general incidence and pathogen functions into the multiple transmission pathways, and which unifies many of the existing cholera models. Careful equilibrium analysis has been conducted in Citation47 and the results are summarized in Theorem 2.2 in Section 2 of the present paper. In particular, the local and global dynamics of the disease-free equilibrium, as well as the existence, uniqueness, and local dynamics of the endemic equilibrium, have been established.
The global stability of the endemic equilibrium for this general model, however, has not been resolved. In fact, to our knowledge, very few studies on cholera modelling have addressed the endemic global dynamics Citation43. Thus, some important epidemiological questions, e.g. whether the long-term disease dynamics approaches an equilibrium and how this depends on the initial size of the infection, remain to be answered. The study of the endemic global stability is not only mathematically important, but also essential in predicting the evolution of the disease in the long run so that prevention and intervention strategies can be effectively designed, and public health administrative efforts can be properly scaled. The challenge, however, in the global analysis of cholera models is that due to the incorporation of the environmental components, the models usually constitute high-dimensional nonlinear autonomous systems for which the classical Poincaré–Bendixson theory Citation14 is no longer valid. The method of monotone flow Citation23 Citation24,Citation40–42 can be applied to a class of high-dimensional dynamical systems which possesses monotonicity (e.g. competitiveness). Unfortunately, this technique is not applicable to most cholera epidemic models which are non-monotone. The method based on Lyapunov functions Citation19 Citation21 is well known for stability analysis, though the fact that there is no systematic way to find Lyapunov functions hinders the application of this approach to many nonlinear systems. Finally, the geometric approach for stability analysis, originally developed by Li and Muldowney Citation9 Citation25 Citation28, has gained much popularity in recent years, especially in dealing with mathematical epidemic models. Nevertheless, the majority of the applications of the geometric approach is concerned with regular epidemiological models such as SIR, SEIR, SIS, SIRS, or the like. In the present paper, we aim to extend this approach to the endemic global stability analysis of the general cholera model, which is a combined human–environment epidemiological model coupling a SIR model with the pathogen components. Such an extension is nontrivial and, to our knowledge, has not been achieved for epidemiological models of this type before.
We organize the remainder of this paper as follows. In Section 2, we briefly present the generalized cholera model and summarize the results established in Citation47. In Section 3, we apply the geometric approach based on the second compound matrix to analyse the three-dimensional system, where the pathogen component is a scalar. In Section 4, we deal with the four-dimensional model where the pathogen component is a vector. The analysis for higher dimensional system is usually more challenging, and the global stability of our four-dimensional system is established by using the geometric approach based on the third compound matrix. In addition, we verify the analytical predictions in Sections 3 and 4 by numerical simulation results. Finally, we close the paper by conclusions in Section 5.
2. Mathematical model
The model consists of the following differential equations:
Based on biological feasibility, the following conditions for f(I, B) and h(I, B) are assumed for I≥0, B≥0:
(a) f(0, 0)=0, h(0, 0)=0; | |||||
(b) f(I, B)≥0; | |||||
(c) | |||||
(d) f(I, B) and h(I, B) are concave; i.e. the matrices D 2 f and D 2 h are negative semi-definite. |
Here and in what follows, we write a vector if each component of V is
; we write a matrix
if A is positive (negative) semi-definite.
The article Citation47 conducted some analysis on this model. Based on the next-generation matrix approach Citation8, the basic reproduction number R 0 was found by
Remark 2.1 We note that the last inequality in assumption (c) above is based on the experimental observation that the pathogen V. cholerae cannot maintain a stable population in the environment in the absence of the inflow from contaminated sewage Citation5. This assumption has been used in several existing cholera models (e.g. Citation5
Citation13
Citation34
Citation44). In addition, when B is a vector, is a matrix. To ensure that R
0 is positive, we will further assume that
is an M-matrix (i.e. a non-singular square matrix with non-positive off-diagonal entries and all principal minors positive). It is known that the inverse of an M-matrix has all its entries being non-negative Citation2
Citation7.
Under these assumptions, the equation h(I, B)=0 implicitly defines a function B=g(I) with g′(I)≥0, and EquationEquation (5) yields
It is also shown that there exists a forward transcritical bifurcation at R 0=1 for this model. Specifically, the following theorem summarizes the dynamics known for the system Equation(1)–(4).
Theorem 2.2
Citation47 When R 0<1, there is a unique DFE, which is both locally and globally asymptotically stable; when R 0>1, the DFE becomes unstable, and there is a unique positive endemic equilibrium which is locally asymptotically stable.
3. Three dimensional system
We will now focus our attention on the global asymptotic stability of the endemic equilibrium for R 0>1. We first consider the case when B is a scalar; i.e. there is only one infectious state (which is assumed to be homogeneous) for the pathogen in the environment. Most cholera models in the literature (e.g. Citation5 Citation18 Citation34 Citation44) fall into this category. With the pathogen component being a scalar, the system Equation(1)–(3) is three-dimensional.
The assumption implies that the surface h=h(I, B) is below its tangent plane at any point
; that is,
We employ the geometric approach based on the second compound matrix Citation9 Citation25 Citation28 to analyse the endemic global stability of this system. A brief summary of this technique is provided in Appendix A.
Based on Theorem 2.2, the DFE, X 0=(N, 0, 0), is unstable when R 0>1. Since the DFE is on the boundary of the domain Δ, this implies the uniform persistence Citation11; i.e. there exists a constant c>0 such that
Proposition 3.1
When R 0>1, the system Equation(1)–(3) is uniformly persistent, and satisfies the assumptions (H1) and (H2) listed in Appendix A.
We proceed to verify the Bendixson criterion q 2¯<0 (see Theorem A.1). The Jacobian matrix of the system Equation(1)–(3) is
Hence, we have established the following theorem:
Theorem 3.2
When R
0>1, the unique endemic equilibrium of the three-dimensional system
Equation(1)–(3)
is globally asymptotically stable in Δ under the assumptions (
a
)– (
d
) and
Equation(13).
Remark 3.3 Theorem 3.2 establishes the global endemic stability for the unified three-dimensional cholera model. In particular, the result can be directly applied to several existing cholera models in the literature, such as those in Citation5 Citation34 Citation44.
To quantify the analysis, we consider the cholera model proposed by Mukandavire et al. Citation34:
Figure 1. A phase portrait of S vs I for the cholera model Equation(16)–(19). The total population is N=10, 000, and the initial condition is I(0)=1000, S(0)=9000 and R(0)=B(0)=0. The curve converges to the endemic equilibrium at S* ≈ 1510, I* ≈ 2.68. Similar pattern is observed for various different initial conditions.
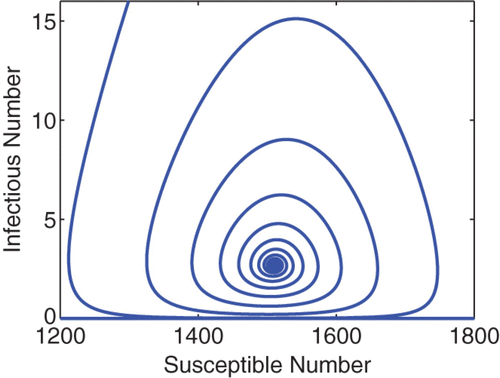
4. Four-dimensional system
We now consider the model Equation(1)–(3) in the case when B is a vector; i.e. there are heterogeneous and multiple states of infectivity for the pathogen outside the human hosts. The representation of pathogen dynamics in multiple states can possibly provide a deeper insight into the pathogen ecology and the complex interaction between the human hosts and the environment, and reflect better the nature of heterogeneity in disease transmission. Without loss of generality, we assume B=[B 1, B 2], where B 1 and B 2 represent two different infectious states of the bacterial concentrations. A similar formulation of B was used in Citation13, where the HI and LI states of the vibrios were modelled. Multiple states of cholera bacterial concentrations were also studied in Citation39.
We consider the following four-dimensional system corresponding to the model Equation(1)–(3):
Let
Proposition 4.1
For any
and any initial value
there is a constant
such that
Similar to Proposition 3.1, the instability of the DFE (N, 0, 0, 0), which is on the boundary of the domain Γ, implies uniform persistence Citation11. Based on Theorem 2.2, we thus obtain
Proposition 4.2
When R 0>1, the system Equation(20)–(23) is uniformly persistent.
We now prove the main result in this section, i.e. the global stability of the endemic equilibrium, using the geometric approach based on the third compound matrix (see Appendix B). To simplify our notations, we will adopt the abbreviations
Theorem 4.3
If R 0>1 and
Proof
The Jacobian matrix of the system Equation(20)–(23) is given by
Next, we calculate the total derivative of V along the trajectory of the compound system Equation(33)–(36). We will separate the discussion for the several cases below.
Case I: V=V 1, then V 2, V 3≤V 1 and
Case II: V=V 2, then V 1, V 3≤V 2. In a way similar to Case I, we can obtain the estimates
Case III-a: V=I|W+Z|, then and
Case III-b: V=I|W|, then WZ<0, , and
Remark 4.4 Theorem 4.3 establishes the global endemic stability for a general four-dimensional cholera model. As a special case, the result can be directly applied to the cholera model in Citation13. We note, however, that the assumption in Equation(32) is a sufficient condition for the global stability and it might restrict the applicability of the result.
Remark 4.5 The analysis presented here can be easily extended to the case with variable host population, where we incorporate immigration and disease-related mortality into the model Equation(20)– Equation(23)
. Thus, Equations {\rm Equation(20)
} and {\rm Equation(21)
} will be replaced by
Figure 2. A phase portrait of S vs I for the cholera model (49)–(53). The total population is N=10, 000, and the initial condition is I(0)=1000, S(0)=9000, and R(0)=B H (0)=B H(0)=0. The curve converges to the endemic equilibrium at S* ≈ 7666 and I* ≈ 0.92. Similar pattern is observed for various different initial conditions.
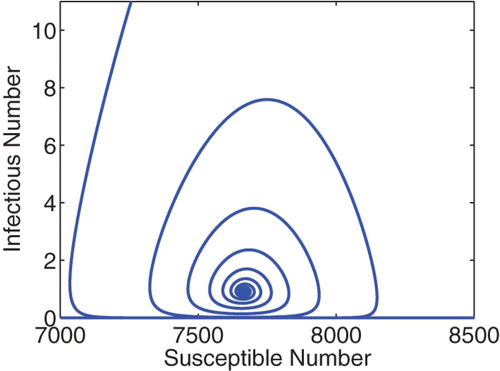
Finally, we present an example to verify the analytical prediction in Theorem 4.3. We consider the cholera model of Hartley et al. Citation13:
5. Conclusions
The present work aims to understand the global dynamics of cholera epidemiology in a general mathematical model which has a potential to incorporate different factors of the human host, the environment, and the pathogen ecology into a unified framework. Such an understanding is important for the effective prevention and intervention strategies against cholera outbreak. The unified global stability results established in this paper can be applied to many published cholera models, including (but not limited to) those in Citation5 Citation13 Citation18 Citation34 Citation44 Citation47.
Due to the incorporation of the environmental component and the coupling of multiple transmission pathways, the cholera model considered in this paper distinguishes itself from regular SIR and SEIR epidemiological models whose global dynamics have been extensively studied Citation15 Citation16,Citation22–24,Citation27 Citation29 Citation32 Citation49. Using the geometric approach, we have carefully investigated both three-dimensional and four-dimensional systems under general settings. The analysis and results presented in this paper not only extend the application of the geometric approach, but also provide a framework for modelling and analysing other infectious diseases such as typhoid fever, amoebiasis, dracunculiasis, giardia, cryptosporidium, and campylobacter Citation31 Citation44 which involve environmental components (e.g. water-borne pathogen).
Acknowledgements
J. Wang acknowledges partial support from the National Science Foundation (under Grant No. DMS-0813691) and the Simons Foundation (under Grant No. 208716). The authors thank the two anonymous referees for their helpful comments to improve this paper.
Notes
This paper is based on an invited talk given at the 3rd International Conference on Math Modeling & Analysis, San Antonio, USA, October 2011.
References
- Alam , A. , LaRocque , R. C. , Harris , J. B. , Vanderspurt , C. , Ryan , E. T. , Qadri , F. and Calderwood , S. B. 2005 . Hyperinfectivity of human-passaged Vibrio cholerae can be modeled by growth in the infant mouse . Infect. Immun. , 73 : 6674 – 6679 .
- Berman , A. and Plemmons , R. J. 1994 . Nonnegative Matrices in the Mathematical Sciences , Philadelphia : SIAM .
- Buonomo , B. and Lacitignola , D. 2008 . On the use of the geometric approach to global stability for three dimensional ODE systems: A bilinear case . J. Math. Anal. Appl. , 348 : 255 – 266 .
- Butler , G. J. and Waltman , P. 1986 . Persistence in dynamical systems . Proc. Amer. Math. Soc. , 96 : 425 – 430 .
- Codeço , C. T. 2001 . Endemic and epidemic dynamics of cholera: The role of the aquatic reservoir . BMC Infect. Dis. , 1 ( 1 )
- Coppel , W. A. 1965 . Stability and Asymptotical Behavior of Differential Equations , Boston, MA : Heath Mathematical Monographs, D. C. Heath .
- Cross , G. W. 1978 . Three types of matrix stability . Linear Algebra Appl. , 20 : 253 – 263 .
- van den Driessche , P. and Watmough , J. 2002 . Reproduction numbers and sub-threshold endemic equilibria for compartmental models of disease transmission . Math. Biosci. , 180 : 29 – 48 .
- Fan , M. , Li , M. Y. and Wang , K. 2001 . Global stability of an SEIS epidemic model with recruitment and a varying total population size . Math. Biosci. , 170 : 199 – 208 .
- Faruque , S. , Islam , M. , Ahmad , Q. , Faruque , A. S.G. , Sack , D. , Nair , G. and Mekalanos , J. 2005 . Self-limiting nature of seasonal cholera epidemics: Role of host-mediated amplification of phage . Proc. Nat. Acad. Sci. , 102 : 6119 – 6124 .
- Freedman , H. I. , Ruan , S. and Tang , M. 1994 . Uniform persistence and flows near a closed positively invariant set . J. Dyn. Differential Equations , 6 : 583 – 600 .
- Goh , K. , Teo , S. , Lam , S. and Ling , M. 1990 . Person-to-person transmission of cholera in a psychiatric hospital . J. Inf. , 20 : 193 – 200 .
- Hartley , D. M. , Morris , J. G. and Smith , D. L. 2006 . Hyperinfectivity: A critical element in the ability of V. cholerae to cause epidemics? . PLoS Med. , 3 : 0063 – 0069 .
- Hartman , P. 1980 . Ordinary Differential Equations , New York : John Wiley .
- Hethcote , H. W. 1976 . Qualitative analysis of communicable disease models . Math. Biosci. , 28 : 335 – 356 .
- Hethcote , H. W. 2000 . The mathematics of infectious diseases . SIAM Rev. , 42 : 599 – 653 .
- Jensen , M. , Faruque , S. M. , Mekalanos , J. J. and Levin , B. 2006 . Modeling the role of bacteriophage in the control of cholera outbreaks . Proc. Nat. Acad. Sci. , 103 : 4652 – 4657 .
- Joh , R. I. , Wang , H. , Weiss , H. and Weitz , J. S. 2009 . Dynamics of indirectly transmitted infectious diseases with immunological threshold . Bull. Math. Biol. , 71 : 845 – 862 .
- Khalil , H. K. 1996 . textit>Nonlinear Systems , Prentice-Hall, Englewood Cliffs, NJ
- King , A. A. , Lonides , E. L. , Pascual , M. and Bouma , M. J. 2008 . Inapparent infections and cholera dynamics . Nature , 454 : 877 – 881 .
- Lajmanovich , A. and Yorke , J. 1976 . A deterministic model for gonorrhea in a nonhomogeneous population . Math. Biosci. , 28 : 221 – 236 .
- Li , G. and Jin , Z. 2005 . Global stability of an SEI epidemic model with general contact rate . Chaos, Solitons Fractals , 23 : 997 – 1004 .
- Li , M. Y. , Graef , J. R. , Wang , L. and Karsai , J. 1999 . Global dynamics of a SEIR model with varying total population size . Math. Biosci. , 160 : 191 – 213 .
- Li , M. Y. and Muldowney , J. S. 1995 . Global stability for the SEIR model in epidemiology . Math. Biosci. , 125 : 155 – 164 .
- Li , M. Y. and Muldowney , J. S. 1996 . A geometirc approach to global-stability problems . SIAM J. Math. Anal. , 27 : 1070 – 1083 .
- Li , M. Y. and Muldowney , J. S. 2000 . Dynamics of differential equations on invariant manifolds . J. Differential Equations , 168 : 295 – 320 .
- Li , M. Y. , Muldowney , J. S. and Driessche , P. V.D. 1999 . Global stability of SEIRS models in epidemiology . Can. Appl. Math. Q. , 7 : 409 – 425 .
- Li , M. Y. , Smith , H. L. and Wang , L. 2001 . Global dynamics of an SEIR epidemic model with vertical transmission . SIAM J. Math. Anal. , 62 : 58 – 69 .
- Li , M. Y. and Wang , L. 2002 . Global stability in some SEIR epidemic models , : 295 – 311 . IMA Volumes in Mathematics and Its Application Vol. 126, Springer-Verlag, Berlin
- Liao , S. and Wang , J. 2011 . Stability analysis and application of a mathematical cholera model . Math. Biosci. Eng. , 8 : 733 – 752 .
- Ma , S. and Xia , Y. 2008 . “ Mathematical Understanding of Infectious Disease Dynamics ” . Edited by: Ma , S. and Xia , Y. Singapore : Institute for Mathematical Sciences, National University of Singapore . Lecture Notes Series Vol. 16
- Mena-Lorca , J. and Hethcote , H. W. 1992 . Dynamic models of infectious diseases as regulator of population sizes . J. Math. Biol. , 30 : 693 – 716 .
- Merrell , D. S. , Butler , S. M. , Qadri , F. , Dolganov , N. A. , Alam , A. , Cohen , M. B. , Calderwood , S. B. , Schoolnik , G. K. and Camilli , A. 2002 . Host-induced epidemic spread of the cholera bacterium . Nature , 417 : 642 – 645 .
- Mukandavire , Z. , Liao , S. , Wang , J. , Gaff , H. , Smith , D. L. and Morris , J. G. 2011 . Estimating the reproductive numbers for the 2008–2009 cholera outbreaks in Zimbabwe . Proc. Nat. Acad. Sci. , 108 : 8767 – 8772 .
- Muldowney , J. S. 1990 . Compound matrices and ordinary differential equations . Rocky Mountain J. Math. , 20 : 857 – 872 .
- Nelson , E. J. , Harris , J. B. , Morris , J. G. , Calderwood , S. B. and Camilli , A. 2009 . Cholera transmission: The host, pathogen and bacteriophage dynamics . Nat. Rev.: Microbiol. , 7 : 693 – 702 .
- Pascual , M. , Rodo , X. , Ellner , S. P. , Colwell , R. and Bouma , M. J. 2000 . Cholera dynamics and El Nino-Southern oscillation . Science , 289 : 1766 – 1769 .
- Pascual , M. , Bouma , M. and Dobson , A. 2002 . Cholera and climate: Revisiting the quantiative evidence . Microbes Infections , 4 : 237 – 245 .
- Shuai , Z. and van den Driessche , P. 2011 . Global dynamics of cholera models with differential infectivity . Math. Biosci. , 234 : 118 – 126 .
- Smith , H. L. 1995 . “ Monotone Dynamical Systems: An Introduction to the Theory of Competitive and Cooperative Systems ” . Providence, RI : American Mathematical Society .
- Smith , H. L. and Zhu , H. R. 1994 . Stable periodic orbits for a class of three dimensional competitive systems . J. Differential Equations , 110 : 143 – 156 .
- Smith , R. A. 1987 . Orbital stability for ordinary differential equations . J. Differential Equations , 69 : 265 – 287 .
- Tian , J. P. and Wang , J. 2011 . Global stability for cholera epidemic models . Math. Biosci. , 232 : 31 – 41 .
- Tien , J. H. and Earn , D. J.D. 2010 . Multiple transmission pathways and disease dynamics in a waterborne pathogen model . Bull. Math. Biol. , 72 : 1502 – 1533 .
- Tudor , V. and Strati , I. 1977 . Smallpox, Cholera , Tunbridge Wells : Abacus Press .
- Vynnycky , E. , Trindall , A. and Mangtani , P. 2007 . Estimates of the reproduction numbers of Spanish influenza using morbidity data . Int. J. Epidemiol. , 36 : 881 – 889 .
- Wang , J. and Liao , S. 2012 . A generalized cholera model and epidemic/endemic analysis . J. Biol. Dyn. , 6 568–589
- World Health Organization, Available at web page: www.who.org.
- Zhang , J. and Ma , Z. 2003 . Global dynamics of an SEIR epidemic model with saturating contact rate . Math. Biosci. , 185 : 15 – 32 .
Appendix A
We briefly describe below the geometric approach based on the second additive compound matrix, developed by Li and Muldowney Citation9 Citation25 Citation28.
For a 3×3 matrix A=[a ij ], the second additive compound matrix is defined as
Now consider the dynamical system
(H1) There exists a compact absorbing set K⊂D; | |||||
(H2) The system (A1) has a unique equilibrium point X* in D. |
It is shown in Citation9 Citation25 Citation28 that X* is globally asymptotically stable if (A1) satisfies (H1)(H2) and a Bendixson criterion that is robust under C 1 local perturbations of F at all non-equilibrium non-wandering points. This criterion is obtained as follows.
Let be a
matrix-valued C
1 function in D. Set
In summary, we have the following theorem:
Theorem A.1
Assume that D is simply connected and the assumptions {\rm ({ H1})} and {\rm (H2)} hold. Then the unique equilibrium X* of (A1) is globally asymptotically stable in D if q¯2<0.
Appendix B
In what follows, we outline the geometric approach based on the third additive compound matrix Citation26 Citation27.
The third additive compound matrix for a 4×4 matrix A=[a ij ] is defined as
For a solution X(t, X 0) of any initial value problem of the dynamic system (A1), the linearized system is
Theorem A.2
Assume that {\rm (H1)} and {\rm (H2)} hold and there are a Lyapunov function V(X, Z), a function K(t), and positive constants c, k, and C such that
(i)
| |||||
(ii)
|
Proof
If this is the Corollary 3.2 in Citation27. For a general differentiable function K(t), the conclusion follows from the Corollary 3.2 in Citation27, since the modified Lyapunov function
satisfies all the conditions. ▪