Abstract
In order to maximize the information that a linearized stability analysis provides, one should work with two free parameters rather than one. Moreover, it is recommended to first consider coefficients in the characteristic equation as parameters and in a second step (try to) invert the map that defines the coefficients in terms of the parameters as they occur in the original equation. Our aim is to substantiate these claims by way of a delay equation example taken from the literature.
1. Introduction
A delay equation is a rule for extending a function of time towards the future on the basis of the (assumed to be) known past. For differential delay equations the rule specifies the derivative at the current time point Citation13Citation17Citation19Citation28, whilst for renewal equations the rule specifies the value of the function itself in the current time point Citation3Citation11Citation14Citation20. Many physiologically structured population models lead to systems of equations that have differential delay as well as renewal components Citation15.Footnote†
The literature on delay equations is overrun with papers in which a one-parameter Hopf bifurcation analysis is presented for differential equations with discrete delay like
And indeed, the Hopf bifurcation theorem itself is usually and conveniently formulated in a one-parameter setting, see, for example, [Theorem X.2.7]Citation13. The aim of this short note is to advocate the use of two parameters rather than one in the following way: first one finds the boundary of the stable region in parameter space characterized by a root of the characteristic equation lying exactly on the imaginary axis (more precisely, a pair of complex conjugate roots). Next, one can apply the Hopf bifurcation theorem to whatever one-dimensional path in the two-parameter plane one is interested in, provided the path intersects the stability boundary transversally.
Our plea, like the one in Citation4, applies to dynamical systems in general, not just to delay equations. But the approach is somehow more powerful if we deal with a scalar equation (see, e.g. Citation27 for a similar observation). And first order scalar delay equations can exhibit oscillations whereas first order scalar ordinary differential equations cannot. Likewise the approach is, as a rule, more powerful in case of point delays than when delay is distributed, see the text around Equation (21).
The characteristic equation is one complex equation, so two real equations if we look for roots on the imaginary axis. So if we have a k-dimensional parameter, the equation defines generically a (k+1−2)-dimensional manifold in (parameter, frequency)-space. When k>2 we cannot possibly parametrize this manifold by just ω, but if k=2 we may indeed attempt to use ω to parametrize a curve in the two-dimensional parameter space. For instance, whenever the parameters occur linearly in the equation one can indeed solve for the parameters in terms of the frequency ω. Also note that this yields not just a convenient parametrization but that it does in fact provide very useful information, as the period of the bifurcating periodic solutions is close to 2π/ω. And there is an additional bonus: human beings are particularly good at absorbing two-dimensional visual information!
The coefficients in the characteristic equation are often composite parameters, in the sense that they may relate in complicated, and sometimes implicit, ways to the parameters in the original delay equation. It then makes sense to follow a two-step procedure:
Step 1: Characterize the stability boundary in coefficient-space.
Step 2: Try to invert the map from original parameters to coefficients.
The second step is often cumbersome, as there may easily be more parameters than coefficients and hence the inverse is, in general, multi-valued.
In [Chapter XI]Citation13 several examples of this approach are presented. We refer to Citation29 for general results on characteristic equations. In Citation10 a numerical variant of our approach is presented. See Citation2 for a quite different numerical approach and a very nice tool. In Citation16 the importance of the second step is illustrated by way of an example (showing that a wrong conclusion can arise if one neglects this step). Finally, we note that some time ago Jim Cushing in a sequence of papers Citation5–9 proved a series of theoretical results showing that one can actually find periodic solutions of the nonlinear problem of fixed period by exploiting the availability of two parameters. Thus the two-parameter plane becomes locally foliated by level curves of the period. Now that numerical bifurcation analysis is becoming more and more powerful, it seems feasible to develop a tool that yields a picture of this foliation.
We aim to substantiate the above general considerations in the next sections by elaborating a well-known example: population dynamics with delayed negative feedback. Here we are motivated by the quest for long period oscillations in the inspiring paper Citation26 about a model for hematopoietic stem cells. Section 2 deals with Step 1. In essence, we just note that the information we are after is already available in the literature. Section 3 is concerned with a special and rather qualitative variant of Step 2: since we are interested in long periods we want small frequencies, and so we investigate the inverse image of the point on the stability boundary corresponding to ω=0, i.e. we aim to characterize the conditions on the original parameters such that one may expect periodic solutions with long periods. In other words, we perform a systematic search for regions in parameter space where one can expect to see long period oscillations (either damped or sustained). We obtain a diagram showing a transcritical bifurcation curve corresponding to the nontrivial steady state becoming positive, and hence biologically meaningful, as well as a Hopf bifurcation curve at which the nontrivial steady state loses stability and a periodic solution arises. The intersection of these curves is a so-called Takens-Bogdanov singularity.
2. The prototype of delayed negative feedback
In this paper we first of all follow Pujo-Menjouet et al. Citation26 and consider the delay differential equation
for the concentration N of blood production stem cells in the resting phase. Here δ is the rate at which stem cells differentiate into precursor cells of the various types of mature blood cells. At a rate β, which depends smoothly on the prevailing concentration N(t), stem cells enter a proliferation phase of duration τ. As during this phase apoptosis occurs at rate γ, only a fraction
of the cells that start the proliferation phase reaches its end. At the end of the proliferation phase the cell divides into two daughter cells, which enter the resting phase.
We assume that feedback is negative: β is a decreasing function of N. It is well-known that the combination of negative feedback and delay can lead to destabilization of a steady state and to oscillations. Often, and also here, this is shown by a Hopf bifurcation analysis.
In Pujo-Menjouet et al. Citation26, they raise the question: how do short cell cycles give rise to long period oscillations? The aim of the present short note is to show that some of their answers can be obtained rather easily and efficiently by performing a two-parameter analysis of the characteristic equation
corresponding to the linearization of Equation (1) around its nontrivial steady state (explicit formulas for α1 and α2 are given in the next section). In fact Equation (2) is well-understood, see [Chapter XI]Citation13 for a textbook treatment. And the understanding can be conveniently summarized in the form of the diagram of , showing how the
-plane is partitioned into regions according to how many roots of Equation (2) lie in the right half-plane.
Figure 1. Bifurcation diagram in the (α1,α2)-plane showing a transcritical bifurcation curve (red) and Hopf bifurcation curves (blue) for kπ<ω<(k+1) π with k=0, 1, 2. The numbers of eigenvalues in the right half of the complex plane are indicated in the boxes. Each number (box) corresponds to a region bounded by the bifurcation curves.
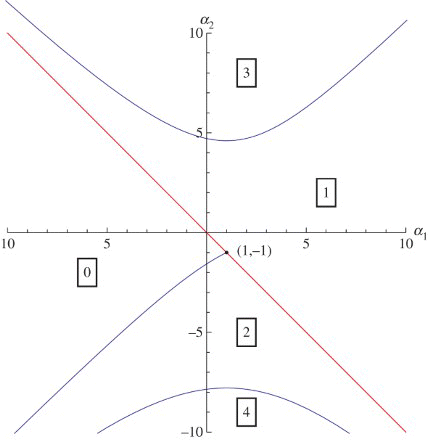
The stability region is characterized by no root at all lying in the right half-plane. Its boundary has two parts:
The line
with
(λ=0 is a root of Equation (2)).
The curve
for
, corresponding to
being a root of Equation (2).
The line and the curve intersect at for which λ=0 is a double root of Equation (2).
A fortunate feature is that the curve is parametrized by the frequency ω of the oscillations of the linearized system at the critical parameter combination. The theory of the Hopf bifurcation, see [Chapter X]Citation13, guarantees that oscillations of the nonlinear system for nearby parameter values have roughly the same frequency. So if we are interested in long periods, we should focus on the curve (3) for small values of ω. For instance, if we consider the part of the curve with , we consider
, so periods between 4 and ∞. The nearer we get to (1,−1), the longer the period will be. This point itself is a co-dimension two bifurcation point called ‘double zero eigenvalue’ or Takens-Bogdanov singularity. The unfolding under natural non-degeneracy conditions can be found in [Section 8.4]Citation21. Also see [Section IX.10]Citation13.
But what is the unit of time that we use when we derive Equation (2)? And how do the stylized parameters α1 and α2 relate to the parameters occurring in Equation (1)? How do we translate the information contained in and Equation (3) into information about solutions of Equation (1)?
We shall answer these questions in the next section. But before doing so, we draw attention to the fact that exactly the same Equation (2) governs the stability character of a nontrivial steady state of equations corresponding to quite different population dynamical settings. Indeed, the data from the famous blowfly experiments of Citation23Citation24 are, as far as known today Citation18, best reproduced by solutions of the delay differential equation
where N is the size of the subpopulation of adults (= reproducing individuals), TD corresponds to the maturation delay, R to reproduction and δ is a per capita adult death rate (note that juvenile survival has to be incorporated in R). In his experiments Nicholson provided limited amount of proteins, an essential ingredient for egg production by adult females. Scramble competition then leads to egg production that is proportional to N for small N, but goes to zero for large N. A convenient function to describe such overcompensation is
where P is the maximal per capita egg production rate, corrected for mortality during the juvenile period, and N0 is a scale parameter for the population level as determined by density dependence. For fish populations one can use the same equation, but interpret the negative density dependence as resulting from ‘egg’ cannibalism Citation12 (this is reminiscent of the discrete time Ricker equation; see Section VI.3 of http://webarchive.iiasa.ac.at/Research/EEP/Metz2Book.html for a renewal equation variant).
3. Parameter maps
The main aim of this section is to derive the relationship between, on the one hand, α1, α2 appearing in Equation (2) and, on the other hand, the parameters δ, γ, τ and the function β appearing in Equation (1).
By introducing
we achieve that we can take
and
The upshot is that Equation (1) now reads
with δ, γ and β(N) dimensionless.
We now deduce Equation (2) from Equation (6). Of course we do so in order to go in a next step in the other direction, i.e. from Equation (2) to Equation (6).
The conditions
and
guarantee that the zero steady state of Equation (6) is unstable and also that Equation (6) has, provided δ>0 and β decreases to a low enough level for large N, a strictly positive steady state N̄ characterized by the equation
From Equation (9) we conclude that Equations (7) and (8) are also necessary conditions for the existence of a strictly positive steady state.
We now substitute into Equation (6), Taylor expands β(N)N for small x and ignores higher order terms. This leads to the linearized equation
with
with
Equation (10) admits a solution of the form if and only if λ satisfies Equation (2). General theory (see, e.g. [Corollary VII.5.12]Citation13) then guarantees that N̄ is stable if all roots of Equation (2) have negative real part and unstable if at least one root has positive real part.
Is it possible to have when α1 and α2 are given by Equation (11)? This would require
and
and hence
, which violates Equation (7). So this is not possible when
. But this very same calculation also shows that we can come arbitrarily close by letting
and
whilst making sure that
. Or, in other words, a necessary condition for obtaining a Hopf bifurcation of a periodic solution with very long period is that the rate at which stem cells differentiate is very small (when we use the length of the proliferation phase as unit of time) and that the fraction of cells that survives the proliferation phase is only slightly larger than 0.5. In retrospect these are very natural conditions: if we use the length of the proliferation phase as the unit of time, the time scale of population turnover is set by the differentiation rate δ and the reproduction rate
.
Thus far the function β is an infinite-dimensional parameter. Sooner or later we need to limit ourselves to a parametrized family such as
or
with parameters N0, β0 and n. (Note that N0 is called θ in Citation25Citation26 and note once again that N0 only sets the scale of N and has no influence on the dynamics. The mathematical requirements on β as well as the biochemical reasons for choosing the decreasing Hill function defined by Equation (13) for this particular model are discussed in Citation22.)
In any case, we have enough freedom to require, for the time being, that
This choice allows us to invert Equation (11) and write
By making a Taylor expansion we deduce that for small ω
Substituting Equation (16) into Equation (9) we find
It remains to satisfy the condition . For definiteness, let us focus on the family (13) whilst taking without loss of generality N0=1. One can easily verify that for β given by Equation (13) we have
This identity allows us to rewrite
in the form
which upon substitution of Equation (18) becomes an explicit expression for
in terms of ω. For ω=0 we have
.
Let us step back and survey the situation. For small values of ω we may choose δ and 2e−γ according to Equation (16). If we next consider β0 as a bifurcation parameter, then a Hopf bifurcation with limiting period 2π/ω takes place when β0 passes the critical value specified by the right hand-side of Equation (20) with
given by Equation (18). A graphical representation of the results for n=3 is provided in .
Figure 2. Critical values of the parameters δ, 2 e−γ, and β0 and the Hopf period T for n=3. The transition from a stable steady state to an unstable steady state happens when we pass from below the curve to above the curve or when β0 increases from below the critical value to above
. For ω=0 we have
.
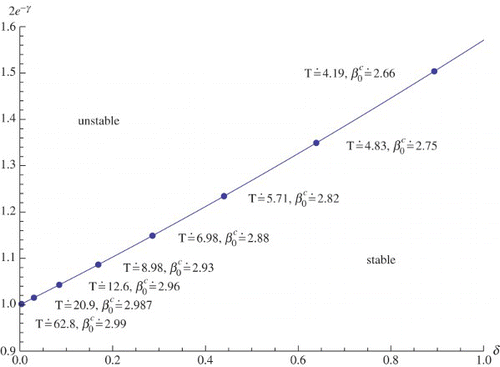
In the case of distributed delay as studied in Citation1, the characteristic equation reads
where the density f(τ) replaces the Dirac measure concentrated, after scaling, in 1. Putting
and separating real and imaginary parts, we can rewrite this as
So for given γ and f we can still parametrize a curve in a two-parameter plane by ω, provided we have at our disposal a numerical procedure that evaluates the integrals. (If we do want to consider γ as one of the ‘free’ parameters, there does not seem to be an easy way to organize the calculations.)
For the blowfly (alias egg cannibalism) model the relation between (α1, α2) and the parameters P, δ and TD is given by
and we conclude that α1 needs to be negative in order to correspond to positive P, δ and TD. This, in turn, corresponds to
and hence the period is at most 4 TD at Hopf bifurcation. We conclude that, even though both the hematopoietic and the ecological problems lead to Equation (2), there is an essential difference in the range of periods at Hopf bifurcation. This clearly illustrates that one cannot just focus on Equation (2), one needs to study the parameter map as well.
4. Conclusion
and become much more informative if along the stability boundary it is indicated whether the Hopf bifurcation is supercritical (implying that the bifurcating periodic solution is stable for parameters close to the bifurcation) or subcritical (implying it is unstable). To decide, one needs to compute the first Lyapunov coefficient and this computation involves higher order terms of the Taylor expansion. So the information is problem specific and it cannot be deduced from just Equation (2). In [Section X.3]Citation13 it is formulated how to compute the direction from linear, second and third order terms. As a rule, the computations require considerable effort, even in the case of a scalar delay equation. To follow the periodic solutions when parameters vary outside a small neighbourhood of the Hopf bifurcation point, one needs sophisticated tools for numerical continuation and bifurcation studies, such as DDE-Biftool (http://twr.cs.kuleuven.be/research/software/delay/ddebiftool.shtml).
Figure 3. repeated with a superimposed curve parametrized by δ in the (α1,α2)-plane corresponding to δ∈(0, 3), β0=1.77, γ=0.2 and n=12.
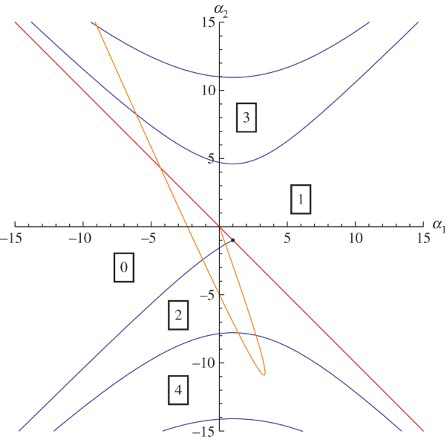
To conclude, let us compare our results with the results presented by Pujo Menjouet et al. in Citation26 and Pujo-Menjouet and Mackey in Citation25. First of all we should mention that our analysis is confined to linearized stability and Hopf bifurcation. Consequently it does not give any information about periodic solutions away from the bifurcation point, such as in contrast to the numerical study of Citation25Citation26. As far as linearized stability and Hopf bifurcation are concerned, our analysis in essence confirms the results of Citation25Citation26. The gain of our graphical representation of the stability domain in parameter space is that it provides a unifying template. This helps to reveal the precise conditions for long periods. To illustrate this, we show in how the parameters α1 and α2 change when, as in Citation25, we fix , γ=0.2 (i.e.
) and n=12, and consider δ as a bifurcation parameter. The orange δ-parametrized curve is defined by Equation (11) with δ∈(0, 3),
, γ=0.2 and n=12. We can clearly see that it crosses the Hopf curve with
. At this point,
and the relatively long period oscillations are born. When
, the nontrivial steady state regains stability and remains stable until the δ-parametrized curve reaches the line
where the nontrivial steady state ceases to exist. That happens at approximately
.
Since one can plot such a curve in the -plane for any values of the fixed parameters, the method of two-parameter bifurcation analysis using a graphical representation provides a fast and easy way to obtain practical information from the model. Moreover, this approach yields exact information about the linearized model, as opposed to the numerical simulations in Citation25Citation26. On the other hand, numerical simulations give of course an approximation of the actual solution to the nonlinear equation.
Whenever one studies a complicated problem by implementing numerical solvers, there is the danger of bugs going unnoticed. By implementing both numerical methods to determine stability boundaries and to experimentally determine the dynamical behaviour of solutions of the initial value problem and by confronting the results, one can test the reliability of both.
Acknowledgements
We thank two anonymous referees for constructive criticism which helped to improve the exposition.
Notes
†Dedicated to Mimmo Iannelli, at the occasion of his 65th birthday, in appreciation of his stimulating friendship, the love for renewal equations that we share.
References
- M. Adimy, F. Crauste, and S. Ruan, A mathematical study of the hematopoiesis process with applications to chronic myelogenous leukemia, SIAM J. Appl. Math. 65(4) (2005), pp. 1328–1352. doi: 10.1137/040604698
- D. Breda, S. Maset, and R. Vermiglio, TRACE-DDE: A Tool for Robust Analysis and Characteristic Equations for Delay Differential Equations, Lecture Notes in Control and Information Sciences Vol. 338, Springer-Verlag, Berlin, 2009.
- D. Breda, O. Diekmann, W.F. de Graaf, A. Pugliese, and R. Vermiglio, On the formulation of epidemic models (an appraisal of Kermack and McKendrick), J. Biol. Dyn. 6 (2012), pp. 103–117. doi: 10.1080/17513758.2012.716454
- H.W. Broer, V. Naudot, R. Roussarie, K. Saleh, and F.O.O. Wagener, Organising centres in the semi-global analysis of dynamical systems, Int. J. Appl. Math. Stat. 12 (2007), pp. 7–36.
- J.M. Cushing, Bifurcation of periodic solutions of integro-differential equations with applications to time delay models in population dynamics, SIAM J. Appl. Math. 33 (1977), pp. 640–654. doi: 10.1137/0133045
- J.M. Cushing, Nontrivial periodic solutions of integro-differential equations, J. Integr. Equ. 1 (1979), pp. 165–181.
- J.M. Cushing, Nontrivial Periodic Solutions of Some Volterra Integral Equations, Lecture Notes in Mathematics Vol. 737, Springer-Verlag, Berlin, 1979.
- J.M. Cushing, Bifurcation of periodic solutions of nonlinear equations in age-structured population dynamics, Nonlinear Phenomena in Mathematical Sciences, Academic Press, New York, 1982.
- J.M. Cushing, Bifurcation of time periodic solutions of the McKendrick equations with applications to population dynamics, Comput. Math. Appl. 9 (1983), pp. 459–478. doi: 10.1016/0898-1221(83)90060-3
- A.M. de Roos, O. Diekmann, P. Getto, and M.A. Kirkilionis, Numerical equilibrium analysis for structured consumer resource models, Bull. Math. Biol. 72 (2010), pp. 259–297. doi: 10.1007/s11538-009-9445-3
- O. Diekmann and M. Gyllenberg, Equations with infinite delay: Blending the abstract and the concrete, J. Differ. Equ. 252 (2012), pp. 819–851. doi: 10.1016/j.jde.2011.09.038
- O. Diekmann, R.M. Nisbet, W.S.C. Gurney, and F. van den Bosch, Simple mathematical models for cannibalism: A critique and a new approach, Math. Biosci. 78 (1986), pp. 21–46. doi: 10.1016/0025-5564(86)90029-5
- O. Diekmann, S.A. van Gils, S.M. Verduyn Lunel, and H.-O. Walther, Delay Equations. Functional, Complex, and Nonlinear Analysis, Springer-Verlag, New York, 1995.
- O. Diekmann, Ph. Getto, and M. Gyllenberg, Stability and bifurcation analysis of Volterra functional equations in the light of suns and stars, SIAM J. Math. Anal. 39 (2007), pp. 1023–1069. doi: 10.1137/060659211
- O. Diekmann, M. Gyllenberg, J.A.J. Metz, S. Nakaoka, and A.M. de Roos, Daphnia revisited: Local stability and bifurcation theory for physiologically structured population models explained by way of an example, J. Math. Biol. 61 (2010), pp. 277–318. doi: 10.1007/s00285-009-0299-y
- G. Enciso and E.D. Sontag, On the stability of a model of testosterone dynamics, J. Math. Biol. 49 (2004), pp. 627–634. doi: 10.1007/s00285-004-0291-5
- T. Erneux, Applied Delay Differential Equations, Surveys and Tutorials in the Applied Mathematical Sciences Vol. 3, Springer, Berlin, 2009.
- W.S.C. Gurney, S.P. Blythe, and R.M. Nisbet, Nicholson's blowflies revisited, Nature 287 (1980), pp. 17–21. doi: 10.1038/287017a0
- J.K. Hale and S.M. Verduyn Lunel, Introduction to Functional Differential Equations, Applied Mathematical Sciences Vol. 99, Springer-Verlag, Berlin, 1993.
- M. Iannelli, Mathematical Theory of Age-structured Population Dynamics, Applied Mathematics Monographs Vol. 7, Giardini editori e stampatori, Pisa, Italy, 1995.
- Y.A. Kuznetsov, Elements of Applied Bifurcation Theory, 3rd ed., Springer-Verlag, Berlin, 2004.
- M.C. Mackey, Unified hypothesis for the origin of aplastic anemia and periodic hematopoiesis, Blood 51 (1978), pp. 941–956.
- A.J. Nicholson, An outline of the dynamics of animal populations, Aust. J. Zool. 2 (1954), pp. 9–65. doi: 10.1071/ZO9540009
- A.J. Nicholson, The self-adjustment of populations to change, Cold Spring Harb. Symp. Quant. Biol. 22 (1957), pp. 153–173. doi: 10.1101/SQB.1957.022.01.017
- L. Pujo-Menjouet and M.C. Mackey, Contribution to the study of periodic chronic myelogenous leukemia, C. R. Biol. 3 (2004), pp. 235–244. doi: 10.1016/j.crvi.2003.05.004
- L. Pujo-Menjouet, S. Bernard, and M.C. Mackey, Long period oscillations in a g0 model of hematopoietic stem cells, SIAM J. Appl. Dyn. Syst. 4 (2005), pp. 312–332. doi: 10.1137/030600473
- P.L. Simon, H. Farkas, and M. Wittmann, Constructing global bifurcation diagrams by the parametric representation method, J. Comput. Appl. Math. 108 (1999), pp. 157–176. doi: 10.1016/S0377-0427(99)00108-9
- H.L. Smith, An Introduction to Delay Differential Equations with Applications to the Life Sciences, Texts in Applied Mathematics Vol. 57, Springer, Berlin, 2011.
- G. Stépán, Retarded Dynamical Systems: Stability and Characteristic Equations, Longman Scientific and Technical, Essex, UK, 1989.