Abstract
In this paper, a two-strain model that links immunological and epidemiological dynamics across scales is formulated. On the within-host scale, the two strains eliminate each other with the strain with the larger immunological reproduction persisting. However, on the population scale superinfection is possible, with the strain with larger immunological reproduction number super-infecting the strain with the smaller immunological reproduction number. The two models are linked through the age-since-infection structure of the epidemiological variables. In addition, the between-host transmission and the disease-induced death rate depend on the within-host viral load. The immunological reproduction numbers, the epidemiological reproduction numbers and invasion reproduction numbers are computed. Besides the disease-free equilibrium, there are two population-level strain one and strain two isolated equilibria, as well as a population-level coexistence equilibrium when both invasion reproduction numbers are greater than one. The single-strain population-level equilibria are locally asymptotically stable suggesting that in the absence of superinfection oscillations do not occur, a result contrasting previous studies of HIV age-since-infection structured models. Simulations suggest that the epidemiological reproduction number and HIV population prevalence are monotone functions of the within-host parameters with reciprocal trends. In particular, HIV medications that decrease within-host viral load also increase overall population prevalence. The effect of the immunological parameters on the population reproduction number and prevalence is more pronounced when the initial viral load is lower.
1. Introduction
Mathematical models have a long history of enriching our knowledge of immunological and epidemiological processes within their separate scales. Viral and bacterial dynamics mathematical models have contributed immensely to our understanding of the within-host interplay of the pathogen with the host immune system in diseases such as HIV, Hepatitis C and malaria (see e.g. [Citation14,Citation21,Citation30] and the references therein). Population-level dynamics of many diseases has been studied by many authors and numerous books and review articles have been devoted to the between-host interactions (see e.g. [Citation7,Citation18] and the references therein). Epidemiological models of infectious diseases have been used in the past hundred years to answer critical public health questions.
The importance of linking mathematical immunology and mathematical epidemiology cannot be underestimated particularly in the light of pathogen evolution and competition [Citation3,Citation17]. Since the seminal paper of Gilchrist and Sasaki [Citation15] that nests a simple within-host model within a susceptible–infected (SI)–resistant epidemic model, a number of authors have considered the connection between these two scales, accounting for the epidemiological scale mostly though the epidemiological reproduction number [Citation2,Citation19,Citation24].Another approach that commonly has been applied is to link the within-host dynamics with the between-host dynamics when both are described by ordinary differential equation models. Such an approach has been used in a number of articles, but it typically does not separate the different time scales on which immunological and epidemiological processes progress [Citation9]. Of course, many articles have followed the nested approach of Gilchrist and Sasaki [Citation4,Citation22].
In this paper, we use the dynamical approach in [Citation15] to formulate a multi-scale immuno-epidemiological model of HIV [Citation13]. A different multi-scale approach to immuno-epidemiological modelling of HIV has been proposed by Milner and Sega [Citation25]. HIV's high virulence and mutation rates make that virus the preferred choice for the study of the evolution of pathogens across the scales [Citation20]. Coombs et al. [Citation8] were the first to use a unified approach to modelling competition of parasites at both the within- and between-host levels. In their model, however, infected individuals are continuously structured by the initial inoculum and an explicit trade-off mechanism for competition between the strains on population level is not modelled. Consequently, the authors find that in the absence of mutation on the within-host scale, the strain that maximizes the between- host reproduction number out-competes the other strain.
In the present article we investigate a scenario where the two strains are subjected to a complete competitive exclusion on the within-host scale. In the context of competitive exclusion, the strain with the maximal immunological reproduction number dominates. The two strains might not have necessarily emerged through mutation from one another. They differ in many of their characteristics and may belong to different subtypes of HIV [Citation39,Citation40]. On the between-host scale, the strain with the higher immunological reproduction number can super-infect individuals infected with the strain with the lower reproduction number. Because in our framework, the strain with the higher within-host reproduction number takes over almost instantaneously, individuals in the population are infected with only one of the strains. Super-infection is a coexistence mechanism considered previously predominantly on an epidemiological level in the context of evolution of virulence [Citation5]. It is closely related to co-infection and some authors argue that super-infection follows from co-infection when the co-infected class equilibrates much faster [Citation1,Citation26]. In that sense, our results derived here for super-infection may also apply to co-infection.
We use the immuno-epidemiological model that we formulate to address two questions:
First, an early epidemiological model of HIV structured by age-since-infection suggested that the variability of infectivity with age-since-infection which presumably follows the within-host viral load, may destabilize the epidemiological dynamics and lead to sustain oscillations [Citation37]. Our model takes the dependence of the infectivity on the viral load explicitly. Consequently, we address the question: Do single-strain periodic solutions exist in the immuno-epidemiological model of HIV? As we show, for the simplest dependence of the transmission and disease-induced death rate on the dynamic within-host viral load, the single-strain equilibrium is locally asymptotically stable and Hopf bifurcation does not occur. We hypothesize that the single-strain model does not exhibit oscillations. We point out that the result that rules out oscillations concerns the boundary (semi-trivial) equilibria, and not the coexistence equilibria.
The second question that we address is: How do the within-host parameters affect the epidemiological reproduction number, prevalence of HIV, and invasion capabilities of the two strains? We find that the epidemiological reproduction number is increasing with within-host parameters that increase the within-host reproduction number, and decreasing with those that decrease the within-host reproduction number. The within-host reproduction number does not depend on the infected cell death rate, and it is not immediately clear how the viral load depends on this parameter. We find that the epidemiological reproduction number is an increasing function of the infected cell death rate. The prevalence seems to depend in an exactly opposite manner on the within-host parameters. That suggests that HIV medications which decrease healthy cells infection rate β and/or the number of virions produced by an infectious cell ν in fact increase the population level of the prevalence of HIV – a trade-off also observed in practice. Furthermore, our results imply that the sensitivities of the epidemiological reproduction number and prevalence with respect to the immunological infection parameters are decreasing functions of the initial viral load, suggesting that by decreasing the amount of virus transmitted HIV medications also increase the sensitivity of the epidemiological parameters with respect to immunological parameters. Finally, we find that the epidemiological invasion reproduction number of strain one decreases with the increase in the immunological reproduction number of strain two, while the invasion reproduction number of strain two increases with increase in the immunological reproduction number of strain two. Although, which strain persists and which strain dies out on the population level is governed by the epidemiological invasion numbers, in the majority of the simulations this relationship depends strongly on the relationship between the within-host reproduction numbers. That is, we find out that the most common outcome is that the strain with the largest immunological reproduction number dominated on the population level.
We formulate our multi-scale model of HIV in the next section. We use a standard immunological and a standard SI epidemiological models. The two models are linked through age-since-infection and through the epidemiological parameters which depend on the within-host viral load. In Section 3 we discuss the trivial and semi-trivial equilibria and their stability. The local stability of the isolated-strain equilibria suggests that sustained oscillations in the single-strain dynamics do not occur. In Section 4 we establish the existence of an interior equilibrium under the condition that both epidemiological invasion numbers are larger than one. Section 5 is devoted to elucidating the connection between within-host viral dynamics and the epidemiology of HIV.
2. A two-strain immuno-epidemiological model with superinfection
In this section we formulate a two-strain immuno-epidemiological model of HIV with superinfection. Individuals infected with strain i experience within-host dynamics, given by a standard immunological single-strain model of HIV. Within a host, the time variable is denoted by τ and signifies time-since-infection. Upon infection, HIV attacks the CD4+ T lymphocytes, which we call the target cells and we denote by x when healthy and by yi when infected by the ith strain of the virus. The number of free virions of strain i is denoted by Vi.
In this model, uninfected target cells are created at a constant rate r and are infected by interactions with free virions of strain i according to a mass action law with rate constant βi. Productively infected cells, yi, produce νi>1 new virions of strain i. The healthy target cell death rate is μ, and cells infected with strain i have death rate di. The parameter δi denotes the strain i viral clearance rate, and si is the shedding rate of strain i. Shedding rate is given by the amount of virus that leaves an infected individual and may serve to infect another individual. The within-host model of strain i is given by the following system of ordinary differential equations:
All parameters and dependent variables of this within-host model and their definitions can be found in . Variations of model (1) have been studied extensively and have been commonly used in the investigation of HIV [Citation31,Citation34] and Hepatitis C dynamics [Citation27]. Mathematically, model (1) has been completely analysed [Citation12].
Table 1. List of parameters.
The within-host reproduction number of strain i in model (1) is computed as follows:
The reproduction number of strain i gives the numbers of secondary infections that one virus i-infected cell will produce in entirely healthy cell population during its lifespan as infected. Model (1) always has an infection-free equilibrium E0=(r/μ, 0, 0), and if ℝi>1, then the infected equilibrium Ei=(xi*, yi*, Vi*) exists with
We now introduce a two-strain epidemiological model with superinfection in the host. We denote by S(t) the number of susceptible human individuals, where t is the chronological time. We structure the infected individuals by age-since-infection τ. Let ij(τ, t) be the density of individuals infected by strain j. Individuals in the class ij(τ, t) experience the same within-host dynamics given by model (1) for strain j. We assume that the epidemiological dynamics of the HIV infection is driven by the following two-strain model with super-infection:
In model (3) Λ is the birth/recruitment rate and m0=mj(0) is the natural death rate of susceptible hosts at zero viral load. Here, we assume that all susceptible individuals have approximately the same equilibrium level of healthy CD4+ T cells. Furthermore, the infected hosts die at a variable rate dependent on their viral load mj(Vj(τ)). We will assume the simplest form of mj(Vj(τ)), that is,
where mjVj(τ) gives the additional host mortality due to the virus. It should be noted that there is an intrinsic issue with this assumption. While the increased viral load during the AIDS phase definitely impacts mortality, this is not the case for the early peak in the viral load. Immediately after infection the viral load grows exponentially, peaks at high levels and then drops low for the asymptomatic phase. This early peak is captured by our within-host model and would imply increased mortality. However, that is not what is observed in practice, where the acute phase causes only mild flu-like symptoms. One way to remedy this is to connect the disease-induced mortality to the T-cell count but this approach also has issues. Another is to assume that mj is, say, a step function, which is zero (or very small) during the acute phase, and a constant afterwards. We obtain our results for a constant value of mj, and note that these results may not extend if mj is in fact a step function. The transmission coefficient of HIV βj(Vj(τ)) is also dependent on the within-host viral load. We may assume that βj(Vj(τ)) is proportional to the viral load at a given age-since-infection τ. Hence,
where Vj(τ) is the viral load of the strain j, qj represents the probability of successful transmission and sj is the shedding rate of strain j. We notice that in the most general case we assume that not only the two strains have different within-host dynamics but they also transmit differently, shed differently and cause different virulence. HIV is a highly mutable pathogen. It is very likely that there are significant differences between two strains which potentially may be from different groups and subtypes. The total population size is given by the sum of all classes:
We assume that individuals are subjected to super-infection [Citation29] in the sense that individuals infected with strain one can get into contact with individuals infected with strain two and get themselves infected by strain two. Vice versa, individuals infected with strain two can get into a contact with individuals infected with strain one and get themselves infected by strain one. The super-infection occurs at a reduced rate where the coefficient of reduction ηi satisfies ηi<1. We note that individuals infected with strain i who get super-infected with strain j have a coefficient of reduction ηi.
We assume that one of the strains ‘takes over’ almost instantaneously, but during the short span when the super-infected individuals are actually infected with both strains, the within-host dynamics of the two variants is governed by the following within-host model:
The long-term outcome of the competition of the two strains in the above model is competitive exclusion. The reproduction numbers of the two strains are again given by Equation (2). The strain with the larger reproduction number wins and eliminates the strain with the smaller reproduction number. Model of the form (4) has first been used in [Citation6,Citation16]. Competitive exclusion has first been proven rigorously by De Leenheer and Pilyugin [Citation11].
The parameters Pj take on values zero and one and control the direction of the super-infection. The values Pj depend on the size of the reproduction numbers ℝ1 and ℝ2 corresponding to virus strain one and strain two. They can be expressed as follows:
We note that if ℝ2>ℝ1 strain two super-infects individuals infected with strain one, that is P1=1 and vice versa. The parameters P1 and P2 are not probabilities, but a binary tool to capture two symmetric models in one.
Model (3) is equipped with the following initial conditions: S(0)=S0 and ij(a, 0)=ϕj(a). All parameters are nonnegative and Λ>0, cj>0, m0>0.
The epidemiological reproduction number of strain j in system (3) is given by the following expression:
The reproduction number of strain j gives the number of secondary infections that one strain j-infected individual will produce in an entirely susceptible population during its lifespan as infectious. This is the reproduction number of the single-strain model and does not depend on the interaction between the strains, that is it includes no super-infection effects.
In the next section we compute explicit expressions for the equilibria and establish their local stability.
3. Disease-free and strain dominance equilibria and their local stability
System (3) has one disease-free equilibrium and two single-strain equilibria. The disease-free equilibrium, in which infection is not present, always exists and is given by
where
In addition, if the basic reproduction number
, for each j there is a corresponding single-strain equilibrium
given by
where
and
The total population size Nj* at the strain j dominance equilibrium is
We note that the system also may have an interior equilibrium point. We discuss this option in Section 4.
Next, we turn to the linearized equations of the disease-free equilibrium. To introduce the linearization at the disease-free equilibrium , let S(t)=S0+x(t), ij(τ, t)=zj(τ, t). The linearized system becomes
We look for solutions of the form x(t)=x̄ eλ t, zj(τ, t)=z̄j(τ) eλ t. For each j we obtain the following eigenvalue problem:
Note that S0*/N0*=1. Solving the differential equation, we obtain
and replacing z̄j in the equation for z̄j(0), we obtain the following characteristic equation for strain j:
where
Now we are ready to establish the following result.
Proposition 3.1
If
then the disease-free equilibrium is locally asymptotically stable. If
it is unstable.
Proof
Assume
Consider λ with ℜ λ≥0. For such λ and each j we have
Hence, the equations
do not have a solution with nonnegative real part and the disease-free equilibrium is locally asymptotically stable.
Now assume
For that fixed j and λ real we have
. Furthermore,
. Hence, the equation
has a real positive root. Therefore, the disease-free equilibrium is unstable. ▪
To investigate the local stability of the endemic equilibrium for a fixed j, we recall that strain j dominance equilibrium
exists if and only if the basic reproduction number corresponding to this strain
is greater than 1. So we assume that
for a fixed j. The local stability of the strain j dominance equilibrium depends on the invasion reproduction numbers
and
for each of the strains. By definition, the invasion reproduction number of the first strain
gives the number of secondary infections that one infected individual with the first strain will produce in a population in which the second strain is at equilibrium. The invasion reproduction numbers of the first and the second strains in our case are given by
where
The results on local stability of single-strain equilibrium
are summarized below
Proposition 3.2
Assume for a fixed j, . Then the single-strain equilibrium
is locally asymptotically stable if
for i, j=1, 2, and i≠j. The single-strain equilibrium
is unstable if
.
Proof
To simplify the presentation, we will assume without loss of generality that j=1, that is . We study the linearized equation around the single-strain equilibrium
. We introduce the following notation for the perturbations
,
, i2(τ, t)=z2(τ, t), N=N1*+n. The system for the perturbations becomes
An approach similar to [Citation23] (see Appendix B in [Citation23) can show that the linear stability of the system is in fact determined by the eigenvalues of the linearized system (10). To investigate the point spectrum, we look for exponential solutions (see the case of the disease-free equilibrium) and obtain a linear eigenvalue problem. The equation for strain two separates from the whole system. We note that
The system for the perturbations of strain two takes the form:
Let
From Equation (11) it follows that
Substituting z̄2(τ) in the equation for Z2, dividing Z2 from both side of the resulting equation, we get the following characteristic equation:
We denote the right-hand side of that equation with
, that is
It can be seen that
is a decreasing function of λ for real λ. Furthermore,
and
. If
, then Equation (12) has at least one positive root, and therefore
is unstable. If
, for λ with ℜλ≥0, we have
Hence, the equation
has no solution with positive real part and all the eigenvalues of this equation have negative real part. Therefore, the stability of
depends on the eigenvalues of the following system:
First, we solve the differential equation
Before we continue, we adopt the following notations
From the first and third equation in Equation (13) we obtain
Therefore,
Linearizing the equation for the total population size
we obtain
It follows from the third equation of Equations (13) and (15) that
Substituting Equations (15) and (16) in the first equation in Equation (13), and then cancelling z1(0) from both sides of the resulting equation, we obtain the following characteristic equation for
where
Here, we use the fact that
From β1(V1(τ))=c1V1(τ) and m1(V1(τ))=m0+m1V1(τ) it follows that
That is,
Evaluated for λ=0 the above equation also gives
Note that
Substituting above relations in Equation (17) we can rewrite the characteristic equation in the following form:
For λ with ℜλ≥0 we have
On the other hand,
implies m1/c1<1. The quotients mi/ci are important quantities and will be used often. Hence, we adopt the following notation for them:
We have that for λ with ℜλ≥0, the following inequality holds:
Hence, for λ with ℜλ≥0, the characteristic equation
has only solutions with negative real parts. Thus, the single-strain equilibrium
is locally asymptotically stable. This concludes the proof. ▪
In conclusion we notice that the results in this section imply that in the single-strain case the endemic equilibrium will be locally stable. Furthermore, the results can be extended to an n-strain scenario, although an appropriate assumptions for the super-infection among n strains have to be made.
4. Existence of the interior equilibrium
In the previous sections we discussed the existence of disease-free equilibrium and strain dominance equilibria and established the local stability of the equilibria under biologically interpretable conditions. In this section, we consider the existence of an interior equilibrium for which both strains are present. We show an interior equilibrium exists under the conditions that the two basic reproduction numbers and the two invasion reproduction numbers
corresponding to each strain are all greater than one. For simplicity, and without loss of generality, we assume that the two basic reproduction numbers in the immunological system satisfy ℝ1>ℝ2, in this case, P1=0 and P2=1. Assume
is the co-existence equilibrium of the system, then it satisfies the following equations:
Setting
Then system (19) becomes
First, we solve the second differential equation in (20)
Solving the first differential equation in (20) and replacing i2*(τ) in the resulting equation from (21), we obtain
Substituting Equation (22) in the expression of Q1 and canceling Q1, we obtain
Substituting Equation (21) in the expression of Q2 and then cancelling Q2, we have
From the first equation in (20) and the fact that
it follows that
To derive the equation for N* in terms of Q1 and Q2 we integrate the equations for i1*(τ) and i2*(τ) in system (20) and add them. We obtain
Hence,
This gives the following expression of N* in terms of Q1 and Q2:
With expressions (25) and (27) for S* and N* in terms of Q1 and Q2 we obtain the following system in Q1 and Q2:
To show the existence of an interior equilibrium, we have to show that this system has a nonzero solution (Q1, Q2). System (28) is nonlinear and cannot be explicitly solved. To show the existence of nonzero solution, we introduce the following notation:
From Equations (24) and (25) (or the second equation in (28)) it follows that Q2 can be expressed as a continuous function of Q1:
We use this expression for Q2 to eliminate Q2 from the first equation in system (28). Consequently, we obtain an equation in Q1 only:
, where
The existence of an interior equilibrium for Equation (19) is now reformulated as the existence of at least one positive Q1 satisfying
for which Q2 as computed from Equation (29) is nonzero. In what follows, we will prove this fact. Let Q1=0, from Equation (29) we get the corresponding Q̄2* and it can be expressed as
We note that in the expression above we have
. Hence, Q̄2* is well defined and positive. It is easy to show that
Let Q2=0. From Equation (23) we obtain that the corresponding Q̄1* can be expressed as
We note that in the expression above, we have
. Hence, Q̄1* is well defined and positive. If Q2=0, then from Equation (29) we have
Now we define a function
It is easy to see that
, F′(Q1)<0, and also
Therefore, there exists a unique Qˆ1* such that
Note that
Hence,
Because F(Q1) is a decreasing function, the above inequality implies that Q̄1*<Qˆ1*. Then, we have
Combining Equations (31) and (32) we conclude that the equation has a positive solution. It is easy to establish that for that value of Q1, the corresponding Q2 is also positive. This establishes the existence of positive solution of system (28), and hence the existence of an interior equilibrium. We summarize the result in the following proposition.
Proposition 4.1
Assume and
. Then (3) has at least one co-existence equilibrium.
5. How does within-host infection affect population level of disease?
Within-host variability of disease progression in HIV is significant. How does the within-host disease transmission affect the epidemiological prevalence of HIV? This is one of the most critical question that can be addressed with immuno-epidemiological models. The main approach to this question, however, is primarily through simulations as explicit solution of the immune HIV system is not feasible. In the next subsection, we estimate the parameters of the linked immuno-epidemiological model.
5.1. Parameter estimation
Two groups of parameters need to be estimated: the epidemiological parameters and the immunological parameters. We set the time unit of t and τ to be in days. A number of articles estimate parameters for the standard within-host HIV model [Citation31,Citation36, particularly viral clearance rate and infected T cells life-span. Considerable variability exits in estimates of the viral clearance rate. Perelson et al. [Citation31] give the viral clearance rate δ≈ 3 days−1. We assume the baseline value for the viral clearance of strain one δ1. Faster viral clearance rates from the plasma have also been suggested, for instance δ2≈ 23 days−1 [Citation10]. We assume this faster clearance rate for strain two. These two very different viral clearance rates correspond to different viral production numbers. Snedecor [Citation35] uses 250 particles per infected cell, which we adopt for the first strain ν1=250, but De Boer et al. [Citation10] suggest much larger numbers. Given the much larger clearance rate, we take ν2=1000.
Perelson et al. [Citation31] also estimate the infected cell life-span given by 1/d. It gives a value of d1≈ 0.5. This is the value we assume for strain one. Now it is widely assumed that the infected cell life-span is about 1 day. Consequently, we assume for strain two d2=1. The uninfected CD4+ T-cell population in the human blood is given to be 5*109 in [Citation35]. Stafford et al. [Citation36] give the death rate of uninfected T cells at about μ=0.01. Since the equilibrium of uninfected T cells in the model is given by r/μ, then r=5*107 T cells per day.
The shedding rate is different for the acute and chronic stage of infection. Several references give information about the viral load in copies per millilitre in the vaginal tract of HIV-infected women and in the semen of infected men. Estimating the total amount of virus shed is possible for the males. Pilcher et al. [Citation32] give the mean plasma viral load in the chronic stage as approximately 100,000 copies/mL. Average 190 lb male has approximately 6000 mL of blood so the total viral load is approximately 6*108. Pilcher et al. [Citation32] also give the viral load in the semen as approximately 8000 copies/mL. The mean amount of semen for an average male is 5 mL so that the total amount of virus in the semen is 48,000 copies RNA. That gives a shedding rate of about s1=0.00008, which we adopt for strain one. For strain two, we adopt twice as big shedding rate s2=0.00015. The amount of virus in the semen can help estimate the initial viral load V0. If all the amount of virus in the semen is absorbed and passed into the blood, then the initial V0≈ 20,000 viral particles, considering that one viral particle has two RNA copies. We vary V0 within the range (1000, 100,000). These parameters give an immune reproduction number of strain one of ℝ1=6.22 and of strain two of ℝ2=6.95. These reproduction numbers are similar to the median within-host reproduction number of 5.7 reported by Stafford et al. [Citation36]. With these parameters, the equilibrium viral load in the blood is computed to be 3.6*109 which is consistent with what is reported in [Citation35].
To estimate the epidemiological parameters, we take the probability of transmission q1=q2=1, so that c1=0.00008 and c2=0.00015. We define m1 and m2 so that the resulting epidemiological reproduction number falls within the reasonable for HIV range. Nishiura [Citation28] reports epidemiological reproduction numbers in the range 3.5−4 for west European countries. That gives an estimates for m1=0.00002 and for m2=0.00004.
To determine the population demographic parameters, we obtain the average human lifespan from [Citation33] to be 70 years. Hence, m0=1/(365*70). Population Reference Bureau also gives the total human population in 2011 as nearly 7 billion people. That gives Λ≈ 275,000. Parameter values are listed in .
Table 2. Values of the within-host and epidemiological parameters.
5.2. How do the within-host transmission parameters impact the epidemiological reproduction number and HIV prevalence?
To answer this question we plot the epidemiological reproduction number as a function of the immunological and epidemiological parameters. In this subsection, we focus on strain one only. The dependence of the viral load Vj(τ) on the infection rate βj is not evident by inspection of the within-host system. Plotting the epidemiological reproduction number as a function of βj reveals that the epidemiological reproduction number is an increasing function of βj (). Furthermore, reveals that even for very small βj, the epidemiological reproduction number is well over one. At first this observation seems counterintuitive. To understand this result, we notice that after integration by parts the epidemiological reproduction number can be written as
Assume now that βj=0 and yj≈ 0. Then,
Hence,
In Equation (33) under the integral we have an exponent of the following integral:
even when Vj(0)=500. Raising the exponent to that number results in a small number. Consequently, the value of the integral in formula (33) is small. This means that the epidemiological reproduction number does not depend in a significant way on the within-host dynamics, and we have
which is what we observe in .
Figure 1. Epidemiological reproduction number vs. the infection rate of blood T cells β and the viral clearance rate δ.
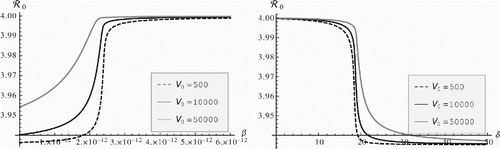
Plotting the epidemiological reproduction number against each within-host parameter reveals that the reproduction number is a monotone function of the parameter when the remaining parameters are fixed as strain one in . The reproduction number is also an increasing function of r, ν and d. However, the reproduction number is a decreasing function of δ and μ. To produce (left), we have taken β as a parameter, solved the within-host single-strain system, obtaining V(τ). Now V(τ) also depends on the parameter β and from this, we have computed as a function of β. The simulation is executed with the computer algebra system Mathematica.
For the parameters of the within-host system, also shows that the reproduction number is bounded and tends to a finite limit as an immunological parameter increases. The value of the limit is not sensitive to the virus initial amount V0. We notice that the epidemiological reproduction number changes over a very small range as the within-host parameter varies. Maximum change of the epidemiological reproduction number occurs at a critical value of the parameter which makes the immunological reproduction number equal to one.
The epidemiological reproduction number is much more sensitive to the epidemiological parameters. It increases when the shedding rate increases and does so almost linearly, without an upper bound (). increases with s and decreases with m0 and m1. shows that as a function of s, the epidemiological reproduction number does not depend on the virus initial amount V0.
The dependence of the HIV prevalence on each immunological parameter is also monotone when the remaining parameters are fixed at values listed in as strain one. We define the total prevalence at an equilibrium as
The dependence of the total prevalence on within-host parameters, however, seems reversed compared with the reproduction number. For instance, HIV total prevalence is decreasing with increasing β (). This dependence implies the somewhat surprising result that increasing β, which increases the within-host viral load, decreases the equilibrial prevalence of the disease. In fact this dependence mimics correctly the current situation with HIV, where various drugs reduce the within-host viral load, including through reduction of β, and extend the lifespan of infected individuals. As a result, even though the incidence is declining, since infected individuals live longer, the overall prevalence is rising [Citation38]. The immuno-epidemiological model in this article captures this trade-off between treatment and prevalence in HIV. We believe this results is predicated on our assumption that the disease-induced mortality is governed by the within-host viral load so that when the within-host viral load is reduced, so is the disease-induced mortality. We believe this effect will be retained even if the disease-induced mortality is dependent on the T cells rather than the viral load. Reducing β will reduce the viral load, keep the T-cell load larger and consequently the disease-induced mortality small.
Figure 3. HIV prevalence vs. the infection rate of blood T cells β. HIV prevalence decreases with increased infection rate and viral load.
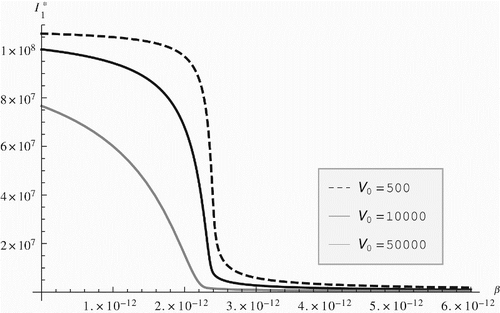
Elasticity is a concept that measures the responsiveness of a quantity Q with respect to a parameter p. Elasticity is defined by the following formula:
The elasticity
gives the percent change in the quantity Q given 1% change in p. The elasticity is positive if Q is increasing with p, and negative if Q is decreasing with p. We investigate the elasticity of the epidemiological reproduction number and the prevalence with respect to the within-host infection-related parameters: ν, β, d and δ. The elasticities of
with respect to ν, β, d are positive, and the elasticity of
with respect to δ is negative. The elasticities of the prevalence I1 with respect to ν, β, d are negative, and the elasticity of I1 with respect to δ is positive. To compare the magnitude of the elasticities with respect to the different within-host parameters, we plot the absolute values of the elasticities in . Furthermore, we plot the magnitude of the elasticities with respect to the initial viral load V0. Because the initial viral load potentially varies within a wide range, and may change as medication is applied, we would like to know how the magnitude of the sensitivities changes with respect to the average initial viral load.
Figure 4. Magnitude of the elasticities of ℛ0 (left figure) and prevalence I1 (right figure) with respect to within-host infection parameters. The elasticities are plotted as a function of the initial viral load V0 and are colour-coded. Red, pink colours correspond to positive values, black and blue to negative values.
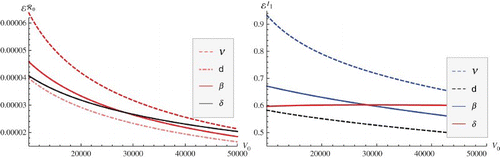
shows that the epidemiological reproduction number is less sensitive to the within-host infection parameters than the prevalence. Furthermore, the magnitudes of the elasticities are generally decreasing functions of the initial viral load V0. In other words, as the average initial viral load in the population decreases, the epidemiological reproduction number and the prevalence become more sensitive to the within-host parameters. Put in the public health perspective, this suggests that HIV medications that decrease ν or β in fact act in two ways: by decreasing the individual viral load, and by increasing the sensitivity of key epidemiological quantities such as the prevalence and the incidence to the change of these parameters. In other words, decreasing the average initial viral load in the population leads to higher impact of these medications on these key epidemiological parameters.
5.3. How does within-host infection affect the invasion numbers of each strain?
The population-level prevalence of each strain is governed by the population invasion reproduction numbers given by expressions (9). In general, the numbers suggest the following outcomes of the population-level competition: (1) If and
, strain two excludes strain one; (2) if
and
, strain one excludes strain two; (3) if
and
, the two strains coexist; (4) if
and
, one of the strains excludes the other based on the initial conditions. To determine the impact of within-host competition of the strains on the population-level competition, we investigate the impact of the within-host competition on the population-level invasion reproduction numbers.
shows that the invasion reproduction number is a decreasing function of the within-host reproduction number of strain two ℝ2 while
is an increasing function of the within-host reproduction number of strain two ℝ2. Furthermore the invasion reproduction numbers exhibit the same trends of change when the initial viral load for strain two increases. Both reproduction numbers experience a jump at the critical value of ℝ2 when ℝ2=ℝ1. The increase in ℝ2 affects much more significantly
when ℝ2<ℝ1 but when ℝ2>ℝ1
is most sensitive to the change of ℝ2. More importantly, if ℝ2<ℝ1 then
and
signifying that strain one excludes strain two on within-host levels as well as on the population level. If, however, ℝ2>ℝ1 then
and
leading to elimination of strain one on within-host levels as well as on the population level. This scenario, depicted in , where the within-host reproduction numbers control the population level of the competition between the strains, was the most common scenario produced in simulations. As η1 and η2 vary other scenarios are also possible. For instance, another scenario we produced was one where strain one remains dominant as the immunological reproduction number of strain two increases so that ℝ2>ℝ1. One uncommon and difficult scenario to produce was the case when both invasion reproduction numbers are larger than one with the parameters in or their variations. However, we were able to find a case in which
and
with the parameters in and η1=0.02, η2=0.027. This scenario is illustrated in . Thus, demonstrates that coexistence in our model indeed occurs. The location of that coexistence equilibrium in the (Q1, Q2) plane is given by the point (644, 6702).
Figure 5. Plots of the invasion reproduction number (left figure) and the invasion reproduction number
(right figure) with respect to within-host reproduction number of strain two ℝ2. Several variants of the invasion reproduction numbers are plotted for different values of the initial viral load of strain two V02. Here, η1=0.1 and η2=0.005.
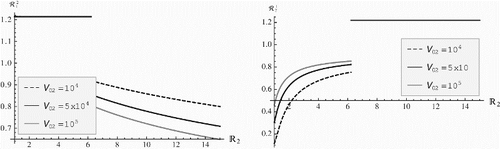
Acknowledgements
Maia Martcheva acknowledges partial support from NSF grant DMS-0817789 and grant DMS-1220342, Xue-Zhi Li acknowledges partial support from NSF of China grants 11271314 and 10911120387. This work was performed when Xue-Zhi Li was visiting the Department of Mathematics, University of Florida from January to March, 2012. Xue-Zhi Li greatly appreciates the hospitality of his hosts.
References
- F.R. Adler and J. Mosquera, Super- and coinfection: Filling the range, in Adaptive Dynamics of Infectious Diseases: In Pursuit of Virulence Management, U. Dieckmann, J.A.J. Metz, M.W. Sabelis, and K. Sigmund, eds., Cambridge University Press, Cambridge, 2002, pp. 138–149.
- S. Alizon and S. Lion, Within-host parasite cooperation and the evolution of virulence, Proc. R. Soc. B 278 (2011), pp. 3738–3747. doi: 10.1098/rspb.2011.0471
- S. Alizon, F. Luciani, and R.R. Regoes, Epidemiological and clinical consequences of within-host evolution, Trends Microbiol. 19(1) (2011), pp. 24–32. doi: 10.1016/j.tim.2010.09.005
- J.B. André and S. Gandon, Vaccination, within-host dynamics, and virulence evolution, Evolution 60(1) (2006), pp. 13–23.
- M. van Baalen and M.W. Sabelis, The dynamics of multiple infection and the evolution of virulence, Am. Nat. 146 (1995), pp. 881–910 doi: 10.1086/285830
- C.L. Ball, M.A. Gilchrist, and D. Coombs, Modeling within-host evolution of HIV: Mutation, competition and strain replacement, Bull. Math. Biol. 69 (2007), pp. 2361–2385. doi: 10.1007/s11538-007-9223-z
- F. Brauer, P. van den Driessche, and J. Wu (eds.), Mathematical Epidemiology, Springer, New York, 2008.
- D. Coombs, M.A. Gilchrist, and C.L. Ball, Evaluating the importance of within- and between-host selection pressures on the evolution of chronic pathogens, Theor. Popul. Biol. 72 (2007), pp. 576–591. doi: 10.1016/j.tpb.2007.08.005
- D.F. Cuadros and G. Garcia-Ramos, Variable effect of co-infection on the HIV infectivity: Within-host dynamics and epidemiological significance, Theor. Biol. Med. Model. 9(9) (2012). doi:10.1186/1742-4682-9-9
- R.J. De Boer, R.M. Ribeiro, and A.S. Perelson, Current estimates for HIV-1 production imply rapid viral clearance in lymphoid tissues, PLoS Comput. Biol. 6(9) (2010), pp. e1000906.
- P. De Leenheer and S.S. Pilyugin, Multistrain virus dynamics with mutations: A global analysis, Math. Med. Biol. 25 (2008), pp. 285–322. doi: 10.1093/imammb/dqn023
- P. De Leenheer and H.L. Smith, Virus dynamics: A global analysis, SIAM J. Appl. Math. 63(4) (2003), pp. 1313–1327. doi: 10.1137/S0036139902406905
- S. Debroy and M. Martcheva, Immuno-epidemiology and HIV/AIDS: A modeling prospective, in Mathematical Biology Research Trends, Lachlan B. Wilson, ed., Nova Publishers, New York, 2008, pp. 175–192.
- S. Debroy, B. Bolker, and M. Martcheva, Bistability and long-term cure in a within-host of Hepatitis C, J. Biol. Syst. 19(4) (2011), pp. 533–550. doi: 10.1142/S0218339011004135
- M.A. Gilchrist and A. Sasaki, Modeling host–parasite coevolution: A nested approach based on mechanistic models, J. Theor. Biol. 218(3) (2002), pp. 289–308. doi: 10.1006/jtbi.2002.3076
- M.A. Gilchrist, D. Coombs, and A.S. Perelson, Optimizing within-host viral fitness: Infected cell lifespan and virion production rate, J. Theor. Biol. 229(2) (2004), pp. 281–288. doi: 10.1016/j.jtbi.2004.04.015
- B. Hellriegel, Immunoepidemiology – bridging the gap between immunology and epidemiology, Trends Parasitol. 17(2) (2001), pp. 102–106. doi: 10.1016/S1471-4922(00)01767-0
- H.W. Hethcote, The mathematics of infectious diseases, SIAM Rev. 42(4) (2000), pp. 599–653. doi: 10.1137/S0036144500371907
- R.D. Holt and M. Barfield, Within-host pathogen dynamics: Some ecological and evolutionary consequences of transients, dispersal mode, and within-host spatial heterogeneity, DIMACS Ser. Discrete Math. Theor. Comput. Sci. 71 (2006), pp. 45–66.
- P. Lemey, A. Rambaut, and O. Pybus, HIV evolutionary dynamics within and among hosts, AIDS Rev. 8 (2006), pp. 125–140.
- Y. Li, S. Ruan, and D. Xiao, The within-host dynamics of malaria infection with immune response, Math. Biosci. Eng. 8(4) (2011), pp. 999–1018. doi: 10.3934/mbe.2011.8.999
- M. Martcheva, An immuno-epidemiological model of paratuberculosis, AIP Conference Proceedings, AIP Publishing, Melville, NY, Vol. 1404, 2011, pp. 176–183.
- M. Martcheva and H.R. Thieme, Progression age enhanced backward bifurcation in an epidemic model with super-infection, J. Math. Biol. 46 (2003), pp. 385–424. doi: 10.1007/s00285-002-0181-7
- G.F. Medley, The epidemiological consequences of optimisation of the individual host immune response, Parasitology 125 (2002), pp. S61–S70. doi: 10.1017/S0031182002002354
- F.A. Milner and L.M. Sega, Integrating immunological and epidemiological models, 18th World IMACS/MODSIM Congress, Cairns, Australia, 13–17 July 2009. Available at http://mssanz.org.au/modsim09
- J. Mosquera and F.R. Adler, Evolution of virulence: A unified framework for coinfection and superinfection, J. Theor. Biol. 195 (1998), pp. 293–313. doi: 10.1006/jtbi.1998.0793
- A.U. Neumann, N.P. Lam, H. Dahari, D.R. Gretch, T.E. Wiley, T.J. Layden, and A.S. Perelson, A model for ovine brucellosis incorporating direct and indirect transmission, Hepatitis C virus dynamics in vivo and the antiviral efficacy of interferon-alpha therapy, Science 282 (1998), pp. 103–107. doi: 10.1126/science.282.5386.103
- H. Nishiura, Correcting the actual reproduction number: A simple method to estimate R0 from early epidemic growth data, Int. J. Environ. Res. Public Health 7 (2010), pp. 291–302. doi: 10.3390/ijerph7010291
- M.A. Nowak and R.M. May, Superinfection and the evolution of parasite virulence, Proc. R. Soc. Lond. B 255 (1994), pp. 81–89. doi: 10.1098/rspb.1994.0012
- M.A. Nowak and R.M. May, Virus Dynamics: Mathematical Principles of Immunology and Virology, Oxford University Press, Oxford, 2000.
- A. Perelson, A. Neumann, M. Markowitz, J. Leonard, and D. Ho, HIV-1 dynamics in vivo: Virion clearance rate, infected cell life-span, and viral generation time, Science 271 (1996), pp. 1582–1586. doi: 10.1126/science.271.5255.1582
- C.D. Pilcher, G. Joaki, I.F. Hoffman, F.E.A. Martinson, C. Mapanje, P.W. Stewart, K.A. Powers, S. Galvin, D. Chilongozi, S. Gama, M.A. Price, S.A. Fiscus, and M.S. Cohen, Amplified transmission of HIV-1: Comparison of HIV-1 concentrations in semen and blood during acute and chronic infection, AIDS 21(13) (2007), pp. 1723–1730. doi: 10.1097/QAD.0b013e3281532c82
- Population Reference Bureau. Available at http://www.prb.org/DataFinder/Geography/Data.aspx?loc=241 (accessed 2012).
- R.R. Regoes, D. Wodarz, and M.A. Nowak Virus dynamics: The effect of target cell limitation and immune responses on virus evolution, J. Theor. Biol. 191 (2010), pp. 451–462. doi: 10.1006/jtbi.1997.0617
- S.J. Snedecor, Comparison of three kinetic models of HIV-1 infection: Implications for optimization of treatment, J. Theor. Biol. 221 (2003), pp. 519–541. doi: 10.1006/jtbi.2003.3202
- M.A. Stafford, L. Corey, Y. Cao, E.S. Daar, D.D. Ho, and A.S. Perelson, Modeling plasma virus concentration during primary HIV infection, J. Theor. Biol. 203 (2000), pp. 285–301. doi: 10.1006/jtbi.2000.1076
- H.R. Thieme and C. Castillo-Chavez, How may infection-age-dependent infectivity affect the dynamics of HIV/AIDS? SIAM J. Appl. Math. 53 (1993), pp. 1447–1479. doi: 10.1137/0153068
- United Nations, UN Millennium Goals report 2010. Available at http://www.un.org/millenniumgoals/pdf/MDG%20Report%202010%20En%20r15%20-low%20res%2020100615%20-.pdf
- Avert. HIV types, subtypes groups and strains. Available at http://www.avert.org/hiv-types.htm
- Wikipedia. Available at http://en.wikipedia.org/wiki/HIV_superinfection