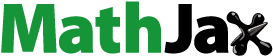
Abstract
Indirect transmission through the environment, pathogen shedding by infectious hosts, replication of free-living pathogens within the environment, and environmental decontamination are suspected to play important roles in the spread and control of environmentally transmitted infectious diseases. To account for these factors, the classic Susceptible–Infectious–Recovered–Susceptible epidemic model is modified to include a compartment representing the amount of free-living pathogen within the environment. The model accounts for host demography, direct and indirect transmission, replication of free-living pathogens in the environment, and removal of free-living pathogens by natural death or environmental decontamination. Based on the assumptions of the deterministic model, a continuous-time Markov chain model is developed. An estimate for the probability of disease extinction or a major outbreak is obtained by approximating the Markov chain with a multitype branching process. Numerical simulations illustrate important differences between the deterministic and stochastic counterparts, relevant for outbreak prevention, that depend on indirect transmission, pathogen shedding by infectious hosts, replication of free-living pathogens, and environmental decontamination. The probability of a major outbreak is computed for salmonellosis in a herd of dairy cattle as well as cholera in a human population. An explicit expression for the probability of disease extinction or a major outbreak in terms of the model parameters is obtained for systems with no direct transmission or replication of free-living pathogens.
1. Introduction
Although direct transmission of infectious diseases from host-to-host is typically considered to be the main route of infection transmission, there are many diseases for which the primary route of transmission is through the environment. Examples of environmentally transmitted infectious agents are Escherichia coli O157:H7 and Salmonella in their animal host populations, and Vibrio cholerae in humans. These environmentally transmitted infectious diseases (ETIDs) can spread through a host population without the need for direct contact between hosts. For example, a susceptible host can become infectious by ingesting a free-living pathogen from the environment. Contaminated food, water, soil, surfaces, and fomites are all possible vehicles for indirect transmission [Citation6,Citation10,Citation14,Citation55]. Some free-living pathogens are capable of long-term survival and even growth within the environment [Citation9,Citation45,Citation58,Citation73]. Moreover, infectious hosts contribute to the load of free-living pathogen in the environment through shedding. This creates a cycle of transmission in which susceptible hosts become infectious by ingesting free-living pathogens from the environment and, in turn, infectious hosts contribute to the level of environmental contamination. On the other hand, the load of free-living pathogens within the environment is reduced by environmental decontamination practices and natural death of the pathogen.
In the theory of infectious disease transmission, a problem of interest is to predict whether the appearance of a small number of infectious individuals (or pathogens) will result in disease extinction or a major outbreak. The basic reproduction number is a well-known threshold which can be used to determine whether an outbreak will occur. The basic reproduction number, denoted by , is defined as the expected number of secondary infections produced by a typical infectious individual during its infectious period in a completely susceptible population [Citation20]. In general, if
, there will be an outbreak which results in the disease becoming endemic, and if
, the disease will disappear from the population. Epidemic models for the transmission of ETIDs have been developed in previous studies [see e.g. Citation5,Citation6,Citation12,Citation18,Citation24,Citation26,Citation43,Citation56,Citation57,Citation62,Citation63,Citation71, and references therein] with particular emphasis on E. coli O157:H7 infection [Citation24], salmonellosis [Citation18,Citation26,Citation43,Citation71], and cholera [Citation56,Citation57,Citation62]. In these studies, the basic reproduction number was used to predict disease persistence or extinction. However,
is an expected value and the predictions based on
may not be valid for populations with a small initial number of infectious hosts or a low initial level of environmental contamination [Citation2,Citation3,Citation33,Citation48]. For instance, if one infectious host is introduced into a completely susceptible population, that host may die or recover before transmitting the infection to a susceptible host. Similarly, if a small number of free-living pathogens are introduced into the environment with susceptible hosts present, those pathogens may die or be removed from the environment through decontamination prior to an indirect transmission [Citation2,Citation3,Citation33,Citation48]. Therefore, in order to determine the probability of an outbreak or disease extinction for these infections, it is necessary to consider a stochastic model. For more information related to stochastic epidemic modelling and stochastic fade-out of epidemics, see [Citation1–3,Citation7,Citation27,Citation33,Citation41,Citation48].
The stochastic counterpart of an ordinary differential equation (ODE) model, is a continuous-time Markov chain (CTMC) model, where time is continuous and the random variables are discrete. In stochastic epidemic theory, there are also thresholds for a major outbreak or disease extinction [Citation2,Citation3]. These stochastic thresholds are similar to the basic reproduction number, but depend on the initial number of infectious hosts and the initial level of environmental contamination. The theory of multitype branching processes is used to determine the stochastic threshold [Citation2,Citation3]. Whittle [Citation67] showed that for the Susceptible–Infectious–Recovered model with an exponentially distributed infectious period, the probability of disease extinction can be determined by the basic reproduction number. If , then the probability of disease extinction is approximately
, where i0=I(0) is the initial number of infectious hosts [Citation2,Citation67]. In the literature (e.g. [Citation2,Citation3,Citation33]), any event other than disease extinction is loosely referred to as a major outbreak. Thus, the probability of a major outbreak is approximately
Several stochastic and semi-stochastic epidemic models have been developed for the transmission of ETIDs [Citation8,Citation34,Citation60,Citation72]. However, none of these models account for replication of free-living pathogens within the environment. For pathogens which are able to replicate and persist in the environment, ignoring indirect transmission and free-living pathogen replication in the environment may not adequately reflect the most influential pathway in the transmission process [Citation24]. In addition, the models in [Citation8,Citation34] do not consider the probability of a major outbreak or host demography. Soumpasis and Butler [Citation60] approximate the probability of a major outbreak using many numerical simulations, but their model ignores indirect transmission through the environment and host demography. The model of Xiao et al. [Citation72] includes host demography and indirect transmission, and the probability of a major outbreak is computed using many numerical simulations. However, such numerical simulations can be replaced by more convenient analytical techniques which are computationally efficient and far less time-consuming.
In a previous work, an ODE model was developed by Bani-Yaghoub et al. [Citation6] for ETIDs which includes host demography, direct and indirect transmission, replication of free-living pathogens, and environmental decontamination. However, the model developed by Bani-Yaghoub did not account for stochasticity in the transmission dynamics which is critical for determining the probability of a major outbreak. Therefore, based on the assumptions of the deterministic model in [Citation6], we develop a CTMC model which accounts for the random variations that occur in the transmission process. The objectives of our investigation are to: (1) explore the effects of indirect transmission, pathogen shedding by infectious hosts, free-living pathogen replication, and environmental decontamination on the probability of disease extinction or a major outbreak, and (2) demonstrate an analytically tractable method for approximating the probability of disease extinction or a major outbreak initiated by a small number of infectious individuals or a low level of environmental contamination.
In the next section, the epidemic model of Bani-Yaghoub et al. is introduced and we show that the basic reproduction number determines the dynamics with respect to disease extinction. In Section 3, a CTMC model is formulated based on the assumptions of the ODE model and a multitype branching process approximation of the CTMC is defined. In Section 4, estimates for the probability of disease extinction or a major outbreak are obtained from the branching process approximation when . These estimates are validated with numerical examples for salmonellosis in a dairy herd and cholera in a human population. For both examples, we investigate the impact of indirect transmission, pathogen shedding by infectious hosts, replication of free-living pathogens, and environmental decontamination on the probability of disease extinction or a major outbreak. The results and their implications for disease control are discussed in Section 5.
2. Deterministic model
2.1. Model development
Let S(t), I(t), and R(t) denote the number of susceptible, infectious, and recovered hosts at time t, respectively, with a total host population . Let P(t) denote the number of free-living pathogens in the environment at time t. Suppose that Λ>0 represents the constant recruitment of hosts, d>0 denotes the natural host mortality rate, and μ≥0 denotes the pathogen-induced mortality rate. Susceptible hosts can be infected by contacting infectious hosts (direct transmission) or by ingesting free-living pathogens from the environment (indirect transmission). Both direct and indirect transmission are modelled using mass action incidence with transmission parameters
and
, respectively. Infectious hosts shed pathogen into the environment, typically through their feces. Assume that the shedding rate α≥0 represents the average number of pathogens shed into the environment per infectious host per day. Infectious hosts can recover from infection at rate γ>0, where 1/γ is the average duration of the infectious period. Recovered hosts are temporarily immune from reinfection, but lose their immunity at rate ν>0, where 1/ν is the average duration of immunity. Free-living pathogens are capable of replicating in the environment at rate r≥0, up to some carrying capacity K>0, but also experience natural death at rate ξ>0 and may be removed from the environment through environmental decontamination at rate δ≥0. illustrates the transition dynamics of the model. The corresponding system of differential equations is
Table 1. Variables and parameters with units for the model (2)–(5).
It can easily be verified that all solutions of Equations (2)–(5) with non-negative initial conditions remain non-negative. Summing Equations (2)–(4) gives the change in the total host population
The unique disease-free equilibrium (DFE) is given by , where
. The deterministic model (2)–(5) was developed and analysed by the co-authors in a previous work [Citation6]. However, as explained in Section 1, a deterministic model does not account for stochasticity in the transmission dynamics which is critical for determining the probability of disease extinction or a major outbreak. For completeness, a brief review of the analytic results are presented in the following subsection.
2.2. Basic reproduction number
The basic reproduction number, , is used to determine the dynamics of the model (2)–(5) regarding disease persistence or extinction. An expression for
can be obtained from the next-generation matrix approach [Citation20,Citation22,Citation23]. Let
denote the vector of infectious variables. Linearizing Equations (3) and (5) about the DFE gives
Following the rationale of [Citation11,Citation26,Citation46], in our model the environment acts as a reservoir for free-living pathogens and so secondary free-living pathogens generated through replication within the environment or shed by infectious hosts are considered as new infectious entities. Thus,
The terms and
represent the average number of secondary infections caused by one infectious host during its infectious period through direct and indirect transmission, respectively. Similarly,
is the average number of secondary free-living pathogens produced through replication by one free-living pathogen during its time in the environment [Citation6].
Since for j=1, 2, 3, it follows from Equation (12) that
The basic reproduction number determines the dynamics of the deterministic model regarding disease persistence or extinction. If , then the DFE is globally asymptotically stable in Γ and if
, there exists a unique endemic equilibrium
which is locally asymptotically stable and the model is uniformly persistent [Citation6]. That is, if
the disease disappears from the population and if
there is a major outbreak and long-term disease persistence.
3. Stochastic model
The basic reproduction number for the deterministic model introduced in Section 2 can be used to determine, on average, whether the appearance of a small number of infectious hosts or free-living pathogens will result in a major outbreak or disease extinction. However, even if , it is possible that disease extinction occurs prior to a major outbreak. This is known as the stochastic fade-out of an epidemic [Citation7,Citation33,Citation41,Citation48]. In order to determine the probability of a major outbreak or disease extinction for an ETID, it is necessary to consider a stochastic model. In this section, we consider a continuous-time Markov chain (CTMC) model with a discrete number of hosts and free-living pathogens. The theory of multitype branching processes is used to estimate the probability of disease extinction or a major outbreak.
3.1. CTMC model
Let S(t), I(t), and R(t) denote discrete-valued random variables for the number of susceptible, infectious, and recovered hosts at time t, respectively, with a total host population of . Let P(t) denote a discrete-valued random variable for the number of free-living pathogens in the environment at time t and let
Table 2. State transitions and rates for the CTMC model.
It follows from the Markov assumption that the time between events is exponentially distributed with parameter
The theory of multitype branching processes is used to approximate the nonlinear CTMC near the DFE and derive an estimate for the probability of disease extinction or a major outbreak. A multitype branching process assumes that the transition rates are linear with respect to the infectious variables I and P. For example, in the transition rates for direct and indirect transmission near the DFE are and
, respectively. Moreover, the transition rate for the replication of free-living pathogens near the DFE is rP.
3.2. Branching process approximation
A multitype branching process can be used to approximate the dynamics of the nonlinear CTMC near the DFE [Citation21,Citation29,Citation52]. Since infectious hosts and free-living pathogens are the only sources of infection, the branching process is applied only to these infectious groups and the susceptible hosts are assumed to be at the disease-free state, .
Infectious hosts can produce secondary infectious hosts (direct transmission) or free-living pathogens (shedding). Similarly, free-living pathogens are capable of producing infectious hosts (indirect transmission) or additional free-living pathogens (replication). The term ‘offspring’ will be used to describe susceptible hosts that become infectious due to direct or indirect transmission as well as free-living pathogens which are shed into the environment by infectious hosts or secondary pathogens which occur through replication within the environment. Assume that the number of offspring produced by an infectious host or a free-living pathogen does not depend on the number of offspring produced by other infectious hosts or free-living pathogens. Moreover, assume that the initial host population is sufficiently large so that . Since the multitype branching process is linear near the DFE, time-homogeneous, and the number of offspring produced by infectious hosts and free-living pathogens is independent, offspring probability generating functions (pgfs) can be defined for the ‘birth’ and ‘death’ of infectious hosts and free-living pathogens. These offspring pgfs can then be used to calculate the probability of disease extinction or a major outbreak [Citation2,Citation4,Citation21,Citation29,Citation40,Citation52].
Additional random variables are defined for the number of infectious hosts or free-living pathogens produced by one infectious host or free-living pathogen. Let Yii and Ypi denote the offspring random variables for infectious hosts. That is, Yii and Ypi denote the number of infectious hosts and free-living pathogens produced by one infectious host, respectively. Similarly, let Yip and Ypp denote the offspring random variables for a free-living pathogen. That is, Yip and Ypp denote the number of infectious hosts and free-living pathogens produced by one free-living pathogen, respectively. The offspring pgf for I defines the probabilities associated with the ‘birth’ of secondary infectious hosts or free-living pathogens, or the ‘death’ of the initial infectious host, given that the process started with only one infectious host, I(0)=1 and P(0)=0. The offspring pgf for I is
The offspring pgf for I is
The offspring pgf for P is
The offspring pgfs always have one fixed point in [0, 1]2, the fixed point (1, 1). If the offspring pgfs are non-singular, then there exists a unique second fixed point in (0, 1)2 [Citation4,Citation21,Citation29,Citation52]. A function fj is called non-singular if it is not a linear function of x1 and x2 such that fj(0, 0)=0. That is, [Citation4,Citation29,Citation52].
The expectation matrix of the offspring pgfs is given by
Since the offspring pgfs for I and P are non-singular and the expectation matrix M is irreducible, there are at most two fixed points of the offspring pgfs in [0, 1]2 [Citation52]. The spectral radius of the expectation matrix determines the existence of a fixed point in (0, 1)2. If the process is subcritical or critical (ρ(M)<1 or ρ(M)=1), then (1, 1) is the only stable fixed point, and if the process is supercritical (ρ(M)>1), then there exists a unique second fixed point [Citation4,Citation21,Citation29,Citation52]. This fixed point is used to determine the probability of disease extinction [Citation4,Citation21,Citation29,Citation52]. That is,
Note that ρ(M) is a threshold for persistence or extinction of the disease in the stochastic model. In particular, if , the disease is eliminated from the host population with probability one and if ρ(M)>1, there is a non-zero probability of a major outbreak. In this way, the value of ρ(M) is similar to the deterministic threshold,
. Recently an equivalence between the deterministic and stochastic thresholds for disease extinction (
) was established by Allen and van den Driessche for more general epidemic models [Citation3].
In general, a simple analytical expression for the extinction probabilities q1 and q2 cannot be obtained. However, there are special cases for which an analytical expression is possible. If there is no direct transmission or replication of the free-living pathogen (i.e. β1=r=0), the extinction probabilities q1 and q2 are given by
In Section 4, we compute the extinction probabilities q1 and q2 numerically and show that the estimate for disease extinction agrees closely with simulations of the CTMC model. A more thorough discussion of multitype branching processes can be found in the literature [Citation4,Citation21,Citation28,Citation29,Citation37,Citation40,Citation42,Citation50].
4. Results
The dynamics of the ODE and CTMC epidemic models are illustrated for salmonellosis in a herd of dairy cattle and cholera in a human population. For each of these examples, we apply the theory of multitype branching processes to estimate the probability of disease extinction or a major outbreak and illustrate the effects of indirect transmission, pathogen shedding by infectious hosts, free-living pathogen replication, and environmental decontamination on the probability of disease extinction or a major outbreak.
4.1. Salmonellosis in dairy cattle
Salmonellosis is the most common bacterial foodborne illness in the USA [Citation15]. It is estimated that in the USA Salmonella causes 1.03 million cases of foodborne disease and 400 deaths annually [Citation54]. There have been numerous research efforts targeted at reducing the number of foodborne Salmonella infections among humans. However, the incidence of foodborne salmonellosis has not changed significantly in the past 15 years [Citation16]. Dairy cattle are one of the major hosts for Salmonella, and control of salmonellosis in dairy herds can lower the risk of contamination of dairy products, thereby reducing the number of cases of human salmonellosis.
lists the model parameters related to Salmonella infection in dairy cattle. The values have been scaled so that the unit of time is one day. We assume that Λ=0.1 so that the initial herd size is approximately , which is the desired herd size indicated by Xiao et al. [Citation71,Citation72]. The rate of environmental decontamination will vary across farms depending on the feces removal system in place. The value of δ=1.2 in corresponds to removal of free-living Salmonella from the environment through daily environmental decontamination with a 70% efficacy (see Appendix 1). Due to a lack of data, the true value of the environmental carrying capacity, K, is unknown. We estimate the carrying capacity to be
(see Appendix 2). Although this value is an estimate, the carrying capacity does not affect the values of
or ℙ0. In [Citation71,Citation72], the direct and indirect transmission rates were assumed to be
and
. For these values,
and
which implies that disease transmission occurs primarily through host-to-host contact. However, it has recently been accepted that transmission of pathogens spreading through the fecal–oral route in their animal host populations occurs primarily indirectly through the contaminated environment and that the direct host-to-host transmission is negligible [Citation11,Citation25,Citation26]. Thus, we assume a smaller value (log10 decrease) of β1 and larger value (log10 increase) of β2 compared with [Citation71,Citation72]. The effects of varying the model parameters on the probability of disease extinction or a major outbreak are explored in Section 4.1.1 and Appendix 3.
Table 3. Model parameters related to salmonellosis in a dairy herd.
For the values in , the reproduction numbers are ,
,
, and
. The solution of the ODE model approaches the endemic equilibrium
with persistence of the infection. Prior to stabilizing at the endemic equilibrium, there is a major outbreak which exceeds the endemic values. For the CTMC model, there are two outcomes: either disease extinction occurs with only a few infectious cases or there is a major outbreak. The ODE solution and one sample path of the CTMC illustrating a major outbreak are plotted in .
Figure 2. Comparison of the ODE solution (dashed) and one sample path of the CTMC (solid) for salmonellosis in a dairy herd illustrating a major outbreak and disease persistence. Parameter values are as in with initial conditions S(0)=99, I(0)=1, R(0)=0, and P(0)=0. The basic reproduction number is ℛ0≈2.64. The probability of a major outbreak is 1−ℙ0=0.8453 (see ). The locally stable endemic equilibrium for the ODE model is (S*, I*, R*, P*)≈(15, 7.7, 69.6, 3.6×108).
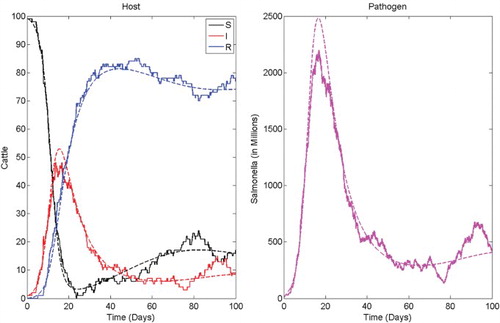
Table 4. Probability of disease extinction, ℙ0, for salmonellosis in a dairy herd calculated from the fixed point of the offspring pgfs and an approximation (Approx.) based on the proportion of 10,000 sample paths that hit zero before reaching endemic levels in numerical simulations of the CTMC model.
4.1.1. Probability of disease extinction or a major outbreak
The probability of disease extinction, ℙ0, is calculated from the branching process approximation and compared to the probability of disease extinction for the CTMC model, obtained from the proportion of 10,000 sample paths for which the sum I(t)+P(t) hits zero before reaching the endemic levels I(t)>8 or . shows that the value of ℙ0 is a good estimate of the probability of disease extinction for the CTMC model. The initial conditions
and
correspond to the average amount of Salmonella shed into the environment each day by one or two infectious cattle, respectively.
In order to determine the sensitivity of these results to variations in the model parameters, we allow the parameter values to fluctuate by of their baseline values in . Then, the strength of sensitivity to fluctuations is illustrated by the slope of the line representing a relationship between the tested model parameter fluctuations and the corresponding probabilities of disease extinction shown in . Notice that the probability of disease extinction is highly sensitive to changes in the indirect transmission rate. Unfortunately, there is a lack of literature regarding the true value of β2 for salmonellosis among dairy cattle. Thus, determining an accurate estimate for the indirect transmission rate could improve our ability to predict major outbreaks of salmonellosis in dairy herds. The probability of disease extinction is also highly sensitive to changes in the rate of environmental decontamination. This rate will vary between farms depending on the feces removal system in place. The efficacy of pathogen removal (in terms of the proportion of pathogens removed from the environment) can be used to determine the decontamination rate (see Appendix 1). For the parameter values tested in (i.e.
of the baseline, baseline, and +50% of the baseline) and the corresponding results in (cases (d), (e), and (f)), the decontamination rates correspond to daily cleaning of cattle pens with a pathogen removal efficacy of 45%, 70%, and 83%, respectively.
Figure 3. Probability of disease extinction, ℙ0, for salmonellosis in a dairy herd calculated from the fixed point of the offspring pgfs as the model parameters fluctuate by±50% of their baseline values in . The five curves indicated in the legend correspond to the five initial conditions considered in . Initial values P(0)=5×107 and P(0)=108 correspond to the average amount of Salmonella shed into the environment each day by one or two infectious cattle, respectively. Explicit values of ℙ0 can be found in Tables A1–A4 in Appendix 3.
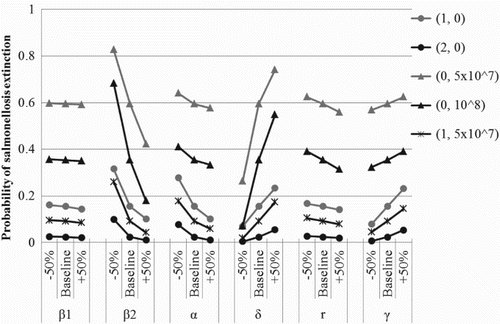
The results in indicate a moderate influence of the pathogen shedding rate on the probability of disease extinction. However, when interpreting this result one needs to be aware that the Salmonella shedding rate in infected cattle is very high (5×107 pathogens/animal/day) and that a meaningful change in the shedding rate from the epidemiology and control points of view would be on the log10 scale (e.g., reduction by 2–3 log10). Because a 50% change was applied to the non-log transformed baseline parameter value for α, the considered fluctuations of and
meant that we tested the values of
and
, respectively which on the log10 scale represent a mere change of approximately
. Therefore, since small tested changes in the value of the shedding rate had moderate effects on the probability of extinction, we expect that a change in α on the epidemiologically more meaningful log10 scale would have a strong effect on the probability of extinction. shows the effect of varying the pathogen shedding rate by ±log10 from its baseline value in . As expected, the pathogen shedding rate has a large impact on the probability of disease extinction.
Although the estimates for the probability of disease extinction are less sensitive to changes in the free-living pathogen replication rate and the rate of recovery, obtaining accurate estimates for these values could improve the ability to predict major outbreaks of salmonellosis in dairy herds and reduce the number of cases of human salmonellosis. Variations in the direct transmission rate have little impact on the probability of disease extinction which reflects the current understanding that indirect transmission through the environment is the primary pathway for transmission of salmonellosis among dairy cattle and supports the findings of Gautam et al. for salmonellosis in pigs [Citation26].
The results in are also relevant for disease control. In particular, the results in and show that for the parameter values in there is a substantially lower probability of disease extinction if infectious cattle are placed in a susceptible herd compared to the appearance of free-living Salmonella in the environment. This difference highlights the fact that a small amount of free-living Salmonella is likely to be removed from the environment through decontamination or natural decay prior to resulting in secondary infections through indirect transmission, whereas a single infectious cow can transmit the infection directly as well as shed large amounts of Salmonella during the average 10-day infectious period. Moreover, control efforts targeting pathogen shedding and indirect transmission are the most effective, followed by efforts focused on environmental decontamination (compare case (d), case (f), and case (a)). Cleaning cattle fecal waste from the barn surface is a common method of environmental decontamination on dairy farms which can effectively reduce the number of free-living Salmonella and reduce the risk of indirect transmission of infection. Likewise, cattle could be kept in tie stalls, which would reduce the rate of direct and indirect transmission.
Vaccines have been developed to prevent transmission of salmonellosis in cattle [Citation59,Citation70]. Previous studies have indicated that these vaccines have different effects on cattle [Citation32,Citation35,Citation36,Citation53,Citation61,Citation66]. Specifically, some vaccines were shown to decrease host susceptibility [Citation53], while others decreased the shedding level [Citation36] or the duration of infection [Citation53]. An available report indicates that salmonellosis vaccination is used on more than a quarter of Irish dairy farms [Citation49]. We are unaware of published reports for other countries, but it is reasonable to assume that a similar vaccination coverage is present in all countries with intensive dairy production. Thus, we consider the effects of two vaccination strategies on the probability of disease extinction: vaccines which reduce the shedding level of infectious cattle and vaccines that shorten the duration of infectiousness. The results in show that if cattle are vaccinated at the time they are placed in the herd, then the probability of disease extinction increases. Based on the model parameters in , vaccines which reduce the shedding level of infectious cattle are more effective than vaccines which reduce the duration of infectiousness (compare case (a), case (f), and case (a)).
Direct transmission and replication of free-living Salmonella in cattle pens do not significantly affect the probability of a major outbreak or disease extinction (see and Tables A1 and A3). Letting , the basic reproduction number approaches a limiting value:
For the parameter values in , the limiting value is
which implies that Salmonella infection in dairy cattle cannot be controlled through direct transmission alone. Similarly, if we let
, the basic reproduction number approaches a limiting value:
For the parameter values in , the limiting value is
. This result implies that salmonellosis cannot be controlled solely by inhibiting the replication of free-living Salmonella in cattle pens. In the case that the barn environment is not suitable for the replication of free-living Salmonella (r=0) and the force of infection due to direct transmission is negligible (
), the probability of disease extinction or a major outbreak can be estimated using the explicit expression in Equation (28).
The stochastic results indicate that in order to prevent a major outbreak it is crucial to screen incoming cattle for infection prior to placement in a susceptible herd. Unfortunately, screening cattle for infection can be difficult since they do not typically show clinical signs of infection. The screening process is further complicated by intermittent shedding patterns in which infectious cattle cycle between periods of shedding and non-shedding. If fecal samples are collected during a period of non-shedding, then one or more infectious cattle could be allowed into the herd. Improved methods for the diagnosis of infectious cattle would be valuable in the prevention of outbreaks. Removal of free-living Salmonella from the environment by cleaning cattle pens is an effective outbreak prevention measure. In addition, tie stall management can reduce the risk of direct and indirect transmission and significantly decrease the probability of a major outbreak. Moreover, vaccination of cattle at the time they are placed in the herd can decrease the probability of a major outbreak and vaccines targeting the shedding level of infectious cattle are more promising than vaccines which reduce the duration of host infectiousness. Finally, for the considered parameter values, direct transmission of infection and the replication of free-living Salmonella in cattle pens do not significantly affect the probability of a major outbreak provided that there is a sufficient level of environmental decontamination.
4.2. Cholera
Cholera is an intestinal infection caused by ingestion of food or water contaminated with the pathogen Vibrio cholerae [Citation68]. In most cases, infection causes mild diarrhea but some cases result in severe diarrhea and vomiting which can lead to severe dehydration and death within a few hours if left untreated [Citation30]. In 2008 and 2009, there was an outbreak of cholera in Zimbabwe with 98,585 reported cases and 4287 deaths [Citation51]. Since 2010, there has been a major outbreak of cholera in Haiti with 684,085 reported cases and 8361 deaths [Citation17]. Most recently, Sierra Leone has experienced an outbreak of cholera with more than 19,000 reported cases and 274 deaths [Citation69]. The World Health Organization estimates that there are 3–5 million cases each year with 100,000–120,000 deaths spread over 40–50 countries [Citation30,Citation68].
lists the model parameters related to cholera in a human population. The values have been scaled so that the unit of time is one day. We assume that Λ=9.1 so the total host population size is approximately 100, 000 which was the population size considered in [Citation63]. The duration of immunity for cholera infection is controversial. However, Kabir states that the duration of immunity is variable and can be up to 3 years [Citation39]. Thus, we use . Although the value of ν is uncertain, it does not affect the values of
or ℙ0. It is possible to treat water wells and latrines by using chemicals such as chlorine or bleach [Citation13]. Although these sanitation methods are highly effective in the removal of free-living V. cholerae from the environment, it is unlikely that widespread sanitation occurs regularly in developing countries where cholera is most prevalent [Citation13]. The decontamination rate will vary between regions and depends on the method of water sanitation and waste treatment being used. Thus, it is difficult to estimate the rate of removal of pathogens from environmental water sources. According to the data in [Citation13], 37% of households in Guinea-Bissau used bleach to disinfect latrines. Thus, we assume removal of free-living V. cholerae from the environment through daily environmental decontamination with a 37% application prevalence in the population (interpreted as efficacy at the population level). Using the formula developed in [Citation5], we estimate the decontamination rate to be δ=0.46 which corresponds to a 37% pathogen removal efficacy (see Appendix 1). Although the decontamination rate is an assumption, the effects of varying the model parameters on the probability of disease extinction or a major outbreak are considered in Section 4.2.1 and Appendix 4.
Table 5. Model parameters related to cholera in humans.
For the values in , the reproduction numbers are ,
,
, and
. The solution of the ODE model exhibits dampened oscillations (recurring outbreaks) before stabilizing at the endemic equilibrium
with persistence of the disease. For the CTMC model, either disease extinction occurs with only a few infectious cases or there is a major outbreak. The solution of the ODE and one sample path of the CTMC model are plotted in . Note that the solution of the deterministic model exhibits recurring outbreaks while the sample path illustrates a single outbreak followed by disease extinction. This difference is due to the fact that the variables for the ODE model are continuous while the random variables for the CTMC model are discrete-valued. The values of I and P in the deterministic model may take on values less than one and produce secondary outbreaks while disease extinction has occured for the stochastic model. This is often referred to as the stochastic fade-out of an epidemic [Citation7,Citation33,Citation41,Citation48].
Figure 4. Comparison of the ODE solution (dashed) and one sample path of the CTMC model (solid) for cholera illustrating recurring outbreaks and disease persistence. Parameter values are as in with initial conditions S(0)=99, 999, I(0)=1, R(0)=0, and P(0)=0. The probability of an outbreak is 1−ℙ0=0.4804 (see ). The locally stable endemic equilibrium is (S*, I*, R*, P*)≈(5.04×104, 75.06, 2.47×104, 3255.3).
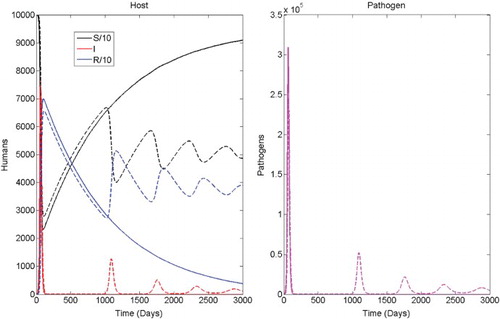
Table 6. Probability of disease extinction, ℙ0, for cholera calculated from the fixed point of the offspring pgfs and an approximation (Approx.) based on the proportion of 10,000 sample paths that hit zero before reaching endemic levels in numerical simulations of the CTMC model.
4.2.1. Probability of disease extinction or a major outbreak
The probability of disease extinction, ℙ0, is calculated from the branching process approximation and compared to the estimate obtained from the proportion of 10,000 sample paths of the CTMC model for which the sum I(t)+P(t) hits zero before reaching endemic levels I(t)>75 or P(t)>3255. The value of ℙ0 is a good estimate of the probability of disease extinction for the CTMC model (see ). The initial conditions and
correspond to the average concentration of V. cholerae shed into the environment each day by one or two infectious individuals, respectively.
To determine the sensitivity of the model to variations in the model parameters, we allow the parameter values to fluctuate by of their baseline values in . The effects on the probability of disease extinction can be seen in . Note that the probability of disease extinction is highly sensitive to changes in the model parameters. The most influential parameter is the environmental decontamination rate. This rate is variable from region to region depending on the methods and frequency of water and/or sewage treatment. The decontamination rates used in and cases (d), (e), and (f) correspond to daily treatment of the aquatic reservoir with a pathogen removal efficacy of 21%, 37%, and 50%, respectively. Although the results are most sensitive to changes in the decontamination rate, reducing the indirect transmission rate results in a slightly larger probability of disease extinction (compare case (a) and case (f)). Variations in the direct transmission rate have the least impact on the estimated probability of disease extinction which reflects the understanding that the primary route of cholera transmission is through the environment. For varying parameter values, there are small changes in the value of the basic reproduction number,
. However, there are substantial changes in the probability of disease extinction or a major outbreak. For instance, if the indirect transmission rate is reduced by 50%, the basic reproduction number changes from
to
. However, the probability of disease extinction increases from
to
for I(0)=1 and P(0)=0. The stochastic results provide valuable information about the likelihood of a major outbreak that cannot be obtained from the deterministic model.
Figure 5. Probability of disease extinction, ℙ0, for cholera in a human population calculated from the fixed point of the offspring pgfs as the model parameters fluctuate by±50% of their baseline values in . The five curves indicated in the legend correspond to the five initial conditions considered in . Initial conditions P(0)=10 and P(0)=20 correspond to the average concentration of V. cholerae shed into the environment each day by one or two infectious individuals, respectively. Explicit values of ℙ0 can be found in Tables A5–A7 in Appendix 4.
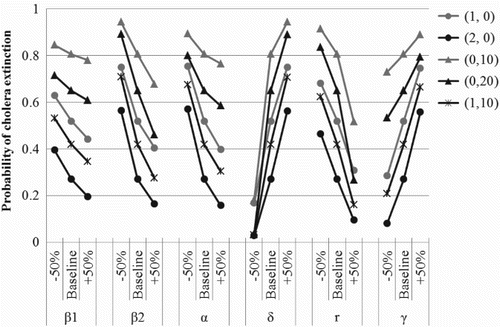
By providing a sufficient amount of clean drinking water, the risk of indirect transmission decreases and there is a substantial increase in the probability of disease extinction (see case (d)). Similarly, proper disposal of human fecal waste (e.g. use of latrines) results in a large increase in the probability of disease extinction (see case (a)). In addition to these control efforts, it is possible to treat water wells and latrines with chemicals to increase the death rate of free-living V. cholerae in the environment [Citation13]. If the decontamination rate is increased, the probability of disease extinction increases significantly (see case (f)). Unfortunately, in developing countries it is unlikely that widespread environmental decontamination occurs continually.
Previous epidemic models for cholera have not considered the replication of free-living V. cholerae in the environment. However, the replication rate of free-living pathogen has a substantial effect on the probability of disease extinction (see and cases (a) and (c)) as well as the number of infectious hosts at equilibrium. As the replication rate of V. cholerae increases from r=0.3 to r=0.45, the number of infectious hosts at the endemic equilibrium increases from to 115.7 whereas if the replication rate is decreased to r=0.15, the endemic level decreases to
. These results suggest that the replication rate of free-living V. cholerae should be considered in cholera models, especially when modelling outbreaks in developing countries where decontamination of the environment is not common and environmental conditions support replication of the free-living pathogen. If we let
, then the basic reproduction number approaches a limiting value:
For this example, the limiting value is
. This implies that cholera cannot be controlled by solely targeting the replication of free-living V. cholerae in the environment. However, this does not imply that control efforts focused on environmental decontamination are ineffective. In fact, the probability of a major outbreak is most significantly affected by fluctuations in the environmental decontamination rate (see ).
Other possible control mechanisms are isolation of infectious hosts and rapid treatment of infectious individuals to reduce the duration of infectiousness. Decreasing the duration of infectiousness results in a large increase in the probability of disease extinction. On the other hand, control efforts targeting direct transmission are not as effective since direct transmission of cholera is rare. Letting , the basic reproduction number approaches a limiting value:
For the parameter values in , the limiting value is
. Thus, it is not possible to eliminate cholera from a population by focusing on direct transmission alone. It is interesting to note that the basic reproduction number is
for β1=0, and
for r=0. This implies that, for the considered parameter values, targeting direct transmission is more effective than targeting the pathogen replication rate. However, the stochastic results illustrate that decreasing the pathogen replication rate has a larger impact on the probability of disease extinction than decreasing the direct transmission rate. Since direct transmission of cholera is not common, some cholera models in the literature ignore direct transmission altogether [Citation31,Citation57]. These models also neglect the replication rate of free-living V. cholerae. For environments which do not support replication of free-living pathogens, the probability of cholera extinction can be estimated using Equation (28). Although the pathogen replication rate was neglected in previous studies on cholera, our results indicate that the replication rate significantly affects the probability of a major outbreak as well as the number of infectious hosts at the equilibrium. Thus, future models for cholera should consider the replication rate of V. cholerae in the aquatic reservoir.
The stochastic results confirm that the control mechanisms recommended by the World Health Organization are effective in preventing cholera outbreaks. Providing clean drinking water and proper disposal of fecal waste result in the largest increases in the probability of disease extinction (see case (d) and case (a)). Increased sanitation of water wells and latrines to increase the death rate of free-living V. cholerae could be an effective method of outbreak prevention (see case (c)). Rapid treatment of infectious hosts to reduce the duration of infectiousness can significantly increase the probability of disease extinction. Control efforts targeting direct transmission or the replication rate of free-living pathogens cannot eliminate cholera unless they are paired with additional control efforts. For the parameter values in , the value of is larger than the values of
and
. This suggests that direct transmission plays a larger role than indirect transmission, shedding by infectious hosts, or pathogen replication. However, our results indicate that control strategies focused on indirect transmission and decreasing the free-living pathogen load are much more effective than strategies focused on direct transmission (see ).
5. Discussion
Indirect transmission, pathogen shedding by infectious hosts, replication of free-living pathogens in the environment, and environmental decontamination impact the emergence and spread of ETIDs. We investigated these factors by developing a nonlinear CTMC model based on the assumptions of the deterministic model introduced by Bani-Yaghoub et al. [Citation6]. A multitype branching process was used to approximate the nonlinear CTMC near the disease-free equilibrium and the theory of multitype branching processes was used to estimate the probability of disease extinction or a major outbreak. For the deterministic model (2)–(5), the basic reproduction number determines the dynamics with respect to disease persistence or extinction. In particular, if , the disease-free equilibrium is globally asymptotically stable and if
, the endemic equilibrium is locally asymptotically stable [Citation6]. Using the CTMC model and corresponding branching process approximation, we estimated the probability of disease extinction or a major outbreak for two ETIDs: salmonellosis in a herd of dairy cattle and cholera in a human population. Our results confirm the importance of indirect transmission, pathogen shedding, replication of free-living pathogens, and environmental decontamination to disease transmission and illustrate that there are differences between the deterministic and stochastic models which have major implications for disease control and outbreak prediction.
For salmonellosis in a herd of dairy cattle, our results suggest that screening cattle for infection is the most effective way to prevent a major outbreak. If infectious cattle are placed in a susceptible herd, it is very likely an outbreak will occur (see and ). Unfortunately, cattle often do not show clinical signs of Salmonella infection. The standard method of screening for infectiousness involves collecting fecal samples from cattle and testing these samples for the presence of Salmonella. This process is complicated by the intermittent shedding of pathogen by infectious cattle. If fecal samples are collected from an infected host during a period of non-shedding or shedding below the detection limit, then infected hosts could be allowed into a susceptible herd. Improved methods of screening cattle for Salmonella infection would be valuable for public health, food safety, and farm management. Frequent and thorough decontamination of the barn surface was shown to significantly decrease the probability of a major outbreak. If cattle are kept in tie stalls, the risk of direct and indirect transmission is decreased which results in large decreases in the probability of a major outbreak. Decontamination efforts and tie stall management can be paired with additional control efforts, such as vaccination of cattle, to decrease the probability of a major outbreak. Moreover, our results suggest that vaccines which reduce the shedding level of infectious cattle are more promising than vaccines which reduce the duration of host infectiousness.
For cholera in a human population, our stochastic results support the control efforts recommended by the World Health Organization. Providing clean drinking water to the population and ensuring hygenic disposal of human fecal waste can greatly decrease the probability of a major cholera outbreak. Increased sanitation of water wells and latrines to increase the death rate of free-living pathogens could also be an effective method of outbreak prevention. Unfortunately, regular treatment of water sources is not widespread in developing countries [Citation13]. Although most of the previous models for cholera do not consider the replication of free-living V. cholerae, our results indicate that the pathogen replication rate can significantly impact the probability of a cholera outbreak. Rapid treatment of infectious individuals to reduce their duration of infectiousness significantly decreases the probability of an outbreak. The results indicate that control strategies targeting direct transmission of cholera can decrease the probability of an outbreak, but control efforts targeting indirect transmission are much more effective.
In the case that there is no direct transmission of infection (β1=0) and no replication of free-living pathogens (r=0), it is possible to obtain a simple analytical expression for the probability of disease extinction or a major outbreak in terms of the model parameters (see Equation (28)). For some ETIDs, such as salmonellosis among pigs and cholera in humans, it is believed that direct transmission of infection is negligible. Moreover, in some regions the environmental conditions may not support replication of free-living pathogens. In such a situation, the expression obtained in Equation (28) provides an estimate for the probability of disease extinction.
There are some limitations of the deterministic and stochastic models presented in this manuscript. In particular, we did not account for removal of free-living pathogens from the environment due to ingestion by hosts. However, the removal of free-living pathogens from the environment through ingestion by a host is negligible when compared to the amount of pathogen shed by infectious hosts and the number of free-living pathogens removed from the environment by natural decay or environmental decontamination. Due to this fact, the majority of previous models for salmonellosis and cholera do not include ingestion of free-living pathogens [Citation18,Citation19,Citation26,Citation43,Citation56,Citation57,Citation62,Citation71]. Additionally, an assumption of our model is that infectious hosts shed pathogens into the environment continuously. However, infectious hosts typically exhibit a pattern of intermittent shedding. Once they become infectious, hosts shed pathogens into the environment at high levels and then cycle between periods of non-shedding or shedding at a lower levels. The next generation of our model will include intermittent pathogen shedding by infectious hosts. In the implementation of the CTMC model, we increase the number of free-living pathogens by one each time the shedding event occurs. However, shedding typically occurs via discrete events in which many pathogens are relased into the environment at one time. To account for this, we include the number of pathogens shed during each event in the shedding rate α. This allowed for a more straightforward comparison of the deterministic and stochastic results. Future stochastic models for ETIDs may consider implementing pathogen shedding as a jump process. Moreover, due to a lack of information regarding the true values of the model parameters, we used estimates or assumptions from previous studies on salmonellosis and cholera. Certain parameters do not affect the value of or ℙ0 (e.g. ν and K). However, obtaining accurate estimates for parameter values such as the direct and indirect transmission rates or the environmental decontamination rate for cholera could be valuable for outbreak prediction and disease control.
In this manuscript, we considered the examples of salmonellosis in dairy cattle and cholera in a human population. However, the model (2)–(5) and the techniques used to obtain an estimate for the probability of disease extinction of a major outbreak can be applied to other ETIDs such as Escherichia coli O157:H7 in a cattle herd, Salmonella infection in pigs, Avian Influenza in wild birds, Campylobacter infection in chickens, and cryptosporidiosis in cattle.
Funding
This work was supported by the National Science Foundation grant [NSF-EF-0913367] to RI.
Acknowledgements
Any opinions, findings, conclusions, or recommendations expressed in this material are those of the authors and do not necessarily reflect the views of the National Science Foundation. We would like to thank two anonymous reviewers for their suggestions which improved the paper.
References
- L.J.S. Allen, An Introduction to Stochastic Processes with Applications to Biology Second Edition, CRC Press, Boca Raton, FL, 2010.
- L.J.S. Allen and G.E. LahodnyJr., Extinction thresholds in deterministic and stochastic epidemic models, J. Biol. Dyn. 6 (2012), pp. 590–611.
- L.J.S. Allen and P. van den Driessche, Relations between deterministic and stochastic thresholds for disease extinction in continuous- and discrete-time infectious disease models, Math. Biosci. 243 (2013), pp. 99–108.
- K.B. Athreya and P.E. Ney, Branching Processes, Springer-Verlag, New York, 1972.
- M. Bani-Yaghoub, R. Gautam, D. Döpfer, C.W. Kaspar, and R. Ivanek, Effectiveness of environmental decontamination in control of infectious diseases, Epidemiol. Infect. 140 (2012), pp. 542–553.
- M. Bani-Yaghoub, R. Gautam, Z. Shuai, P. van den Driessche, and R. Ivanek, Reproduction numbers for infections with free-living pathogens growing in the environment, J. Biol. Dyn. 6 (2012), pp. 923–940.
- M.S. Bartlett, Stochastic Population Models in Ecology and Epidemiology, Methuen, London 1960.
- A.D.C. Berriman, D. Clancy, H.E. Clough, and R.M. Christley, Semi-stochastic models for Salmonella infection within finishing pig units in the UK, Math. Biosci. 245 (2013), pp. 148–156.
- D.J. Bolton, C.M. Byrne, J.J. Sheridan, D.A. McDowell, and I.S. Blair, The survival characteristics of a non-toxigenic strain of Escherichia coli O157:H7, J. Appl. Microbiol. 86 (1999), pp. 407–411.
- S.A. Boone and C.P. Gerba, Significance of fomites in the spread of respiratory and enteric viral disease, Appl. Environ. Microbiol. 73 (2007), pp. 1687–1696.
- L. Bourouiba, A. Teslya, and J. Wu, Highly pathogenic avian influenza outbreak mitigated by seasonal low pathogenic strains: Insights from dynamic modeling, J. Theor. Biol. 271 (2011), pp. 181–201.
- R. Breban, J.M. Drake, and P. Rohani, A general multi-strain model with environmental transmission: Invasion conditions for the disease-free and endemic states, J. Theor. Biol. 264 (2010), pp. 729–736.
- S. Cairncross, J. Ensink, and T. Kahawita, Evaluation of the WASH activities undertaken to prevent and control cholera outbreaks in Guinea-Conakry & Guinea-Bissau – systematic literature review (June 2009-December 2009), 2009. Available at http://www.unicef.org/evaldatabase/files/LSHTM_WASH_Evaluation_last_Guinea-Bissau.pdf (accessed 22 May 2014).
- T. Caraco and I.-N. Wang, Free-living pathogens: Life-history constraints and strain competition, J. Theor. Biol. 250 (2008), pp. 569–579.
- Centers for Disease Control and Prevention, Preliminary FoodNet data on the incidence of infection with pathogens transmitted commonly through food – 10 states, 2009, MMWR 59 (2010), pp. 418–422.
- Centers for Disease Control and Prevention, Vital signs: Incidence and trends of infection with pathogens transmitted commonly through food – foodborne diseases active surveillance network, 10 U.S. sites, 1996–2010, MMWR 60 (2011), pp. 749–755.
- Centers for Disease Control and Prevention, Cholera in Haiti, 2013. Available at http://wwwnc.cdc.gov/travel/notices/watch/haiti-cholera (accessed 22 May 2014).
- P.P. Chapagain, J.S. van Kessel, J.S. Karns, D.R. Wolfgang, E. Hovingh, K.A. Nelen, Y.H. Schukken, and Y.T. Grohn, A mathematical model of the dynamics of Salmonella Cerro infection in a US dairy herd, Epidemiol. Infect. 136 (2008), pp. 263–272.
- C.T. Codeço, Endemic and epidemic dynamics of cholera: The role of the aquatic reservoir, BMC Infect. Dis. (2001). doi:10.1186/1471-2334-1-1.
- O. Diekmann, J.A.P. Heesterbeek, and J.A.J. Metz, On the definition and the computation of the basic reproduction ratio R0 in models for infectious diseases in heterogeneous populations, J. Math. Biol. 28 (1990), pp. 365–382.
- K.S. Dorman, J.S. Sinsheimer, and K. Lange, In the garden of branching processes, SIAM Rev. 46 (2004), pp. 202–229.
- P. van den Driessche and J. Watmough, Reproduction numbers and sub-threshold endemic equilibria for compartmental models of disease transmission, Math. Biosci. 180 (2002), pp. 29–48.
- P. van den Driessche and J. Watmough, Further notes on the basic reproduction number, in Mathematical Epidemiology, F. Brauer, P. van den Driessche, and J. Wu, eds., Lecture Notes in Mathematics, Vol. 1945, Springer, Berlin, 2008, pp. 159–178.
- R. Gautam, M. Bani-Yaghoub, W.H. Neill, D. Döpfer, C. Kaspar, and R. Ivanek, Modeling the effect of seasonal variation in ambient temperature on the transmission dynamics of a pathogen with a free-living stage: Example of Escherichia coli O157:H7 in a dairy herd, Prev. Vet. Med. 102 (2011), pp. 10–21.
- R. Gautam, M. Kulow, D. Park, T. Gonzales, J. Dahm, M. Shiroda, A.J. Stasic, D. Döpfer, C. Kaspar, and R. Ivanek, Transmission of Escherichia coli O157:H7 among cattle is influenced by the level of environmental contamination, Epidemiol. Infect. (2014). doi:10.1017/S0950268814000867.
- R. Gautam, G.E. Lahodny Jr., M. Bani-Yaghoub, P.S. Morley, and R. Ivanek, Understanding the role of cleaning in the control of Salmonella Typhimurium in grower-finisher pigs: A modelling approach, Epidemiol. Infect. (2013). doi:10.1017/s0950268813001805.
- N.S. Goel and N. Richter-Dyn, Stochastic Models in Biology, Academic Press, New York, 1974.
- P. Haccou, P. Jagers, and V.A. Vatutin, Branching Processes Variation, Growth, and Extinction of Populations, Cambridge Studies in Adaptive Dynamics, Cambridge Univ. Press, Cambridge, MA, 2005.
- T.E. Harris, The Theory of Branching Processes, Springer-Verlag, Berlin, 1963.
- J.B. Harris, R.C. LaRocque, F. Qadri, E.T. Ryan, and S.B. Calderwood, Cholera, Lancet 379 (2012), pp. 2466–2476.
- D.M. Hartley, J.G. MorrisJr., and D.L. Smith, Hyperinfectivity: A critical element in the ability of V. cholerae to cause epidemics? PLoS Med. 3 (2006), pp. 63–69.
- L.C. Heider, R.W. Meiring, A.M. Hoet, W.A. Gebreys, J.A. Funk, and T.E. Wittum, Evaluation of vaccination with a commercial subunit vaccine on shedding of Salmonella enterica in subclinically infected dairy cows, J. Am. Vet. Med. Assoc. 233 (2008), pp. 466–469.
- O.A. van Herwaarden and J. Grasman, Stochastic epidemics: Major outbreaks and the duration of the endemic period, J. Math. Biol. 33 (1995), pp. 581–601.
- A.A. Hill, E.L. Snary, M.E. Arnold, L. Alban, and A.J.C. Cook, Dynamics of Salmonella transmission on a British pig grower-finisher farm: A stochastic model, Epidemiol. Infect. 136 (2008), pp. 320–333.
- J.K. House and B.P. Smith, Profitable strategies to control salmonellosis in dairy cattle, Proceedings of the WBC Congress, Quebec, Canada.
- J.K. House, M.M. Ontiveros, N.M. Blackmer, E.L. Dueger, J.B. Fitchhorn, G.R. McArthur, and B.P. Smith, Evaluation of an autogenous Salmonella bacterin and a modified live Salmonella serotype Choleraesuis vaccine on a commercial dairy farm, Am. J. Vet. Res. 62 (2001), pp. 1897–1902.
- P. Jagers, Branching Processes with Biological Applications, Wiley Series in Probability and Mathematical Statistics – Applied Probability and Statistics, Wiley-Intersci, London, 1975.
- M.A. Jensen, S.M. Faruque, J.J. Mekalanos, and B.R. Levin, Modeling the role of bacteriophage in the control of cholera outbreaks, Proc. Natl. Acad. Sci. 103 (2006), pp. 4652–4657.
- S. Kabir, Cholera vaccines: The current status and problems, Rev. Med. Microbiol. 16 (2005), pp. 101–116.
- S. Karlin and H. Taylor, A First Course in Stochastic Processes, Academic Press, New York, 1975.
- D.G. Kendall, Deterministic and stochastic epidemics in closed populations, Proc. Symp. Math. Stat. Prob. 3rd, Berkeley 4, 1956.
- M. Kimmel and D. Axelrod, Branching Processes in Biology, Springer-Verlag, New York, 2002.
- C. Lanzas, S. Briend, R. Ivanek, Y. Lo, P.P. Chapagain, K.A. Ray, P. Ayscue, L.D. Warnick, and Y.T. Gröhn, The effect of heterogeneous infectious period and contagiousness on the dynamics of Salmonella transmission in dairy cattle, Epidemiol. Infect. 136 (2008), pp. 1496–1510.
- C. Lanzas, L.D. Warnick, R. Ivanek, P. Ayscue, D.V. Nydam, and Y.T. Gröhn, The risk and control of Salmonella outbreaks in calf-raising operations: A mathematical modeling approach, Vet. Res. (2008). doi:10.1051/vetres:2008038.
- J.T. LeJeune, T.E. Besser, and D.D. Handcock, Cattle water troughs as reservoirs of Escherichia coli O157:H7, Appl. Environ. Microbiol. 67 (2001), pp. 3053–3057.
- M. Lélu, M. Langlais, M.-L. Poulle, and E. Gilot-Fromont, Transmission dynamics of Toxoplasma gondii along an urban-rural gradient, Theor. Popul. Biol. 78 (2010), pp. 139–147.
- Z. Lu, Y.T. Grohn, R.L. Smith, D.R. Wolfgang, J.A.S. van Kessel, and Y.H. Schukken, Assessing the potential impact of Salmonella vaccines in an endemically infected dairy herd, J. Theor. Biol. 259 (2009), pp. 770–784.
- D. Ludwig, Persistence of dynamical systems under random perturbations, SIAM Rev. 17 (1975), pp. 605–640.
- J. Mee, Management of infectious diseases in split-calving dairy herds, 2009. Available at http://www.teagasc.ie/publications/2009/20091105/management_of_infectious_diseases.pdf (accessed 22 May 2014).
- C.J. Mode, Multitype Branching Processes Theory and Applications, Elsevier, New York, 1971.
- Z. Mukandavire, S. Liao, J. Wang, H. Gaff, D.L. Smith, and J.G. MorrisJr., Estimating the reproductive numbers for the 2008-2009 cholera outbreaks in Zimbabwe, Proc. Natl. Acad. Sci. USA 108 (2011), pp. 8767–8772.
- S. Pénisson, Conditional limit theorems for multitype branching processes and illustration in epidemiological risk analysis, Ph.D. diss., Mathematische Statistik und Wahrscheinlichkeitstheorie, Institut für Mathematik der Unversität, Postdam, Germany, 2010.
- J.A. Robertsson, A.A. Lindberg, S. Hoiseth, and B.A. Stocker, Salmonella typhimurium infection in calves: Protection and survival of virulent challenge bacteria after immunization with live or inactivated vaccines, Infect. Immun. 41 (1983), pp. 742–750.
- E. Scallan, R.M. Hoekstra, F.J. Angulo, R.V. Tauxe, M.-A. Widdowson, S.L. Roy, J.L. Jones, and P.M. Griffin, Foodborne illness acquired in the United States – major pathogens, Emerg. Infect. Dis. 17 (2011), pp. 7–15.
- E. Scott and S.F. Bloomfield, The survival and transfer of microbial contamination via cloths, hands and utensils, J. Appl. Bacteriol. 68 (1990), pp. 271–278.
- Z. Shuai and P. van den Driessche, Global dynamics of cholera models with differential infectivity, Math. Biosci. 234 (2011), pp. 118–126.
- Z. Shuai, J.H. Tien, and P. van den Driessche, Cholera models with hyperinfectivity and temporary immunity, Bull. Math. Biol. 74 (2012), pp. 2423–2445.
- L.W. Sinton, R.R. Braithwaite, C.H. Hall, and M.L. Mackenzie, Survival of indicator and pathogenic bacteria in bovine feces on pasture, Appl. Environ. Microbiol. 73 (2007), pp. 7917–7925.
- B.P. Smith, F.G. Habasha, M. Reina-Guerra, and A.J. Hardy, Immunization of calves against salmonellosis, Am. J. Vet. Res. 41 (1980), pp. 1947–1951.
- I. Soumpasis and F. Butler, Development and application of a stochastic epidemic model for the transmission of Salmonella Typhimurium at the farm level of the pork production chain, Risk Anal. 29 (2009), pp. 1521–1533.
- G. Steinbach and H. Meyer, Efficacy of subcutaneous inoculation of calves with ‘Murivac’ inactivated salmonellosis vaccine, Tierarztliche Praxis. 22 (1994), pp. 529–531.
- J.H. Tien and D.J.D. Earn, Multiple transmission pathways and disease dynamics in a waterborne pathogen model, Bull. Math. Biol. 72 (2010), pp. 1506–1533.
- J.H. Tien, H.N. Poinar, D.N. Fisman, and D.J.D. Earn, Herald waves of cholera in nineteenth century London, J. R. Soc. Int. 8 (2011), pp. 756–760.
- M. Vital, H.P. Füchslin, F. Hammes, and T. Egli, Growth of Vibrio cholerae O1 Ogawa Eltor in freshwater, Microbiol. 153 (2007), pp. 1993–2001.
- X. Wang, R. Gautam, P.J. Pinedo, L.J.S. Allen, and R. Ivanek, A stochastic model for transmission, extinction and outbreak of Escherichia coli O157:H7 in cattle as affected by ambient temperature and cleaning practices, J. Math. Biol. (2013). doi:10.1007/s00285-013-0707-1.
- A. Weber, C. Bernt, K. Bauer, A. Mayr, The control of bovine salmonellosis under field conditions using herd-specific vaccines, Tierarztliche Praxis. 21 (1993), pp. 511–516.
- P. Whittle, The outcome of a stochastic epidemic: A note on Bailey's paper, Biometrika 42 (1955), pp. 116–122.
- World Health Organization, Cholera vaccines: WHO position paper, Wkly. Epidemiol. Rec. 85 (2010), pp. 117–128.
- World Health Organization, Cholera in Sierra Leone: The case study of an outbreak, 2012. Available at http://www.who.int/features/2012/cholera_sierra_leone/en.
- C. Wray, W.J. Sojka, J.A. Morris, and W.J.B. Morgan, The immunization of mice and calves with gal E mutants of Salmonella typhimurium, J. Hyg. 79 (1977), pp. 17–24.
- Y. Xiao, R.G. Bowers, D. Clancy, and N.P. French, Understanding the dynamics of Salmonella infections in dairy herds: A modelling approach, J. Theor. Biol. 233 (2005), pp. 159–175.
- Y. Xiao, D. Clancy, N.P. French, and R.G. Bowers, A semi-stochastic model for Salmonella infection in a multi-group herd, Math. Biosci. 200 (2006), pp. 214–233.
- H. Xu, N. Roberts, F. Singleton, R. Attwell, D. Grimes, and R. Colwell, Survival and viability of nonculturable Escherichia coli and Vibrio cholerae in the estuarine and the marine environment, Microb. Ecol. 8 (1982), pp. 313–323.
Appendix 1. Estimation of the environmental decontamination rates for salmonellosis and cholera
Using the technique described in [Citation5], the relationship between the decontamination rate δ and the efficacy of environmental decontamination represented as the proportion of free-living pathogens removed from the environment () each day is given by
For salmonellosis, the baseline value of δ=1.2 in corresponds to a pathogen removal efficacy of 70% [Citation26]. For cholera, the baseline value of δ=0.46 in corresponds to an efficacy of 37% [Citation13].
Appendix 2. Estimation of the carrying capacity of free-living Salmonella in the environment
It follows from Equation (6) that . Thus, there will be no more than 100 cattle in the herd in the long-run. According to Sinton et al. [Citation58], the carrying capacity of free-living Salmonella in cattle fecal debris could be as high as
pathogens per gram of feces. On average, cattle produce 30 kg of feces per day [Citation65]. Thus, if there are 100 cattle, the environmental carrying capacity of free-living Salmonella is approximately
pathogens. The true value of the environmental carrying capacity is unknown.
Appendix 3. Sensitivity analysis for salmonellosis
Tables A1–A3 illustrate the effects of varying the model parameters by of their baseline values in on the probability of disease extinction for salmonellosis in a herd of dairy cattle. The results in illustrate the effect of varying the pathogen shedding rate α by ±log10 of its baseline value in .
Appendix 4. Sensitivity analysis for cholera
Tables A5–A7 illustrate the effects of varying the model parameters by of their baseline values in on the probability of disease extinction for cholera in a human population.