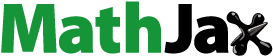
Abstract
In this paper, we propose and analyse a virus dynamics model with humoral immune response including latently infected cells. The incidence rate is given by Beddington–DeAngelis functional response. We have derived two threshold parameters, the basic infection reproduction number and the humoral immune response activation number
which completely determined the basic and global properties of the virus dynamics model. By constructing suitable Lyapunov functions and applying LaSalle's invariance principle we have proven that if
, then the infection-free equilibrium is globally asymptotically stable (GAS), if
, then the chronic-infection equilibrium without humoral immune response is GAS, and if
, then the chronic-infection equilibrium with humoral immune response is globally asymptotically stable. These results are further illustrated by numerical simulations.
1. Introduction
During the last decades, several dangerous viruses have appeared which attack the human body and some of them cause death. These prompt many researchers to study mathematical modelling and model analysis of the interaction between the host cells and viruses such as human immunodeficiency virus (HIV) (see e.g. [Citation3–7,Citation9,Citation15,Citation16,Citation18,Citation22,Citation24]), hepatitis B virus (HBV) [Citation2,Citation12], hepatitis C virus (HCV) [Citation14,Citation20,Citation21], human T cell leukemia [Citation11] and dengue virus [Citation23], etc. There are many benefits from mathematical models of viral infection including: (i) they provide important quantitative insights into viral dynamics in vivo, (ii) they can improve diagnosis and treatment strategies which raise hopes of patients infected with viruses, (iii) they can be used to estimate key parameter values that control the infection process.
The basic viral infection model which was proposed by Nowak and Bangham [Citation15] is a three-dimensional ordinary differential equations and contains three variables x, y and v representing the concentrations of the uninfected target cells, infected cells and free virus particles, respectively. To provide more accurate modelling for the viral infection, the effect of immune response has to be considered. The immune system has two main responses to viral infections, the cell mediated immunity and humoral immunity. The cell mediated immunity is based on the Cytotoxic T Lymphocyte cells which are responsible to attack and kill the infected cells. The humoral immunity is based on the antibodies that are produced by the B cells. The function of the antibodies is to attack the viruses [Citation16]. In some infections such as in malaria, the cell mediated immunity is less effective than the humoral immunity [Citation1]. In the literature, several mathematical models have been formulated to consider the humoral immune response into the viral infection models (see e.g. [Citation13,Citation17,Citation25]). The basic model of viral infection with humoral immune response has been introduced by Murase et al. [Citation13] and Wang and Zou [Citation25] as:
(1)
(1)
(2)
(2)
(3)
(3)
(4)
(4) where z denotes the concentration of the B cells. Uninfected cells are generated from sources within the body at a rate λ, die at rate dx and become infected at rate
, where β is the rate constant describing the infection process. Infected cells are produced at rate
and die at rate ay. Free virus particles are produced from infected cells at rate ky, die at rate uv and are removed from the body due to antibodies at rate rzv. B cells are activated at rate gzv and die at rate
. Parameters λ, d, β, a, k, u, r, g and μ are positive. The model may describe the dynamics of several viruses such as HIV, HBV and HCV. In case of HIV, x will represent the concentration of the uninfected CD4
T cells while in case of HBV or HCV it represents the hepatocyte cells.
Model (Equation1(1)
(1) )–(Equation4
(4)
(4) ), does not take into consideration the latently infected cells which are due to the delay between the moment of a virus contacts an uninfected target cell and the moment of producing infectious viruses. Latently infected cells have been incorporated into the basic viral infection model in [Citation18,Citation19]. The global stability of viral infection models with latently infected cells has been studied in several works (see e.g. [Citation6,Citation10]). However, in [Citation6,Citation10], the humoral immune response has been neglected.
We note that, the incidence rate of infection presented in model (Equation1(1)
(1) )–(Equation4
(4)
(4) ) is given by bilinear form. Recently, Huang et al. [Citation9] have proposed a viral infected model with Beddington–DeAngelis functional response,
, where
. This form generalizes the Holling type II functional response
by adding a term
in the denominator which models mutual interference between viruses [Citation9]. When
and
, the Beddington–DeAngelis functional response is simplified to a saturation response [Citation22]. In [Citation9], both the humoral immune response and the latently infected cells were not modelled.
The aim of this paper is to propose a virus dynamics model with humoral immune response taking into consideration both latently and actively infected cells and investigate its basic and global properties. The incidence rate is given by Beddington–DeAngelis functional response. Using Lyapunov functions, we prove that the global dynamics of the model is determined by two threshold parameters, the basic infection reproduction number and the humoral immune response activation number
.
2. The model
In this section, we propose a virus dynamics model with humoral immunity and Beddington–DeAngelis functional response, taking into consideration the latently infected cells and the actively infected cells.
(5)
(5)
(6)
(6)
(7)
(7)
(8)
(8)
(9)
(9) where γ and α are positive constants, w and y denote the concentrations of latently infected and actively infected cells, respectively. Equation (Equation6
(6)
(6) ) describes the dynamics of the latently infected cells and show that they are die at rate ew and are converted to actively infected cells at rate
. The fractions
and q with
are the probabilities that upon infection, an uninfected cell will become either latently infected or actively infected. The other variables and parameters of the model have the same meanings as given in model (Equation1
(1)
(1) )–(Equation4
(4)
(4) ).
2.1. Positive invariance
We note that model (Equation5(5)
(5) )–(Equation9
(9)
(9) ) is biologically acceptable in the sense that no population goes negative. It is straightforward to check the positive invariance of the non-negative orthant
by model (Equation5
(5)
(5) )–(Equation9
(9)
(9) ) (see e.g. [Citation19]). In the following, we show the boundedness of the solutions of model (Equation5
(5)
(5) )–(Equation9
(9)
(9) ).
Proposition 1.
There exist positive numbers such that the compact set
is positively invariant.
Proof.
Let , then
where
. Hence
, if
, where
. Since x, w and y are non-negative, then
,
,
if
. On the other hand, let
, then
where
. Hence
, if
, where
. Since
and
, then
and
if
, where
.
2.2. Equilibria and biological thresholds
In the following we give a lemma which gives the existence of positive equilibria of the model.
Lemma 1.
For system (Equation5(5)
(5) )–(Equation9
(9)
(9) ) there exist two threshold parameters
and
with
such that
if
then there exists only one positive equilibrium
if
then there exist only two positive equilibria
and
and
if
then there exist three positive equilibria
and
.
Proof.
Let the right-hand sides of Equations (Equation5(5)
(5) )–(Equation9
(9)
(9) ) equal zero, then we get that system (Equation5
(5)
(5) )–(Equation9
(9)
(9) ) can admit three equilibria:
(i) Infection-free equilibrium , where
, which represents the state where there are no viruses in the body.
(ii) Chronic-infection equilibrium without humoral immune response where
We note that,
exists when
. Let us define the threshold parameter
as:
(iii) Chronic-infection equilibrium with humoral immune response
, where
where
. Clearly
exists when
. Now we are ready to define the second threshold parameter
as:
Now we show that
,
and
. Clearly
. From the equilibrium conditions of
we have
then
Moreover,
and then,
.
Similarly, one can show that . Now we show that if
, then
and
. From the equilibrium conditions of
, we have
Then
It follows that,
. Finally, since
, then we get
2.3. Global stability
In this subsection, we prove the global stability of the three equilibria of system (Equation5(5)
(5) )–(Equation9
(9)
(9) ) employing the method of Lyapunov function. In the remaining parts of the paper we shall use the following function:
,
Theorem 1.
If then
is globally asymptotically stable
GAS
in Ω.
Proof.
Define a Lyapunov function as follows:
The time derivative of
along the trajectories of (Equation5
(5)
(5) )–(Equation9
(9)
(9) ) satisfies
(10)
(10) Thus if
then
for all
. Using [Citation8, Theorem 5.3.1], we find that the solutions of system (Equation5
(5)
(5) )–(Equation9
(9)
(9) ) converge to a set Γ, where Γ is the largest invariant subset of
. From Equation (Equation10
(10)
(10) ) we have
if and only if
,
and
. We note that, for any element belongs to Γ we have
then
. From Equation (Equation8
(8)
(8) ) we get
, and thus
. Moreover, from Equation (Equation7
(7)
(7) ) we get
. Hence,
if and only if
,
and
. The global stability of
follows from LaSalle's invariance principle.
Lemma 2.
Suppose that then
exist satisfying
Proof.
Let , then we have
(11)
(11)
(12)
(12)
(13)
(13)
(14)
(14) Suppose that,
. Using the conditions of the equilibria
and
we have
and from inequalities (Equation11
(11)
(11) ) and (Equation12
(12)
(12) ) we get:
which leads to contradiction. Thus,
. Using the equilibrium conditions for
we have
, then
From inequalities (Equation11
(11)
(11) ) and (Equation14
(14)
(14) ), we get
Theorem 2.
If then
is GAS in Ω.
Proof.
Define the following Lyapunov functional
and calculate its time derivative along the trajectories of (Equation5
(5)
(5) )–(Equation9
(9)
(9) ) we obtain
Applying
we obtain
(15)
(15) Using the equilibrium conditions for
:
we obtain
Then Equation (Equation15
(15)
(15) ) becomes
Since the geometrical mean is less than or equal to the arithmetical mean, then
From Lemma 2 we have if
then
. It follows that, if
, then
for all
. It can be seen that,
if and only if
,
,
,
and
. LaSalle's invariance principle implies the global stability of
.
Theorem 3.
If then
is GAS in
.
Proof.
We construct the following Lyapunov functional
The time derivative of
along the trajectories of (Equation5
(5)
(5) )–(Equation9
(9)
(9) )
Applying
, we get
Using the other equilibrium conditions for
:
we get
and
Thus, if
then
and
. Since the geometrical mean is less than or equal to the arithmetical mean, then
. The solutions of system (Equation5
(5)
(5) )–(Equation9
(9)
(9) ) converge to the largest invariant subset of
. It can be seen that,
if and only if
,
and
. If
, then
and from Equation (Equation8
(8)
(8) ) we have
, which gives
. Hence,
at
. LaSalle's invariance principle implies the global stability of
.
Remark 1.
The parameter is the standard basic infection reproduction number in the literature of viral infection models. It measures the average number of newly infected cells produced from any one infected cell at the infection-free equilibrium [Citation16]. Thus,
is the threshold parameter that determines whether a chronic-infection can be established without humoral immune response. If
, then the viruses will be cleared from the body. Therefore, using effective anitiviral drug therapy can control and prevent the infection by making
. In case of
, the infection become chronic. The parameter
represents the humoral immune response activation number and determines whether a persistent humoral immune response can be established. When
, the infection always becomes chronic, but no humoral response can be established. When
, the infection always becomes chronic with humoral response.
3. Numerical simulations
In this section, we perform some numerical simulations for model (Equation5(5)
(5) )–(Equation8
(8)
(8) ) to confirm our theoretical results given in Theorems 1-3. The values of the model's parameters are given as:
cells mm
day
,
day
,
virus cells
day
,
day
,
virus
mm
,
cells
mm
,
day
,
day
,
day
,
,
cells
mm
day
, and
day
. The parameters β and g will be chosen below. All computations were carried out by MATLAB. We have the following cases:
Case (I) Stability of . We choose
virus
mm
day
and
virus
mm
day
. Using these data we compute
and
. Figures show that the numerical results are consistent with Theorem 1. We can see that, the concentrations of uninfected cells is increasing and tends to it normal value
cells mm
, while the concentrations of latently infected cells, actively infected cells, free viruses and B cells are decaying and tend to zero. In this case, the virus particles will be removed from the body.
Case (II) Stability of . We take
virus
mm
day
and
virus
mm
day
. In this case,
and
. Figures – show that the numerical results are consistent with Theorem 2. We can see that, the trajectory of the system will tend to the chronic-infection equilibrium without humoral immune response
. In this case, the infection becomes chronic but without persistent humoral immune response.
Case (III) Stability of . We choose
virus
mm
day
and
virus
mm
day
. Then we compute
and
. From Figures – we can see that, our simulation results are consistent with the theoretical results of Theorem 3. We observe that, the trajectory of the system will tend to the chronic-infection equilibrium with humoral immune response
. In this case, the infection becomes chronic but with persistent humoral immune response.
4. Conclusion
In this paper, we have proposed and analysed a virus dynamics model with humoral immune response. The model describes the interaction between the uninfected target cells, latently infected cells, actively infected cells, free virus particles and B cells. The incidence rate has been given by Beddington–DeAngelis functional response. We have derived two threshold parameters, the basic infection reproduction number and the humoral immune response activation number
which completely determine the basic and global properties of the virus dynamics model. Using Lyapunov method and applying LaSalle's invariance principle we have proven that if
, then the infection-free equilibrium is GAS, if
, then the chronic-infection equilibrium without humoral immune response is GAS, and if
, then the chronic-infection equilibrium with humoral immune response is GAS. Numerical simulations have been performed for the model. We have shown that both numerical and theoretical results are consistent.
Acknowledgements
The authors acknowledge with thanks DSR technical and financial support. The authors are also grateful to professor J. M. Cushing and to the anonymous reviewers for constructive suggestions and valuable comments, which improve the quality of the article.
Disclosure statement
No potential conflict of interest was reported by the authors.
Funding
This article was funded by the Deanship of Scientific Research (DSR), King Abdulaziz University, Jeddah.
References
- J.A. Deans and S. Cohen, Immunology of malaria, Ann. Rev. Microbiol. 37 (1983), pp. 25–50. doi: 10.1146/annurev.mi.37.100183.000325
- S. Eikenberry, S. Hews, J.D. Nagy, and Y. Kuang, The dynamics of a delay model of hepatitis B virus infection with logistic hepatocyte growth, Math. Biosc. Eng. 6 (2009), pp. 283–299. doi: 10.3934/mbe.2009.6.283
- A.M. Elaiw, Global properties of a class of HIV models, Nonlinear Anal. Real World Appl. 11 (2010), pp. 2253–2263. doi: 10.1016/j.nonrwa.2009.07.001
- A.M. Elaiw, Global properties of a class of virus infection models with multitarget cells, Nonlinear Dynam. 69 (2012), pp. 423–435. doi: 10.1007/s11071-011-0275-0
- A.M. Elaiw, Global dynamics of an HIV infection model with two classes of target cells and distributed delays, Discrete Dyn. Nat. Soc. 2012. Article ID 253703.
- A.M. Elaiw and S.A. Azoz, Global properties of a class of HIV infection models with Beddington–DeAngelis functional response, Math. Method Appl. Sci. 36 (2013), pp. 383–394. doi: 10.1002/mma.2596
- A.M. Elaiw, I.A. Hassanien, and S.A. Azoz, Global stability of HIV infection models with intracellular delays, J. Korean Math. Soc. 49 (2012), pp. 779–794. doi: 10.4134/JKMS.2012.49.4.779
- J.K. Hale and S.V. Lunel, Introduction to Functional Differential Equations, Springer-Verlag, New York, 1993.
- G. Huang, W. Ma, and Y. Takeuchi, Global properties for virus dynamics model with Beddington–DeAngelis functional response, Appl. Math. Lett. 22 (2009), pp. 1690–1693. doi: 10.1016/j.aml.2009.06.004
- A. Korobeinikov, Global properties of basic virus dynamics models, Bull. Math. Biol. 66 (2004), pp. 879–883. doi: 10.1016/j.bulm.2004.02.001
- M.Y. Li and H. Shu, Global dynamics of a mathematical model for HTLV-I infection of CD4+ T cells with delayed CTL response, Nonlinear Anal. Real World Appl. 13 (2012), pp. 1080–1092. doi: 10.1016/j.nonrwa.2011.02.026
- J. Li, K. Wang, and Y. Yang, Dynamical behaviors of an HBV infection model with logistic hepatocyte growth, Math. Comput. Modelling 54 (2011), pp. 704–711. doi: 10.1016/j.mcm.2011.03.013
- A. Murase, T. Sasaki, and T. Kajiwara, Stability analysis of pathogen-immune interaction dynamics, J. Math. Biol. 51 (2005), pp. 247–267. doi: 10.1007/s00285-005-0321-y
- A.U. Neumann, N.P. Lam, H. Dahari, D.R. Gretch, T.E. Wiley, T.J Layden, and A.S. Perelson, Hepatitis C viral dynamics in vivo and the antiviral efficacy of interferon-alpha therapy, Science 282 (1998), pp. 103–107. doi: 10.1126/science.282.5386.103
- M.A. Nowak and C.R.M. Bangham, Population dynamics of immune responses to persistent viruses, Science 272 (1996), pp. 74–79. doi: 10.1126/science.272.5258.74
- M.A. Nowak and R.M. May, Virus Dynamics: Mathematical Principles of Immunology and Virology, Oxford University, Oxford, 2000.
- M.A. Obaid and A.M. Elaiw, Stability of virus infection models with antibodies and chronically infected cells, Abstr. Appl. Anal. 2014 (2014). Article ID 650371. doi: 10.1155/2014/650371
- A.S. Perelson and P.W. Nelson, Mathematical analysis of HIV-1 dynamics in vivo, SIAM Rev. 41 (1999), pp. 3–44. doi: 10.1137/S0036144598335107
- A.S. Perelson, D.E. Kirschner, and R. De Boer, Dynamics of HIV infection of CD4+ T cells, Math. Biosci. 114(1) (1993), pp. 81–125. doi: 10.1016/0025-5564(93)90043-A
- R. Qesmi, J. Wu, J. Wu, and J.M. Heffernan, Influence of backward bifurcation in a model of hepatitis B and C viruses, Math. Biosci. 224 (2010), pp. 118–125. doi: 10.1016/j.mbs.2010.01.002
- R. Qesmi, S. ElSaadany, J.M. Heffernan, and J. Wu, A hepatitis B and C virus model with age since infection that exhibits backward bifurcation, SIAM J. Appl. Math. 71(4) (2011), pp. 1509–1530. doi: 10.1137/10079690X
- X. Song and A.U. Neumann, Global stability and periodic solution of the viral dynamics, J. Math. Anal. Appl. 329 (2007), pp. 281–297. doi: 10.1016/j.jmaa.2006.06.064
- P. Tanvi, G. Gujarati, and G. Ambika, Virus antibody dynamics in primary and secondary dengue infections, J. Math. Biol. 69 (2014), pp. 1773–1800. doi: 10.1007/s00285-013-0749-4
- L. Wang and M.Y. Li, Mathematical analysis of the global dynamics of a model for HIV infection of CD4+ T cells, Math. Biosci. 200(1) (2006), pp. 44–57. doi: 10.1016/j.mbs.2005.12.026
- S. Wang and D. Zou, Global stability of in-host viral models with humoral immunity and intracellular delays, J. Appl. Math. Model. 36 (2012), pp. 1313–1322. doi: 10.1016/j.apm.2011.07.086