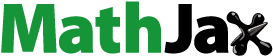
ABSTRACT
This paper introduces a novel immuno-eco-epidemiological model of competition in which one of the species is affected by a pathogen. The infected individuals from species one are structured by time-since-infection and the within-host dynamics of the pathogen and the immune response is also modelled. A novel feature of the model is the impact of the species two numbers on the ability of species one to mount an immune response. The within-host model has three equilibria: an extinction equilibrium, pathogen-only equilibrium and pathogen and immune response equilibrium which exists if the immune response reproduction number . The extinction equilibrium is always unstable, the pathogen-only equilibrium is stable if
, and the coexistence equilibrium is stable whenever it exists. The between-host competition model has six equilibria: an extinction equilibrium, three disease-free equilibria: species one-only equilibrium, species two-only equilibrium and a disease-free species coexistence equilibrium. There are also two disease-present equilibria: species one-only disease equilibrium and disease coexistence equilibrium. The existence and stability of these equilibria are governed by six reproduction numbers. Results show that for a non-fatal disease, the disease coexistence equilibrium is stable whenever it exists.
AMS SUBJECT CLASSIFICATION:
1. Introduction
Lotka–Volterra models have been successfully used to describe ecological interactions, such as competition and predation, since the beginning of the twentieth century. Lotka–Volterra models by themselves, however, did not address questions related to the interplay between host-pathogen and ecological interactions.
Approximately 75% of the recently emerging pathogens affecting humans come from animal origin [Citation15]. Understanding the distribution of pathogens in wild and domestic animal populations is a first step in studying dangerous zoonotic microorganisms. Wild animals, however, rarely exist in isolation. They are subjected to basic ecological interactions, such as predation and competition [Citation39]. There is a rich and growing literature on the interplay of community interactions and host-pathogen dynamics (see reviews in [Citation29,Citation35,Citation49]). Early papers that incorporated infectious disease into classic models of competition and predation include in particular [Citation4,Citation28]. Parasites with multiple hosts can also lead to indirect competitive interactions (apparent competition) both by direct transmission [Citation32] and by transmission via vectors [Citation12]; parasitism can also modify the outcome of preexisting competitive interactions [Citation10].
The interest in the interplay of infectious disease and community interactions, an area now termed eco-epidemiology, was enhanced by Chattopadhyay and Arino [Citation16]. The introduction of disease in the competition between two species has led to destabilization in the dynamics [Citation20]. More recently, disease infecting a predator has been found to lead to complex dynamics and chaos [Citation52]. Most of these models consist of ordinary differential equations and do not address how ecological interactions affect the host's immune response and how the within-host pathogen reproduction and immune response affect the ecological interaction (but see [Citation33,Citation51] for the impact of immunity).
Bridging the immunological scale and the epidemiological scale has been fascinating mathematical biology researchers for more than a decade [Citation21,Citation30]. The dependence of the epidemiological reproduction number on within-host pathogen load and immune responses is of key interest as it explains how the within-host dynamics affects the population-level persistence of the disease [Citation19,Citation31,Citation34,Citation36,Citation40,Citation50,Citation53]. Martcheva studied how the pathogen load affects the population-level prevalence of HIV and found that a medication-mediated decrease in viral load increases the population-level prevalence of HIV [Citation43]. This rather perplexing finding is well known among the public health authorities and is attributed to increasing the lifespan of HIV-infected individuals.
The importance of multi-scale immuno-epidemiological modelling is best highlighted by its role in studying evolution [Citation46]. Gilchrist and Sasaki [Citation27] were the first to address co-evolution but since then evolution of virulence has been attracting significant attention. Because evolution typically involves multiple strains, a number of approaches have been developed to handle the emergent complexity [Citation1,Citation2,Citation5,Citation17,Citation18,Citation25,Citation26,Citation37,Citation38]. One key limiting case (and the assumption we make in our model) is that each infected host harbors only a single strain of the pathogen.
Mathematically speaking, several different ways have been proposed for linking the within-host and between-host scales through (single-strain) differential equation models on both scales. The simplest approach seems to result in a system of ODE models, part of which describes the within-host dynamics while another part describes the between-host dynamics [Citation14,Citation22,Citation23]. These models often represent an environmentally driven disease and the within-host and between-host system are linked through the contaminated environment. The simplicity of the approach has multiple advantages and allows for significant analysis, which in turn leads to insight into biological implications. The other two modelling approaches use ODEs for the immunological model and PDEs for the epidemiological. In one of these approaches, perhaps the most complex one, the epidemiological model consists of physiologically structured PDEs in which the structural independent variables are the dynamical variables of the ODE immune model. The first such model was proposed by Martcheva and Pilyugin [Citation44] (see also [Citation41]) and since then has been improved upon by several authors [Citation6,Citation7,Citation24,Citation47]. This approach presents interesting mathematical challenges (such as the potential for measure-valued solutions) but somewhat restricts the incorporation of significant realism into the immune system. The second approach involving PDEs, originally proposed by Gilchrist and Sasaki [Citation27], links the within-host model and the between-host model through an age-since-infection variable and dependence of the epidemic parameters on the within-host variables. This approach allows for incorporation of the necessary realism in the immunological and the epidemiological systems and has been studied mathematically more often [Citation19,Citation40,Citation42,Citation43,Citation45,Citation48]. This approach leads to what is called nested immuno-epidemiological models and it is the approach we will take in this article.
Little work has been done at the interface of eco-epidemiological and immuno-epidemiological modelling, although incorporating immune responses in the Lotka– Volterra predation or competition model is a natural step to take the community interactions one step further and study how ecological interactions affect the host's immune response and how the within-host pathogen reproduction and immune response affect the ecological interaction (but see [Citation33,Citation51] for the impact of immunity). The only article we are aware of studies the interplay between predation and within-host dynamics of disease in the prey [Citation11]. In this article we introduce and investigate an eco-epidemiological model of competition with disease in species one and a nested immunological model of the within-host dynamics of the pathogen with the immune response. We further incorporate one further level of competition – we allow the population size of species two to affect the ability of species one to mount an immune response to the pathogen. We term these novel type of models immuno-eco-epidemiological models.
In the next section we introduce the within-host and the between-host eco-epidemiological model. In Section 3 we analyse the within-host model. In Section 4 we study the equilibria of the nested immuno-eco-epidemiological model and we define six multi-scale reproduction numbers that determine the existence of the equilibria. In Section 5 we study the stability of the equilibria of the nested immuno-eco-epidemiological model. Section 6 contains summary of our results.
2. An immuno-eco-epidemiological model
In this section we introduce a two-species ecological competition model, in which one of the species is infected by a pathogen, while the other is not affected by the same pathogen. Species one, that is infected by the pathogen, is structured by age-since-infection and its immune status is taken into account. The resulting immuno-eco-epidemiological model portrays the interaction of the pathogen with the immune system and how this interaction affects the global dynamics among the species. We also assume that the competition between the species exercises pressure on species one that affects its immune response to the pathogen. This model is a combination of ODEs and PDEs. The PDE model represents the population-level dynamics between the species. The host system has been classified into two species, where the number of species one is given by . Species one is in competition with species two whose numbers are given by
. The following sets of equations represent the PDE model.
(1)
(1)
represents the number hosts of species one susceptible to the pathogen and
is the corresponding number of infected hosts of species one at time t with age of infection τ. This definition leads to the following equation:
We assume that the disease is not transmitted vertically and for species one only susceptible individuals reproduce. The growth rate of the number of susceptible hosts is described by a logistic function where is the intrinsic growth and
is the carrying capacity of species one.
represents the interference that comes from species two in the growth of species one population.
is the natural death rate of species i and
represents the rate of infection for species one. α represents the rate at which the infected hosts are removed. The dynamics of species two have been modelled in a similar way using a logistic function to represent the growth of the population.
is the intrinsic growth and
is the carrying capacity of species two.
represents the interference of species one in the growth of the species two population. The PDE model above combines a Lotka–Volterra competition model with and SI epidemic model.
The ODE model represents the multiplication of virus/parasite inside a host. The within-host model captures the interplay of the virus with the immune system. We focus on these two components only as the main components of what we want to model within-host – the pathogen and the immune response, which is affected by the competition. The model is general enough to capture the within-host processes in a variety of diseases. ‘Predator–prey style’ immune models have been used widely in the literature to understand a variety of within-host and within-host-between-host processes [Citation8]. In this ODE system, represents the number of virus particles and
represents the healthy immune cells with age of infection τ. The following ODE model gives the dynamics in the number of the healthy immune cells and the number of virus particles.
(2)
(2)
We assume that the growth of the virus population occurs in a logistic fashion, where is the carrying capacity of the virus inside a host and q is the intrinsic growth rate. a is the elimination rate of the virus particles by the healthy immune system of the host. Healthy immune cells are represented by
. We use immune cells as a proxy to antibodies which mark the pathogen for destruction. Furthermore, we assume that the immune-response cells are cleared at a rate d. The constant
is the immune response activation rate in the absence of interference from species two. D represents a scaling factor. We note that the immune system contains as a parameter the number of species two
which affects negatively the immune response. We assume that being subjected to competition with species two, species one is stressed to an extent that modifies it's internal ability to mount an effective immune response to the pathogen. The impact of stress on immune system functioning is well recognized [Citation3].
The parameters of the population-level model are linked to the dynamic variables of the within-host model in the following way. It has been assumed that the rate of infection and the rate of removal of the infected hosts
are proportional to the within-host viral load.
(3)
(3) where
and
are constants of proportionality. The assumption for linking
linearly to the pathogen load is common [Citation27,Citation50]; however, newer results obtained from comparison with data suggest that a better linking is a Hill function [Citation42]. Nonetheless, we stay with the simplest case. The linking for
typically reflects also the immune response [Citation27,Citation50]; however, the presence of the immune response creates a trade-off for the impact of the competition of species two. In this article, we are only trying to investigate the negative effect due to stress. We notice that we have assumed that the disease has no recovery, which is characteristic of many diseases of wild animals (e.g. Feline Immunodeficiency Virus (FIV) in lions).
3. Analysis of the ODE model
The within-host ODE model describes the interplay between the virus and the host immune system. To understand better its dynamics, we begin by defining the equilibria of the model and then investigate the stability of each equilibrium. The equilibria are time-independent solutions of the ODE model, obtained by equating each of the equations in the ODE model to 0.
The question of equilibria in the immunological model is not completely trivial, as the model involves the time-dependent size of species two . We would investigate the equilibria at some fixed value of
which is to be determined later. Assume
and
be the equilibrium points.
(4)
(4)
Solving the above set of equations leads to the following equilibria of this system, given as ordered pairs . The immunological extinction equilibrium
and the endemic equilibrium
always exist. The co-existence equilibrium is given by
where
We see that the equilibrial level of species two suppresses the immune response and supports higher level of the virus. We observe that this equilibrium exists if and only if
. The immune-response reproduction number
is given by
This number gives the number of secondary immune particles that one immune particle stimulates when the virus is at carrying capacity and species two is at equilibrial value
.
3.1. Local stability of the equilibria
We explore the stability of the equilibria defined in the previous subsection. The presence of time-dependent species two size in system (Equation2
(2)
(2) ) is a significant complication. We assume that the equilibrium in the ODE immune system occurs after the epidemic system has stabilized at equilibrium. Therefore,
where
is any equilibrial value of epidemic system. We believe the analysis of this section can be carried out with
rather than
; however, the dependence of
on t may have implications for the conclusions. A more careful analysis of this scenario will be considered in a future work.
We consider the following asymptotically autonomous ODE model:
(5)
(5)
Theorem 3.1.
The extinction equilibrium is always unstable. The pathogen-only equilibrium
is locally asymptotically stable if
and unstable if
. The coexistence equilibrium
is locally asymptotically stable if
.
Proof.
The local stability of an equilibrium is given by the Jacobian of the system (Equation5(5)
(5) ). The Jacobian of the above system (Equation5
(5)
(5) ) is:
At the trivial equilibrium
the Jacobian reduces to
The two roots of this matrix are q and
. Since q>0, the extinction equilibrium is always unstable. At the endemic equilibrium
the Jacobian reduces to the following matrix
The roots of this Jacobian matrix are
and
. The roots are both negative if and only if
which is negative if and only if
. When
, we have that
and the endemic, immune-response-free equilibrium
is unstable.
Now, we will explore the co-existence equilibrium . At this equilibrium,
and
satisfy the following set of equations:
(6)
(6)
This simplifies the Jacobian to the following form:
Exploring the properties of this Jacobian matrix, we observe that and
. This shows that
is locally asymptotically stable.
3.2. Global stability of equilibria
In the case , the only locally stable equilibrium is
. To see the global stability of
, notice that from the first equation in Equation (Equation2
(2)
(2) ) we have that
Then from the second equation, we have
Hence
. From here, it is not hard to prove that
which establishes the global stability of
.
In the case of , only equilibrium
is locally asymptotically stable. To its see global stability for system (Equation2
(2)
(2) ), we use the fact that the coexistence equilibrium is locally stable whenever it exists. The term
is treated as a parameter. We use Dulac's criterium on the open first quadrant
to rule out periodic orbits. Let
and
Define Dulac's auxiliary function . Then we find that
By Dulac's criterion, there are no periodic solutions. By Poincare Bendixon theorem, the solutions converges to an equilibrium. When
, both
and
are unstable. Since we have already proved the local stability of
, by Poincare Bendixon theorem, we conclude that the co-existence equilibrium
is globally asymptotically stable.
From this analysis, we observe that the ODE model has two regimes: if the virus persist but the immune system does not get activated. If
, both the virus and the immune system persist. The system (Equation2
(2)
(2) ) does not predict that the virus can be cleared. Thus, the system models chronic infections. This is reasonable since animal populations do not receive treatment and many infections for them are not cleared.
4. Equilibria of the PDE model
In this section we will explore the equilibria of the PDE model. We incorporate the within-host model with its dynamical properties; that is the within-host model is not at equilibrium.
We begin by introducing some notation that we will use throughout the article. The probability of survival of species one as infected until time τ post infection is given by
We note that the probability of survival depends on the within-host viral load
through
Equation (Equation3
(3)
(3) ). Furthermore, we denote by
We note that G depends on
through the within-host system. G in the text will also stand for
. We note that
gives the probability of dying from natural causes while infectious. We further adopt the following two notations:
and
The existence and stability of the equilibria of the immuno-eco-epidemiological model (Equation1
(1)
(1) ) depend on a number of reproduction numbers and invasion reproductions numbers. We list these key quantities in the following definition.
Definition 4.1.
We define the reproduction numbers used in this model as follows.
The basic reproduction number for species one
is defined as
Species one only disease-free reproduction number
Species one only susceptible-disease reproduction number
where
The basic reproduction number for species two
is defined as
The endemic reproduction number for species one is given by
where
is the solution of equation for the species one endemic equilibrium with
. This equation will be defined in the following section.
The endemic reproduction number for species two is defined as
The co-existence species one-species two, no disease reproduction number is given by
The equilibria are obtained by equating the time derivatives to 0. Since each of the variables is independent of time, we let
This leads to the following set of equations for the equilibria:
(7)
(7)
We will obtain the equilibrium as an ordered set , where the elements in the ordered set satisfy the relationship,
.
4.1. The eco-epidemiological extinction equilibrium
The eco-epidemiological extinction equilibrium always exits, since it always satisfies Equations (Equation7
(7)
(7) ).
4.2. Semi-trivial equilibria
Case 1: Disease-free Equilibria
(a) We observe that there exists a semi-trivial species one equilibrium in the form . Note that this equilibrium exists, if and only if
.
(b) Now, we will explore the semi-trivial species two equilibrium where species one is not present. Let us assume . It is clear from this assumption that
. Hence, the species two equilibrium is defined as
. To find
, we have to solve the following equation:
(8)
(8) Solving for
, we obtain
Note that this equilibrium exists if and only if the reproduction number of species two is larger than one:
.
Case 2: Semi-trivial endemic equilibria. Species one endemic equilibrium exists if and only if species one-disease reproduction number is larger than one. This result is given by the following theorem:
Theorem 4.1.
There exists an endemic equilibrium in the form
if and only if
.
Proof.
We examine the boundary equilibria when and
. In this case the system for the equilibria (Equation7
(7)
(7) ) reduces to solving the following set of equations:
(9)
(9)
Solving for , we obtain
Recall that . Using this expression in the boundary condition, we obtain the value of
:
Using the equation
, we obtain the value of
:
Recall that
. Thus, we can express every term in the equilibrium as a function of
. Substituting all these in the first equation of (Equation9
(9)
(9) ) we obtain the following quadratic equation for
:
Note that
Hence, the constant term in the quadratic polynomial is negative. The leading co-efficient is positive. By intermediate value theorem, it can be proved that there always exists a unique positive solution for
. It remains to prove that
.
To see that , we rewrite the polynomial in a different form. We can define
as the solution of the polynomial
where
We observe at , we have
From the structure of the endemic reproduction number
, we have
. Note that as
,
approaches a negative quantity. Hence, there exists a positive solution
such that
. Note that in this case
. This proves the existence of an endemic species one equilibrium
where
.
4.3. Species co-existence equilibria
There are several equilibria where the two species coexist. These are species one-species two no disease equilibrium and species one-disease-species two equilibrium.
Case 1: Species one- species two, no disease equilibrium.
This equilibrium is obtained from solving the following sets of linear equation in two variables.
(10)
(10)
Lemma 4.1.
System of Equations (Equation10(10)
(10) ) has a solution when
and
.
Proof of Lemma.
Proof of Lemma
Note and
.
From these two inequalities, it also follows that . Hence from Cramer's rule, we have positive solution for the set of linear equation in (Equation10
(10)
(10) ). This completes the proof.
Case 2: Disease-present coexistence equilibrium .
Now, we consider the disease-coexistence equilibrium. The existence is given by the following theorem.
Theorem 4.2.
Assume
and
. Then, there exists
.
Proof.
The system that has to be solved to obtain this equilibrium is given by
(11)
(11)
Solving for we obtain
. Using the boundary conditions, we obtain
The other equations can be solved to express
is terms of
and
as follows:
Using the last equation in (Equation11
(11)
(11) ) we can express
as a function of
as
This expresses every term as a function of
. Thus, the solution
is obtained by solving the equation
where
and
Note that for
we have Q>0.
Since and
, as solution of Equation (Equation5
(5)
(5) ), depends on
, we can consider
, a function of
.
At N=Q in the characteristic equation, we have
(12)
(12)
From the argument presented in the Proof of Theorem 3.1, we can claim that . As
, it clearly follows that
.
Observe that
Hence,
From the fact that
, we have
Hence,
The first multiple in the product is negative. From
, we have that
Therefore, the second factor is also negative. We have that
. By the intermediate value theorem, we can now claim that there exist a solution
to the equation
such that
. From this condition and from the definition of
, it is clear that there exist a positive solution
which clearly defines this equilibrium.
5. Local stability of the equilibria
In this section, we will explore the stability of the different equilibria. In general, if the equilibrium is defined as the ordered n-tuple , where
we will observe the change in the dynamical system when the system is perturbed from its equilibrium state. The following defines the perturbations applied to the system.
(13)
(13)
With this perturbations, the system changes to the following form:
(14)
(14)
Linearizing these equations and only retaining the terms which are linear we obtain the following set of equations for the perturbations.
(15)
(15)
5.1. Stability of the trivial equilibrium
Theorem 5.1.
When the trivial equilibrium is locally asymptotically stable. It is unstable when
where
, i.e. the equilibrium is locally asymptotically stable when both
are less than one and unstable when at least one of them is greater than one.
Proof.
This equilibrium is defined by . This leads to the following set of equations:
(16)
(16)
The solution of is given by
. From the last equation in (Equation16
(16)
(16) ) it is clear that when
and hence the system is unstable. When
, we have to investigate other eigenvalues which come from the other equations in the system given by Equation (Equation16
(16)
(16) ). Note the boundary condition on η forces the general solution to be of the form
for all t. This leads to the fact
.
Hence we have the solution of s as . Note that
and hence the system is unstable. When
it follows that
and hence the system is locally asymptotically stable.
Hence, all possible solutions to this system of equations are either negative or have negative real parts if and only if both and
are less than one. If any one of these is greater than one, the system is unstable.
5.2. Stability of the semi-trivial equilibria
In this section, we explore the local stability of semi-trivial equilibria. There are three semi-trivial equilibria. We begin by deriving the local stability of the boundary equilibria
and
, and then explore the stability of the other non-trivial boundary equilibrium
.
5.2.1. Stability of 

Theorem 5.2.
This equilibrium is stable when
but
and
.
Proof.
Note in this case we have . Substituting
for this equilibrium in the equation of stability in Equation (Equation15
(15)
(15) ), where
, we have the following set of equations:
(17)
(17)
The last equation can be solved to obtain a closed-form solution
Substituting
, we obtain the solution as,
From the definition of it follows that if
this equilibrium is unstable. When
, we shall explore other eigenvalues in this system. We linearize the system using the following functions,
. This gives the following set of equations:
(18)
(18)
Solving the equation for η we have . Substituting this in the boundary condition, the equation leads to the following characteristic equation
where
Note that as . We observe that
When it is clear that
. Thus, there exists a positive solution for λ when
and the system is unstable. For the case, when
, let us assume there exists a
with nonnegative real part
. This shows that
whenever
, which is a contradiction. Hence, there does not exist any root λ with nonnegative real part when
. All roots are either negative or have negative real part. We will explore another possible eigenvalue in this case. From the first equation in (Equation18
(18)
(18) ) we have,
. Substituting the value of
, we have the following eigenvalue,
. Note this equilibrium is unstable when
and stable when
.
5.2.2. Stability of 

The equilibrium in this part is given by where
. This equilibrium exists only if
.
Theorem 5.3.
Assume . The equilibrium
is locally asymptotically stable if and only if
.
Proof.
Substituting and
in the linearized equations for local stability (Equation14
(14)
(14) ), we obtain the following set of equations:
(19)
(19)
We look for separable solutions of Equations (Equation19(19)
(19) ) in the form
. Substituting these forms in the system of Equations (Equation19
(19)
(19) ) and simplifying the system we obtain the following linear eigenvalue problem:
(20)
(20)
It is easy to observe from the boundary condition of that
. The eigenvalues will follow from solving the other equations. From
it follows that
. The first equation in (Equation20
(20)
(20) ) changes to the following form:
(21)
(21)
We will determine the eigenvalues for the following two possibilities of .
Case 1 Let . This will reduce Equation (Equation21
(21)
(21) ) to
When the reproduction number for species two , we have
. In this case we continue with Case 2. On the other hand, when
the eigenvalue λ will be positive and as a consequence the equilibrium will be unstable. Note the reproduction number as defined before is given as
Case 2 Let . In this case, the remaining eigenvalue will be given by the equation involving
as
(22)
(22)
Substituting this reduces to
which is a negative quantity. Hence, if
, this equilibrium is locally asymptotically stable. This completes the proof.
5.2.3. Stability of 

The endemic equilibrium is given by
, where
The next theorem gives the stability of . To state the theorem, define
Theorem 5.4.
Assume exists. If
then
is unstable. If
then
if
and
then
is locally asymptotically stable;
if
and
then
is unstable.
Remark 5.1.
In the case , we could not prove that the system is stable. In addition, it is not hard to see that the linearized system does have a positive eigenvalue in this case. Thus, a possibility exists that a pair of complex conjugate eigenvalues cross the imaginary axis causing Hopf bifurcation to occur and the presence of sustained oscillations.
Proof.
Using the linearization defined in Equation (Equation14(14)
(14) ) and using the values of this equilibrium, we arrive at the following set of equations:
(23)
(23)
Note that the equation for separates from the remaining equations and we have
When
, the exponent is positive and hence the system is unstable. To determine the stability of this system when
, we have to investigate the remaining eigenvalues.
We are considering roots of the characteristic equation which are different from the previous one. We look for separable solutions in the form, . We solve for η to obtain the solution in the form
. Using this set of equations and substituting this in Equation (Equation23
(23)
(23) ), we have
(24)
(24)
Solving this system for and
from the first equation in Equation (Equation24
(24)
(24) ), we obtain
(25)
(25)
Combining the two equations from Equation (Equation25(25)
(25) ), we obtain the following solution for
:
Substituting this equation in the second equation in Equation (Equation24(24)
(24) ) we obtain the characteristic equation
where
and B denotes the constant
. The eigenvalues of the problem (Equation24
(24)
(24) ) are the values of λ that solve the characteristic equation. Let λ be the solution for the characteristic equation
.
Using the following notations, we define the respective functions.
The notations reduce the characteristic equation to the form
(26)
(26)
Let with
. For these λ, the right-hand side of this equation satisfies
The left-hand side gives
(27)
(27) The last inequality holds, because
. In addition,
In the second case when , it is not hard to see that the characteristic equation has a positive root.
5.3. Stability of the co-existence equilibria
In this section, we shall explore the stability of the co-existence equilibria of the two species both when the disease is present in species one and when it is absent.
5.3.1. Stability of the equilibrium 

In this case the equilibrium values and
satisfy the following set of equations:
Theorem 5.5.
Assume and
. Assume also
. Then, the equilibrium
is locally asymptotically stable.
Proof.
The set of equations for the equilibrium values reduce the stability equation to the following form:
(28)
(28)
Using the substitution , it reduces the equation for η to
which can be integrated to obtain
(29)
(29)
Using the boundary condition, we obtain the characteristic equation as , where
If there exists a solution λ of the characteristic equation, such that , we have
whereas
which is a contradiction. This last inequality follows from the fact that
. Hence, all roots are negative or have negative real parts. Now, we explore the remaining roots. We assume
. Note that this results in
. The roots are obtained from the following set of equations:
(30)
(30)
Using the solution in the form, , we have
(31)
(31)
This can be combined to obtain the characteristic equation , where
is the polynomial function given as
Since we have in this case, all roots λ are either negative or have negative real parts. Hence, this equilibrium is always stable whenever
.
5.3.2. Stability of the equilibria 

Regarding the stability of the disease-present coexistence equilibrium, we have the following result:
Theorem 5.6.
Let . If
then the equilibrium
is locally asymptotically stable whenever it exists.
Proof.
From the linearization presented in Equation (Equation15(15)
(15) ), we have the following sets of equations:
(32)
(32)
We look for solutions in the form . This transforms the set of equations to the following form:
(33)
(33) where
. We further note that from the equilibrium equations, we have
. Solving the last equation in Equation (Equation33
(33)
(33) ), we obtain
in terms of
as follows:
Substituting this in the first equation of (Equation33
(33)
(33) ), we obtain an explicit form of s in terms of
and η as follows:
(34)
(34)
We replace s using the following form . The boundary condition of the PDE in Equation (Equation33
(33)
(33) ) reduces to
, where
and
. Using these substitutions in Equation (Equation35
(35)
(35) ), we obtain the following form:
(35)
(35)
Combining all these equations, we obtain the characteristic equation , where
is given by
(36)
(36) where
Note that we can rearrange to write it in the form,
It is clear that and
. Note that
Hence, we have
(37)
(37) where we recall that
,
, and that
. Consequently, the equilibrium is unstable if
, or alternatively if
.
Now, we consider the case . Then
,
and
. Hence,
To show that in this case the characteristic equation does not have roots with nonnegative real parts, we write
where
and
. Since the characteristic equation is given by
, one can take a common denominator of
to obtain
Further simplification leads to
Using the expression for
above, we have
Hence, the characteristic equation simplifies to
Thus, in the case
the characteristic equation splits into two equations:
If we rewrite the first equation as
then, for λ with
we have
. On the other hand
and hence this equation does not have roots with nonnegative real parts. The second equation is a quadratic equation:
In order for this equation to have only roots with negative real parts, the coefficients must be positive. We consider the constant term. The expression
has the same sign as
This also implies that
. This completes the proof.
6. Discussion
In this paper we define a novel immuno-eco-epidemiological model of species competition. The model is based on a Lotka–Volterra competition model, where species one is infected by a pathogen, that does not affect species two. Competition of species in nature where only one of the species is affected by a pathogen is rare, but examples can be found. For instance, cutaneous fibromas caused by papillomaviruses affect white-tailed deer. The disease is restricted to deers and does not affect sheep, which are a natural competitor for food [Citation9,Citation13]. A broader application of this model is as a threshold case modelling well the circumstances when one of the species is seriously affected by the disease, while the other is affected little. This, for instance occurs often in competition between wild life and domestic animals, since domestic animals, even if subjected to a pathogen, are often treated and there is less implication of the pathogen to their well-being. Recognizing the importance pathogen affecting one species in competition with another, Anderson and May first considered this scenario [Citation4]. The novelty here is that the second species interferes with the first not only in the competition for resources but also though lowering its ability to effectively combat the pathogen on within-host level.
Infected individuals are structured by age-since-infection, and the within-host dynamics between the pathogen and the immune response is taken into account. A novel feature of the model is the dependence of the within-host dynamics of species one on the numbers of species two. Thus, we incorporate competition on two levels: ecological and within host, where the competition from species two obstructs species one from mounting an effective immune response against the pathogen.
The within-host model, at an equilibrium of species two, has three equilibria: extinction equilibrium, which is always unstable, pathogen-only equilibrium, which is stable if the immune response reproduction number and pathogen and immune response equilibrium, which is stable if
. The immune response reproduction number depends on the equilibrial level of species two; hence, the more individuals in species two, the weaker immune response will species one mount.
The age-since-infection structured eco-epidemiological model has six equilibria, whose existence is controlled by six reproduction numbers. Eco-epidemiological extinction equilibrium always exists. There are also three disease-free equilibria: one corresponding to healthy species one
, one corresponding to species two,
and one corresponding to the coexistence of healthy species one and species two
which exist under appropriate conditions of the reproduction numbers. Finally, there is a unique species one-disease equilibrium
and (potentially multiple) disease-coexistence equilibria
, which also exist under appropriate conditions on the reproduction numbers.
To investigate the stability of the eco-epidemiological equilibria, we again assume that the within-host system is computed at the equilibrial level of species two. We find that the extinction equilibrium is locally asymptotically stable if the max of the species one and species two basic reproduction numbers is below one, , and unstable if
. Species one equilibrium
is stable if the basic reproduction number of species one is above one, but
and
. Species two equilibrium
is stable if the basic reproduction number of species two is above one but
. The disease-free coexistence equilibrium
is stable whenever
. Species one-disease equilibrium
is locally asymptotically stable if
and
with
. If
but
this equilibrium is unstable (saddle). In the case of a non-fatal disease, that is
, the disease-present coexistence equilibrium is locally asymptotically stable whenever it exists.
In summary, this is a complex model with multiple equilibria and conditions for existence and stability of these equilibria. The ecological competition of the species is affected by the within-host dynamics of the pathogen through the effect of the within-host dynamics exercised on the threshold quantities governing the outcome of the competition of the species and the impact of the disease.
Acknowledgments
The authors also acknowledge the extremely helpful remarks of one referee which lead to improvement of the paper. This article has benefitted from discussion with Michael Barfield and Robert D. Holt.
Disclosure statement
No potential conflict of interest was reported by the authors.
Additional information
Funding
References
- S. Alizon and S. Lion, Within-host parasite cooperation and the evolution of virulence, Proc. R. Soc. London, Ser. B 278 (2011), pp. 3738–3747.
- S. Alizon, F. Luciani, and R.R. Regoes, Epidemiological and clinical consequences of within-host evolution, Trends Microbiol. 19 (2011), pp. 24–32.
- American Psychological Association, Stress weakens the immune system, Available at http://www.apa.org/research/action/immune.aspx.
- R.M. Anderson and R.M. May, The invasion, persistence and spread of infectious diseases within animal and plant communities, Philos Trans R Soc Lond B Biol Sci. 314(1167) (1986), pp. 533–570.
- J.-B. André and S. Gandon, Vaccination, within-host dynamics, and virulence evolution, Evolution 60(1) (2006), pp. 13–23.
- O. Angulo, F. Milner, and L. Sega, A SIR epidemic model structured by immunological variables, J. Biol. Syst. 21 (2013), p. 1340013.
- O. Angulo, F.A. Milner, and L.M. Sega, Immunological models of epidemics, MACI (Matematica Aplicada, Computacional e Industrial) 4 (2013), pp. 53–56.
- R. Antia, B.R. Levin, and R.M. May, Within-host population dynamics and the evolution and maintenance of microparasite virulence, Amer. Nat. 144(3) (1994), pp. 457–472.
- W.E. Armstrong, White-tailed deer competition with goats, sheep, cattle and exotic wildlife, in Wildlife Management Handbook, 2011, pp. V-A1–V-A4. Available at http://roberts.agrilife.org/files/2011/06/whitetailed_deer_competitionother_animals_17.pdf.
- M. Begon, R.G. Bowers, N. Kadianakis, and D.E. Hodgkinson, Disease and community structure: The importance of host self-regulation in a host–host-pathogen model, Amer. Nat. 139 (1992), pp. 1131–1150.
- S. Bhattacharya, M. Martcheva, and X.-Z. Li, A predator–prey-disease model with immune response in infected prey, J. Math. Anal. Appl. 411(1) (2014), pp. 297–313.
- M.B. Bonsall and R.D. Holt, Apparent competition and vector-host interactions, Israel J. Ecol. Evol. 56 (2010), pp. 393–416.
- T.A. Campbell and K.C. VerCauteren, Diseases and parasites, in Biology and management of White-Tailed Deer, D. Hewitt, ed., Boca Raton, FL, CRC Press, 2011, pp. 219–249.
- X. Cen, Z. Feng, and Y. Zhao, Emerging disease dynamics in a model coupling within-host and between-host systems, JTB 361 (2014), pp. 141–151.
- Centers for Disease Control and Prevention, National center for emerging and zoonotic infectious diseases, Available at http://www.cdc.gov/ncezid/.
- J. Chattopadhyay and O. Arino, A predator–prey model with disease in the prey, Nonlinear Anal. 36 (1999), pp. 747–766.
- D. Coombs, M.A. Gilchrist, and C.L. Ball, Evaluating the importance of within- and between-host selection pressures on the evolution of chronic pathogens, Theoret. Popul. Biol. 72 (2007), pp. 576–591.
- T. Day, S. Alizon, and N. Mideo, Bridging scales in the evolution of infectious disease life histories: theory, Evolution 65 (2011), pp. 3448–3461.
- S. DebRoy and M. Martcheva, Immuno-epidemiology and HIV/AIDS: A Modeling prospective, in Mathematical Biology Research Trends, L.B. Wilson, ed., Nova Publishers, New York, 2008, pp. 175–192.
- P. van den Driessche and M.L. Zeeman, Disease induced oscillations between two competing species, SIAM J. Appl. Dyn. Syst. 3 (2004), pp. 601–619.
- J. Dushoff, Incorporating immunological ideas in epidemiological models, J. Theoret. Biol. 180 (1996), pp. 181–187.
- Z. Feng, J. Velasco-Hernandez, B. Tapia-Santos, and M. Leite, A model for coupling within-host and between-host dynamics in an infectious disease, Nonlinear Dyn. 68 (2011), pp. 401–411.
- Z. Feng, J. Velasco-Hernandez, and B. Tapia-Santos, A mathematical model for coupling within-host and between-host dynamics in an environmentally-driven infectious disease, MBS 241(1) (2013), pp. 49–55.
- A. Gandolfi, A. Pugliese, and C. Sinisgalli, Epidemic dynamics and host immune response: a nested approach, J. Math. Biol. 70(3) (2015), pp. 399–435.
- V.V. Ganusov, C.T. Bergstrom, and R. Antia, Within-host population dynamics and the evolution of microparasites in a heterogeneous host population, Evolution 56 (2002), pp. 213–223.
- M.A. Gilchrist and D. Coombs, Evolution of virulence: Interdependence, constraints, and selection using nested models, Theoret. Pop. Biol. 69 (2006), pp. 145–153.
- M.A. Gilchrist and A. Sasaki, Modeling host-parasite coevolution: a nested approach based on mechanistic models, J. Theoret. Biol. 218 (2002), pp. 289–308.
- K.P. Hadeler and H.I. Freedman, Predator-prey populations with parasitic infection, J. Math. Biol. 27 (1989), pp. 609–631.
- M.J. Hatcher and A.M. Dunn, Parasites in Ecological Communities: From Interactions to Ecosystems, Cambridge University Press, Cambrige, 2011.
- B. Hellriegel, Immunoepidemiology: Bridging the gap between immunology and epidemiology, Trends Parasitol. 17 (2001), pp. 102–106.
- R.D. Holt and M. Barfield, Within-host pathogen dynamics: Some ecological and evolutionary consequences of transients, dispersal mode, and within-host spatial heterogeneity, DIMACS Ser. Discrete Math. Theoret. Comput. Sci. 71 (2006), pp. 45–66.
- R.D. Holt and J. Pickering, Infectious disease and species coexistence: a model of Lotka–Volterra form, Amer. Nat. 126 (1985), pp. 196–211.
- R.D. Holt and M. Roy, Predation can increase the prevalence of infectious disease, Amer. Nat. 169 (2007), pp. 690–699.
- G.L. Johnston, D.L. Smith, D.A. Fidock, and R. Antia, Malaria's missing number: calculating the human component of R0 by a within-host mechanistic model of Plasmodium falciparum infection and transmission, PLOS Comput. Biol. 9(4) (2013), p. e1003025.
- M.J. Keeling and P. Rohani, Modelling Infectious Diseases in Humans and Animals, Princeton University Press, Princeton, NJ, 2008.
- T. Kostova, Persistence of viral infections on the population level explained by an immunoepidemiological model, Math. Biosci. 206 (2007), pp. 309–319.
- P. Lemey, A. Rambaut, and O. Pybus, HIV evolutionary dynamics within and among hosts, AIDS Rev. 8 (2006), pp. 125–140.
- F. Luciani, S. Alizon, and C. Fraser, The evolutionary dynamics of a rapidly mutating virus within and between hosts: The case of hepatitis C virus, PLoS Comput. Biol. 5 (2009), p. e1000565.
- M. Martcheva, Evolutionary consequences of predation for pathogens in prey, Bull. Math. Biol. 71(4) (2009), pp. 819–844.
- M. Martcheva, An Immuno-epidemiological model of paratuberculosis, AIP Conf. Proc. 1404 (2011), pp. 176–183.
- M. Martcheva, An evolutionary model of influenza a with drift and shift, J. Biol. Dyn. 6(2) (2012), pp. 299–332.
- M. Martcheva, An Introduction to Mathematical Epidemiology, Springer, New York, 2015.
- M. Martcheva and X.-Z. Li, Linking immunological and epidemiological dynamics of HIV: the case of super-infection, J. Biol. Dyn. 7(1) (2013), pp. 161–182.
- M. Martcheva and S.S. Pilyugin, An epidemic model structured by host immunity, J. Biol. Syst. 14 (2006), pp. 185–203.
- M. Martcheva, S. Lenhart, S. Eda, D. Klinkenberg, E. Momotani, and J. Stable, An Immuno-epidemiological models for Johne's disease in cattle, Vet. Res. 46 (2015), p. 69.
- N. Mideo, S. Alizon, and T. Day, Linking within- and between-host dynamics in the evolutionary epidemiology of infectious diseases, Trends Ecol. Evol. 23 (2008), pp. 511–517.
- F.A. Milner and L.M. Sega, Integrating immunological and epidemiological models, in 18th World IMACS/MODSIM Congress, Cairns, Australia, 13–17 July 2009. Available at http://mssanz.org.au/modsim09.
- E. Numfor, S. Bhattacharya, S. Lenhart, and M. Martcheva, Optimal control of nested within-host and between-host model, Math. Model. Nat. Phenom. 7(2) (2014), pp. 171–203.
- R.S. Ostfeld, F. Keesing, and V.T. Eviner, The ecology of infectious diseases: progress, challenges, and frontiers, in Infectious Disease Ecology: Effects of Ecosystems on Disease and of Disease on Ecosystems, R.S. Ostfeld, F. Keesing, and V. Eviner, eds., Princeton University press, Princeton, NJ, 2008, pp. 469–482.
- A. Pugliese, The role of host population heterogeneity in the evolution of virulence, J. Biol. Dyn. 5 (2011), pp. 104–119.
- M. Roy and R.D. Holt, Effects of predation on host-pathogen dynamics in SIR models, Theoret. Popul. Biol. 73 (2008), pp. 319–331.
- D. Stiefs, E. Venturino, and U. Feudel, Evidence of chaos in eco-epidemic models, Math. Biosci. Eng. 6 (2009), pp. 855–871.
- D.M. Vickers and N.D. Osgood, A unified framework of immunological and epidemiological dynamics for the spread of viral infections in a simple network-based population, Theoret. Biol. Med. Model. 4 (2007), p. 49.