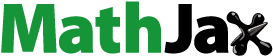
ABSTRACT
In biochemical networks transient dynamics plays a fundamental role, since the activation of signalling pathways is determined by thresholds encountered during the transition from an initial state (e.g. an initial concentration of a certain protein) to a steady-state. These thresholds can be defined in terms of the inflection points of the stimulus–response curves associated to the activation processes in the biochemical network. In the present work, we present a rigorous discussion as to the suitability of finite-time Lyapunov exponents and metabolic control coefficients for the detection of inflection points of stimulus–response curves with sigmoidal shape.
2010 MATHEMATICS SUBJECT CLASSIFICATION:
1. Introduction
The study of long-term dynamics of mathematical models describing biological phenomena has received considerable attention in the past, with particular attention to steady-states and oscillatory solutions. Many methods for studying steady-state signals in biological models based on ordinary differential equations (ODE) have been developed (see, e.g. [Citation10,Citation15] and the references therein). However, transient effects can play a fundamental role as well. For instance, in biological networks the sequence of cellular processes can be affected by the short-term behaviour of time-varying signals, as is observed, for example, in cell apoptosis [Citation1], transient pathology [Citation14] or phytotransduction [Citation18]. Typically, the activation of signal transduction pathways is determined by thresholds encountered during the transition from an initial state (e.g. an initial concentration of a certain protein) to a steady state. These thresholds can be defined in terms of the inflection points of the stimulus–response curves associated to the activation processes in the biochemical network, such as cell differentiation, apoptosis, DNA damage response, etc. In our investigation, such stimulus–response curves will be assumed to have a sigmoidal shape (to be formally defined later), as is common in the determination of thresholds in biological networks [Citation3,Citation4].
Previous investigations have focused on network properties leading to sigmoidal stimulus–response curves in the steady-state dynamics [Citation17,Citation21]. For small biological networks, stimulus–response curves of the transient and long-term dynamics can be studied in detail, leading to a straightforward identification of putative thresholds. However, in larger biochemical networks the identification of putative thresholds can be more challenging, in particular in the case when the network components present sigmoidal response curves during different time intervals. Therefore, it is essential to develop quantitative measures that are suitable for the identification of putative thresholds during the transient behaviour of biological networks.
Examples of quantitative measures to characterize the transient dynamics of signal transduction pathways include metabolic control coefficients (MCC) [Citation14] and finite-time Lyapunov exponents (FTLE) [Citation1,Citation19]. In fact, MCC were originally introduced to study the dynamics near steady states (see [Citation10,Citation15]), but then proved to be still effective for the analysis of transient behaviour. Ingalls and Sauro [Citation14] extended the steady-state results of MCC to special types of dynamic response, e.g. periodic behaviour and trajectories near stable steady states, by measuring time-varying sensitivities along arbitrary solutions. Aldridge et al. [Citation1] employed FTLE to identify phase-space domains of high sensitivity to initial conditions, which enables the identification of separatrices that quantitatively characterize network responses in terms of initial conditions determining cell death or survival.
Rateitschak and Wolkenhauer [Citation19] suggested that FTLE can be used to reliably identify thresholds as inflection points of stimulus–response curves in transient dynamics, while MCC are more suitable for comparing the amplitude of different thresholds. Nevertheless, their conclusions are based on numerical simulations of three examples and mathematical proofs of their claims are missing. In the present paper, we tackle the question whether FTLE and MCC are indeed reliable measures for detecting thresholds in stimulus–response curves with sigmoidal shape, and if so, under what conditions meaningful estimates of such thresholds can be obtained.
The paper is structured as follows. In Section 2, we introduce the basic mathematical framework required for our study. Then, our main objects of interest, FTLE and MCC, are defined in Section 3. There, we derive one of the main results of the paper, which establishes a connection between FTLE and MCC. In Section 4, the concept of (sigmoidal) stimulus–response curve is introduced, and the main concern here is to investigate the suitability of both FTLE and MCC to detect inflection points of stimulus–response curves. The main results of the paper are illustrated by numerical examples. Some concluding remarks are presented at the end of the paper.
2. Basic setup and notation
Throughout this paper, we consider the nonautonomous differential equation
(1)
(1) where
,
and
is a continuous function and continuously differentiable in the second argument. Let
be the solution operator of Equation (Equation1
(1)
(1) ), i.e.
solves Equation (Equation1
(1)
(1) ) subject to the initial condition
. Here, it is assumed that for every
,
exists on the whole interval I. Furthermore, denote by
the time-T map
(2)
(2)
Moreover, to study the evolution of small perturbations near a solution ,
, of Equation (Equation1
(1)
(1) ), we consider the variational equation along
(3)
(3) We further denote by
the transition matrix of the linear system (Equation3
(3)
(3) ), i.e. for any
,
solves the problem (Equation3
(3)
(3) ) subject to the initial condition
.
Similarly to Equation (Equation2(2)
(2) ), we define the time-T transition matrix
of Equation (Equation3
(3)
(3) ) by
(4)
(4) Due to Equations (Equation2
(2)
(2) ) and (Equation4
(4)
(4) ) and the relation
it follows immediately that
(5)
(5)
To conclude this preliminary part, we introduce the symbol to denote the Grassmann manifold of all k-dimensional subspaces of
.
3. FTLE and their relation to MCC
Denote by the ordered singular values of a matrix
, which are defined as the square roots of the eigenvalues of
[Citation13]. The FTLE associated with a solution
of Equation (Equation1
(1)
(1) ) on the interval
are defined as [Citation7–9]
(6)
(6) see also [Citation20]. In our discussion, it will be convenient to use the following alternative expressions for the FTLE
(7)
(7) for all
,
and
, where
stands for the Euclidean norm in
. The formulae (Equation7
(7)
(7) ) are a direct consequence of the Courant–Fischer theorem for singular values [Citation13].
The largest FTLE can be interpreted as a measure of the maximal average exponential separation rate between the solution
starting at x and neighbouring trajectories with initial point infinitesimally close to x, over the time interval
, see [Citation8].
Example 3.1.
Consider the planar system
(8)
(8) with
and
(9)
(9)
System (Equation8(8)
(8) ) can be interpreted in a biological framework as follows. The first equation describes an exponential growth without saturation, which may represent the reproduction behaviour of a certain organism in a favourable environment. The function
,
, can be associated with the population density of a second organism that decays exponentially in absence of the first. According to Equation (Equation8
(8)
(8) ), the only source term of the second organism is given by the function g, which is the well-known Hill function. It was first introduced by Hill [Citation12] at the beginning of the twentieth century to describe the relationship between oxygen tension and the saturation of haemoglobin with oxygen, under equilibrium conditions. The Hill function has been used in numerous biological applications and beyond, in particular to describe biological phenomena exhibiting sigmoidal kinetics [Citation3,Citation6,Citation11].
Throughout this paper, we will consider system (Equation8(8)
(8) ) for the case
,
and
. Let us compute the FTLE associated with a solution of Equation (Equation8
(8)
(8) ) starting at
defined over the interval
. The time-T map of Equation (Equation8
(8)
(8) ) has the explicit form (cf. (Equation2
(2)
(2) ))
(10)
(10) With this expression, we can compute the time-T transition matrix via Equation (Equation5
(5)
(5) ), which gives
(11)
(11)
In Figure we show the behaviour of the corresponding FTLE as varies, for T=1 fixed. In this example,
can be computed explicitly by combining Equations (Equation11
(11)
(11) ) and (Equation6
(6)
(6) ). In this case,
are
-independent, as can be seen from Equation (Equation11
(11)
(11) ).
Figure 1. FTLE computed for system (Equation8(8)
(8) ) at T=1.
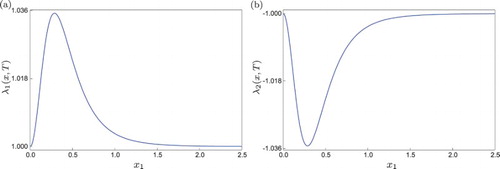
Let us now introduce another quantity that, together with FTLE, will play a central role in our discussion. In the context of metabolic control analysis [Citation11], one of the main concerns is to analyse the role of a specific substance in reactions taking place in living cells. Due to the high number of variables and regulatory interrelations involved in such processes, different approaches have been developed in order to quantitatively characterize the various metabolic pathways determining fluxes and metabolite concentrations. One way to deal with this issue is by means of the so-called MCC, defined as (cf. [Citation19])
(12)
(12) for
such that
, where
and
. The matrix
is referred to as the metabolic control matrix. The coefficient
gives a (dimensionless) local measure of the relative change in the ith output of the system with respect to an infinitesimal relative change in the jth component of the initial conditions, in the vicinity of a reference state x. The MCC can also be interpreted as a sensitivity measure (with respect to initial conditions), which is a concept widely used in engineering applications [Citation2].
For , let us denote by
a diagonal matrix with entries
in the diagonal. A straightforward computation shows that
(13)
(13) holds for all
and
with
,
. The expression (Equation13
(13)
(13) ) establishes a clear connection between the time-T transition matrix
and the metabolic control matrix
. This relation motivates the main result of the present section shown below.
Theorem 3.1.
Suppose that there exist constants 0<m<M such that the set
is not empty. Then the following estimates
(14)
(14) hold for all
and
.
Proof.
Define a family of linear operators ,
, parametrized by
. Note that
is invertible for all
and
(15)
(15)
(16)
(16)
(17)
(17)
(18)
(18)
hold for all
and
. In order to prove Equation (Equation14
(14)
(14) ), we will use the formulae introduced in Equation (Equation7
(7)
(7) ) for the computation of FTLE as follows. Choose
for some
. By Equations (Equation13
(13)
(13) ), (Equation15
(15)
(15) ) and (Equation17
(17)
(17) ) it follows for
that
(19)
(19) Introducing
in Equation (Equation19
(16)
(16) ) and taking the infimum over all
yield
Next, after taking the supremum over all
, we arrive at
Due to the fact that for any
there exists a
such that
, we can write the last inequality as
By using formula (Equation7
(7)
(7) ) for the computation of FTLE we obtain
(20)
(20) for all
. Following a similar argument allows us to show for
that (see Equations (Equation16
(16)
(16) ) and (Equation18
(18)
(18) ))
(21)
(21) Applying formula (Equation7
(7)
(7) ) to (Equation21
(18)
(18) ) yields
(22)
(22) for all
. Combining Equations (Equation20
(17)
(17) ) and (Equation22
(19)
(19) ) shows the validity of the inequality (Equation14
(14)
(14) ).
Theorem 3.1 provides an estimate between FTLE and a logarithmic quantity given in terms of the metabolic control matrix defined before. In fact, the scalar
(23)
(23) is the so-called relative instability exponent, which is introduced by Rateitschak and Wolkenhauer [Citation19] to measure the largest relative change in the system response when the initial conditions are perturbed.
4. Sigmoidal stimulus–response curves
The characterization of the input–output relation in biological networks is commonly done via the so-called stimulus–response curves, in which a scalar output is plotted against a scalar input of the network at a fixed time. In our mathematical framework, such curves can be defined as the family of functions
(24)
(24) where all components of
but
are assumed to be fixed.
In practice, stimulus–response curves are oftentimes strictly monotone (increasing or decreasing [Citation11,Citation16]), meaning that their first derivative with respect to the input does not change sign on suitably chosen intervals. Another common feature of stimulus–response curves is their sigmoidal form [Citation3], characterized by a bell-shaped first derivative, as will be made precise later. Rateitschak and Wolkenhauer [Citation19] took advantage of this geometrical attribute and defined a threshold value as the inflection point of a sigmoidal stimulus–response curve. These thresholds play an important role in biological applications, as they can be associated to an initial condition (e.g. concentration of a protein) activating a certain biological process. Mechanisms leading to sigmoidal (also referred to as ultrasensitive) stimulus–response curves include cooperativity, feed-forward loops and enzymes operating under saturation [Citation3]. Rateitschak and Wolkenhauer [Citation19] pointed out the importance of having quantitative measures that allow the detection of thresholds in a reliable manner. Their empirical study suggested that FTLE may be a suitable choice for such a measure, and in this section we will present a rigorous discussion regarding this conjecture.
In what follows, a stimulus–response curve , with
and
fixed, is said to be sigmoidal on a given interval
if there exist
such that
and
(25)
(25)
Sigmoidal curves, also referred to as S-shaped curves, are commonly used to describe growth patterns in ecological applications. In this context, the conditions shown above can be interpreted as follows. The first one means that the response curve is strictly increasing, that is, larger population densities lead to bigger growth rates. However, for low population densities the growth rate is assumed to increase slowly, which is characterized by the value L (typically small). Thus, according to the third condition in Equation (Equation25(22)
(22) ), for population densities in the interval
the growth rate does not exceed the boundary L. After this interval, there is an acceleration in the population growth, which can be observed in the interval
, where the growth rate becomes larger than L (see fourth condition in Equation (Equation25
(22)
(22) )). After this acceleration phase, the growth rate declines (below L), and the population usually approaches a saturation value. Below we present an example showing a stimulus–response curve with a sigmoidal shape, according to the conditions in Equation (Equation25
(22)
(22) ).
Example 4.1.
Consider again the planar system (Equation8(8)
(8) ). One stimulus–response curve associated to this system is given by (cf. Equation (Equation10
(10)
(10) ))
(26)
(26)
A straightforward computation shows that
is sigmoidal for T=1 and any fixed
, with a=0.02, b=1.70, L=0.5,
and
. In Figure the behaviour of the stimulus–response curve (Equation26
(23)
(23) ) and its first derivative with respect to the input
is displayed.
Similarly to the concept of stimulus–response curve introduced in Equation (Equation24(21)
(21) ), we will consider a family of scalar functions induced by the largest
FTLE (see Equation (Equation6
(6)
(6) )), defined as
(27)
(27) where all components of
but
are assumed to be fixed. In the remainder of this section we will establish a relation between extremal points of
, with
fixed, and inflection points (thresholds) of sigmoidal stimulus–response curves.
Figure 2. (a) Sigmoidal stimulus–response curve of system (Equation8(8)
(8) ) for
and T=1, on the interval
with L=0.5 and
. (b) First derivative of the stimulus–response curve with respect to the input
.
![Figure 2. (a) Sigmoidal stimulus–response curve of system (Equation8(8) x1′(t)=κx1(t),x2′(t)=g(x1(t))−x2(t),(8) ) for x2=10 and T=1, on the interval [0.02,1.70] with L=0.5 and (α,β)≈(0.1217,0.5852). (b) First derivative of the stimulus–response curve with respect to the input x1.](/cms/asset/969b01e1-9dc6-40e4-b83b-1de8f3cee933/tjbd_a_1204016_f0002_c.jpg)
Theorem 4.1.
Consider a stimulus–response curve with
and
fixed, sigmoidal on the interval
where α and β are as in Equation (Equation25
(22)
(22) ). Let
(28)
(28) If the estimate
(29)
(29) holds, then the maximum of
lies in
.
Proof.
Let us begin by recalling a basic fact regarding the spectral norm [Citation5, p. 72]
(30)
(30) for any
. According to Equation (Equation29
(26)
(26) ), M is the global maximum of
on
, and due to Equation (Equation25
(22)
(22) ), this extreme value must be attained at some
. By Equations (Equation5
(5)
(5) ), (Equation7
(7)
(7) ), (Equation24
(21)
(21) ) and (Equation30
(27)
(27) ) it follows that
(31)
(31)
Let us now consider any . Following a similar argument as before, combined with Equations (Equation28
(25)
(25) ) and (Equation29
(26)
(26) ), we have that
(32)
(32) Combining the inequalities (Equation31
(28)
(28) ) and (Equation32
(29)
(29) ) implies that for any
,
. Therefore, the global maximum of
must lie in
.
Note that in the conditions shown in Equation (Equation25(22)
(22) ), α, β and L need not be unique, while given L, the endpoints α, β are determined uniquely from
Equation (Equation25
(22)
(22) ) (see, e.g. Figure (b)). Therefore, the interval
can be chosen to be the smallest one satisfying the assumptions of Theorem 4.1, which allow us to conclude that the points at which the global maxima of
and
occur are close to each other. For the planar system (Equation8
(8)
(8) ) this is indeed the case, as can be seen from Figures (a) and (b). In fact, it can be shown that the inflection point of the stimulus–response curve (Equation26
(23)
(23) ) coincides with the extrema of the FTLE. The mathematical explanation for this will be given in the following theorem.
Theorem 4.2.
Assume the time-T map to have continuous partial derivatives up to order 3, for all
. Let
be a vector in
with
. Then the estimates
(33)
(33) hold, where
represents the standard scalar product in
.
Proof.
First, by differentiating Equation (Equation6(6)
(6) ) we obtain that
(34)
(34) Therefore, we need to get an estimate for the directional derivative on the right-hand side of the expression above. Choose
sufficiently close to zero. It follows for any
that (see Equations (Equation7
(7)
(7) ) and (Equation5
(5)
(5) ))
Dividing the last inequality by h and letting
yields
which combined with Equation (Equation34
(31)
(31) ) produces the estimate (Equation33
(30)
(30) ).
Note that if we consider system (Equation1(1)
(1) ) with d=1, the time-T transition matrix can be written explicitly as
Also, we have that
which implies
From this last equation and Equation (Equation5
(5)
(5) ), it follows that
holds for all
and
, which means that the estimate (Equation33
(30)
(30) ) is optimal for the one-dimensional case.
Example 4.2.
Let us apply Theorem 4.2 to the model (Equation8(8)
(8) ). Assume
to be fixed and take
. It follows from Equations (Equation10
(10)
(10) ), (Equation24
(21)
(21) ), (Equation27
(24)
(24) ) and (Equation33
(30)
(30) ) that
This means that every inflection point of the stimulus–response curve
is a critical point of the induced FTLE
. In our example, this point is located at
(see Figure ).
Example 4.3.
Rateitschak and Wolkenhauer [Citation19] were particularly interested in studying activation thresholds of gene transcription networks with cooperativity, which is a well-known mechanism that can lead to stimulus–response curves with sigmoidal shape [Citation3]. Specifically, they considered the following system that describes the temporal concentration changes of the components of a gene transcription network
(35)
(35) where K>0 and g is the Hill function (Equation9
(9)
(9) ). For the case K=10,
and
, the numerical results in [Citation19] suggest that the inflection point
of the stimulus–response curve
coincides with the maximum point
of the induced FTLE
, for T=2 and
. Our computations, however, show that there is a difference between these points, with an approximate relative error
which indicates that, in this case, the critical point
of the induced FTLE provides a good approximation of the inflection point of the stimulus–response curve. But this need not be always the case. If we consider system (Equation35
(32)
(32) ) for K=0.7,
, it can be shown that the sigmoidal stimulus–response curve
has an inflection point at
, while the closest critical point of the induced FTLE is located at
, for T=2,
and
. This results in an approximate relative error
which is significantly larger than in the previous case. In Figure we present some of the relevant curves associated to the case K=0.7,
.
Figure 3. (a) Sigmoidal stimulus–response curve of system (Equation35(32)
(32) ) (with K=0.7,
,
) for T=2,
and
. (b) First derivative of the stimulus–response curve with respect to the input
. An inflection point of
is detected at
. (c) Induced FTLE. (d) Enlargement of the boxed region shown in panel (c). The global maximum of
on the interval
is located at
.
![Figure 3. (a) Sigmoidal stimulus–response curve of system (Equation35(32) ηj(xj,T)=1TlnΨ(x1,…,xj,…,xd,T)2≤1Tlndmaxp,q=1,…,d(Ψ(x1,…,xj,…,xd,T))pq≤1Tln(dδ)<1Tln(M).(32) ) (with K=0.7, ηH=2, ρH=1) for T=2, x2=0.1 and x3=0.5. (b) First derivative of the stimulus–response curve with respect to the input x1. An inflection point of s31(⋅,T) is detected at xinf≈0.165071. (c) Induced FTLE. (d) Enlargement of the boxed region shown in panel (c). The global maximum of η1(⋅,T) on the interval [0,1] is located at xmax≈0.108876.](/cms/asset/bdc384fc-e912-4210-8b3c-c1962f183d3c/tjbd_a_1204016_f0003_c.jpg)
To conclude this section, let us briefly discuss the suitability of the MCC introduced in Equation (Equation12(12)
(12) ) to detect inflection points of stimulus–response curves. In [Citation19], the authors investigate numerically the possibility of detecting such inflection points via extrema of MCC. Let us then suppose that the stimulus response curve
has an inflection point at some
, for
and
fixed. From Equations (Equation12
(12)
(12) ) and (Equation24
(21)
(21) ), it follows that
Therefore, to detect the inflection point
of
via extremal points of MCC, one must have that
(36)
(36) a condition that is not always satisfied, as can be verified , for example, in Example 4.1.
5. Concluding remarks
The identification of thresholds plays a major role in biochemical networks, as they determine the activation sequence of intracellular signalling pathways. In many applications, the threshold can be defined in terms of a concentration of a protein triggering a certain biological process. In mathematical terms, this threshold can be identified as an inflection point of a stimulus–response curve, as defined in Section 4. In a previous work by Rateitschak and Wolkenhauer [Citation19], the detection of activation thresholds of sigmoidal stimulus–response curves via FTLE is studied in detail. The authors conjectured that FTLE provide an unambiguous and reliable way to identify the thresholds. Their conclusion, however, is based on numerical simulations of three examples, one of which has been revised in the present paper (Example 4.3). In this example, our computations showed that there is a small difference () between the extremal point of the largest FTLE and the inflection point of the stimulus–response curve considered. Despite of the difference, in this particular case one can conclude that the approximation is indeed reliable. Nevertheless, this need not be always the case, as was shown for the same system (Equation35
(32)
(32) ) with K=0.7 and
, for which the error was about 1359 times larger.
There are cases where an extremal point of the largest FTLE and the inflection point of a stimulus–response are exactly the same, see for instance Example 4.2. This can be explained in terms of Theorem 4.2, which gives an estimate for the modulus of the directional derivative of all FTLE. Therefore, the utilization of FTLE to identify activation thresholds has to be carried out with care, in view of the results shown in this paper. According to our study, all we can expect in general is that the extremal point of the largest FTLE and the inflection point of a stimulus–response curve lie in a certain interval , whose size will depend on how steep the stimulus–response curve is, see Theorem 4.1 and the remark thereafter. A closer look into this matter is given in the numerical experiment shown in Figure . In panel (a) we computed a family of sigmoidal stimulus–response curves of system (Equation35
(32)
(32) ) obtained by varying the parameter K. Here, we can see that the steepness of the stimulus–response curves increases as the parameter grows. Furthermore, we investigated how the difference between the inflection point
of the stimulus–response curve
and the maximum point
of the induced FTLE
varies with respect to K. The result is shown in Figure (b) in logarithmic scale. The almost straight line indicates that the difference
decreases as fast as
, with
. Therefore, the numerical observations confirm the relation between steepness of stimulus–response curves and approximation of their inflection points by maximum points of FTLE, as predicted by Theorem 4.1.
Figure 4. (a) Sigmoidal stimulus–response curves of system (Equation35(32)
(32) ) (with
,
) for T=2,
,
and
. The arrow indicates the direction of increasing K. (b) Difference between the inflection point
of the stimulus–response curve
and the maximum point
of the induced FTLE
, as a function of the parameter K.
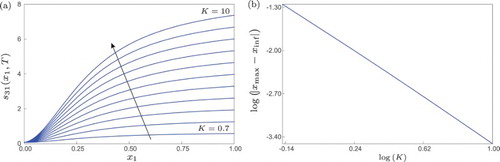
Another aspect analysed numerically by Rateitschak and Wolkenhauer [Citation19] is the detection of activation thresholds via extremal points of MCC. Their numerical observations indicate that MCC do not reliably detect all thresholds and suffer from false positives. In order to gain more insight into this matter, we tried to establish a relation between extremal points of MCC and inflection points of stimulus–response curves, and reached to the same conclusion, analytically shown in Equation (Equation36(33)
(33) ).
Acknowledgments
The authors would like to thank Dr K. Rateitschak for fruitful discussions.
Disclosure statement
No potential conflict of interest was reported by the authors.
Additional information
Funding
References
- B.B. Aldridge, G. Haller, P.K. Sorger, and D.A. Lauffenburger, Direct Lyapunov exponent analysis enables parametric study of transient signalling governing cell behaviour, Syst. Biol. 153(6) (2006), pp. 425–432. doi: 10.1049/ip-syb:20050065
- H.W. Bode, Network Analysis and Feedback Amplifier Design, D. Van Nostrand Co., Inc., New York, 1945.
- S. Choi (ed.), Introduction to Systems Biology, Humana Press, New Jersey, 2007.
- A. Goldbeter and D.E. Koshland, An amplified sensitivity arising from covalent modification in biological systems, Proc. Natl. Acad. Sci. USA 78(11) (1981), pp. 6840–6844. doi: 10.1073/pnas.78.11.6840
- G.H. Golub and C.F. Van Loan, Matrix Computations, 4th ed., The Johns Hopkins University Press, Baltimore, MD, 2013.
- S. Goutelle, M. Maurin, F. Rougier, X. Barbaut, L. Bourguignon, M. Ducher, and P. Maire, The Hill equation: A review of its capabilities in pharmacological modelling, Fund. Clin. Pharmacol. 22(6) (2008), pp. 633–648. doi: 10.1111/j.1472-8206.2008.00633.x
- G. Haller, Finding finite-time invariant manifolds in two-dimensional velocity fields, Chaos 10(1) (2000), pp. 99–108. doi: 10.1063/1.166479
- G. Haller, A variational theory of hyperbolic Lagrangian coherent structures, Phys. D 240(7) (2011), pp. 574–598. doi: 10.1016/j.physd.2010.11.010
- G. Haller and T. Sapsis, Lagrangian coherent structures and the smallest finite-time Lyapunov exponent, Chaos 21(2) (2011) (7 p.). doi: 10.1063/1.3579597
- R. Heinrich and T.A. Rapoport, Linear steady-state treatment of enzymatic chains. General properties, control and effector strength, Eur. J. Biochem. 42(1) (1974), pp. 89–95. doi: 10.1111/j.1432-1033.1974.tb03318.x
- R. Heinrich and S. Schuster, The Regulation of Cellular Systems, Chapman & Hall, New York, 1996.
- A.V. Hill, The possible effects of the aggregation of the molecules of haemoglobin on its dissociation curves, J. Physiol. 40 (1910), pp. 4–7.
- R.A. Horn and C.R. Johnson, Matrix Analysis, 2nd ed., Cambridge University Press, New York, 2013.
- B.P. Ingalls and H.M. Sauro, Sensitivity analysis of stoichiometric networks: An extension of metabolic control analysis to non-steady state trajectories, J. Theoret. Biol. 222(1) (2003), pp. 23–36. doi: 10.1016/S0022-5193(03)00011-0
- H. Kacser and J.A. Burns, The control of flux, Symp. Soc. Exp. Biol. 27 (1973), pp. 65–104.
- P. Lansky, O. Pokora, and J.-P. Rospars, Stimulus–Response Curves in Sensory Neurons: How to Find the Stimulus Measurable with the Highest Precision, Proceedings of the Second International Symposium on Brain, Vision and Artificial Intelligence, Naples, 2007, pp. 338–349.
- T. Millat, E. Bullinger, J. Rohwer, and O. Wolkenhauer, Approximations and their consequences for dynamic modelling of signal transduction pathways, Math. Biosci. 207(1) (2007), pp. 40–57. doi: 10.1016/j.mbs.2006.08.012
- M. Mohtashemi and R. Levins, Transient dynamics and early diagnostics in infectious disease, J. Math. Biol. 43(5) (2001), pp. 446–470. doi: 10.1007/s002850100103
- K. Rateitschak and O. Wolkenhauer, Thresholds in transient dynamics of signal transduction pathways, J. Theoret. Biol. 264(2) (2010), pp. 334–346. doi: 10.1016/j.jtbi.2010.02.001
- S.C. Shadden, F. Lekien, and J.E. Marsden, Definition and properties of Lagrangian coherent structures from finite-time Lyapunov exponents in two-dimensional aperiodic flows, Phys. D 212(3–4) (2005), pp. 271–304. doi: 10.1016/j.physd.2005.10.007
- A. Tiwari, J.C.J. Ray, J. Narula, and O.A. Igoshin, Bistable responses in bacterial genetic networks: Designs and dynamical consequences, Math. Biosci. 231(1) (2011), pp. 76–89. doi: 10.1016/j.mbs.2011.03.004