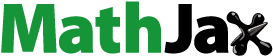
ABSTRACT
The study of the role of environmental variation in community dynamics has traditionally assumed that the environment is a stationary stochastic process or a periodic deterministic process. However, the physical environment in nature is nonstationary. Moreover, anthropogenically driven climate change provides a new challenge emphasizing a persistent but frequently ignored problem: how to make predictions about the dynamics of communities when the nonstationarity of the physical environment is recognized. Recent work is providing a path to conclusions with none of the traditional assumptions of environmental stationarity or periodicity. Traditional assumptions about convergence of long-term averages of functions of environmental states can be replaced by assumptions about temporal sums, allowing convergence and persistence of population processes to be demonstrated in general nonstationary environments. These tools are further developed and illustrated here with some simple models of nonstationary community dynamics, including the Beverton-Holt model, the threshold exponential and the lottery model.
Introduction
How should one realistically model the dynamics of populations and communities? There will never be agreement on the most important features of nature even when a common question or goal has been agreed upon. However, temporal change in the physical environment has long been recognized as a major driver of population change [Citation1]. Despite its major implications for the structure of populations and communities, it remains a highly specialized topic within theoretical ecology [Citation14]. Moreover, even within this specialized area, the physical environmental fluctuations considered in most models are distinctly lacking in realism. Most commonly, the environment is assumed to be a periodic deterministic process, really only suitable for the study of seasonal or diurnal variation [Citation34], or if modelled as a stochastic process, environmental fluctuations are assumed to be independent over time with an invariant distribution. Thus, in discrete time the environment is represented as a sequence of independent and identically distributed (i.i.d.) random variables, or in continuous time as a white noise process [Citation11].
Some studies have ventured beyond i.i.d. and white noise processes specifically to understand the effects of temporal autocorrelation on population and community dynamics [Citation26,Citation40,Citation41], but the most general environmental assumption commonly considered is stationarity, in other words, the environment is assumed to have long-term repeatability as exemplified by laws of large numbers: over any sufficiently long period of time, averages of environmental states settle down to constant values, and any period of time is as good as any other because they all have the same statistical properties. Periodic, i.i.d., and continuous-time white noise environments are special kinds of stationary environment. However, natural environmental variation does not have the long-term repeatability property characteristic of stationarity, except as a crude approximation [Citation29,Citation31].
The absence of environmental repeatability is a major challenge to science [Citation11]. The standard approach to studying communities in nature assumes that they are well defined entities with characteristics that are determined over a time span of years. Thus, they are assumed to be defined by an equilibrium, about which populations fluctuate, or alternatively, by a stationary distribution of population fluctuations, which is in essence an equilibrium of another sort [Citation11,Citation19,Citation38,Citation42]. Although few would deny that communities change on longer spans, such changes are generally treated as perturbations of equilibrium, or with environmental change, as changes in the equilibrium itself. The absence of environmental repeatability, however, calls into question the existence of the equilibrium in the first place [Citation42]. If the equilibrium concept is not applicable, what can be done? The theory of nonautonomous dynamics points the way by generalizing the concept of an attractor in dynamical systems theory to a nonautonomous attractor [Citation37,Citation46]. A related idea in theory of random dynamical systems is a random attractor [Citation3,Citation4,Citation7,Citation37]. These areas now include substantial applications in biology [Citation7,Citation8,Citation35,Citation36].
From these ideas comes the generalization of the concept of a stable equilibrium, the aedt, or an asymptotic environmentally determined trajectory [Citation11]. The aedt characterizes a system not as a point or a stationary probability distribution, but as preferred trajectory that a system is predicted to follow. This idea was introduced for ecological population and community models incorporating climate fluctuations perceived as nonstationary stochastic variation [Citation11]. Thus, this setting deviates from the emphasis on stationary variation in random dynamical systems theory. Moreover, it deviates from the emphasis in nonautonomous dynamics where strictly bounded solutions are sought because stochastic environmental variation will inevitably lead to violations of strict limits on the size of a population. Probabilistic limits apply, as in the stochastic boundedness concept [Citation9,Citation16,Citation17,Citation43], with the concern being bounding a population away from 0 as well as away from infinity.
This article advances study of the aedt including both its existence and its properties. The most important developments show how ideas used to study persistence of populations and communities in stationary environments can be generalized to the nonstationary case. These ideas concern growth of a population process from boundary states (where some species are extinct), which has a major role in both the deterministic permanence theory [Citation25,Citation30,Citation33], and stochastic persistence theory [Citation43]. Positive average growth rates are key to persistence conditions in these theories, but in a nonstationary environment are not in general well defined. Here it is shown that in nonstationary environments, convergence of sums of boundary growth rates to infinity has the potential to play a similar role to positive average boundary growth rates. Infinite boundary growth sums are shown to both guarantee convergence of the population process on an aedt, and to show that the aedt does not converge on boundary states. These developments apply to three simple models, but for general nonstationary environmental processes.
The models studied here are the Beverton-Holt model, the threshold exponential, and the lottery model. Both the Beverton-Holt model and the lottery model are classic models in population biology due to their simple illustrations of fundamental ideas. The Beverton-Holt model [Citation24] corresponds to a discrete-time evaluation of the continuous-time logistic model (the text-book example of density-dependent population dynamics), while the lottery model provides one of the simplest examples of how temporal environmental fluctuations can promote species coexistence [Citation18]. The Beverton-Holt model has been studied extensively in a variable environment but most commonly for the case of a periodic environment [Citation5]. Any serious population theory for nonstationary environments would need to cover this model, but it is also valuable due to the simplicity of its illustration of the aedt [Citation11]. For the lottery model, the key interest is how the introduction of nonstationarity alters the fundamental role of environmental fluctuations in species coexistence. The threshold exponential model, on the other hand, has not previously had a significant role in the development of ideas in population biology apart from its use in elementary extinction-time theory [Citation39]. In this article, its role is to provide a fundamental demonstration of how infinite limiting sums of growth rates can guarantee the existence of the aedt, and replace averages of growth rates in the demonstration of population persistence. Moreover, this model is shown to have an important role in bounding other population models in a nonstationary environment, allowing their properties to be better understood.
Preliminaries
A population process, {Nt}, represents the density (numbers per unit area) of a population or populations, as a function of time. This process is assumed to be driven by changing environmental conditions specified by an environmental process {Et}. In general, {Et} is assumed to be a stochastic process making {Nt} a stochastic process too. However, the development here focuses on one realization of the environment, and so proceeds deterministically, in effect conditioning on a specific {Et} outcome. Only occasional reference to a stochastic context is needed. The process {Nt} is thought of as starting at a time s, with t mostly meaning the current time. The variables u, v, and w are used for other times. The notation {Ns, t} is used when it is useful to emphasize the influence of the starting time, s, and expanded yet further to {Nn,s,t} to specifically indicate that the process starts at the value n at time s, with the variable Nn,s,t meaning the specific value of the process at time t given n and s.
Note that key concerns will be demonstrating that the value n and the starting time s do not greatly influence Nn,s,t if t – s is large in senses to be defined below. All the models discussed here use discrete time, but that is no necessity [Citation11]. Of most importance, when different trajectories of {Nt} are considered, they are all driven by the same fixed environmental sequence {Et}. These trajectories most commonly differ through differences in initial values and times, i.e. differences in n and s in the notation Nn,s,t. As a short hand, when two trajectories of the same process are being compared, one will be denoted Nt while the other will be denoted . It will be assumed here also that Nt is a state variable of the system in the sense that if
for any t, then the trajectories remain equal for all subsequent times.
In the simplest form, a discrete-time process satisfying the conditions specified here is expressible as
(1)
(1) where
indicates the Hadamard or element by element product (
), and the function λ of Nt and Et is the vector of ‘finite rates of increase’ of the m species, following traditional ecological terminology. There is no immigration in this model. Thus, the system is internally sustaining. The simplest illustration of the ideas to be investigated here comes from the single-species Beverton-Holt model [Citation5,Citation11,Citation17,Citation20–22]. In this model, the environmental parameters Et = (Rt, αt), are respectively the maximium finite rate of increase and the intraspecific competition coefficient, both of which are positive and fluctuate over time. The model is then defined by the formula for λt,
(2)
(2)
The Beverton-Holt model conveniently yields an explicit solution. The transformation yt = 1/Nt renders the iteration (1) linear [Citation17], which is then easily solved to give [Citation11]
(3)
(3) The most important issue is the division of this expression into dependence on the initial state, and independence of it. The degree of dependence overall on the initial state, ys, comes from
(4)
(4) Note that a viable population must have a preponderance of R values greater than 1, as otherwise λ would be mostly < 1 and the population would go extinct. By restricting consideration to viable populations, we are dealing with situations in which expression (4) would be expected to approach 0 as either t → ∞ or s → − ∞. In either case, the initial state dependence in equation (3) vanishes. If we stipulate that (4) vanishes as t → ∞, the concept of forward convergence is illustrated: any two trajectories, defined by their initial states ys, converge on each another other as t increases. Thus, asymptotically, there is but a single trajectory going into the future, the aedt, i.e. the asymptotic environmentally determined trajectory. On the other hand, if we stipulate that (4) vanishes as s → − ∞, we see that any two trajectories starting at the same time, but at different values, y’ and y’’, say, will become asymptotically the same as this common starting time vanishes into the distant past (yy’,s,t – yy”,s,t → 0 as s → – ∞). In general, that does not mean that asymptotically there is just one trajectory because yt (equivalently Nt) might not actually converge as s → − ∞ even though different trajectories converge on each other. However, in the Beverton-Holt model, yt (and Nt) do converge provided only that expression (4) vanishes as s → − ∞, and the result is
(5)
(5) The justification of this assertion is that the series in [] in expression (5), whose partial sum appears on the RHS of expression (3), has nonnegative terms, and therefore converges to a finite positive value or + ∞. Thus, vanishing of (4) as s → − ∞ is sufficient for convergence of expression (3) as s → − ∞. If the series in expression (5) is + ∞, then
is uniformly 0, but is nevertheless the unique aedt achieved as s → −∞. Formally, the aedt can be defined as follows:
Definition 1:
Given a population process, {Nt} an aedt is a population trajectory on which all other trajectories converge in one of the following two senses:
Forward convergence:
, as t → ∞, whenever Ns > 0 for a fixed starting time s.
Backward convergence:
, as s → – ∞, for all t, for every fixed Ns > 0.
Although the aedt in the definition of backward convergence is unique, this is not the case for the forward aedt. In general, it is only asymptotically unique in the sense that if and
are both forward aedts fitting definition 1 for the same starting time s, then
as t → ∞. Under the definition, the existence of a forward aedt from a specific time s implies that any trajectory {Nn,s,t} having a positive value at time s is in fact an aedt, and the difference between it and any other trajectory {Nn’,s,t} satisfying the same initial conditions must vanish as t increases (Nn’,s,t − Nn,s,t → 0 as t → ∞). However, in the backward case, in general it is possible to have two trajectories converge on each other as the starting time, s, recedes into the past (Nn’,s,t − Nn,s,t → 0, as s → – ∞) without either trajectory actually converging, i.e. without there being a backward aedt. In these cases, the actual starting time, s, influences the values of the trajectory at fixed later times no matter how distant in time that start is, even though the effect of the starting value, Ns, itself has no influence asymptotically on Nt (Nn’,s,t − Nn,s,t → 0, as s → – ∞). Note also, that in the case of forward convergence, if
, as t → ∞, for a particular s, for all Ns > 0, it must apply for all earlier s provided the λ’s are strictly positive because then s’ < s, and Ns’ > 0 implies Ns > 0, and hence that the statement for the particular s extends also for all earlier s. The statement would extend to later s under the condition that for s’ > s and Ns’ > 0 there is a trajectory with Ns > 0 leading to Ns’.
Having determined that a process converges on an aedt, we know that it can be characterized independently of arbitrary features, such as its starting time and starting value. The question then is what the aedt is like. Of foremost importance is whether it guarantees population persistence [Citation11,Citation43]. One way of demonstrating this outcome is by bounding it by a simpler process whose persistence is easily demonstrated. One such process is the threshold exponential.
The threshold model
The threshold exponential model [Citation39] is a single-species model defined in discrete-time by the formula
(6)
(6) In simple terms, the process satisfies the difference equation Nt +1 = RtNt until it reaches the value K, which it is prevented from exceeding. Here, Et = Rt, and in a constant environment (Rt ≡ R), this model has very simple behaviour. It simply increases to K (provided R > 1), and stays there. The variable environment case is best understood by defining
(7)
(7) notation that we apply to all models, with rt referred to as the growth rate or more correctly, the per capita growth rate [Citation12]. It means
(8)
(8) Also, in general we can define,
(9)
(9) Note that in both the threshold exponential and in the Beverton-Holt model,
.
In a stationary environment, the persistence of a process is commonly investigated by studying
(10)
(10) in circumstances under which is it time invariant due to joint stationarity of the environment and population variables on which it may depend [Citation43]. For example, for the two models considered so far,
is simply a function of the environment, and would have a time invariant mean in a stationary environment. Positive values of the quantity (10), and its generalizations to the multispecies case, are used to show that the population densities have a stationary distribution in which all species have positive densities and therefore persist in the sense of stochastic boundedness [Citation16,Citation17,Citation43]. Instead, in the nonstationary case, we put conditions on the sum
(11)
(11) Naturally, there is a forward sum condition
(12)
(12) and a backward sum condition
(13)
(13) referred to here as forward and backward ‘infinite sum conditions.’ For the Beverton-Holt model, these are the conditions for expression (4) to vanish respectively in the forward and backward senses, and hence for forward and backward aedts to exist. For the threshold exponential, the implications of these conditions are just a little stronger, as shown in the appendix:
Theorem 1:
Under the forward infinite sum condition (12), the threshold exponential converges on an aedt, , in the forward sense. Moreover, for any initial time s and any positive
, there is a time τ such that t ≥ τ implies
whenever n and n’ ≥ ϵ.
As stated in the theorem, the threshold exponential has the unusual feature that any two positive trajectories become exactly equal after a finite amount of time when the forward infinite sum condition applies. This outcome occurs because the monotonicity of the threshold exponential means that no two trajectories can cross, and when the lower trajectory hits K, the upper trajectory must hit K too, making them equal from that time on. A corresponding property holds for backward convergence when the backward infinite sum condition holds:
Theorem 2:
Under the backward infinite sum condition (13), the threshold exponential is backward convergent on a unique aedt, . Moreover, for any positive ϵ < K, and any time w, there is a time v < w, such that s ≤ v and Ns ≥ ϵ implies
for all t ≥ w.
The trajectories of the threshold exponential satisfying the forward infinite sum condition have the important property that every trajectory takes the value K infinitely often into the future. Thus, the process cannot converge to 0, demonstrating a certain degree of persistence of the population. A trajectory of the threshold exponential cannot necessarily be continued indefinitely into the past. For example, if Rs−1 < 1 but Ns = K, there is no possible value of Ns−1 satisfying the equation for the dynamics of the system. However, the backward aedt is a complete trajectory, i.e. continues indefinitely into the past. It is the unique trajectory that reaches K infinitely often into the past, i.e. in the set of times { … , s −2, s −1} (Appendix). However, there are infinitely many trajectories with the property lim t →−∞ Nt = 0 when the backward the infinite sum condition is satisfied (Appendix) making the state N = 0 a repeller.
The threshold exponential model is a relatively crude model of density-dependent dynamics, but is valuable for several reasons. First, it simply illustrates the key convergence concepts. Second, by showing that K is reached infinitely often, it illustrates a concept of population persistence, although a relatively weak one, because unlike the stochastic boundedness condition, a population might nevertheless spend ever larger times ever closer to 0 into the future or into the past. Third, the threshold exponential can be used to provide bounds on other more complex models, facilitating their analysis. This third use depends on the following result whose proof is sketched in the appendix.
Lemma 1:
Let Nt and Mt be two population processes satisfying the iterations
with the common environment process Et. If either F or H is monontone nondecreasing in its first argument then, Ns ≥ Ms and F(L, Et) ≥ H(L, Et), for all L and t, implies that Nt ≥ Mt for all t > s.
This lemma can now be applied to the Beverton-Holt model. The Beverton-Holt model cannot dominate the threshold exponential in complete generality, however. To illustrate one such case when it does, assume that the ratio Rt /αt = σ, fixed. Then define the dominated threshold exponential R parameter as , where Rt is for the dominating Beverton-Holt, for some K less than σ. It then follows that for a given Nt and parameter values, the Beverton-Holt λt is never less than the threshold exponential λt (see the appendix). As both model iterations are monotone nondecreasing in population density, they satisfy Lemma 1. Defining
, the behaviour of the Beverton-Holt is constrained by the behaviour of the sum
(14)
(14) where ϵ = – ln(1 − K/σ), and
is the low density growth rate (9) for the Beverton-Holt model. If the sum (14) converges to ∞ in the forward or backward direction, we know that the process must reach at least K infinitely often in the forward or backward direction respectively. In particular, in the backward direction, convergence of the process on a positive aedt is then guaranteed, while the infinite sum conditions (12) and (13) alone allow the possibility that the backward aedt is uniformly 0, and the forward aedt converges on 0 as t → ∞. Requiring expression (14) to converge to ∞, however, is much stricter than the infinite sum conditions (12) and (13) because it requires that the sums of the
must not only converge to ∞, but converge to ∞ at a rate asymptotically exceeding a linear function. Chesson [Citation11] provides some less strict alternative convergence conditions by very different methods.
The lottery model
The examples so far are of relatively simple single-species models. The lottery model, however, is a multispecies model in general, and its dynamics in a variable environment are of much interest because in the lottery model, the variable environment is a mechanism of species coexistence, i.e. the variable environment is necessary for the persistence of multiple species. This fact has been well-studied for the case of i.i.d environments [Citation10,Citation16,Citation27,Citation28,Citation43], with some extensions to more general stationary cases [Citation13,Citation18]. Moreover, there is considerable interest in applying it and related models to species coexistence in nature [Citation2,Citation6,Citation14,Citation45], including the potential that it may help explain the classic latitudinal species diversity gradient for forest trees [Citation44]. Thus, when and whether nonstationary environment fluctuations can be a mechanism of species coexistence is of great interest, which we investigate here in the nonstationary lottery model.
The lottery model can be defined by
(15)
(15) The subscripts i and j label the species. The adult death rates, δ, are all assumed to be positive and less than 1. Thus, individuals live more than 1 time period, but are not immortal. The environment process is Et = (B1,t, … , Bn,t), the vector of per capita births for a unit of time, for each species.
As the species’ densities add to a constant in this model, which can be conveniently set to 1, it becomes one-dimensional in the two-species case, and the development here considers just this one-dimensional case. However, extensive treatments exist for the full n species model in the stationary case [Citation10,Citation27]. In the two-species case, the model is most conveniently studied on the log-odds scale:
(16)
(16) This one-dimensional model is monotonic, i.e. Ni, t +1 is a strictly increasing function of Ni, t, and therefore trajectories that have different starting values cannot cross. Moreover, with the sum of the densities set to 1, inspection of equation (Equation15
(15)
(15) ) shows that a species density, Ni,t, cannot decrease more than δi in one time period, or increase by more than the maximum of the δs of all species. In particular, this means that if we choose a constant c satisfying
(17)
(17) then Ni,t cannot cross the interval [c, 1 − c] without entering it. Further, if
and
, then [c, 1 − c] must be entered infinitely often by Ni,t as t → ∞, a critical feature for analyzing the model.
To study the difference between trajectories with different initial values, use for the higher trajectory and Zt for the lower trajectory, with
, and
. In this two-species case, Nt will be used for
. Because the lottery iteration (15) is strictly increasing in Nt, the signs of ΔZt and ΔNt are always positive. For simplicity, only the case of equal δs is studied for which it can be shown (Appendix) that,
(18)
(18) where ρt = B1, t /B2, t, and
(19)
(19) with ‘
‘ meaning maximum. Because 0 < δ < 1, and
, except for ρt = 1 when it is 0, inequality (18) shows that the trajectories on the log-odds scale must come closer together as time progresses. Note that when ρt > 1, species 1 increases relative to species 2 (Zt+1 − Zt > 0), and when ρt < 1, species 2 increases relative to species 1 (Zt+1 − Zt < 0). The condition for ΔZt to change, and it can never increase with time, is simply ρt ≠ 1 (inequality (18)). However, two issues limit the convergence of the trajectories, and these are the magnitudes of the deviations of the ρt from 1, and the magnitudes of the Nt. Although ΔZt is nonincreasing over time, ΔNt can fluctuate. For instance if Nt approaches 0 or 1, then ΔNt must approach 0 even if ΔZt remains bounded above a positive value due to the nonlinear scale difference between Nt and Zt, as shown by the formula
(20)
(20) (Appendix). It follows that the RHS of (18) can be negligible if Nt takes an extreme value. To ensure convergence of ΔZt to zero, the values of both ρt and Nt must be constrained. To see how this works, note first of all that expression (18) implies
(21)
(21) Defining A to be the set of times
(22)
(22) where ϵ is some positive number, and s and t are arbitrary except for the restriction s < t. Then (21) implies that
(23)
(23) because the terms of the sum in (21) are all nonnegative. Defining #A to be the number of elements in A, ΔNmin to be the minimum value of ΔNu on A, and noting that ΔZt > 0 for all finite t, expression (23) implies that
(24)
(24) Thus, if #A → ∞, ΔNmin must converge to 0 for fixed ΔZs. Here, changes in the set A can be due to changing s, changing t or both. The appendix now shows from expression (20) that
(25)
(25) As the RHS of inequality (25) is strictly increasing in ΔZt, #A → ∞ implies that ΔZt → 0.
In the appendix of Chesson [Citation11] it is remarked, but not proved, that if is constrained above a fixed positive value, and Nt enters some closed interval [c, 1 − c] ⊂ (0, 1) infinitely often for t ∈ {s, s + 1, … }, then ΔZt must converge to 0, as t → ∞. The results here validate this claim. If there is no such interval [c, 1 − c] that Nt enters infinitely often, then Nt must converge either on 0 or on 1 because condition (17) precludes
and
from both being true. Convergence of Nt on 0 or 1 implies that ΔNt converges to 0 because ΔZt is nonincreasing by inequality (18), and equation (20) then implies that ΔNt approaches 0 as Nt approaches on 0 or 1. Thus, forward convergence of trajectories occurs on the scale of Nt, but not necessarily on the Zt scale, simply from the requirement that
has a positive infinum. However, it would be desirable to remove this restriction, demonstrate convergence on the Z scale, and persistence of the species in some suitable sense. These goals can be achieved by specifying appropriate infinite sum conditions on the growth rates and a suitable probability space on which the process Nt is defined as a stochastic process. The case of an i.i.d. environment, {Et}, has been thoroughly investigated [Citation16,Citation23,Citation43] but the goal of this manuscript is the nonstationary case.
Infinite sum conditions for the lottery model
A number of different conditions can be placed on lottery model growth rates for convergence results. First note that for the two-species lottery model with equal δs, the λs can be written as functions of Nt as follows,
(26)
(26) where it should be recalled that
and
. Thus, the rate of change of Zt can now be written as
(27)
(27) This function is monotone decreasing in Nt as is shown in the derivation of expression (18) in the appendix. Thus, it has the important property that r1,t(Nt) ≥ r1,t(c) whenever Nt ≤ c. From the perspective of species 2, r2,t(1−Nt) is defined to be − r1,t(Nt). Here we define the critical sums,
(28)
(28) These sums define lower bounds on the cumulative change in the density of each species on the log-odds scale whenever the density of the species in question is ≤ c.
The forward infinite sum conditions are
(29)
(29) Note that because ri,t(c) is a decreasing function of c, whenever either condition (28) holds for one value of c it holds also for all smaller positive values of c. If the forward infinite sum conditions are in force, Nt enters the interval [c, 1 − c] infinitely often for c < (1 − δ)/2. This result can be understood from the fact that whenever species 1 falls below c, its growth on the Z scale is at least r1,t (c). The infinite sum condition then implies that over a finite interval of time it must increase to reach c again. The first infinite sum condition implies this process would repeat indefinitely, and hence that N1, t ≥ c for infinitely many t > s. The second infinite sum condition implies the same for N2,t . From the fact that Nt cannot jump from below c to above 1 − c without entering [c, 1 − c], we see Nt ∈ [c, 1 − c] for infinitely many t > s. This result is stated formally as the lemma
Lemma 2:
If conditions (29) apply with c < (1 − δ)/2, the two species lottery model with equal δ values has the property
If we also impose the condition, m(ρt) ≥ ϵ > 0, then we see that forward convergence must occur. However, this condition is stricter than desirable, and it is relaxed in the next section.
Nonstationary environment assumptions
Assume that {ρt} is a stochastic process on a probability space . Assume that the infinite sum conditions (29) hold with probability 1. Treat the initial state, Ns, as nonrandom, and define
be the σ-algebra induced by the random variables ρs, ρs+1, … , ρt, i.e. conditioning on
(history up to time t) means conditioning on ρs, ρs+1, … , ρt. Note that
is a nested increasing sequence of σ-algebras, and so is ‘filtration’ in the terminology of probability theory [Citation32]. For completeness, define
, i.e. the empty set and the whole space. However, the results here would still apply with the history included in the
extending back to −∞. We can state the condition needed to obtain forward convergence with probability 1:
Assume that there exist positive ϵ and p such that for all t ≥ s
(30)
(30) This condition relaxes the requirement that m(ρt) is always greater than ϵ with the requirement that there is some minimum probability that it will be regardless of past events. Under the infinite sum conditions (29), there is a sequence of times {μn} for which Nt ∈ [c, 1 − c] starting with s and μ0 = s for Ns ∈ [c, 1 − c]. The times μn – 1 are optional times with respect to the filtration
as defined in probability theory [Citation32] because
. (The displacement by one unit of time in these relationships occurs because events defined by the sequence Ns, Ns+1, … , Nt belong to
, Nt being a function of ρt−1 and Nt−1.) Let
be the σ-algebra for events concerning
, i.e. these are the events up to the optional time μn – 1 [Citation32], and are defined formally as
. With this definition,
also means that the event
[Citation32]. Together with (30), this finding means
Lemma 3:
(31)
(31) From this result and the extended Borel–Cantelli lemma (e.g. page 108 of [Citation32]), we see that
for infinitely many n with probability 1, i.e. Nt ∈ [c, 1 − c] for infinitely many times t when
. Thus, the number of elements in the set A defined by (22) convergences on ∞ as t increases, with probability 1. It follows that ΔZt converges to 0 as t → ∞ with probability one, for any pair of starting values of Ns ∈ [c, 1 − c]. In other words the two-species lottery model is forward convergent on an aedt with probability one. This result generalizes simply to any pair of starting values ∈ (0,1) because if the infinite sum conditions hold for one value of c, they hold for all smaller positive values of c. Thus, c can always be chosen to encompass any desired initial states. These considerations lead to the following theorem, for which a more systematic demonstration can be found in the appendix.
Theorem 3:
If the infinite sum conditions (29) hold with probability 1, for some positive c < (1 − δ)/2 and condition (30) applies, then ΔZt converges to 0 as t → ∞ with probability 1 for any fixed s, and any fixed values of Ns and .
Backward convergence in the lottery model
For backward convergence, we need the backward infinite sum conditions
(32)
(32) Once again, because ri,t(c) is a decreasing function of c, whenever either condition (32) holds for one value of c it holds also for all smaller positive values of c. Proving backward convergence in the lottery model is somewhat more difficult than forward, and so for simplicity, we revert to the case where m(ρt) has a positive infimum. The following result is proved in the appendix.
Theorem 4:
Under the infinite sum conditions (32) for some positive c < (1 − δ)/2, and the additional hypothesis that m(ρτ) ≥ ϵ > 0 for all τ < t for some positive ϵ, then
There exists a strictly decreasing sequence of integers {vn} ≤ t with the property s ≤ vn+1 and Ns ∈ [c, 1 − c] implies Nu ∈ [c, 1 − c] for at least one u ∈ {vn+1 + 1, … , vn}.
ΔZt → 0 as s → −∞, for all pairs
and Ns of starting values ∈ (0, 1).
This theorem proves convergence of trajectories on each other, but does not prove the existence of an aedt in the backward sense. It thus leaves open the possibility that Nt continues to vary without convergence as s is decreased for fixed values of Ns. Thus, even though the actual value of Ns does not matter, i.e. Nn’,s,t – Nn,s,t → 0 as s → – ∞, the starting time s does matter, i.e. Nn,s,t does not approach a limit as s decreases towards – ∞. Nevertheless, it is possible to constrain the possible behaviour of the trajectories by comparison with the threshold exponential.
Threshold exponential bounds on the lottery model
The threshold exponential with K = c < δ, and
(33)
(33) bounds the species 1 lottery iteration from below, as shown in the appendix, and the same formula but with (ρt)−1 replacing ρt gives the corresponding Rt = R2, t for the threshold exponential lower bound for species 2. Thus, ri, t = ln Ri, t gives the infinite sum conditions for bounding each Ni, t by a threshold exponential that hits the value c infinitely often,
(34)
(34) The forward direction is straightforward. With the conditions applying for t → ∞, bounding of both species by a threshold exponential ensures that each exceeds c infinitely often, and if c < (1 − c)/2, we see again that Nt enters the interval [c, 1 − c] infinitely often, with forward convergence implications as discussed above. Of more interest is the backward direction. Then we can define liminf and limsup bounds on the lottery model in the backward direction as follows
(35)
(35)
(36)
(36) Here, n is any fixed number between 0 and 1. Under the conditions of Theorem 4, the value of n has no effect on the results, but if conditions of Theorem 4, do not apply, (35) and (36) can be redefined as respectively the supremum and infimum over n. First note that if
is the aedt of the threshold exponential bounding the lottery Ni, t, then
(37)
(37) To see this, note that we can choose m ≤ n, and then Mm,s,t ≤ Nn,s,t, and on taking limits in s, the lower bound in (37) is validated, with the upper bound validated in the same way. Of most importance, continuity and monotonicity of the lottery iteration imply that
and
both satisfy the lottery iteration and indeed provide complete solutions of the lottery model. They have the property induced by the bounding threshold exponentials of not converging on 0 or 1, and thus providing a measure of persistence. When they are the same, they define a unique aedt in the backward sense. It is not clear how generally equality of
and
occurs in a nonstationary environment. However, in the case of a stationary environment, invariance of the statistical properties of the environment process with time shifts imply the same for (
) jointly as a bivariate stochastic process. Thus, like its environment process Et, (
) is itself a stationary stochastic process. In particular, this means that the corresponding log-odds scale processes,
and
, cannot move apart as t decreases as the inequality (18) requires when they differ. Thus, they are identical, implying that lims → −∞ Nt exists, is the unique aedt, and is a stationary stochastic process supported on (0,1). This result extends and complements previous results for the case of an i.i.d. environment process [Citation16,Citation43]. These results are summarized as follows.
Theorem 5:
Under the infinite sum conditions (34) for s → – ∞, the processes and
are trajectories of the lottery model with the properties
and
,
In the case that the environment is a stationary stochastic process, with probability 1,
, the unique backwards aedt, and
is a stationary stochastic process.
Discussion
The concept of the aedt reflects a common type of generalization in mathematics where a Euclidean point is generalized to a function. What does it mean here for the application? Is asks ecologists to conceptualize populations and communities not in terms of an equilibrium determined by average environmental conditions, but as a trajectory determined by ever-changing environmental conditions, with those changing conditions having a critical role. In this way, it can shift the focus to the roles that those changing conditions have, and therefore be conceptually useful in conservation and management, especially in the face of anthropogenically-driven climate change.
From the point of view of ecological theory, it demands a search for concepts and new tools of analysis. Yet, as shown here through the use of conditions on sums rather than on averages, traditional methods can point in the direction of new methods once the nature of the generalization is understood. Although these methods have been illustrated here with very simple models that are one-dimensional and monotonic, strong results are nevertheless provided for environmental sequences of essentially arbitrary character. This suggests the potential for more fundamental understanding by identifying those characteristics of an environmental sequence truly needed for outcomes like convergence and persistence not so evident when the environment is simply assumed to be i.i.d., periodic or constant. Naturally, using the methods discussed here is much more involved than traditional methods, but at the same time they lead in fundamentally new more realistic directions, with exciting potential for further development of ecological theory rising to the long-standing challenge of the ever-changing nature of the physical environment.
Forward convergence is in general much more straightforward than backward convergence, as shown here especially for the lottery model. In many cases, it will be sufficient for applications where the focus on the future of a process is adequate. Although it has the less satisfactory property that the aedt in that sense is only asymptotically unique in general, asymptotics and approximations are facts of science that make many otherwise intractable problems solvable. Establishing that an aedt exists in the backward sense is intellectually satisfying due to the uniqueness, and the information it gives on the role of the past, although its use in applications is potentially less important. From a mathematical perspective, it offers rich possibilities for investigation, which I hope the developments here illustrate, but if not, there is no doubt about this in previous work on nonautonomous dynamical systems [Citation37]. This mathematical interest promises great potential for enriching theoretical ecology.
Of the specific models studied here, the lottery model is perhaps of most interest due to its application to understanding how species coexistence occurs in a variable environment [Citation18], and recent claims that the mechanism of species coexistence that it illustrates contributes to the latitudinal gradient in tree species diversity [Citation44]. For forward convergence, there are few restrictions on the nature of the environmental variation. For the infinite sum conditions to be satisfied it is necessary for each species to be favoured over the other every so often, i.e. sometimes ρt must be greater than 1, and sometimes less than 1. Simply requiring both and
is sufficient for the forward infinite sum conditions (29) and (34) to be satisfied, for some c > 0, and is also compatible with the condition (30), which ensures that the amount of change taking place does not converge to zero with time. This last condition also precludes the possibility that limt → ∞ ρt = 1. These restrictions allow wide variation in environmental conditions, with little resemblance to stationarity. For example, the amount of change per unit time might slow down greatly, essentially attenuating the timescale, with ρt and
less and less often jumping much above 1. In principle, it is also possible to have ever longer periods of time favouring one species relative to the other. However, such conditions would lead to population sizes undergoing large swings also, as illustrated in Chesson and Huntly [Citation13], which do not correspond to realistic coexistence conditions in nature even though according to the results here neither species would converge on 0.
For convergence of any two trajectories in the backward sense in the lottery model, the conditions as developed here are more restrictive than for forward convergence because as yet there is no corresponding stochastic development. Hence a strict bound on the closeness of ρ to 1 was applied instead to ensure that enough change over time occurs. Otherwise, however, the restrictions on the nature of environmental change in the backward direction are not much different from the forward direction, allowing wide variation from stationarity. However, it has not yet been possible to prove the existence of backward aedt under general circumstances.
The infinite sum conditions fundamentally mean that when a species approaches low density it has a long-term net tendency to recover, just as positive average low-density growth rates imply for models in stationary environments. However, in all examples considered here, infinite sum conditions have served two different roles. The first of these, which has occupied most attention, is convergence of trajectories on each other and on the aedt. The second is preventing the trajectories from converging on 0. These two, however, are closely related. This fact is perhaps most obvious for the lottery model where entering the interval [c, 1 − c] infinitely often, which simply requires that neither species converge on 0, was used in the proofs of trajectory convergence. In essence population trajectories are brought together by the negative density dependence of population change (Zt+1 − Zt is a decreasing function of Nt whenever it is not zero), which is strongest in the midrange of abundances defined by [c, 1 − c]. The threshold exponential illustrates the phenomenon in extreme form because when a trajectory reaches the upper density limit, K, where it is driven by the infinite sum conditions, it merges with all higher trajectories, giving convergence in finite time.
This paper is but a sample of the possible relevant ecological considerations of nonstationary community theory. Chesson [Citation11] goes further in this regard, in particular to consider application of the stronger persistence concept, stochastic boundedness [Citation9], and the nature of the nonstationary distribution of the process {Nt}. Here only a weak persistence idea is studied, namely nonconvergence of Nt on 0 as t → ∞ or −∞, although the approach here does go a little further by identifying particular positive values that Nt must exceed infinitely often. Nevertheless, stochastic persistence in a nonstationary environment is another example of where previous ideas and results [Citation17,Citation43] offer intellectual fodder for generalization, with major implications for nonstationary community theory.
Acknowledgements
I am grateful for comments on these developments prior to writing the manuscript from Xiaoying Han. This work was supported by NSF grant DEB-1119784.
Disclosure statement
No potential conflict of interest was reported by the author.
ORCID
Peter Chesson http://orcid.org/0000-0001-8931-8012
Additional information
Funding
References
- H.G. Andrewartha and L.C. Birch (ed.), The Distribution and Abundance of Animals, University of Chicago Press, Chicago, 1954.
- A.L. Angert, T.E. Huxman, P. Chesson, and D.L. Venable, Functional tradeoffs determine species coexistence via the storage effect, Proc Natl Acad Sci U S A 106 (2009), pp. 11641–11645. Available at<Go to ISI>://000267972700040. doi: 10.1073/pnas.0904512106
- L. Arnold (ed.), Random Dynamical Systems, Springer-Verlag, Berlin, 1998.
- R. Bhattacharya and M. Majumdar (ed.), Random Dynamical Systems: Theory and Applications, Cambridge University Press, New York, 2007.
- M. Bohner and H. Warth, The Beverton–Holt dynamic equation, Appl Anal 86 (2007), pp. 1007–1015. doi: 10.1080/00036810701474140
- C.E. Caceres, Temporal variation, dormancy, and coexistence: a field test of the storage effect, Proc Natl Acad Sci U S A 94 (1997), pp. 9171–9175. doi: 10.1073/pnas.94.17.9171
- T. Caraballo and X. Han (ed.), Applied Nonautonomous and Random Dynamical Systems, Springer Nature, Cham, Switzerland, 2016.
- T. Caraballo, X. Han, P. Kloeden, A. Rapaport, Dynamics of nonautonomous chemostat models, in Continuous and Distributed Systems II, Studies in Systems, Decision and Control 30, Sadovnichiy V.A., Zgurovsky M.Z., eds., Springer International Publishing, Cham, Switzerland, 2015. pp. 105–120.
- P. Chesson, Predator-prey theory and variability, Annu Rev Ecol Syst 9 (1978), pp. 323–347. Available at http://search.netscape.com/ns/boomframe.jsp?query=Annual+Review+of+Ecological+Systems&page=1&offset=0&result_url=redir%3Fsrc%3Dwebsearch%26req-uestId%3Dd527ebaf778035b3%26clickedItemRank%3D1%26userQuery%3DAnnual%2BRe-view%2Bof%2BEcological%2BSystems%26clickedItemURN%3Dhttp%253A%252F%252Fwww.jstor.org%252Fjournals%252F00664162.html%26invocationType%3D-%26fromPage%3DNSBoom%26amp%3BampTest%3D1&remove_url=http%3A%2F%2Fwww.jstor.org%2Fjournals%2F00664162.html. doi: 10.1146/annurev.es.09.110178.001543
- P. Chesson, Multispecies competition in variable environments, Theor Popul Biol 45 (1994), pp. 227–276. doi: 10.1006/tpbi.1994.1013
- P. Chesson, AEDT: A new concept for ecological dynamics in the ever-changing world, PLoS Biol 15 (2017), pp. e2002634. Available at<Go to ISI>://WOS:000404510400022. doi: 10.1371/journal.pbio.2002634
- P. Chesson, Updates on mechanisms of maintenance of species diversity. J Ecol 106 (2018), pp. 1773–1794. doi: 10.1111/1365-2745.13035
- P. Chesson and N. Huntly, Temporal hierarchies of variation and the maintenance of diversity, Plant Species Biol 8 (1993), pp. 195–206. doi: 10.1111/j.1442-1984.1993.tb00070.x
- P. Chesson, N.J. Huntly, S.H. Roxburgh, M. Pantastico-Caldas, and J.M. Facelli, The storage effect: definition and tests in two plant communities, in Temporal Dynamics and Ecological Process, Kelly C.K., Bowler M.G., Fox G.A., eds., Cambridge University Press, Cambridge, 2013. pp. 11–40
- P.L. Chesson, The stabilizing effect of a random environment, J Math Biol 15 (1982), pp. 1–36. doi: 10.1007/BF00275786
- P.L. Chesson and S. Ellner, Invasibility and stochastic boundedness in monotonic competition models, J Math Biol 27 (1989), pp. 117–138. doi: 10.1007/BF00276099
- P.L. Chesson and R.R. Warner, Environmental variability promotes coexistence in lottery competitive systems, Am Nat 117 (1981), pp. 923–943. doi: 10.1086/283778
- K. Cuddington, The ‘balance of nature’ metaphor and equilibrium in population ecology, Biol Philos 16 (2001), pp. 463–479. Available at http://dx.doi.org/10.1023/A%3A1011910014900. doi: 10.1023/A:1011910014900
- J.M. Cushing and S.M. Henson, A periodically forced Beverton-Holt equation, J Differ Equ Appl 8 (2002), pp. 1119–1120. Available at <Go to ISI>://000179207900003. doi: 10.1080/1023619021000053980
- M. De la Sen, The generalized Beverton-Holt equation and the control of populations, Appl Math Model 32 (2008), pp. 2312–2328. Available at <Go to ISI>://WOS:000258438000009. doi: 10.1016/j.apm.2007.09.007
- M. De La Sen and S. Alonso-Quesada, On the impulsive Beverton-Holt equation and extinction conditions in population dynamics, Nat Resour Model 24 (2011), pp. 514–534. Available at <Go to ISI>://WOS:000296131700004. doi: 10.1111/j.1939-7445.2011.00106.x
- S. Ellner, Convergence to stationary distributions in two-species stochastic competition models, J Math Biol 27 (1989), pp. 451–462. Available at C:∖ante_lab∖Articles∖Ellner, S. P∖1989_Convergence to stationary distributions in two-species competition models.pdf doi: 10.1007/BF00290639
- H.T.M. Eskola and S.A.H. Geritz, On the mechanistic derivation of various discrete-time population models, Bull Math Biol 69 (2007), pp. 329–346. Available at <Go to ISI>://000243649800016. doi: 10.1007/s11538-006-9126-4
- B.M. Garay and J. Hofbauer, Robust permanence for ecological differential equations, minimax, and discretizations, SIAM J Math Anal 34 (2003), pp. 1007–1039. Available at <Go to ISI>://000183167700001. doi: 10.1137/S0036141001392815
- A. Gonzalez and R.D. Holt, The inflationary effects of environmental fluctuations in source-sink systems, Proc Natl Acad Sci U S A 99 (2002), pp. 14872–14877. Available at <Go to ISI>://000179224800048. doi: 10.1073/pnas.232589299
- J. Hatfield and P. Chesson, The multispecies lottery competition model: a diffusion analysis, in Structured Population Models in Marine, Freshwater, and Terrestrial Systems, S. Tuljapurkar and H. Caswell, eds., Chapman and Hall, New York, 1997, pp. 615–622.
- J. Hatfield and P.L. Chesson, Diffusion analysis and stationary distribution of the two-species lottery competition model, Theor Popul Biol 36 (1989), pp. 251–266. doi: 10.1016/0040-5809(89)90033-6
- S.T. Jackson, Conservation and resource management in a changing world: extending historical range of variation beyond the baseline, in Historical Environmental Variation in Conservation and Natural Resource Management, John Wiley & Sons, Ltd, Chichester, UK, 2012. pp. 92–109
- V.A.A. Jansen and K. Sigmund, Shaken not stirred: on permanence in ecological communities, Theor Popul Biol 54 (1998), pp. 195–201. Available at C:\ante_lab\Articles\Jansen, V.A.A. et al\1998_Shaken not stirred–on permanence in ecological communities.pdf doi: 10.1006/tpbi.1998.1384
- P.D. Jones, K.R. Briffa, T.P. Barnett, and S.F.B. Tett, High-resolution palaeoclimatic records for the last millennium: interpretation, integration and comparison with general circulation model control-run temperatures, Holocene 8 (1998), pp. 455–471. Available at<Go to ISI>://000075390900009. doi: 10.1191/095968398667194956
- O. Kallenberg (ed.), Foundations of Modern Probability Theory, Springer, New York, 1997.
- Y. Kang and P. Chesson, Relative nonlinearity and permanence, Theor Popul Biol 78 (2010), pp. 26–35. doi: 10.1016/j.tpb.2010.04.002
- C.A. Klausmeier, Successional state dynamics: A novel approach to modeling nonequilibrium foodweb dynamics, J Theor Biol 262 (2010), pp. 584–595. Available at <Go to ISI>://000274676700003. doi: 10.1016/j.jtbi.2009.10.018
- P.E. Kloeden, C. Pötzsche, Nonautonomous Dynamical Systems in the Life Sciences, Springer, Cham, Switzerland, 2013. pp. 312.
- P.E. Kloeden and C. Pötzsche, Nonautonomous bifurcation scenarios in SIR models, Math Methods Appl Sci 38 (2015), pp. 3495–3518. Available at http://onlinelibrary.wiley.com/doi/10.1002/mma.3433/abstract. doi: 10.1002/mma.3433
- P.E. Kloeden and M. Rasmussen (ed.), Nonautonomous Dynamical Systems, Mathematical Surveys and Monographs, Vol. 176, American Mathematical Society, Providence, RI, 2011.
- J. Kricher (ed.), The Balance of Nature: Ecology’s Enduring Myth, Princeton University Press, Princeton, 2009.
- R. Lande, Risks of population extinction from demographic and environmental stochasticity and random catastrophes., Am Nat 142 (1993), pp. 911–927. Available at hard copy available. doi: 10.1086/285580
- D.C. Reuman, R.F. Costantino, R.A. Desharnais, and J.E. Cohen, Colour of environmental noise affects the nonlinear dynamics of cycling, stage-structured populations, Ecol Lett 11 (2008), pp. 820–830. Available at<Go to ISI>://000257655400007. doi: 10.1111/j.1461-0248.2008.01194.x
- J. Ripa and A.R. Ives, Food web dynamics in correlated and autocorrelated environments, Theor Popul Biol 64 (2003), pp. 369–384. Available at http://www.sciencedirect.com/science/article/B6WXD-4961VXG-1/2/e7f5477a2329392211e10b3628980119. doi: 10.1016/S0040-5809(03)00089-3
- K. Rohde (ed.), Nonequilibrium Ecology, Cambridge University Press, New York, 2005.
- S.J. Schreiber, M. Benaim, and K.A.S. Atchade, Persistence in fluctuating environments, J Math Biol 62 (2011), pp. 655–683. Available at<Go to ISI>://000289301800002. doi: 10.1007/s00285-010-0349-5
- J. Usinowicz, C.-H. Chang-Yang, Y.-Y. Chen, J.S. Clark, C. Fletcher, N.C. Garwood, Z. Hao, J. Johnstone, Y. Lin, M.R. Metz, T. Masaki, T. Nakashizuka, I.-F. Sun, R. Valencia, Y. Wang, J.K. Zimmerman, A.R. Ives, and S.J. Wright, Temporal coexistence mechanisms contribute to the latitudinal gradient in forest diversity, Nature advance online publication (2017). Available at http://dx.doi.org/10.1038/nature24038.
- J. Usinowicz, S.J. Wright, and A.R. Ives, Coexistence in tropical forests through asynchronous variation in annual seed production, Ecology 93 (2012), pp. 2073–2084. Available at <Go to ISI>://000309517200011. doi: 10.1890/11-1935.1
- S.V. Zelik and V.V. Chepyzhov, Regular attractors of autonomous and nonautonomous dynamical systems, Dokl Math 89 (2014), pp. 92–97. Available at <Go to ISI>://WOS:000333204700025. doi: 10.1134/S106456241401030X
Appendix
Proof
Proof of Theorem 1
For forward convergence, note that monotonicity of the process ensures that any two trajectories must maintain their relative order. Thus, if K ≥ n’ > n, then Nn’,s,t ≥ Nn,s,t for all t > s. Define
(A1)
(A1) If τn,s is finite, then because Nn’,s,t ≥ Nn,s,t, Nn’,s,t = Nn,s,t for t = τn,s, and for all later t. To see that τn,s is finite, note that ln Nn,s,t = Σ(s, t) + ln n < lnK for t < τn,s. Infinite τn,s means that Σ(s, t) + ln n < lnK for all finite t ≥ s. But as Σ(s, t) → ∞ as t → ∞, there is a finite t > s for which Σ(s, t) + ln n ≥ lnK, contradicting the postulate that τn,s is infinite. Thus, τn,s is finite. Indeed, τn,s is the first t > s, for which Σ(s, t) + ln n ≥ lnK, at which point, the two trajectories Nn’,s,t and Nn,s,t have merged. Finally, monotonicity of τn,s in n, means that n ≥ ϵ implies τn,s ≤ τϵ,s and hence t ≥ τ = τϵ,s implies Nn’,s,t = Nn,s,t = Nϵ,s,t, which can be chosen as
.
Proof
Proof of Theorem 2
Backward convergence is more complex due to the fact that not all trajectories can be continued backwards in time. However, the forward proof gives the key method. First, I demonstrate that any two trajectories starting at the same time s must converge as s decreases. Consider again trajectories Nn’,s,t and Nn,s,t, as above, but now t is fixed and I first show that Nn’,s,t and Nn,s,t become equal when s is decreased sufficiently. First define
(A2)
(A2) Note that the set on the RHS of (A2) is nonempty because Σ(s, t) converges to ∞ as s → −∞, and so eventually remains above any specified value Σ(u, t) as u is decreased further. It follows that Sn,t is finite. Because s ≤ Sn,t implies Σ(u, t) ≥ ln K – ln n, it follows as for forward convergence that Nn,s,u hits K for some u ∈{s + 1, … , t} at which point Nn’,s,u = Nn,s,u = K, proving Nn’,s,t = Nn,s,t.
The next issue is to show that Nn,s,t converges as s → −∞, i.e. the influence of the starting time diminishes to 0 as it recedes into the past, and so lims →−∞ Nt defines the aedt, . Note that the first part of the proof shows that any such limit is independent of the starting value n. I do this in a way that is analogous to showing that {Nn,s,t} is a Cauchy sequence in s by showing that after s has been reduced sufficiently, no further change in {Nn,s,t} is possible for fixed n and t. With Sn,t defined by (A2) assume s’ < s ≤ Sn,t. The previous result shows that both Nn,s,u and Nn,s’,u must reach K by t. First assume that Nn,s’,s ≥ Nn,s,s. Then both Nn,s,u and Nn,s’,u must reach K for some u ∈ {s + 1, s + 2, … , t}, and remain equal after reaching K. Now consider the case that Nn,s’,s < Nn,s,s. Let v be the last time u before s that Nn,s’,u reaches K or let v = s’ if there is no such time u. As v < Sn,t, Σ(v, t) ≥ ln K – ln n and therefore Nn,s’,u must reach K at some time u in {v + 1, v + 2, … , t} because Nn,s’,v = K (> n) or = n. However, by definition of v, and the hypothesis Nn,s’,s < Nn,s,s = n < K, this time cannot occur in the interval {s’+1, … , s} and so must occur in the interval {s + 1, s + 2, … , t}. Because Nn,s’,u ≤ Nn,s,u, Nn,s,u and Nn,s’,u must become equal when Nn,s’,u hits K in the interval {s + 1, s + 2, … , t}. This means that Nn,s,t remains constant in s for s ≤ Sn,t and has therefore converged at that point. Moreover, these results show that
for s = Sn,t i.e. simply by starting the process at this s or earlier for the given initial value yields the value of aedt exactly at the specified time t. Note that although Sn,t is a decreasing function of n, the starting value of the process, because trajectories converge on each other in the backwards sense, regardless of their positive starting value, they all end up at
having started sufficiently before Sn,t.
Now choose ϵ positive and less than K. Define . The above results show substituting ϵ for n and w for t, means that,
. Because once two trajectories become equal they remain equal for all subsequent times, this means also that
, for all t ≥ w. Now substituting s for s’ in the above results, we see that
still leads to convergence on the aedt by time w. Thus,
, for all t ≥ w. Finally, as Nn’,s,u and Nn,s,u become equal by time w with
and n’ > n = ϵ, we see that Ns ≥ ϵ, means
for all t ≥ w.
Proof that K is hit infinitely often in the threshold exponential
In the forward direction, the proof shows that K is reached in finite time starting from an arbitrary time s under the forward infinite sum condition. As s can be moved up to the first time K is hit, another finite hitting time follows every hitting time. Hence there are infinitely many of them.
For the aedt on { … , s −2, s −1}, the proof of convergence on the aedt, above, identifies the time Sn,t as is sufficient to yield the aedt by time t for the given starting value. For simplicity of notation call this time S(t). As shown above, , and moreover, Nn,S(t), u must hit K for some u ∈ {S(t) + 1, … , t}. Denote the last such time τ1. The aedt proof implies that earlier starting trajectories, from the initial value n must equal Nn,S(t), u by u = τ1. Hence
. Now assuming τn has been defined, redefine t = τn − 1. The same process identifying τ1 now identifies τn+1 < τn with
. Hence
infinitely often on { … , s −2, s −1}, for any s.
To see that under the backward infinite sum condition, infinitely many trajectories exists with the property lim t →−∞ Nt = 0, for a given t, let s0 be the last s for which Σ(s, t) ≤ 0, i.e. Σ(s, t) > 0 for all s < s0. Thus, for all u < s0, Σ(u, t) > 0, but since Σ(u, s0) + Σ(s0, t) = Σ(u, t) > 0, this means Σ(u, s0) > 0 for all u < s0. Choose any 0 < n < K, and define , and
(A3)
(A3) Then the process satisfies the threshold exponential iteration for u < s0 because it never reaches K for u < s0, and so it is simply an exponential iteration there. It naturally extends into the future using the full iteration threshold exponential formula. Because Σ(u, s0) → ∞ as u → −∞, it follows that limt →−∞ Nt = 0. As n is arbitrary, there is a continuum of such processes.
Proof of the monotonicity lemma
It is sufficient to prove an induction step: given Nt ≥Mt, and monotonicity of F, Nt +1 = F(Nt, Et) ≥F(Mt, Et) ≥ H(Mt, Et) = Mt +1. Alternatively, assuming monotonicity of H, Nt +1 = F(Nt, Et) ≥H(Nt, Et) ≥ G(Mt, Et) = Mt +1.
Domination of the threshold exponential by the Beverton-Holt
Neglecting time, t, and letting the threshold exponential R be defined in terms of the Beverton-Holt R as R(1 – K/σ), with K < σ, then the ratio of the Beverton-Holt to the threshold exponential
is
(A4)
(A4) For
this is equal to
(A5)
(A5) which is a montone increasing function of N, and so for
i.e.
, (A5) is greater than or equal to
For
, we have (A4) equal to
As this is decreasing in N, for
, i.e.
, the λ ratio is greater than or equal to
which is equal to 1. Hence the Beverton-Holt λ is always greater than or equal to the threshold exponential λ under the given parameter relationship.
Lottery model
Derivation of the inequality (18) for trajectory deviation.
First write
(A6)
(A6) It follows that
(A7)
(A7) which means
(A8)
(A8) Differentiating with respect to Nt gives
(A9)
(A9) Now the part in {} is
Note that
, and so the expression above reduces to
to give a numerator in (A9) equal to
(A10)
(A10) The denominator can be rearranged as follows
Note that the second to last and third to last terms above combine to yield
But this is simply minus the last term because
. It follows that the denominator reduces to
(A11)
(A11) Thus, we see that the derivative of (A8) with respect to Nt is the ratio of (A10) to (A11). Thus,
(A12)
(A12) where n2 = 1 − n1. Inequality (18) of the text then follows by noting first of all that the integrand above is nonnegative (positive when ρ ≠ 1). Next note that each factor of the denominator of the integrand is increasing in n1 when ρ > 1, and is maximal, with the value 1 + (1 − δ)(ρ − 1) = δ + (1 − δ)ρ at n1 = 1, and for ρ < 1 is decreasing and therefore maximal for n2 = 1, with the value, δ + (1 − δ)ρ−1. It follows that the denominator of the integrand is uniformly less than
, which proves the inequality (18) of the text.
Note that this derivation also shows that Zt + 1 – Zt is a nonincreasing function of Nt and strictly decreasing when ρ ≠ 1, as the derivative of Zt + 1 – Zt in Nt is computed as (A9) and simplifies to minus the integrand in (A12).
Bounding ΔZ by ΔN
By definition of ΔZ, we have
(A13)
(A13) which rearranges to
(A14)
(A14) Given N ∈ [c, 1 − c], the fact that ΔZ > 0, and N(1 − N) is minimized for N = c and 1 − c we obtain
(A15)
(A15)
Note also that ΔZt is monotone nonincreasing in t. Thus, the largest value of t under consideration minimizes the RHS. In particular ΔNmin of the text can be entered into the LHS of (11), while ΔZt is entered into the RHS, and the inequality remains valid.
Proof
Proof of Theorem 3
Theorem 3 depends on Lemma 3. To see that Lemma 3 is correct, note that on the set {μn = t}, except for a set of probability zero. However, on {μn = t},
, by condition (30). As this true for all t ≥ s, the lemma is proved.
The extended Borell-Cantelli lemma is presented as corollary 6.2 in Kallenberg [Citation32]. It can be restated for application here as
The extended Borell-Cantelli Lemma Let be a probability space and
a sequence of sub σ-algebras of
with the property,
. If {An} is a sequence of events with
, then the two events
differ by a set of probability 0.
Here we apply this lemma to as defined in the text and
. By lemma 3,
sums to infinity over n with probability 1. Thus, the extended Borel Cantelli Lemma says that
for infinitely many n, with probability 1, under the forward infinite sum conditions (29), which guarantee the existence of the infinite sequence {μn}. The set of times
(equation 22 of the text) thus grows without bound as t → ∞, with probability 1. The theorem then follows from the remark under inequality (25) of the text.
Backward convergence in the lottery
Throughout assume c < (1 − δ)/2, and define χ = {ln 1 − ln(1 − c), which is the value of Z for N = c. Define , which is the maximum decline in Z from χ. The developments use the notation below.
Table
Lemma A1
Each set contains at least one τm, i.e. one time τ for which Nτ ≥ c. Moreover,
, for all n for which
exists.
Proof
Proof of Lemma A1
, by definition of
. Therefore, either
in which case τ1
, or
because ψ is the maximum possible one-step decline in Z from X. In the second case
because
necessarily has the same sign as
. This means that
(A20)
(A20) From this we can conclude that either
(A21)
(A21) or Nτ ≥ c for some
. In either case, Nτ ≥ c for some
. Thus, in all cases
.
To prove the rest by induction, assume there is a value of , and choose the maximum such value. Now,
(A22)
(A22) because, by definition of
, no value of
can yield a greater value for
(A23)
(A23) Thus,
(A24)
(A24) Hence
(A25)
(A25) As a consequence, N must rise to ≥ c at some time between
and
, i.e.
following the same reasoning as the initial step, and the fact that τm is maximal in
. This completes the induction step. As every
contains at least one τm, it necessarily follows that
, for all n for which
exists, completing the proof.
Lemma A2
Assume the infinite sum conditions
(A26)
(A26) Then for every w ≤ t, there is a v < w with the property that there are at least two values of
for every s ≤ v, for j = 1, 2. Further, there is a τ ∈ {v + 1, … , w} with the property, Ns(τ) ∈ [c, 1 − c] whenever c < (1 − δ)/2.
Proof
Proof of Lemma A2
Given w < t, define τ* such that
(A27)
(A27) for all v ≤ τ*, j = 1, 2. Such a value exists because of the fact that both rj,u(c)sum to infinity. As
, for every s ≤ τ*, there is at least one
. If
is the largest
, then
(A28)
(A28) by (A27). Thus,
, but since
is the largest
it follow that
, i.e. there is at least one
for each j for every s ≤ τ*.
Now choose v so that
(A29)
(A29) for every τ ≤ v, j = 1,2. For s ≤ v, it follows that there is at least one
, j = 1, 2. This means that the interval {v + 1, … , w} has at least two values of
for each j. Thus, the interval has at least one value of τj,s,n, for each j. This means that either Ns, τ ∈ [c, 1 − c] for some τ ∈{v + 1, … , w}, or Ns, u crosses the interval [c, 1 − c] for u in {v + 1, … , w}. With c < (1 − δ)/2, the interval cannot be crossed without entering it. Thus, N(τ) ∈ [c, 1 − c] for some τ ∈{v + 1, … , w}.
Proof
Proof of Theorem 4
Part (1) is a simple consequence of Lemma A2 because w is arbitary, the {vn+1 + 1, … , vn} can be defined inductively as nonoverlapping {v + 1, … , w} intervals from the lemma. Part (2) then uses inequalities (24) and (25) of the text.
Threshold exponential bounds on the lottery model
For ρ > 1, the ratio of the lottery λ to the threshold exponential λ is
(A30)
(A30)
For , this becomes
(A31)
(A31) which is an increasing function of N. As
,
, which means (A31) is greater (A31) with
substituted for N, to give
With
, (A30) becomes
(A32)
(A32) which is a decreasing function of N for ρ > 1. Hence (A32) is minimized for
, i.e. for
. Substituting in (A32) leads to
which completes the demonstration that the lottery λ is greater than the threshold exponential λ given by equation (33) of the text for ρ > 1. The demonstration for ρ ≤ 1, proceeds in a parallel but simpler fashion.
Proof
Proof of Theorem 5
To prove that and
are trajectories of the lottery model, note that if f is a continuous strictly increasing real valued function and {xn} is a real-valued sequence, then
and the same result applies also to the liminf. If Nt +1 = F(Nt, Et) defines the lottery iteration, then F is continuous and strictly increasing in its first argument, and so we have
which means
, and hence that
is a trajectory of the lottery model. Flipping species, or using an analogous proof, shows the same for
. In a similar manner, monotonicity and continuity of F means also that these results to apply
and
in terms of the supremum and infimum over n.
The fact that and
are bounded by the threshold exponential aedts shows that
for an infinite sequence of values of t values converging to – ∞, with the same result for
, which proves part (a) of the theorem.
To prove part (b), equality of and
in the stationary case, note that the pair (
) is a Borel measurable function of the stationary sequence {ρu, u = t, t – 1, t – 2, …}. Due to time invariance (by definition) of the distribution of a stationary sequence, {ρu, u = t – w, t – w – 1, …} has the same distribution for all integers w. It follows that (
) has the same bivariate probability distribution for all integers w, or more succinctly, (
) has the same distribution for all t. A slight extension of this argument, moreover, shows that {(
)} is a stationary stochastic process. In particular, it follows that the log odds transformations (
) have the same distribution for all t. Note, however, inequality (18) of the text: substitute
to conclude that whenever ρt ≠ 1 and ΔZt ≠ 0, ΔZt+1 < ΔZt, and in general ΔZt+1 ≤ ΔZt. Thus, it is possible to define the limiting variable
, which exists as a positive real number or +∞ since ΔZu is nondecreasing as u decreases. Moreover, Y ≥ ΔZt. This issue now is to show that Y and ΔZt are equal and identically 0 when both the infinite sum conditions, and the stationary environment assumption hold.
The infinite sum conditions and ΔZt ≠ 0, mean Y > ΔZt because ρu must be different from 1 for infinitely many u as u → – ∞, meaning that ΔZu > ΔZu+1 ≥ ΔZt for infinitely many u < t by inequality (18). Thus, P(Y > ΔZt) > 0, unless P(ΔZt ≠ 0) = 0. Now Y, as the limit of ΔZu has a distribution equal to the limiting distribution of ΔZu . By stationarity, the distribution of ΔZu does not change with u. It follows that Y and ΔZt have identical distributions, but as shown below, this is incompatible with the P(Y > ΔZt) > 0 and P(Y ≥ ΔZt) = 1 (Y ≥ ΔZt, noted above, under all conditions). This contradiction means P(ΔZt ≠ 0) = 0, i.e. ΔZt = 0 with probability 1, i.e. with probability 1. It follows that
exists, and is independent of n. Hence it is the unique backward aedt,
. Moreover,
is a stationary stochastic process.
A loose end in this proof is that P(Y > ΔZt) > 0 and P(Y ≥ ΔZt) = 1, cannot both be true if Y and ΔZt have the same distribution. To see this, let Vq be the indicator of the event {Y ≤ q}, i.e. Vq equals 1 if the event occurs, and is otherwise zero. Define Wq to be the indicator of the event {ΔZt ≤ q}. Now P(Y ≥ ΔZt) = 1 means Vq – Wq ≥ 0, with probability 1. If Y > ΔZt, then there are infinitely many rational numbers q between Y and ΔZt, which means , where Q is the rational numbers. However, the monotone convergence theorem applies, as this is a countable nonnegative series. Hence,
. But
, by identity of distribution. Thus, the series is zero meaning
.
Construction of an environmental sequence precluding backward convergence on an aedt
In the lottery model, the mapping Nt to Nt + 1, for finite values of ϵt = ln ρt, is invertible and takes the interval (0,1) onto (0,1). Thus, for any s < t, there is a value of Ns ∈ (0,1) for which Nt is any desired value ∈ (0,1). It follows that the mapping Ns to Nt is also continuous and invertible from (0,1) onto (0,1) for any finite sequence of finite environmental values ϵu, u = s, … , t −1. Moreover, the mapping Nt to Nt + 1 is a continuous function of ϵt = ln ρt, with the property that Nt + 1 >, = or < Nt according to ϵt >, = or < 0. Finally, constant nonzero values of ϵu mean that Ns, t converges on 1 or 0, according to ϵt > or < 0, as s → − ∞ from any fixed value of Ns,s ∈ (0,1). These facts mean that there is always a finite environmental sequence ϵu, u = s, … , t −1, driving Ns, t to any given value ∈ (0,1) from any given value of Ns,s ∈ (0,1). Thus, there is a sequence of integers, sn, with sn →−∞ monotonically as n → −∞, and environmental sequences with the property that the resulting complete sequence {ϵu, u = … , t − 2, t − 1} takes
to
for odd n, and to
for even n. Thus, Ns, t does not converge as s → −∞. One particular construction of such an environmental sequence chooses a fixed ϵ > 0, then defines s1 to be the maximum value of s for which Ns, t ≥ ¾, with Ns, s = ½, and ϵu = ϵ, u = s, … , t−1. Then
is reduced if necessary to achieve
. Next, the sequence
is constructed in the same way to give
, but with with ϵu = −ϵ except for the value
which is reduced in magnitude to achieve exactly ¼. This process is repeated indefinitely, alternating positive and negative ϵu sequences to construct the whole environmental sequence.