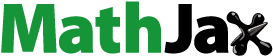
Abstract
In this paper, we introduce and deal with the delayed nutrient-microorganism model with a random network structure. By employing time delay τ as the main critical value of the Hopf bifurcation, we investigate the direction of the Hopf bifurcation of such a random network nutrient-microorganism model. Noticing that the results of the direction of the Hopf bifurcation in a random network model are rare, we thus try to use the method of multiple time scales (MTS) to derive amplitude equation and determine the direction of the Hopf bifurcation. It is showed that the delayed random network nutrient-microorganism model can exhibit a supercritical or subcritical Hopf bifurcation. Numerical experiments are performed to verify the validity of the theoretical analysis.
1. Introduction
The existence of the Hopf bifurcation in ecological systems is a hot investigation field, since its practical significance in biological control. Therefore, there are many results concerning the Hopf bifurcation in ecological systems that have been reported, see Refs. [Citation5–7,Citation12]. One of the most important results induced by the Hopf bifurcation is periodic solutions, including spatially homogeneous and spatially non-homogeneous periodic solutions. As a result, it is necessary to study or determine the stability of the periodic solution. As we know, there are two common techniques to study the direction of the Hopf bifurcation in a delayed differential equation, they are centre manifold reduction (CMR) and MTS, see Refs. [Citation2,Citation8,Citation11].
It is noticed that there are few results about the direction of the Hopf bifurcation in a delayed reaction-diffusion system with network structure have been reported [Citation9,Citation10], where the direction of the Hopf bifurcation is determined by employing the method of CMR. However, different from the technique is adopted in [Citation9,Citation10], we mainly attempt to use MTS to compute amplitude equation, and determine the direction of the Hopf bifurcation in a delayed nutrient-microorganism model with random network structure. More precisely, the system we consider takes the form
(1)
(1) This model is called nutrient-microorganism system in the sediment, and first proposed by Baurmann and Feudel in [Citation1](continuous form). All parameters
in (Equation1
(1)
(1) ) are positive constants;
is time delay, it implies that some time delay is required for microorganism to consume nutrient in the sediment.
For system (Equation1(1)
(1) ), we assume that it is defined on an undirected network with N nodes and there are no self-loops;
and
are the densities of the microorganism and the nutrient on node i, respectively;
is the
discrete Laplacian matrix of network with its elements
, where
is the adjacency matrix encoding the network connection, this indicates it satisfies
if there is a link connecting from patch i to patch j. If not,
when there is no any link connecting from patch i to patch j, and
is the Kronecker's delta [Citation4]. Moreover, the degree of the ith node is defined by
, and the connection probability between node i and node j for
is
.
This paper is structured as follows. In Section 2, we establish the existence results of the Hopf bifurcation of the model (1). In Section 3, the amplitude equation of the Hopf bifurcation is deduced. As a result, the supercritical or the subcritical Hopf bifurcation can be yield by analysing the amplitude equation. We perform the numerical simulations to verify the theoretical analysis in Section 4, and some discussions are made in Section 5.
2. Existence of the Hopf bifurcation
In this section, we give some conditions to ensure the existence of the Hopf bifurcation of the networked model (1). To this end, we first consider the existence and stability of positive equilibria for model (1).
Lemma 2.1
[Citation3]
The possible positive equilibria of model (1) can be found as follows
(i) | if | ||||
(ii) | if | ||||
(iii) | if |
Lemma 2.2
[Citation3]
When diffusion and delay are absent, the following statements are valid.
(i) | If | ||||
(ii) | If |
Thereby, in view of Lemma 2.1 and Lemma 2.2, we only focus on the dynamical behaviours near , and we denote it by
for simplicity. Now, we shall perform the linear stability analysis of system (1) near the positive equilibrium
. For this purpose, let
and
be the small perturbations, then the linear system of (1) evaluated at
can be written as follows
(2)
(2) where
and
Let
be the eigenvalues of the discrete Laplacian matrix
, and suppose that
is the subspace generated by the eigenfunctions associated to the topological eigenvalue
. Then, the general solution of system (2) can be rewritten as
with
. Inserting them into (2), one has
It then follows that
(3)
(3) where
Let
be the solution of Equation (3), we have
Thereby, if one of the conditions in the following
is satisfied, one yields
(4)
(4) We thus obtain the critical value τ of the Hopf bifurcation is
(5)
(5) where
. In addition, a straightforward calculation shows that
Moreover, note that
this implies that the transversality condition of the Hopf bifurcation is satisfied. Combine this with (Equation5
(5)
(5) ) we claim that the delayed network model (1) undergoes the Hopf bifurcation at
when
for
and
. Especially, the delayed network model (1) admits a Hopf bifurcation at
when
, and in this case the periodic solution bifurcated from the Hopf bifurcation is spatially homogeneous.
3. Direction of the Hopf bifurcation
In this section, we shall employ MTS to derive amplitude equations and determine the direction of the Hopf bifurcation. We first define and
, where
and
can be found in (Equation4
(4)
(4) ) and (Equation5
(5)
(5) ), respectively. Now, a rewritten form of the networked system (1) can be read as
(6)
(6) where we set
, and
with
In addition, the nonlinear term
takes the form
with
To employ the technique of MTS, let ε be a small perturbation parameter. Then introducing the time scales
, this induces that
(7)
(7) Solution
can be decribed as
(8)
(8) We thus obtain a fact that
(9)
(9) By a similar manner, we can write the nonlinear term
as follows
(10)
(10) where
and
Next, we introduce the small perturbation of the Hopf bifurcation parameter
with
. Keep this in mind, denote
and put (7)-(10) into (6), one has
:
(11)
(11)
:
(12)
(12)
:
(13)
(13) Considering the solution of perturbation Equation (Equation11
(11)
(11) ) near the Hopf mode, we have
(14)
(14) where we assume that
is the complex amplitude and
We thus obtain
This means that the perturbation Equation (Equation12
(12)
(12) ) has a particular solution with the form
(15)
(15) where the vectors
and
are unknown. Thence, to determine them we should insert (Equation15
(15)
(15) ) into (Equation12
(12)
(12) ). Then equating like terms of
and
, we have
As a result, it follows from (Equation14
(14)
(14) ) and (Equation15
(15)
(15) ) that
Now inserting all of them and (Equation14
(14)
(14) )–(Equation15
(15)
(15) ) into the perturbation (Equation13
(13)
(13) ), one obtains
(16)
(16) where
is the conjugation term,
represents the non-secular term and
with
where
Then perturbation Equation (Equation16
(16)
(16) ) possesses its solution when the Fredholm alternative condition is satisfied. It implies that the following orthogonal condition should be satisfied
(17)
(17) where we take
and
the inner product satisfies
. Then using (Equation17
(17)
(17) ) and noticing
, we obtain
(18)
(18) where we denote
In what follows, for the purpose of investigating the Hopf bifurcation near
, we shall find the amplitude equation on the centre manifold, it is useful for investigating the Hopf bifurcation. To this end, let
with z = x + iy, then it follows from (Equation18
(18)
(18) ) that
(19)
(19) where
and
represent the real part and imaginary part of •. Now, we let
and
, then (Equation19
(19)
(19) ) becomes
(20)
(20) where
and
.
By virtue of (Equation20(20)
(20) ), we have the following results about the direction of the Hopf bifurcation.
Theorem 3.1
The direction of the Hopf bifurcation depends on (Equation20(20)
(20) ). More precisely
(i) | if | ||||
(ii) | if
|
Proof.
It is easy to verify that (Equation20(20)
(20) ) has a unique positive equilibrium (corresponding to the Hopf bifurcation)
, and thus its existence condition is
. It indicates (i) is true. Moreover, Equation (Equation20
(20)
(20) ) has a unique eigenvalue, say λ, and
. By employing linear stability analysis theory, we know that (ii) is valid. The proof is completed.
4. Numerical simulations
In this section, we main verify the effectiveness of Theorem 3.1 by numerical simulations. We assume that the numbers of the node are 100, namely we take N = 100 in the random network model (1). Moreover, we fix the connecting probability p = 0.35 between different nodes and
for
. We now choose the parameters in system (1) are
and
, then one obtains the positive equilibrium
and
. This means that
and
are satisfied in Theorem 3.1.
Figure shows that the distribution of solutions with the development of moments. When taking time delay
, we find that the positive equilibrium
is locally asymptotically stable, see Figure . Figure suggests that there is a supercritical Hopf bifurcation, and the periodic solution with the spatial homogeneity bifurcated from the Hopf bifurcation is stable in the delayed network model (1), where we choose
. As such, the results in Theorem 3.1 are valid.
5. Conclusions
We deal with a nutrient-microorganism model with time delay and random network structure in this paper. By employing time delay τ as the critical parameter of the Hopf bifurcation, we explore its occurrence conditions. For the direction of the Hopf bifurcation, we try to adopt MTS to derive amplitude equation near , it is found that the sign of
determines the existence of the Hopf bifurcation. Namely the Hopf bifurcation exists when
, and there is no Hopf bifurcation when
. Moreover, the sign of
determines the direction of the Hopf bifurcation with hypothesis
. More precisely, the Hopf bifurcation is supercritical (resp. subcritical) and the periodic solution is stable (resp. unstable) when
(resp.
). Numerical simulations indicate that our theoretical analysis is valid, and compared with the works done in [Citation9,Citation10] we claim that MTS is a easier technique to determine the direction of the Hopf bifurcation in a delayed network model than CMR. For more interesting results about this networked model, for example, resonant/nonresonant Hopf bifurcation, will be further considered.
Disclosure statement
No potential conflict of interest was reported by the author(s).
Additional information
Funding
References
- M. Baurmann and U. Feudel, Turing patterns in a simple model of a nutrient-microorganism system in the sediment, Ecol. Complex. 1 (2004), pp. 77–94.
- M. Bentounsi, I. Agmour, and N. Achtaich, The Hopf bifurcation and stability of delayed predator-prey system, Comput. Appl. Math. 37(5) (2018), pp. 5702–5714.
- M.X. Chen, R.C. Wu, B. Liu, and L.P. Chen, Hopf-Hopf bifurcation in the delayed nutrient-microorganism model, Appl. Math. Model. 86 (2020), pp. 460–483.
- Y. Ide, H. Izuhara, and T. Machidac, Turing instability in reaction diffusion models on complex networks, Physica A. 457 (2016), pp. 331–347.
- A. Kumar and B. Dubey, Modeling the effect of fear in a prey-predator system with prey refuge and gestation delay, Int. J. Bifurcat. Chaos. 29(14) (2019), pp. 1950195.
- Y.Q. Liu, D.F. Duan, and B. Niu, Spatiotemporal dynamics in a diffusive predator prey model with group defense and nonlocal competition, Appl. Math. Lett. 103 (2020), pp. 106175.
- K. Manna and M. Banerjee, Stability of Hopf-bifurcating limit cycles in a diffusion-driven prey-predator system with Allee effect and time delay, Math. Biosci. Eng. 16(4) (2019), pp. 2411–2446.
- B. Roy, S.K. Roy, and D.B. Gurung, Holling-Tanner model with Beddington-DeAngelis functional response and time delay introducing harvesting, Math. Comput. Simul. 142 (2017), pp. 1–14.
- Y.L. Shi, Z.H. Liu, and C.R. Tian, Hopf bifurcation in an activator-inhibitor system with network, Appl. Math. Lett. 98 (2019), pp. 22–28.
- C.R. Tian, Z. Ling, and L. Zhang, Delay-driven spatial patterns in a network-organized semiarid vegetation model, Appl. Math. Comput. 367 (2020), pp. 124778.
- T.H. Zhang, Y.L. Song, and H. Zang, The stability and Hopf bifurcation analysis of a gene expression model, J. Math. Anal. Appl. 395 (2012), pp. 103–113.
- H.Y. Zhao and D.Y. Wu, Point to point traveling wave and periodic traveling wave induced by Hopf bifurcation for a diffusive predator-prey system, Discrete Cont. Dyn. Syst. S. 13(11) (2020), pp. 3271–3284.