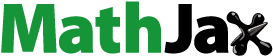
Abstract
In this paper, we propose a stochastic delay mutualistic model of leaf-cutter ants with stage structure and their fungus garden, in which we explore how the discrete delay and white noise affect the dynamic of the population system. The existence and uniqueness of global positive solution are proved, and the asymptotic behaviours of the stochastic model around the positive equilibrium point of the deterministic model are also investigated. Furthermore, the sufficient conditions for the persistence of the population are established. Finally, some numerical simulations are performed to show the effect of random environmental fluctuation on the model.
1. Introduction
Mutualism is a universal phenomenon in the ecosystem, which refers to the cooperative survival relationship among species. For example, euryhaline tilapia co-exist and evolve with chlorella, the similiar relationship also happens to pollinating fine moths and Euphorbiacecae plants, legumes and rhizobium, and so on. Sometimes, predation behaviours also lead to reciprocal effect. Leaf mites can increase cucumber yield by consuming some cucumber seedlings. These interesting phenomena have attracted the attention of scholars. In 1925, Lotka and Volterra [Citation16, Citation20] have successively established a mathematical model of the interaction between biological populations, which was known as Lotka–Volterra model. After that, many scholars have studied the dynamic behaviour of Lotka–Voterra cooperative model. For example, Yao et al. [Citation24] added impulse and harvest term on the basis of previous studies, and generalized the research results of Lotka–Volterra cooperation model by coincidence degree theory and extension theorem of inequality analysis in 2006. Then in 2017, Feng et al. [Citation8] supplemented delay and impulse to Lotka–Volterra cooperation model, by constructing appropriate Lyapunov functions, sufficient conditions for the existence and uniform asymptotic stability of periodic solutions of the system are obtained. It is worth mentioning that there are also plenty of models based on reality that are different from Lotka–Volterra model, for instance, Kang et al. [Citation12] established a model of leaf-cutter ants and their fungus garden, which introduces in detail about how they cooperate with each other. The model is as follows:
(1)
(1) where
is the total biomass of ants including workers, larvae, pupae and eggs at time t,
is the total biomass of the fungus at time t,
and
can be considered as a parameter measuring the division of labour in the colony of ants. The meaning of other parameters is shown in Table , all parameters are positive constants.
Table 1. The meaning of some parameters.
The mutualism relationship between leaf-cutter ants and their fungus garden that we intend to study in this paper is very fascinating. The ant is very good at cutting leaves with its tail, hence it was named the ‘leaf-cutter ant’. In the Amazon rainforest, they carry leaves back to their nest not for food, but to plant fungus. To supply a place with good environment for fungus' growth, the leaves will be cut into smaller pieces and stack together after they are moved into the nest, then, under the meticulous care of the leaf-cutter ants, the mycelia quickly grows into a fungus garden. In fact, the relationship between the leaf-cutter ants and their fungus garden just like human plant mushroom and eat them, except that they don't sell the fungus to other leaf-cutter ant tribes. After the leaf-cutter ants have scattered their mycelia, if they do not look after the mycelia carefully, such as weeding, fertilizing and spraying, they will not produce a good harvest, exactly as humans grow crops.
The environment inside the nest is very favourable for the growth of the fungus, which also creates hotbeds for the growth of miscellaneous bacteria. Obviously, leaf-cutter ants face two difficulties during the cultivation process. One is how to ensure that the fungus is not contaminated by other bacteria. The other is how do they protect themselves from microbes in an environment surrounded by so many bacteria. In fact, leaf-cutter ant's mouth and forelimb are infested with parasites that can produce streptomycin, they can adjust the active ingredient of streptomycin automatically according to the species of harmful bacteria that have been present. The infected fungus will be picked out and threw away by the ants, if streptomycin does not kill the bacteria. The ants are surrounded by a wide range of pathogenic bacteria all the time, why the ants are not infected by microbes is that they have chosen to live symbiosis with a bacterium of the genus Pseudonocardia. The bacteria attach to the ant's body like armour, they secrete antibiotics once the ant is invaded by the pathogen, which help the ant fight off the intruding pathogen and mould. So far, scientists have isolated a variety of strains from the leaf-cutter ant and extracted the corresponding gene sequence to test whether the corresponding antibodies they produced are active in humans. Scientists hope to use these special bacteria to produce new antibiotics to help humans treat diseases more effectively.
In this paper, the functional response function is defined as the culture rate of fungus by leaf-cutter ants. In 1975, J. R. Beddington and D. L. DeAngelis proposed Beddington–DeAngelis type functional response [Citation3, Citation6], referred to as B–D functional response, which is the combination of Holling II functional response and ratio-dependent functional response, has the advantages of prey-dependent functional response and ratio-dependent functional response. Obviously, both of the biomass of leaf-cutter ants and fungus can affect the fungus culture rate. Leaf-cutter ants would reduce the culture rate when there are enough fungus, and on the contrary, they would increase the culture rate when there are less fungus, however, the upper limit of culture rate is related to the number of worker ants among leaf-cutter ants. To have a better explanation of the dynamic behaviour between leaf-cutter ants and their fungus garden, B-D functional response is considered in this paper.
For the sake of making the model more realistic, many scholars introduce stage structure into population model on account of the different abilities and behaviours of species at different ages [Citation1, Citation13, Citation15, Citation18, Citation21, Citation23]. Leaf-cutter ants are classified as the maturity and the immaturity, the adult leaf-cutter ants will participate in the cultivation of fungus, while the immaturity, including juvenile ants, eggs and pupae, need to be fed by adult leaf-cutter ants. Then, we get the following model with stage structure and B-D function response
(2)
(2) where
represent the biomass of immature and mature ants, respectively,
represents the biomass of fungus,
is the rate of death,
,
are parameters that measure the maximum growth rate of ants and fungus, respectively,
indicates the collection rate of fungus collected by adult ants, a can be considered as a parameter measuring the division of labour in the colony of ants, all parameters are strictly positive.
Time delay is an important factor in the biological mathematical model [Citation2, Citation11, Citation19, Citation22]. In this paper, we introduce gestation time into the model, it is a period that the fungus begins to grow from spores. To cultivate fungus, leaf-cutter ants need to cut leaves first, after the leaves are transported back to the nest, the gardener ants will be responsible for the subsequent cultivation of the fungus garden, the leaves are cleaned and arranged in tight rows and in suitable positions by the gardener ants, and then they are decomposed by the secretions of the gardener, which are rich in enzymes and can form a mucus layer on the leaves' surface. With abundant nutrients, the mucus layer is a natural culture medium in which the gardener ants ‘seed’ a small amount of their own fungus, and then the careful care of ants makes the fungi grow. The period of the fungus from spores to starting growing is a relatively complex gestation time, which can be called ‘gestation time’, then the model (Equation2(2)
(2) ) with time delay can be described as
(3)
(3) where τ represents the gestation delay.
An ecosystem is inevitably disturbed by random fluctuations such as temperature, humidity, rainfall, and sometimes even artificial factors in the wild [Citation5, Citation7, Citation10, Citation11, Citation14, Citation17, Citation25]. The sudden torrential rain will bring devastating disaster to the leaf-cutter ants, and the drought will increase the death rate of the fungus garden, hence, it is reasonable to consider the random fluctuations in the mutualistic system and it will enrich the dynamic behaviour of the population. Therefore, we consider that the mortality of leaf-cutter ants and fungus are affected by the natural environment in our model.
Let
where
denote the independent standard Brownian motion, and
denote the intensity of white noise [Citation17, Citation25]. Then, the stochastic system takes the form
(4)
(4) and
(5)
(5) where
and
are nonnegative continuous function on
,
.
We have the following problems to be solved about this system: first, investigate whether the model has a globally unique positive solution, and how about the asymptotic behaviour of the model, then we want to know whether the sufficient conditions for the persistence of the model can be established. Naturally, we hope to analyse the influence of the environment disturbance on the model.
2. Existence and uniqueness of global positive solutions
In this section, we study the existence and uniqueness of positive solution of system, which is the basis of studying the long-time behaviour of the model. First of all, we present some known results and lemma which will be used later.
In general, consider the d-dimensional stochastic differential equation
(6)
(6) with the initial value
.
denotes a d-dimensional standard Brownian motion defined on the complete probability space
. The family of all nonnegative functions
defined on
, such that they are continuously twice differentiable in X and once in t. The differential operator L of Equation (Equation6
(6)
(6) ) is defined by Mao [Citation17].
If L acts on a function
, then
where
,
,
. In view of Itô formula, if
, then
Theorem 2.1
For any given initial condition (Equation5(5)
(5) ), model (Equation4
(4)
(4) ) has a unique global positive solution
on
, and the solution will remain in
with probability 1.
Proof.
Since the coefficients of system satisfy the local Lipschitz condition, then for any initial value (Equation5(5)
(5) ), there exists a unique local solution
,
on
, where
is the explosion time. To verify that this solution is global, we only need to prove that
a.s. For this purpose, let
be large enough such that every component of
lay within the interval
. For each integer
, we define stopping time:
We can see that,
is increasing as
, define
( denotes the empty set). Let
and
a.s. Hence to complete the proof, we need to show that
.
Define a -function
, applying the
formula [Citation17], one can compute that
Then we have
and
, where
,
,
.
(7)
(7) Note that
. We can get
For any
and
, we obtain
(8)
(8) where
Equation (Equation8
(8)
(8) ) and the Gronwall inequality [Citation4, Citation17] imply that
It then follows that
(9)
(9) We can hence show that
Additionally, letting
in Equation (Equation9
(9)
(9) ) gives
(10)
(10) For
,
(11)
(11) where
. Similarly, we obtain that
and
Repeating this procedure, one can show
with probability one for any inter
. Therefore,
a.s.
3. Asymptotic property
After introducing white noise into the deterministic model, the solution of the system will be disturbed under the influence of random fluctuations. Therefore, we will discuss the asymptotic property of stochastic system (Equation4(4)
(4) ) around positive equilibrium point of the corresponding deterministic model (Equation3
(3)
(3) ).
System (Equation3(3)
(3) ) has the same equilibrium point as system (Equation2
(2)
(2) ). Hence, we just need to consider the following equations:
(12)
(12) We can deduce
from the second equation of (Equation12
(12)
(12) ), we get
.
From the third equation of (Equation12(12)
(12) ), we have
that is
where
, let
,
,
.
Then
(13)
(13) Then, if the condition
is satisfied, we can get one or two positive solutions of (Equation13
(13)
(13) ), simplicity, let's write them all as
. We denote all the positive equilibria as
.
To facilitate the study, we define the following assumptions:
(14)
(14)
Theorem 3.1
For any given initial condition (Equation5(5)
(5) ), if hypothesis
and
are established, the solution
of system (Equation4
(4)
(4) ) has the property
(15)
(15) where
and
,
is positive equilibrium point of model (Equation3
(3)
(3) ),
are positive constants to be determined later.
Proof.
Define the function
(16)
(16) where
And
are positive constants to be determined later.
The positive equilibrium satisfies the following properties:
(17)
(17) By
formula, we obtain
Therefore, we have
(18)
(18) where
Choosing
then details calculation process of
is given in Appendix, then we have
We can obtain
(19)
(19) Integrating both sides of (Equation18
(18)
(18) ) from 0 to t and taking the expectation, we get
(20)
(20) Dividing both sides by t and taking the limit superior, we have
(21)
(21) Obviously,
(22)
(22) where
(23)
(23) We have completed the proof.
Remark 3.1
Theorem 3.1 shows that if the initial condition (Equation5(5)
(5) ) holds, the solution of system (Equation4
(4)
(4) ) oscillates around the equilibrium point
of system (Equation3
(3)
(3) ) under certain conditions, and the upper bound of the solution of system (Equation4
(4)
(4) ) is positively correlated with the intensity of
.
4. Persistence
In nature, whether the population has the conditions for persistence is an issue of great concern to us. Before discussing the persistence of stochastic system, we give the following assumption:
(24)
(24) where
,
.
Theorem 4.1
For any given initial condition (Equation5(5)
(5) ), if assumptions
,
and
hold, the system (Equation4
(4)
(4) ) is persistent, that is
(25)
(25)
Proof.
According to (Equation15(15)
(15) ), we have
(26)
(26) As we know,
and
, from
, we yield
(27)
(27) By the condition of
, we have
(28)
(28) Similarly, when
,
This completes the proof of Theorem 4.1.
5. Numerical simulations
In this section, we will verify theory results and discuss the effect of environmental noise. We use the Milstein's method to simulate the stochastic model (Equation4(4)
(4) ) [Citation9], the numerical scheme for stochastic model (Equation4
(4)
(4) ) is given by
(29)
(29) where
are the Gaussian random variables
, and time increment
.
We adopt the following parameter values:
(30)
(30) We fix the initial value
except for the other specification, we obtain
. Next we take into account the effect of environmental noise intensities on the system by comparing the following different situations. Use matlab to plot diagrams of those cases.
In case 1, we take
, we conclude that
, and
, it is obvious that the conditions of
,
, and
in Theorem 4.1 are satisfied, the system is persistence. It is easy to see that the solution of stochastic model oscillate around the equilibrium point of the deterministic model and maintain persistent in Figure , when the white noise is small. To satisfy the conditions of Theorem 4.1, we should select the value of
as smaller as possible, so there is no need to verify
in the following cases because we just take it sufficient small.
Then, to analyse the effect of
, we change
and
in case 2 and case 3, respectively, the value of
in case 2, and
in case 3, we can conclude that case 2 satisfy Theorem 4.1 but case 3 do not. After comparing Figures and , we find out that the change of
will affect the fluctuation of the whole system. More specifically, when
increases appropriately, the solution of the leaf-cutter ants and the fungus remain persistent below the equilibrium point of the deterministic model, and all three populations of the stochastic model are rapidly extinct when
is large enough, which indicates that a random variation of the death rate of immature leaf-cutter ants will turn the persistent population into extinction.
Furthermore, we chose
and
in case 4 and case 5 to explore the effect of
on the system (Equation4
(4)
(4) ),
can satisfy Theorem 4.1, but
cannot satisfy Theorem 4.1. Which can be concluded by comparing Figures and is that the small changes of
will have a great impact on the whole system, and even lead to the extinction of the fungus (see Figure c,d).
At last, comparing Figures and that plotted by case 6 which
and case 7 which
, we can make inquiry for the impact of
on system (Equation4
(4)
(4) ),
can satisfy Theorem 4.1, but
cannot satisfy Theorem 4.1, we can conclude that
have the same impact as
on the system, that is to say when
increases appropriately, the solution of the leaf-cutter ants and the fungus remain persistent below the equilibrium point of the deterministic model, and all populations are rapidly extinct when
is large enough, which indicates that a random variation of the death rate of immature leaf-cutter ants will turn the persistent population into extinction.
6. Conclusion
In this paper, we propose a stochastic delay mutualistic model of the leaf-cutter ants with stage structure and their fungus garden. First of all, we analyse the existence and uniqueness of the positive solution, and consider the asymptotic property of the positive solution, then we give the sufficient conditions for the persistence of the population. By exploring some numerical simulations, we conclude the impact of the impact of environmental disturbances on the system. In nature, the secretion of leaf-cutter ants contains many unknown antibodies, so it is of great scientific significance to maintain the persistence of leaf-cutter ants and their fungus. The research results of this paper provide a good solution for preventing the extinction of species. That is, to artificially reduce the impact of environmental disturbances on the system, such as watering the fungus nursery during drought.
Figure 1. The evolution of for model (Equation4
(4)
(4) ) and its corresponding deterministic model (Equation3
(3)
(3) ) with initial value
: (a) time series diagram of
in case 1:
and (b) phase diagram of
in case 1:
.
![Figure 1. The evolution of (x1(t),x2(t),y(t)) for model (Equation4(4) {dx1(t)=(r1x2(t)y(t)−d1x1(t)−cx1(t))dt+σ1x1(t)dB1(t),dx2(t)=(cx1(t)−d2x2(t))dt+σ2x2(t)dB2(t),dy(t)=[r2ax2(t−τ)y(t−τ)m+m1y(t−τ)+m2x2(t−τ)−d3y(t)−r3x2(t)y(t)]dt+σ3y(t)dB3(t),(4) ) and its corresponding deterministic model (Equation3(3) {dx1(t)dt=r1x2(t)y(t)−d1x1(t)−cx1(t),dx2(t)dt=cx1(t)−d2x2(t),dy(t)dt=r2ax2(t−τ)y(t−τ)m+m1y(t−τ)+m2x2(t−τ)−d3y(t)−r3x2(t)y(t),(3) ) with initial value (x1(0),x2(0),y(0))=(2,1,3): (a) time series diagram of x1(t),x2(t),y(t) in case 1: σ1=0.05,σ2=0.05,σ3=0.01 and (b) phase diagram of x1(t),x2(t),y(t) in case 1: σ1=0.04,σ2=0.03,σ3=0.02.](/cms/asset/3dbba90a-8e58-4d00-84b1-38b53e18f097/tjbd_a_2099590_f0001_oc.jpg)
Figure 2. The evolution of for model (Equation4
(4)
(4) ) and its corresponding deterministic model (Equation3
(3)
(3) ) with initial value
: (a) time series diagram of
in case 2:
; (b) phase diagram of
in case 2:
; (c) time series diagram of
in case 3:
; (d) phase diagram of
in case 3:
.
![Figure 2. The evolution of (x1(t),x2(t),y(t)) for model (Equation4(4) {dx1(t)=(r1x2(t)y(t)−d1x1(t)−cx1(t))dt+σ1x1(t)dB1(t),dx2(t)=(cx1(t)−d2x2(t))dt+σ2x2(t)dB2(t),dy(t)=[r2ax2(t−τ)y(t−τ)m+m1y(t−τ)+m2x2(t−τ)−d3y(t)−r3x2(t)y(t)]dt+σ3y(t)dB3(t),(4) ) and its corresponding deterministic model (Equation3(3) {dx1(t)dt=r1x2(t)y(t)−d1x1(t)−cx1(t),dx2(t)dt=cx1(t)−d2x2(t),dy(t)dt=r2ax2(t−τ)y(t−τ)m+m1y(t−τ)+m2x2(t−τ)−d3y(t)−r3x2(t)y(t),(3) ) with initial value (x1(0),x2(0),y(0))=(2,1,3): (a) time series diagram of x1(t),x2(t),y(t) in case 2:σ1=0.4,σ2=0.05,σ3=0.01; (b) phase diagram of x1(t),x2(t),y(t) in case 2:σ1=0.4,σ2=0.05,σ3=0.01; (c) time series diagram of x1(t),x2(t),y(t) in case 3:σ1=1.4,σ2=0.05,σ3=0.01; (d) phase diagram of x1(t),x2(t),y(t) in case 3:σ1=1.4,σ2=0.05,σ3=0.01.](/cms/asset/a2c79604-27a1-4cfb-950c-f32ed229711b/tjbd_a_2099590_f0002_oc.jpg)
Acknowledgments
We are grateful to the editor and reviewers for their valuable comments and suggestions that greatly improved the presentation of this paper.
Disclosure statement
No potential conflict of interest was reported by the author(s).
Additional information
Funding
References
- W.G. Aiello and H.I. Freedman, A time-delay model of single-species growth with stage structure, Math. Biosci. 101 (1990), pp. 139–153.
- J.F.M. Al-Omari, A stage-structured predator-prey model with distributed maturation delay and harvesting, J. Biol. Dyn. 9 (2015), pp. 278–287.
- J.R. Beddington, Mutural interference between parasites or predators and its effect on searching efficiency, J. Animal Ecology 44 (1975), pp. 331–340.
- Y.M. Cai, S.Y. Cai, and X.R. Mao, Stochastic delay foraging arena predator–prey system with Markov switching, Stoch. Anal. Appl. 38 (2020), pp. 191–212.
- Z.W. Chen, R.M. Zhang, J. Li, S.W. Zhang, and C.J. Wei, A stochastic nutrient-phytoplankton model with viral infection and Markov switching, Chaos Solitons Fractals 140 (2020), p. 110109.
- D.L. DeAngelis, R.A. Goldstein, and R. O'neill, A model for trophic interacting, Ecology 56 (1975), pp. 811–892.
- Y. Deng and M. Liu, Analysis of a stochastic tumor-immune model with regime switching and impulsive perturbations, Appl. Math. Model. 78 (2020), pp. 482–504.
- C.H. Feng, Existence and uniqueness of positive almost periodic solutions for a class of impulsive Lotka–Volterra cooperation models with delays, British J. Math. Computer Sci. 22 (2017), pp. 1–8.
- D. Higham, An algorithmic introduction to numerical simulation of stochastic differential equations, SIAM Rev. 43 (2001), pp. 525–545.
- Y.L. Huang, W.Y. Shi, C.J. Wei, and S.W. Zhang, A stochastic predator–prey model with Holling II increasing function in the predator, J. Biol. Dyn. 15 (2021), pp. 1–18.
- W. Ji and M. Liu, Optimal harvesting of a stochastic commensalism model with time delay, Phys. A Statist. Mechan. Appl. 527 (2019), p. 121284.
- Y. Kang, R. Clark, M. Makiyama, and J. Fewell, Mathematical modeling on obligate mutualism: interactions between leaf-cutter ants and their fungus garden, J. Theor. Biol. 289 (2011), pp. 116–127.
- Q. Liu, D. Jiang, T. Hayat, A. Alsaedi, and B. Ahmad, Dynamical behavior of a stochastic predator-prey model with stage structure for prey, Stoch. Anal. Appl. 38 (2020), pp. 647–667.
- G.D. Liu, H.K. Qi, Z.B. Chang, and X.Z. Meng, Asymptotic stability of a stochastic may mutualism system, Comput. Math. Appl. 79 (2020), pp. 735–745.
- C. Liu, Q. Zhang, Y. Zhang, and X. Duan, Bifurcation and control in a differential-algebraic harvested prey–predator model with stage structure for predator, Int. J. Bifurcation Chaos 18 (2008), pp. 3159–3168.
- A.J. Lotka, Elements of physical biology, Am. J. Public. Health.15 (1925), p. 812.
- X.R. Mao, Stochastic Differential Equations and Applications, Horwood Publishing, West Sussex, 1997.
- M. Peng and D.Z. Zhang, Bifurcation analysis and control of a delayed stage-structured predator–prey model with ratio-dependent Holling type III functional response, J. Vibration Control 26 (2020), pp. 1232–1245.
- Y. Takeuchi, Y. Saito, and S. Nakaoka, Stability, delay, and chaotic behavior in a Lotka–Volterra predator–prey system, Math. Biosci. Eng. 3 (2006), pp. 173–187.
- V. Volterra, Variations and fluctuations of the number of individuals in animal species living together, ICES J. Marine Sci. 3 (1928), pp. 3–51.
- W.D. Wang and L.S. Chen, A predator–prey system with stage-structure for predator, Computers Math. Appl. 33 (1997), pp. 83–91.
- J. Wei, Bifurcation analysis in a scalar delay differential equation, Nonlinearity 20 (2007), pp. 2483–2498.
- F.Y. Wei and Q.Y. Fu, Globally asymptotic stability of a predator-prey model with stage structure incorporating prey refuge, Int. J. Biomath. 9 (2016), p. 1650058.
- X.J. Yao and F.J. Qin, On four positive almost periodic solutions to a Lotka–Volterra cooperative system with impulses and harvesting terms, J. Southwest China Normal University 4 (2016), pp. 7–14.
- X.W. Yu and S.L. Yuan, Asymptotic properties of a stochastic chemostat model with two distributed delays and nonlinear perturbation, Discrete Continuous Dyn. Systems Ser B 25 (2020), pp. 2373–2390.
Appendix
If Equation (Equation19(19)
(19) ) holds, the following equations should be satisfied:
(A1)
(A1)
(A2)
(A2)
(A3)
(A3)
(A4)
(A4)
(A5)
(A5) From Equation (EquationA1
(A1)
(A1) ), we can obtain
.
It is easy to conclude that Equation (EquationA5(A5)
(A5) ) holds, if we have the following equations:
Considering Equations (EquationA2
(A2)
(A2) ) and (EquationA5
(A5)
(A5) ), if we have
then Equation (EquationA2
(A2)
(A2) ) holds.
From Equations (EquationA4(A4)
(A4) ) and (EquationA5
(A5)
(A5) ),
, at last we need
, combine with
, so we need
, that is
.
In summary, we have