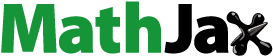
Abstract
In this paper, we consider a stochastic two-species predator–prey system with modified Leslie–Gower. Meanwhile, we assume that hunting cooperation occurs in the predators. By using Itô formula and constructing a proper Lyapunov function, we first show that there is a unique global positive solution for any given positive initial value. Furthermore, based on Chebyshev inequality, the stochastic ultimate boundedness and stochastic permanence are discussed. Then, under some conditions, we prove the persistence in mean and extinction of system. Finally, we verify our results by numerical simulations.
1. Introduction
It is well known that the predator–prey relationship widely exists between two species. Therefore, predator–prey systems have been studied extensively, and the rich results have been achieved. Leslie [Citation1] and Leslie et al. [Citation2] assumed that the carrying capacity of the predator's environment is proportional to the number of prey and introduced the following predator–prey system:
(1)
(1) Here
and
denote the population densities of prey and predator at time t.
, and f are all positive parameters. a and r are the intrinsic growth rate of the prey and predator, respectively. b means the competitive strength among individuals of the prey. f is the maximum value of the per capita reduction rate of predator. The term
is called the functional response function, which describes the number of prey successfully attacked by individual predators. The term
is called the Leslie–Gower term, and it implies that the carrying capacity of the predator's environment is proportional to the number of prey, and not a constant (Logistic form).
Aziz-Alaoui [Citation3] and Nindjin et al. [Citation4] explained that the predator may convert to catch other populations when the prey x is severely scarce. However, its growth will be limited even if its most favourite food x is not available in abundance. In order to solve such a problem, they thought it was necessary to add a positive constant m to the denominator. Thus, system (Equation1(1)
(1) ) becomes the following predator–prey system with modified Leslie–Gower:
(2)
(2) In general, the functional response function
represents the attack power of predator on prey. In the classical Lotka–Volterra system,
takes the form
. This is reasonable since the number of prey hunted by the individual predator is naturally proportional to the number of the prey. Further, this function was revised by Holling, and three functional response functions were proposed to describe the predator behaviour on prey. One of Holling functional response functions is Holling II functional response, which takes the following form
ρ is the encounter rate between predators and their prey, and h is the handling time of predator on prey. In recent years, the Leslie–Gower predator–prey model with Holling II functional response has been widely studied by many scholars. Arancibia-Ibarra et al. [Citation5] considered a modified Leslie–Gower system with Holling-type II schemes and Allee effect. It was revealed that system with a strong Allee effect had two positive equilibrium points and system with a weak Allee effect had three positive equilibrium points. Abid et al. [Citation6] investigated a predator–prey model with modified Leslie–Gower and Holling-type II functional response. They discussed the existence and boundedness of solutions, then analysed the existence and local stability of the possible steady states. Moreover, the conditions of global stability of the interior equilibrium were established.
In nature, cooperative behaviour within populations is a very widespread phenomenon. Alves et al. [Citation7] advised that cooperative predators may benefit from their behaviour since the success of predator's attacks on prey increases. They added a cooperation term to describe the attack of a predator on a prey and studied a predator–prey system with hunting cooperation. The attack rate (or encounter rate) of the predator was assumed to be . Therefore, the corresponding Holling II functional response function with hunting cooperation is as follows:
where
is the attack rate of the per predator on the prey,
represents the intensity of the predator cooperation in hunting. Then, based on system (Equation2
(2)
(2) ), we have the following system
(3)
(3) On the other hand, in the real world, environmental noise exists everywhere, which is bound to have an impact on the development of populations. Therefore, many scholars introduced white noise into predator–prey systems and studied the influence of white noise on predator–prey systems. Xu et al. [Citation8] considered a stochastic predator–prey system with modified Leslie–Gower and Holling-type IV schemes. They proved the stochastic boundedness and stochastic permanence, then they investigated the persistence in mean and extinction. Meanwhile, the existence of a ergodic stationary distribution was discussed. Zhou et al. [Citation9] studied a stochastic predator–prey model with modified Leslie–Gower and Holling-type II schemes. Especially, sufficient conditions of persistence in mean and extinction were given. The rich results for the stochastic population systems can also see [Citation10–12].
In this paper, we assume that in system (Equation3(3)
(3) ), the intrinsic growth rates a and r of the prey and predator are affected by white noise, that is,
where
are mutually independent Brownian motions, and
represent the intensities of the white noises. Then, corresponding to system (Equation3
(3)
(3) ), a stochastic two-species predator–prey system with modified Leslie–Gower and hunting cooperation among predators can be described as follows:
(4)
(4) The dynamic behaviours of the stochastic predator–prey system (Equation4
(4)
(4) ) may provide effective ideas for population protection. From the biological sense, the first concern is that the solution of the system is non-negative, then the stochastic permanence and extinction are also very significant issues. Therefore, our paper is organized as follows. In Section 2, by Itô formula and proper Lyapunov function, it is shown that there is a unique global positive solution
to system (Equation4
(4)
(4) ) for any positive initial value
. In Section 3, the stochastic ultimate boundedness of solution is studied. In Section 4, it is proved that system (Equation4
(4)
(4) ) is stochastically permanent under Assumption A. In Section 5, some sufficient conditions for permanence in mean and extinction of system (Equation4
(4)
(4) ) are obtained. Finally, we present numerical examples to verify our theoretical results.
In this paper, unless otherwise specified, we let be a complete probability space with a filtration
satisfying the usual conditions (i.e. it is increasing and right continuous all P-null sets). Also let
,
.
2. Positive and global solutions
It is necessary that proving the population density of prey and predator is non-negative. In general, in order to prove that there exists a unique global solution for any given initial value for a stochastic differential equation, the coefficients of the equation need to satisfy the linear growth condition and the local Lipschitz condition. However, the coefficients of system (Equation4(4)
(4) ) neither satisfy the linear growth condition, nor local Lipschitz condition. In this section, by making the change of variables and applying Itô formula, we will firstly show there is a unique positive local solution for system (Equation4
(4)
(4) ).
Lemma 2.1
For any given initial value , there is a unique local positive solution
to system (Equation4
(4)
(4) ) for
a.s., where
is the explosion time.
Proof.
Letting , and applying Itô formula, we get the following system
(5)
(5) and the initial value
. Obviously, the coefficients of system (Equation5
(5)
(5) ) are locally Lipschitz continuous, so there exists a unique local solution
of system (Equation5
(5)
(5) ) on
, where
is the explosion time. Therefore, there is also a unique local positive solution
of system (Equation4
(4)
(4) ) for any given initial value
on
, here
.
Next, we will prove system (Equation4(4)
(4) ) has a global positive solution for any given initial value
.
Theorem 2.2
For any given initial value , there is a unique solution
for system (Equation4
(4)
(4) ) on
, and the solution will remain in
with probability one, namely
for all
almost surely.
Proof.
By Lemma 2.1, we know that there is a unique local positive solution for system (Equation4(4)
(4) ). Further, we show that this solution is global, i.e.
. Let
and
be sufficiently large such that
and
all lie within the interval
. For each integer
, define the stopping time:
where
(∅ is the empty set). Obviously,
is increasing as
. Let
. Easy to get
If we can show
, then
Now, we only need to prove
If not, we assume
a.s., there are two constants T>0 and
, such that
Therefore, there exists an integer
such that when
, we have
(6)
(6) In order to finish our proof, we will construct
-function
under two different cases.
Case 1: If , we define a function
as follows:
Since
for all u>0, it is known that the function
. Letting
, for
, and applying Itô formula to
, we obtain
here
where
and
are positive constants. Since
for
, we have
where
, consequently
Therefore, integrating sides of the above inequality from 0 to
and then taking expectation, we get
Further, by Gronwall inequality, we get
where
The remainder of the proof is similar to Theorem 1 in [Citation8], we omit the details.
Case 2: If , we define a
-function
as follows:
Applying Itô formula to
, we obtain that
where
Here
and
are positive constants. Similar to the proof under Case 1, we obtain the existence of a global and positive solution.
3. Stochastically ultimate boundedness
Definition 3.1
[Citation13]
The solution of system (Equation4
(4)
(4) ) is said to be stochastically ultimately bounded, if for any
, there is a positive constant
, such that for any given initial value
, the solution
of system (Equation4
(4)
(4) ) has theproperty that
(7)
(7)
Before proving boundedness, we first prove the following lemma.
Lemma 3.2
There exists a constant independent of the initial value
, such that the solution
of system (Equation4
(4)
(4) ) has the following property
(8)
(8)
Proof.
Define
(9)
(9) where
. Hence, for
, by the Itô formula, we have
(10)
(10) Here
Noting that
and
it is followed by
where
. Further, there is a constant
, such that
Substituting the above inequality into (Equation10
(10)
(10) ) gives
Once again by the Itô formula, we get
Integrating both sides of the above inequality from 0 to t, and then taking expectations, we get
This implies that
On the other hand, we have
Therefore, we can obtain
Based on the above conclusion, we can obtain the stochastically ultimate boundedness of the solution of system (Equation4
(4)
(4) ).
Theorem 3.3
The solution of system (Equation4(4)
(4) ) is stochastically ultimately bounded.
Proof.
By Lemma 3.2, there is such that
Now, for any
, let
, then by Chebyshev inequality, we have
Hence
By Definition 3.1, the solution of system (Equation4
(4)
(4) ) is stochastically ultimately bounded.
4. Stochastic permanence
Definition 4.1
[Citation14]
The solution of system (Equation4
(4)
(4) ) is said to be stochastically permanent, if for any
, there exists a pair of positive constants
and
, such that for any initial value
, the solution
to system (Equation4
(4)
(4) ) has the properties that
For convenient of discussion, we give the following assumption.
Assumption A
.
Theorem 4.2
If Assumption A holds, there exists a positive constant , which is independent of the initial value
, such that the solution
of system (Equation4
(4)
(4) ) has the property that
(11)
(11) where
is an arbitrary positive constant satisfying
(12)
(12) and p>0 satisfying
(13)
(13)
Proof.
Define , by the Itô formula, we obtain
where
Under Assumption A, there certainly exists a constant θ such that it satisfies (Equation12
(12)
(12) ). And denote
, then by the Itô formula, we get
where
By (Equation13
(13)
(13) ), we can choose p>0 sufficiently small such that it satisfies
. Thus by the Itô formula, we compute
where
It is easy to get that
and
Therefore
By
, it is no difficult to see that there is a constant
such that
Then
Integrating both sides of the above inequality from 0 to t, and then taking the expectation, we obtain that
where
, so
Note that
Therefore
Theorem 4.3
Under Assumption A, system (Equation4(4)
(4) ) is stochastically permanent.
Proof.
By Theorem 4.2, there exists F>0 such that
Thus, for any
, let
, then by Chebyshev inequality, we obtain
It is straightforward to see that
In other words
On the other hand, by Theorem 3.3, we can see that there is a constant
, such that
that is
By Definition 4.1, the solution of system (Equation4
(4)
(4) ) is stochastically permanent.
5. Persistent in mean and extinction
In this section, we discuss the persistence in mean and extinction for system (Equation4(4)
(4) ).
Definition 5.1
[Citation15]
(1) | The population | ||||
(2) | The population |
Theorem 5.2
Suppose be a solution of system (Equation4
(4)
(4) ) with the initial value
, then:
(i) | If | ||||
(ii) | If | ||||
(iii) | If |
Proof.
(i) Applying Itô formula to , we have
Integrating both sides from 0 to t the above equation, it yields
Further, we have
(14)
(14) Applying the strong law of large numbers of local martingales, we obtain
Then dividing by t on both sides of (Equation14
(14)
(14) ), and letting
, we can easily derive
therefore
Hence for arbitrary
, there exist
, such that
for
. Thus,
Following Lemma A.1 of [Citation16], and by Comparison Theorem for the stochastic equation, we have
For the arbitrary of
, we have
Similar to the proof of the above (i) and Theorem 3.4 of [Citation16], we may obtain conclusion (ii) and (iii). The details are omitted.
6. Numerical simulations
By using the Milstein method in [Citation17], we can get the discretization system corresponding to system (Equation4(4)
(4) ) as follows:
(15)
(15) where
is time increment,
are two independent Gaussian random variables, and
.
Example 6.1
For system (Equation4(4)
(4) ), we choose
By Theorem 3.3, we know that the solution of system (Equation4(4)
(4) ) is stochastically ultimately bounded. In order to verify this conclusion, we draw the solution curve ‘
’ with parameters (1)
, (2)
, and (3)
respectively. In Figure , the figures of solution ‘
’ with the initial value
are drawn, which shows that the numerical result is identical to our theoretical result. Note that the solution of the deterministic system (4) (system (4) with
) is ultimately bounded since
and
, which leads to
and
. Therefore, we conclude that noises do not influence the ultimate boundedness of the solution.
Figure 1. Solutions of system (Equation4(4)
(4) ) with
Green lines:
. Red lines:
. Blue lines:
.
![Figure 1. Solutions of system (Equation4(4) {dx(t)=x(t)[a−bx(t)−(λ+αy(t))y(t)1+h(λ+αy(t))x(t)]dt+σ1x(t)dB1(t),dy(t)=y(t)(r−fy(t)m+x(t))dt+σ2y(t)dB2(t).(4) ) with a=0.8, b=0.3, λ=0.5, α=0.25, h=1, r=0.5, f=0.8, m=1. Green lines: σ1=σ2=0. Red lines: σ1=σ2=0.15. Blue lines: σ1=σ2=0.3.](/cms/asset/0314a7f2-4e2f-46f8-820f-b7cbf37d2b28/tjbd_a_2366495_f0001_oc.jpg)
Example 6.2
For system (Equation4(4)
(4) ), we choose
Considering system (4) with It is easily know that the solution
of the deterministic system (Equation4
(4)
(4) ) with the initial value
is positive. Therefore, it is true, for
, that
Further, we have
. Let
. Then we have
. Meanwhile, similar to Example 6.1, the ultimate boundedness of the solution for the deterministic system (4) is easily obtained. Hence, by Definition 4.1, we know that system (Equation4
(4)
(4) ) with
is permanent.
Next, the white noises are considered. Let . By computing, we have
which implies that all the parameters satisfy ‘Assumption A’. Similarly, we choose
It is obvious that ‘Assumption A’ is also true. By Theorem 4.3, system (Equation4
(4)
(4) ) is stochastically permanent. In Figure , we draw figures of solution ‘
’ with the initial value
, and the stochastical permanence of system (Equation4
(4)
(4) ) is shown.
Figure 2. Solutions of system (Equation4(4)
(4) ) with
Green lines:
. Red lines:
. Blue lines:
.
![Figure 2. Solutions of system (Equation4(4) {dx(t)=x(t)[a−bx(t)−(λ+αy(t))y(t)1+h(λ+αy(t))x(t)]dt+σ1x(t)dB1(t),dy(t)=y(t)(r−fy(t)m+x(t))dt+σ2y(t)dB2(t).(4) ) with a=1, b=0.6, λ=0.8, α=0.25, h=1, r=1.5, f=1, m=0.2. Green lines: σ1=σ2=0. Red lines: σ1=σ2=0.2. Blue lines: σ1=σ2=0.5.](/cms/asset/44f49976-4cc8-48e5-b4ea-ac260be0ffa5/tjbd_a_2366495_f0002_oc.jpg)
It is revealed by Theorem 4.3 that white noises do not influence the permanence when the intensity of white noises is not too strong and satisfies ‘Assumption A’.
Example 6.3
For system (Equation4(4)
(4) ), we choose
These parameters are the same as those in Example 6.2. In order to see the influence of white noises, in the following, we change the intensity of white noises.
(i) | Let | ||||
(ii) | Let | ||||
(iii) | let |
Figure 3. Solutions of system (Equation4(4)
(4) ) with
Red lines:
. Blue lines:
.
![Figure 3. Solutions of system (Equation4(4) {dx(t)=x(t)[a−bx(t)−(λ+αy(t))y(t)1+h(λ+αy(t))x(t)]dt+σ1x(t)dB1(t),dy(t)=y(t)(r−fy(t)m+x(t))dt+σ2y(t)dB2(t).(4) ) with a=1, b=0.6, λ=0.8, α=0.25, h=1, r=1.5, f=1, m=0.2. Red lines: σ1=0.2,σ2=1.8. Blue lines: σ1=σ2=0.](/cms/asset/7f2573df-470f-462c-95de-d1bb3721f44a/tjbd_a_2366495_f0003_oc.jpg)
Figure 4. Solutions of system (Equation4(4)
(4) ) with
Red lines:
. Blue lines:
.
![Figure 4. Solutions of system (Equation4(4) {dx(t)=x(t)[a−bx(t)−(λ+αy(t))y(t)1+h(λ+αy(t))x(t)]dt+σ1x(t)dB1(t),dy(t)=y(t)(r−fy(t)m+x(t))dt+σ2y(t)dB2(t).(4) ) with a=1, b=0.6, λ=0.8, α=0.25, h=1, r=1.5, f=1, m=0.2. Red lines: σ1=1.5,σ2=0.2. Blue lines: σ1=σ2=0.](/cms/asset/9228a635-ee0d-40eb-a70b-4bbf8f324bec/tjbd_a_2366495_f0004_oc.jpg)
Figure 5. Solutions of system (Equation4(4)
(4) ) with
Red lines:
. Blue lines:
.
![Figure 5. Solutions of system (Equation4(4) {dx(t)=x(t)[a−bx(t)−(λ+αy(t))y(t)1+h(λ+αy(t))x(t)]dt+σ1x(t)dB1(t),dy(t)=y(t)(r−fy(t)m+x(t))dt+σ2y(t)dB2(t).(4) ) with a=1, b=0.6, λ=0.8, α=0.25, h=1, r=1.5, f=1, m=0.2. Red lines: σ1=1.5,σ2=1.8. Blue lines: σ1=σ2=0.](/cms/asset/0edce9f2-9fba-4328-991d-060c93a4bc63/tjbd_a_2366495_f0005_oc.jpg)
7. Conclusion
In this paper, a stochastic predator–prey system with modified Leslie–Gower and hunting cooperation among predators is studied. We first prove the existence and uniqueness of a global positive solution, and we investigate the stochastically ultimate boundedness, and the stochastic permanence of the solution. Then several sufficient conditions are established for persistence in mean and extinction. In the end, using Matlab software, some numerical simulations are provided to verify our results.
Some interesting conclusions are obtained. First, the ultimate boundedness of solution is not influenced by noises no matter how strong the intensity of noises is. However, white noises may influence the persistence. Noting that all parameters are the same except the values of and
in Example 6.2 and 6.3; therefore, the influence of white noise is revealed directly by comparing Figures and –. Figure reveals a fact that the permanence do not influenced by white noises when the intensity of white noises is not too strong and satisfies ‘Assumption A’. But, when the intensity of white noises is strong enough, what will occur for the dynamic behaviours of system (4)? The changes of the solution for system (4) are clear in Figures –. If the predator is influenced by strong noise, its persistence will be destroyed, and it may be go to extinct(see Figure ). It is the same for the prey (see Figure ). Further, If the predator and the prey are influenced by strong noises, their persistence will be destroyed, and they may be go to extinct(see Figure ). Therefore, in order to the persistence of system (4), system should avoid being affected by white noise.
In the future, we will study other properties further. For instance, we will investigate the existence of the stationary distribution for system (Equation4(4)
(4) ) and others.
Disclosure statement
No potential conflict of interest was reported by the author(s).
Additional information
Funding
References
- Leslie PH. Some future notes on the use of matrices in population mathematics. Biometrika. 1948;35:213–245. doi: 10.1093/biomet/35.3-4.213
- Leslie PH, Gower JC. The properties of a stochastic model for the predator-prey type of interaction between two species. Biometrika. 1960;47:219–234. doi: 10.1093/biomet/47.3-4.219
- MA Aziz-Alaoui. Study of a Leslie-Gower–type tritrophic population model. Chaos, Solitons Fract. 2002;14:1275–1293. doi: 10.1016/S0960-0779(02)00079-6
- Nindjin AF, MA Aziz-Alaoui, Cadivel M. Analysis of a predator-prey model with modified Leslie-Gower and Holling-type II schemes with time delay. Nonl Anal. 2006;7:1104–1118. doi: 10.1016/j.nonrwa.2005.10.003
- C Arancibia-Ibarra, Flores J. Dynamics of a Leslie-Gower predator-prey model with Holling-type II functional response, Allee effect and a generalist predator. Math Comput Simul. 2021;188:1–22. doi: 10.1016/j.matcom.2021.03.035
- Abid W, Yafia R, MA Aziz-Alaoui, et al. Dynamics analysis and optimality in selective harvesting predator-prey model with modified Leslie-Gower and Holling-Type II. Nonauton Dyn Syst. 2019;6:1–17. doi: 10.1515/msds-2019-0001
- Alves MT, Hilker FM. Hunting cooperation and Allee effects in predators. J Theoret Biol. 2017;419:13–22. doi: 10.1016/j.jtbi.2017.02.002
- Xu DS, Liu M, Xu XF. Analysis of a stochastic predator-prey system with modified Leslie-Gower and Holling-type IV schemes. Phys A. 2020;537:122761. doi: 10.1016/j.physa.2019.122761
- Zhou DX, Liu M, Liu ZJ. Persistence and extinction of a stochastic predator-prey model with modified Leslie-Gower and Holling-type II schemes. Adv Differ Equ. 2020;179:1–16.
- Liu M. Dynamics of a stochastic regime-switching predator-prey model with modified Leslie-Gower Holling-type II schemes and prey harvesting. Nonl Dyn. 2019;96:417–442. doi: 10.1007/s11071-019-04797-x
- Lv JL, Wang K. Stochastic predator-prey model with Leslie-Gower and Holling-type II schemes with regime switching. Rocky Mt J Math. 2018;48:1201–1218. doi: 10.1216/RMJ-2018-48-4-1201
- Wei CJ, Liu JN, Zhang SW. Analysis of a stochastic eco-epidemiological model with modified Leslie-Gower functional response. Adv Differ Equ. 2018;119:1–17.
- Li XY, Mao XR. Population dynamical behavior of non-autonomous Lotka-Volterra competitive system with random perturbation. Discrete Contin Dyn Syst. 2009;24:523–545. doi: 10.3934/dcds.2009.24.523
- Lv JL, Wang K. Asymptotic properties of a stochastic predator-prey system with Holling II functional response. Commun Nonl Sci Numer Simul. 2011;16:4037–4048. doi: 10.1016/j.cnsns.2011.01.015
- Zhao DL, Yuan SL. Dynamics of the stochastic Leslie-Gower predator-prey system with randomized intrinsic growth rate. Phys A. 2016;461:419–428. doi: 10.1016/j.physa.2016.06.010
- Ji CY, Jiang DQ, Shi NZ. Analysis of a predator-prey model with modified Leslie-Gower and Holling-type II schemes with stochastic perturbation. J Math Anal Appl. 2009;359:482–498. doi: 10.1016/j.jmaa.2009.05.039
- Higham DJ. An algorithmic introduction to numerical simulation of stochastic differential equations. SIAM Rev. 2001;43:525–546. doi: 10.1137/S0036144500378302