ABSTRACT
A consensus gap exists between scientific and public opinion on the existence and causes of anthropogenic climate change (ACC). Public opinion on ACC is influenced by individual trust in science and the use of different media sources. We used systematic review and applied meta-analysis to examine how trust in science and the use of new versus traditional, and centralized versus user-generated media are related to public opinion on ACC. We compiled two data sets: trust in science (n = 13, k = 18) and media use (n = 12, k = 68). Our results showed a positive relationship between the levels of trust, media use, and ACC beliefs in line with the scientific consensus (i.e. pro-social ACC beliefs), with media use being moderated by nationality. Additionally, the effect size for using new or user-generated media sources was twice as large as using traditional or centralized media sources.
Introduction
Anthropogenic climate change (ACC), primarily attributed to the indiscriminate burning of fossil fuels and resulting increases in atmospheric concentrations of carbon dioxide (CO2), is one of the most demanding global challenges of our time. However, despite volumes of growing empirical evidence in support of ACC, there is still a large gap between scientific and public opinion on the existence, causes, and effects of ACC. Several independent studies have shown that scientific support for ACC varies between 90 and 100%, whereas only 67% of Americans and 78% of Europeans believe that the biosphere is warming or that it is caused by human activities (Cook et al., Citation2013; Cook et al., Citation2016; Cook et al., Citation2018; Doran & Zimmerman, Citation2011; Fairbrother et al., Citation2019; Lynas et al., Citation2021; Oreskes, Citation2004). Known as the “consensus gap,” discussion has focused on reasons for the large disparity in scientific and public opinion over ACC.
Public opinion on ACC may be influenced by people’s trust in scientists and the type of media that individuals use to gather information on ACC. How scientists and the scientific practice are viewed and valued by the public has changed over time (Funk, Citation2017; Gauchat, Citation2012; King & Short, Citation2017; KNAW, Citation2013; Krause et al., Citation2019; Rainie et al., Citation2015; Wynne, Citation2006). Science and scientists were considered a distant, elite group of individuals that were highly respected over most of the nineteenth and twentieth century. More recently, dissemination of science via the internet and open access publishing have made science and scientists more accessible than ever before (Brossard, Citation2013; Prior, Citation2005; Su et al., Citation2015). On the one hand, it allows the public audiences to read scientific papers that used to be locked behind paywalls; on the other hand, it enables individuals or organizations to “cherry-pick” studies they like, while dispensing with those they do not, or to “quote-mine” and thus distort the conclusions of the research. This has possibly led to a blurring in the public view of what is seen as “scientific evidence,” a personal opinion or a (deliberate) one-sided representation of a scientific subject.
Over the past two decades the emergence of new media sources, available through access to the internet, and including social media forums (Twitter, Reddit, Facebook, blogs, etc.) are likely to have played a significant role in influencing public opinion on ACC. These platforms are generally unregulated, meaning that anyone can write their opinion online on a range of topics without any need for accountability or accuracy. ACC in media remains plagued by misrepresentations of the scientific consensus. Instances of false balance, overemphasis of scientific uncertainty in climate models and projections, or altogether inaccurate interpretations of uncertainty and what that means for mitigation, create misunderstanding and are often (directly or indirectly) influenced by the fossil fuel industry, ACC denial groups, and think tanks (Boykoff & Boykoff, Citation2004; Dunlap & Brulle, Citation2020; Moser, Citation2010; Shackley & Wynne, Citation1996).
Digital dissemination has also enabled groups with a vested interest in denial of ACC, such as fossil-fuel funded think tanks and lobbying groups, to more easily reach large numbers of the general population, and to use fear and propaganda to drive a wedge between scientific and public opinion (Almiron et al., Citation2020; Bloomfield & Tillery, Citation2019; Dunlap & Brulle, Citation2020). Most worryingly, these new sources of information have provided a very visible platform to non-experts who promulgate false information, which, to the lay audiences is often hard to distinguish from accurate scientific output (Kozyreva et al., Citation2020; Slater, Citation2007; Vraga et al., Citation2019).
There have been multiple studies into the effects of media use and trust in science on public opinion of ACC. However, due to the high context dependency of these types of studies meta-analysis is necessary to draw general conclusion, as the effects reported in those studies do not indicate a clear trend or are sometimes even contradictory. Therefore, here we provide a meta-analysis on the influence of trust in science and the use of different media sources to see whether there is a general pattern emerging on these factors influencing public opinion on ACC.
Scientific authority and public trust
Public trust in science and scientists has been expressed in multiple, sometimes overlapping, terms in the academic literature: confidence in scientists, the cultural authority of science, scientific authority, or deference to science (we refer to Howell et al., Citation2020 for a clear distinction between these terms). These terms express different levels of authoritarianism, but all of them are based on a premise about to what extent scientific knowledge is valued and trusted over other sources of information (Howell et al., Citation2020). The cultural authority of science is based on its perceived impartiality and objectivity, the lack of economic or political interests of science, and autonomy of scientists (O’Brien, Citation2012).
Most societies today look to scientists when it comes to providing a reliable base of knowledge for policy development, innovation, and solving issues across a vast range of fields (King & Short, Citation2017). An important part of that authority is built on public and political trust and if one of these assumptions is lacking, the cultural authority of science may lose credibility. Although there is no clear decline in the public's overall trust in science (Gauchat, Citation2012; Krause et al., Citation2019), the issue of a possible legitimacy problem in the political and cultural role of scientists in society has become a subject of public and academic discourse (Gauchat, Citation2011; Huber et al., Citation2019; Krause et al., Citation2019). However, specifically in the case of ACC, the perceived credibility of science among a certain part of the public has decreased, probably due to political beliefs or a general lack in trust as a result of controversial events (i.e. climategate) and deliberate public discrediting of climate science by climate change contrarians (Cook et al., Citation2018; Dunlap & Brulle, Citation2020; Kulin et al., Citation2021; Lewandowsky, Citation2021).
Media use as a source of scientific information
Online and print media have traditionally been the primary source of scientific information for most people (Brossard, Citation2013; Jarreau & Porter, Citation2018; Su et al., Citation2015). Over the past two decades, however, the use of online media sources has been steadily rising, sometimes even replacing older traditional media platforms. This provides new opportunities, but also new challenges, in terms of scientific accuracy (Brossard, Citation2013; Huber et al., Citation2019; O’Neill & Boykoff, Citation2010; Su et al., Citation2015). The rise of online media has given the public increased access to non-scientific information and the opportunity to consult multiple sources that are becoming increasingly hard to verify (Huber et al., Citation2019; Vraga et al., Citation2019).
Media content is shaped by the information it contains and the way that information is presented. How information is presented, or framed, by a communicator (journalist, editor, etc.) influences how the receiver (audience) will interpret and internalize the information that is conveyed (Boykoff, Citation2008; Nisbet, Citation2014; Stecula & Merkley, Citation2019). Framing theory is important in understanding how different messages create different understandings and responses by the audience as framing is often more important in shaping opinions than factual content (Baum & Potter, Citation2008; Nisbet, Citation2014). Journalistic norms in media that are created by journalists and editors in a process of centralized content creation (e.g. a newspaper or TV channel) play an important role in how ACC has been framed over time (Boykoff, Citation2008; Boykoff & Boykoff, Citation2007; Brüggemann & Engesser, Citation2017). However, online media sources provide a largely user-generated and unregulated forum for non-experts to spread (dis)information. This makes it increasingly difficult for the non specialist reader to separate science-based information from non-scientific or even false information due to practical (e.g. the increased spread of fake news) and psychological factors (Kozyreva et al., Citation2020; Lewandowsky & Van der Linden, Citation2021; Slater, Citation2007; Vraga et al., Citation2019). The lack of journalistic norms and editorial boards in a subset of privately curated, often online, sources could lead to differences in content and messaging between media types (Kozyreva et al., Citation2020).
Interplay between trust in science and media use
Research into public opinion on scientific subjects has been approached employing three main models, (1) the knowledge-attitude model, assuming a relationship between access to information leading to a higher level of knowledge of science, in turn leading to a more favorable attitude towards it, (2) the alienation model, describing a dissociation from science by the public in line with a general alienation from governmental institutions, and (3) the cultural meaning of science, looking at how different public views on what science is influences either acceptance or reservation towards science (King & Short, Citation2017; see Gauchat, Citation2011 for a detailed description of these models). Focusing on the issue of ACC, attention has been shifted from a scientific literacy model (based on the same assumptions as the knowledge-attitude model), to researching cognitive factors, ideology, personal experience with science, and social environments as contributing factors to public attitude towards climate science (Brownlee et al., Citation2013; Eom et al., Citation2019; Gauchat, Citation2011; Lewandowsky et al., Citation2019; O’Brien, Citation2012; Wynne, Citation2006). Research shows that media use and trust in science are often positively correlated for scientific subjects like nanotechnology (Anderson et al., Citation2012; Lee & Scheufele, Citation2006), agricultural technology (Brossard & Nisbet, Citation2006), and also ACC (Diehl et al., Citation2019; Hmielowski et al., Citation2014).
Additionally, political ideology has an influence on both media use and trusts in science. For trust in science, studies have shown that political opinion influences scientific trust, but these studies are mostly USA-focused and may therefore not accurately translate to other countries (Baker et al., Citation2020; Hamilton & Safford, Citation2020; McCright et al., Citation2013). Trust in science in the USA is relatively stable among liberals and moderates, but showed a decline among conservatives between 1974 and 2010 (Gauchat, Citation2012). Baker et al. (Citation2020) show that being politically conservative has a negative effect on one’s trust in science in general. Contrarily, political ideology has also been proven to be an inconsistent moderator for trust in science in other studies, and the influence of political ideology on trust cannot always be interpreted based on left versus right political leaning (Brewer & Ley, Citation2013; Hamilton & Safford, Citation2020; Pechar et al., Citation2018).
Political ideology and the related social pressures and elite cues can influence an individual’s trust in or deference to science (Brossard & Nisbet, Citation2006; Gauchat, Citation2012; Hamilton & Safford, Citation2021; Merkley & Stecula, Citation2021). Additionally, an individual’s political beliefs and elite cues from political figures can influence the use and choice of media sources, as well as the uptake, acceptance and internalization of new information found there (Brulle et al., Citation2012; Carmichael & Brulle, Citation2017; Carvalho, Citation2007; Roser-Renouf et al., Citation2009). We therefore analyze political ideology as a moderator for both trust in science and media use.
Using meta-analysis, we aim to create an overview of the available literature on trust in science and media use on public opinion on ACC. From this, we aim to infer if there is an overall positive or negative effect of these parameters on public opinion on ACC. More specifically, we aim to answer if (1) a higher level of trust in science is related to an increase in beliefs about ACC that are in line with the broad scientific consensus on ACC’s definition and solutions, defined as “pro-social beliefs” by Diehl et al. (Citation2019). We also ask the question if that trust is moderated by either political ideology or nationality. These analyses will add to a clearer understanding of the role of political ideology in trust in science and in turn public opinion on ACC. Secondly, we aim to answer (2) if there is a relationship between media use and pro-social ACC beliefs in general. In particular, we will identify whether there is a difference in pro-social ACC beliefs between the use of new (post-internet) and user-generated media versus traditional (pre-internet) and centralized media, and whether political views or nationality moderate this relationship.
Methodology
A priori power analysis
Before identifying the relevant reports, we performed an a priori power analysis (cf. Jackson & Turner, Citation2017), using the R package metapower (Griffin, Citation2020), to know how many effect sizes (k) would be enough to achieve the standard level of power (.80). We assumed three levels of heterogeneity in the meta-analysis model: low (25%), medium (50%), and high (75%; cf. Higgins & Green, Citation2020); two effect sizes: low (r = .10) and medium (r = .30); two groups of studies with k = 10, and k = 20; we assumed that average sample size per study was 100. For the low effect size, we never achieved the required minimum power if r = .10 and k = 10; however, if k = 20, the achieved power was at least .80 if heterogeneity was .25 and .50. For the medium effect size, we achieved the required minimum power for each k and each level of heterogeneity (see Appendix 1 for details). We concluded that the minimum required sample size of coded effect sizes was 10. All the appendices, data sets, and analysis code are available on the Open Science Framework (OSF) at https://osf.io/w3syv/.
Systematic literature research
Identification of reports
We collected two different sets of reports: articles investigating (1) the relationships between trust in science and public opinion on ACC, and (2) the relationships between types of media used and public opinion on ACC. Items were retrieved from Scopus and Web of Science (WoS) published until February 2021. For each data set, we systematically collected papers based on a search of title, abstract and keywords with a fixed search query, including many synonyms for each concept (we used the overarching term “scientific authority” for the dataset to include “trust in science,” “trust in scientists” or “confidence in scientists,” etc.); see Appendix 2 for the queries. We identified 65 (Scopus) plus 43 (WoS) for scientific authority (SA), and 271 (Scopus) and 108 items (WoS) for media use (MU). For both concepts, the results of the two databases were combined and duplicates removed. Additional reports were added via snowballing and going through the reference lists of included articles and publications of included authors. Additionally, in 2016, Hornsey et al. performed an extensive meta-analysis on “determinants and outcomes of belief in climate change.” The studies included in their analysis were checked for suitability for ours. However, we found significant differences in search methodology and definitions used by Hornsey et al., which makes their analysis not as comparable as it may seem at first. From their included studies, three were in line with our definitions and selection criteria, but unfortunately, the reported effect sizes were not directly suitable, and could not be retrieved after repeated contact with the respective authors. These papers were therefore not included in the statistical analysis.
Selection of relevant reports
Relevant reports were selected in two rounds and based on a pre-determined set of inclusion criteria. The first round only selected reports based on the contents of the abstract, using the online collaborative platform Rayyan (Ouzzani et al., Citation2016). The second round contained a further selection based on the full content of each report. Most importantly, the included reports were required to be based on a measure of public opinion (e.g. a survey, poll/panel), to be observational (no pre and post-test conditions), and to focus on the relationship between either SA or MU and opinion on ACC in general. Opinion on ACC was conceptualized in several different ways, e.g. awareness of, worry about, or attitude towards ACC. In our analysis, these diverse conceptualizations were considered equivalent expressions of opinion on ACC as in line with scientific consensus, as the number of the selected reports was too low to split out the types of conceptualizations in our models. To make sure that the effect sizes were measuring a person’s opinion on global climate change and not on one or more specific (future or local) effects of climate change, we excluded ACC reports investigating opinions about specific consequences of ACC (e.g. flooding events). Decisions whether to include the identified report were made by two referees. Kappa scores for consistency were 0.937 for SA and 0.975 for MU what indicates very high consistency in decisions. Each discrepancy was discussed. Ultimately, for statistical analysis, the SA database consisted of 13 studies with 46,359 participants, the MU database contained 12 studies with 15,116 participants. and represent Preferred Reporting Items for Systematic Reviews and Meta-Analyses (PRISMA; Page et al., Citation2021) flow charts. Subsequently, relevant information was coded into a data set by a single coder, with a separate row for each reported effect size.
Meta-analysis
Included variables
Other characteristics of the studies were coded into respective database, including: nationality, sample size, political ideology mean and standard deviation (SD) (if available), the method of measurement of SA or MU, media-type (e.g. newspaper, TV), the type of ACC opinion conceptualization (e.g. believe in, awareness of), the original statistic in each report and its standard error (SE), p-value, and the original effect size.
Calculation of effect sizes
For this study, we used Pearson’s r as an effect size. All the included articles reported either correlations, regression coefficients, or other multivariate models. For a sound meta-analysis, zero-order, or unstandardized correlations (which were deemed a good estimation of r) were required for each reported effect (Peterson & Brown, Citation2005; Roth et al., Citation2017). For SA, multiple reports provided more than one effect size, for instance when multiple conceptualizations of ACC were used (e.g. “ACC belief” as well as well as “awareness of ACC”). Hence studies could be represented by multiple effect sizes in the database that were based on one data set. To avoid overrepresentation of these studies in the overall analysis, these effect sizes were aggregated, averaged, and hence reflected in one combined effect size (cf. Borenstein et al., Citation2009; Lipsey & Wilson, Citation2001).
Unfortunately, many MU articles reported only the standardized regression coefficients and did not include unstandardized correlation table. In cases where only standardized beta-values or other regression results were available, authors were contacted to provide zero-order correlations or provide the raw data for calculation of zero-order correlations (Pearson’s r). Unfortunately, almost half of the reports could not provide suitable data for analysis and were therefore excluded from the statistical analysis. We provide a comparison of the included and excluded papers in Appendix 2.
In order to account for media source, we categorized media types as detailed as the reports could provide: (1) TV, (2) newspaper in print, (3) online newspaper, (4) blog, (5) internet, (6) radio, (7) social media, (8) mixed. We coded the effect size per type, which resulted in multiple effect sizes per study. The dependencies between these effect sizes were controlled for in the main analyses. Media type was subsequently coded into two different binary categories and analyzed as moderators: (1) traditional vs. new media, meaning media that existed before the internet (e.g. radio) and media that did not (e.g. digital newspapers); (2) centralized vs. user-generated media, meaning media that have centralized content creation (e.g. print and digital newspapers, radio, TV), or de-centralized content that is generated by its users (e.g. social media, blogs, Wikipedia).
Moderators coding and data-analysis
For the meta-analysis, we used the metafor R package to fit fixed and random-effects meta-analysis models (Viechtbauer, Citation2010). We also used R packages clubSandwich (Pustejovsky, Citation2022), dmetar (Harrer et al., Citation2019), meta (Balduzzi et al., Citation2019), orchaRd (Nakagawa et al., Citation2021), psych (Revelle, Citation2022), readr (Wickham et al., Citation2022), robumeta (Fisher et al., Citation2017), tidyverse (Wickham et al., Citation2019), and weightr (Coburn & Vevea, Citation2019).
In the MU dataset, not all the articles reported the same number of media sources. Some articles only reported results for two media sources (hence two effect sizes), others for six or more sources. We used non-aggregated data in the analysis since aggregation would lose us the opportunity to look into the moderating effect of the various media sources. We therefore used a three-level random-effects (multilevel) meta-analysis (Pastor & Lazowski, Citation2018), consisting of a basic model, a model that took into account sampling variance (level 1), within-study variance (level 2), and between-study variance (level 3) by means of metafor. The heterogeneity levels were calculated for each level. In order to estimate whether multilevel analysis was a suitable approach, we analyzed the contribution of the multilevel model to explanation of the within and between-study variance. For both datasets, nationality and political ideology were coded into binary values (USA/Non-USA, liberal/conservative) and analyzed as moderators.
Additionally, the MU data set gave us the opportunity to code nationality to a continental level for moderator analysis.
Assessing and adjusting for publication bias and sensitivity analysis
To assess publication bias, p-curve analysis was carried out on non-aggregated data (for the MU data set); however, for further analyses it was necessary to aggregate the MU data set completely as multilevel meta-analysis function of metafor does not allow to use more techniques on non-aggregated data. In consequence, for SA and MU data sets, a funnel plot, and Egger’s test (Egger et al., Citation1997) of funnel plot asymmetry, trim-and-fill analysis (Duval & Tweedie, Citation2000), a selection models analysis (Vevea & Hedges, Citation1995), and a PET-PEESE adjustment (Stanley & Doucouliagos, Citation2014) were performed. To test the sensitivity of our models, GOSH analysis (Olkin et al., Citation2012), and leave-one-out and outlier analyses (Harrer et al., Citation2022) were performed.
Results
Study characteristics
The systematic literature research for SA resulted in 13 reports with suitable data for the meta-analysis, representing 18 effect sizes. A few reports were excluded after the initial search, mostly because the trust in scientist turned out not to be part of the statistical analysis, intervention methods were used, or there was a lack of data availability. See Appendix 2 for the selection criteria used in this research and an overview of excluded reports and reasons for exclusion (see for details). The MU systematic research returned 12 reports that were suitable for further statistical analysis. Unfortunately, many reports had to be excluded after the initial search due to an overall lack of available data (see for details). For both data sets, many authors were contacted multiple times, but in many instances, this did not lead to access to effect sizes or data sets. Ten MU reports that turned up in the search did initially provide data on the relationships between MU and ACC opinion, but the types of provided data ((un)standardized beta-values) were unsuitable for meta-analysis and unfortunately zero-order correlations could not be made available by the authors. In Appendix 2, we provide an overview of the characteristics of these 10 reports that had to be excluded from the MU analysis, versus those of the 12 reports that were included in the meta-analysis.
Publication years of the included SA reports ranged from 2008 to 2020, had a wide range of generally large sample sizes between 306 and 18,785, and the aggregated effect sizes (k = 13) range from –0.32 to 0.43 (84.6% of effect sizes were higher than 0). The countries covered were the USA (n = 8), Switzerland (1), Canada (1), India (1), and a combination of two or more countries (2). For MU, the non-aggregated effect sizes (k = 68) range from –0.19 to 0.27 (69.1% of effect sizes were higher than 0). Publication years of the included reports ranged from 2009 to 2017, the sample sizes ranged between 453 and 3988, and covered the USA (6), Australia (1), South Korea (1), Germany (3), India (1), and China (1). Risk of bias analysis did not show any risks for both collections of papers (for details see Appendix 3; based on McGuinness & Higgins, Citation2020).
Meta-analysis
Trust in science
First, the fixed-effects model was applied to the aggregated data to test the overall effect size of trust in science on pro-social ACC beliefs. A statistically significant positive effect was found, indicating that a higher level of trust in science was associated with more pro-social beliefs (r = 0.230; p < .001). The subsequent random-effects model analysis was also statistically significant, r = 0.193; 95% prediction intervals ranged from –0.272 to 0.657; see for details. Prediction intervals indicate how much the effect sizes can vary (Borenstein, Citation2022). presents the distribution of the effect sizes across the studies. The I2 value indicated that approximately 99% of the heterogeneity in the effects was due to the between-study differences. I2 indicates how much variance of true effects is reflected in the observed variance of the effect sizes (Borenstein, Citation2022), and, according to the Cochrane Collaboration, a value of I2 greater than 75% suggests high heterogeneity (Higgins & Green, Citation2020). Note also that large heterogeneity is generally associated with high effect sizes, not only with large differences between them (Linden & Hönekopp, Citation2021). To cross-validate this analysis on the aggregated data, we also performed an analysis on non-aggregated data (k = 18) by means of the robumeta R package, leading to a significant overall effect size of r = 0.201 (SE = .067), 95% CI [0.056; 0.347], t(12) = 3.010, p = .011, τ2 = .037, I2 = 99.121%.
Figure 3. Forest plot for the random effects model for the effect sizes of trust in science. The values on the bottom indicate the meta-analytical effect size, confidence intervals, and the dashed line reflects the prediction interval.
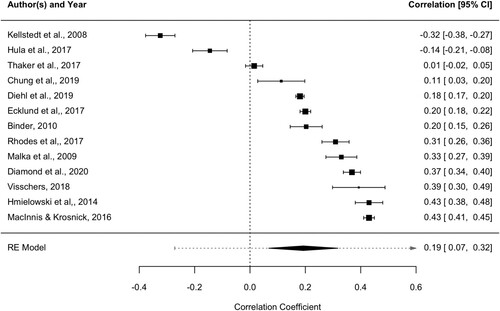
Table 1. Meta-analysis results for trust in science and media use.
A moderator analysis showed that political ideology was not a significant moderator for the meta-analytic effect size, p = .554; . However, the number of studies that contained information on political ideology was low (k = 7), which might be too low to achieve sufficient statistical power for such an analysis. Nationality was also not statistically significant as a moderator. Additionally, publication year was also not significant (see for details).
Table 2. Results moderator analyses for trust in science and media use.
Sensitivity analysis and publication bias
Outlier analysis, by means of a dmetar function, identified 6 outliers and removing these outliers (k = 7) resulted in a significant overall effect size of 0.245, p < .001, I2 = 90.9%; and it was an increase of 23.8% as compared to the initial meta-analytic effect size of 0.193. Based on more advanced outlier analysis (GOSH), we concluded that the impact of the atypical studies is not substantial, so we decided not to exclude them (Appendix 4). This shows that working with the aggregated data and inclusion of the outliers, lead to a more modest estimation of the overall effect size. For full results of the sensitivity and publication bias analysis, we refer to Appendix 5.
presents the publication bias results. The funnel plot was symmetrical – this was supported by a non-significant rank correlation Egger’s test, indicating that the hypothesis of a symmetrical plot cannot be rejected. For details, see (a,b). A selection models analysis was also non-significant, χ2(1) = .142, p = .707. Additionally, the trim-and-fill-analysis revealed no new studies to be added to the right part of the funnel plot but suggested two potential missing studies on the left side of funnel plot (with values close to r = 0); all these findings were a sign of a minor publication bias.
Table 3. Results for publication bias and sensitivity analyses.
Media use
All the MU models were based on non-aggregated data, as our main interest is in the effects of different media sources and aggregating the data per study would lose that information. presents the distribution of the effect sizes across the studies. We used the random-effects model and tested three models: (a) the final model that accounted for the within and between-study variance, (b) a model that accounted only for the within-study variance (level 2), and (c) a model that accounted for the between-study variance (level 3) (see ). The fit statistics (AIC/BIC) for each model were compared. The comparisons indicated that the final model had better fit than the level-2 and level-3 models (–114.77/–108.15 vs. –108.839/–104.430, p = .005; vs. 260.492/264.901, p < .001; respectively).
Figure 5. Forest plot for the random effects model for the effect sizes of media use. The values on the bottom indicate the metaanalytical effect size, confidence intervals, and the dashed line reflects the prediction interval.
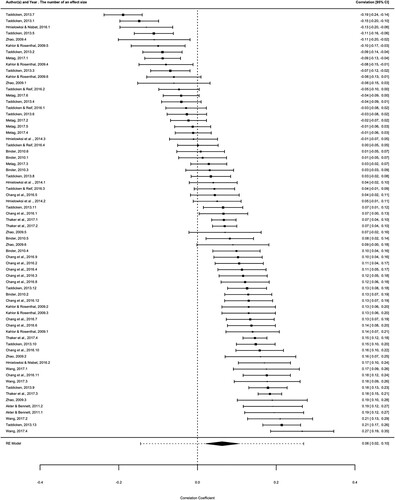
The basic level model showed a small, but significant effect size of r = 0.063; the 95% prediction intervals for the final multilevel model ranged from –0.145 to 0.270 (cf. Schäfer & Schwarz, Citation2019). The level-2 model showed r = 0.057, whereas the level-3 model r = 0.066. The distribution of heterogeneity shows a low level of heterogeneity at level 1, i.e. sampling variance, I2 = 6.930%, most of the heterogeneity resided in the within-study variance (level 2; I2 = 63.309%), and the between-study variance (level 3; I2 = 29.758%). Following Borenstein (Citation2022), the higher the I2 value, the more variance of true effects the observed effects reflect. To cross-validate this multilevel analysis we performed with metafor, we also conducted a random-model analysis on the same data (k = 68) by means of the robumeta R package, leading to a significant overall effect size of r = 0.066, SE = .022, 95% CI [0.018; 0.114], t(11) = 3.000, p = 0.011, τ2 = .010, I2 = 92.094%.
Similar to the SA data set, about half of the included studies provided data on political ideology (k = 33). A moderator analysis showed that political ideology was a non-significant moderator for MU (p = 0.879; see for details). Nationality was a significant moderator of the meta-analytic effect size, but only if North America (r = 0.043) was compared with other continents: Asia (r = 0.133, p = .001), and Australia (r = 0.193, p = .026). The contrast North America vs. Europe (r = –0.004, respectively) was statistically non-significant (p = .074). Additionally, the publication year was a non-significant moderator for the overall effect size ().
We ran analyses with two different divisions of media categories as moderators: (1) traditional vs. new media, and (2) centralized media vs. user-generated media (). The first model was significant, showing that the effect of new media (r = 0.093) on pro-social ACC beliefs is about twice that of traditional media (r = 0.046). In the second model, we found both media types to have a significant positive effect, with user-generated media (r = 0.106) having more than twice the effect size of centralized media (r = 0.049).
Overall, the results showed a small, but significant relationship between MU and pro-social ACC beliefs. This effect was moderated by nationality/continent and media type. The use of media that is classified as new or user-generated have about twice the effect on pro-social ACC beliefs than traditional or centralized media, i.e. using new or user-generated media makes it slightly more likely for someone to have an opinion on ACC that is in line with scientific consensus.
Sensitivity analysis and publication bias
The analysis showed 2 studies and removing these outliers (trimmed k = 10) resulted in a significant, but smaller overall effect size of r = 0.040, with lower heterogeneity, I2 = 78.8%. As for trust in science, the GOSH analysis showed no impact of atypical studies, and hence no support for excluding those studies (Appendix 4).
The analyses for assessing publication bias show no substantial sign of publication bias (). The symmetry of the funnel plot was supported by a non-significant Egger’s test; see (a,b) for details. The trim-and-fill-analysis added two potential missing studies to the right part of the plot, but no studies were added to the left part of the plot. Moreover, the selection models analysis was statistically significant, χ2(1) = 4.335, p = .037, suggesting a lack of studies with higher effect sizes. These findings show no indication of publication bias defined as a potential lack of publication of null findings (close to r = 0).
Discussion
The analyses of trust in science (r = 0.19) and media use (r = 0.06) showed a significant, positive effect size for pro-social ACC beliefs, and for media use both nationality and media category proved to be significant moderators. New and user-generated media have an effect size almost twice that of traditional and centralized media. It is important to note that due to a wide variety of definitions and operationalizations used in the included reports, we had to put together slightly different interpretations of climate change opinion, trust in science, and media use to have enough effect sizes for statistical analysis. While this may have created some noise in the data sets, we still find significant effects in both of them, signaling that these patterns are robust, and they give a general idea of the effects of media use and trust in science on pro-social ACC beliefs.
Trust in science
The positive effects we found of the trust in science or scientists on pro-social ACC beliefs, mirrors similar effects that have been reported in literature on different scientific subjects, for instance in attitudes towards nano-technology, biotechnology, GM foods, stem cell research, and the acceptance of science and technology in general (Anderson et al., Citation2012; Brossard & Nisbet, Citation2006; Lang & Hallman, Citation2005; Lee & Scheufele, Citation2006; Liu & Priest, Citation2009; Lewandowsky et al., Citation2012). There is evidence that trust in scientists could be a moderator of the consensus effect in climate change, and that higher perceived levels of expertise and trustworthiness improve the credibility of scientific communicators (Fiske & Dupree, Citation2014; Van der Linden, Citation2021). In turn, it has been shown that a perception of scientific consensus increases the acceptance of human-mediated climate change and that this in turn may increase support for ACC mitigating policies (Lewandowsky et al., Citation2012; Van der Linden et al., Citation2015). Brick et al. (Citation2021) showed that scientists are of great importance in helping people understand the mechanisms of climate change and the impact of ACC as this understanding can be one of the major drives of the changes in people’s behavior contributing to ACC (cf. Hornsey & Lewandowsky, Citation2022).
Reports from the USA turned out to be overrepresented in the scientific literature and hence in our data set: more than 50% of the studies included for trust in science were USA-based, and as a result the nationality moderator could only be interpreted in the USA versus the rest of the world context, as there were not enough effect sizes available on the other countries included in the data set. However, our results show that nationality did not moderating the relationship between trust in science and pro-social ACC beliefs.
Political ideology was not a significant moderator for pro-social ACC beliefs in this study. This was unexpected, but, as mentioned, this may be explained by the lack of available effect sizes for this study. Other studies have shown that trust in scientists is issue-specific in the case of climate change, and that more right leaning, and socially conservative people tend to believe that there is lower scientific consensus on ACC and to be generally less accepting of scientific authority (Cook et al., Citation2018; Diehl et al., Citation2019). A more conservative or liberal political ideology is associated with certain ideas and preferences with regard to government interference, regulations, (individual) freedom, and reliance on science. Research shows that conservatives have higher trust in science than liberals or progressive-thinkers, and that conservatives are likely (more than liberals) to have more trust in science on technological and economic production innovations, than trust in science that identifies negative effects of economic production, like decreased public health or environmental effects (McCright et al., Citation2013). Conservative trust is also influenced by the extent to which a person thinks government policy should be based on science to begin with (Gauchat, Citation2015; Hamilton, Citation2015 McCright et al., Citation2013;). Other pre-existing ideological beliefs, like religion and nationalist ideology, may also be important factors influencing the dynamic between political ideology and trust in science (Baker et al., Citation2020; Kulin et al., Citation2021).
Media use
One media source is not necessarily always more in line with scientific consensus on ACC than the other, and media serve both as a stage as well as a watchdog for ACC contrarian voices (Boykoff, Citation2008; Kozyreva et al., Citation2020). The effect size of media use on pro-social ACC beliefs was small, but statistically significant. So, despite the wide variety of media and countries in this study, with probably different subjects and frames, being covered by different content creators and editors that make different decisions and create media for different reasons (e.g. news value, raising awareness, or persuasion), we still found a significant effect, indicating that the effect is consistent and robust across these differences (Boykoff, Citation2008; Nerlich et al., Citation2010).
However, the moderator analysis showed a significant difference between traditional and new media, and more importantly between user-generated and centralized media. Contrary to our expectations, the positive effect of consumption of user-generated media on pro-social ACC beliefs was twice as large when compared to consumption of centralized content. Whereas centralized content is created by professional journalists and editors, often aiming to adhere to journalistic norms, like objectivity, fairness, accuracy, balance (Boykoff & Boykoff, Citation2004), user-generated content is generally thought of as free from these “rules,” more unfiltered, and allows more freedom to the creator (Kozyreva et al., Citation2020). However, these inherent journalistic rules apparently do not lead to media content that is necessarily better at enforcing scientifically valid ACC opinions than user-generated content. As the “propaganda model” from Herman and Chomsky (Citation1988) indicates, journalists and centralized media content are often influenced by market forces, economic incentives like advertisement revenue, and ownership (Edwards & Cromwell, Citation2006 Herman & Chomsky, Citation1988;). Until the early 2000s the journalistic norm of “balanced reporting” has actually caused a misbalanced view of ACC in centralized media (i.e. false balance) (Boykoff & Boykoff, Citation2004, Citation2007). However, later research has shown how journalism is moving away from this and towards better representations (Brüggemann & Engesser, Citation2017; Schmid-Petri et al., Citation2017).
It is sometimes assumed that online and especially user-generated sources on climate change suffer more often from information with lower journalistic quality, less fact-checks, or often lack clearly stated or epistemically valid sources (Kozyreva et al., Citation2020). Despite the general tendency to assume that the use of social media, blogs, and other user-generated content leads to a potentially flawed understanding of the role of anthropogenic factors in driving climate change, this meta-analysis shows that taken together, the use of user-generated media is positively and more strongly correlated to pro-social ACC beliefs than centralized, more “controlled” media. Further research is needed to indicate if this is due to whether audiences that are already more informed on climate change use user-generated sources more often than the less-knowledgeable part of the public, or whether online sources are better in conveying climate change information, despite the presence of incorrect information.
With regard to the opportunities of user-generated media to convey information to the public, possible factors that could play a role, are first the more interactive and personal communication on user-generated media, that resemble aspects of interpersonal communication, which has been shown to have stronger effects on awareness and knowledge than traditional news media (Schäfer, Citation2012). In addition, research has shown that social media are more persuasive in bringing across information, perhaps adding to the retention of content encountered there (Chen et al., Citation2021). Second, content on social media can make ACC feel more proximate (Anderson, Citation2017; Rosenthal, Citation2022). Psychological distance has proven to be a barrier to creating awareness and behavioral change with regard to ACC (Clayton, Citation2019 Jones et al., Citation2017; Kruse, Citation2011; Leiserowitz, Citation2005;). Content on social media is already filtered and adapted to personal networks and preferences before it reaches the user. Therefore, information on ACC may also be filtered to an extent that it shows causes, effects, circumstances, and social context that make it more personal to the user and decreases psychological distance from the issue (Harris et al., Citation2018 Myers et al., Citation2012; Spence et al., Citation2012;). Third, there is the possibility that the effects of “echo-chambers” and “filter bubbles” on social media platforms may not be as important as previously expected (Bruns, Citation2019; Tuitjer & Dirksmeier, Citation2021). There is some evidence for the existence of echo chambers on X (previously Twitter), but research also points out that this does not translate to Facebook, and studies into echo chambers on single platforms limit the generalizability (Dubois & Blank, Citation2018). It is only one platform among many that research subjects are able to interact with, leading to a possibly much more varied media diet, which in turn helps to limit the effect of echo chambers (Bruns, Citation2019 Dubois & Blank, Citation2018; Tuitjer & Dirksmeier, Citation2021;).
It is generally assumed that a media source is either more politically progressive or conservative in its stance on ACC, based on the ideology of their editorial board or policies (Brüggemann & Engesser, Citation2017; Schmid-Petri et al., Citation2017). Even though our study did not show significant moderating effects of political ideology, literature shows that political ideology can influence personal media choice via agenda setting, elite cues, and in-group/out-group thinking (Diehl et al., Citation2019; Merkley & Stecula, Citation2021). However, our results show that the effect size for conservatives is only slightly higher than that of liberals and statistically non-significant. This indicates that a person who adheres a certain political ideology and chooses their media sources accordingly, does not necessarily get a one-sided or skewed view on ACC.
Nationality/continent proved to be a significant moderator for most continents, except Europe. We want to point out that for Australia there were only two effect sizes from the same report, making this result not very reliable. Nevertheless, it is interesting to see that effect sizes differed significantly between continents, probably due to how media-landscapes and ACC coverage are not identical between countries, and differences in how opinion on ACC differs on a national level to begin with (Arnold et al., Citation2016 Capstick et al., Citation2015; Ratter et al., Citation2012;).
Representing scientific consensus in media
It is important to consider the role of trust in science in climate policy and the representation of scientific authority in media, as there is increasing tension between the authority of science, political authority, and public opinion. Trust in media also plays an important role, as trust in where a message is coming from can influence how its content is taken up and internalized (Fawzi et al., Citation2021 Strömbäck et al., Citation2020; Zerva et al., Citation2020;). This can apply to media types, but also specific outlets, and can differ between topics (Roser-Renouf et al., Citation2009; Tsfati et al., Citation2022).
As other studies have shown, media do have the power to make or break the public’s view on ACC (Boykoff, Citation2008; Boykoff & Boykoff, Citation2007; Koehler, Citation2016). ACC is framed in public outreach via mass media and used to attract diverse groups of voters along the political spectrum, while the scientific consensus is not necessarily followed or properly addressed (Brown & Havstad, Citation2016 McCright et al., Citation2013;). The lack of a sense of urgency among the public and politics about this issue is to a large part due to the fact that mainstream media still do not give fully accurate representations of ACC and its serious consequences, the most effective solutions, and the scientific consensus (Feldman et al., Citation2017; Moser, Citation2016; Petersen et al., Citation2019). For instance, this is done by using visuals that underplay the seriousness of ACC (DiFrancesco & Young, Citation2010; Wang et al., Citation2017). Additionally, consistently communicating effective solutions like phasing out fossil fuels or cutting down flying can drive away important corporate advertisers on which media depend for revenue. ACC remains a low priority in many countries, partly because of a lack of public concern and hence demand for better policies. A consistent and accurate representation of the existing consensus among scientists can improve overall ACC beliefs and support for mitigating policies, especially when represented in visuals and in a personal way, showing individual scientists, and using user-generated, social media (Bayes et al., Citation2020 Dixon et al., Citation2015; Harris et al., Citation2018; Huber et al., Citation2019;).
As scientific authority, trust in science, and the perception of scientific consensus can improve support for ACC mitigating policies, it is important for media to be aware about how they represent these to their public (Ding et al., Citation2011; Lewandowsky et al., Citation2012; Van der Linden et al., Citation2015). Especially in the context of ACC, the policies and mitigation measures advised and supported by science are sometimes in conflict with politicians’ priorities, such as promoting a “healthy” (growing, fossil fuel-based) economy and maintaining high public popularity to ensure re-election. Moreover, politicians are subject to influences and pressures from lobbyists, for instance, coming from the fossil fuel industry (Brown & Havstad, Citation2016; Brulle, Citation2018; Graham et al., Citation2020 Litfin, Citation2000; Victor, Citation2009;). Science-based ACC mitigation policies challenge the societal and political status quo, which is based on libertarian values (e.g. a capitalist focus on economic growth, overconsumption, and personal freedom) (Barnhart & Mish, Citation2017; Kantartzis & Molineux, Citation2011; Lewandowsky, Citation2021 McCright et al., Citation2013; Peck & Tickell, Citation2002; Sewpaul, Citation2015;), leading to politicization of the problem.
Recommendations for future research
The lack of available and suitable data among the media use literature unfortunately forced us to discard some relevant papers for the statistical analysis. As a recommendation for further research, we want to first advocate for better management and sharing of data. Many data sets that could have been of value for this research were either lost, inaccessible, or not documented well enough to be useful. Also, the publication of zero-order correlations, underlying the more elaborate regression or SEM models that are often published, are very valuable for further (meta-)analyses, but were often not made available. Second, the overrepresentation of the USA and other Western countries and additionally the high focus on traditional, printed media in media research limits the scope of these fields (Schäfer & Schlichting, Citation2014). We would therefore like to encourage future research to focus on a wider diversity of countries, and more on new and user-generated media, as these media-types are becoming more and more relevant as the main road for science communication.
Conclusion
In this paper, we have presented a meta-analysis of the correlations between trust in science, media use, and people’s opinion about ACC. We found a positive relationship between trust in science and pro-social ACC beliefs. Similarly, media use had a positive relationship with pro-social ACC beliefs, which was moderated by nationality and media category. For user-generated online media we obtained a higher effect size of pro-social ACC opinions when compared to media based on centralized content creation. Despite assumptions often made about social media, user-generated media were positively correlated with an opinion on ACC that is in line with scientific consensus. The interplay between how media show and explain the issue of climate change, on what channels, and in particular how much attention is paid to communicating the scientific consensus on the existence and urgency of this problem, is an important element in converging scientists’ and public opinion on ACC and closing the consensus gap.
Supplementary Material
Download Zip (4 MB)Disclosure statement
No potential conflict of interest was reported by the author(s).
References
- *= part of meta-analysis database.
- *Akter, S., & Bennett, J. (2011). Household perceptions of climate change and preferences for mitigation action: The case of the carbon pollution reduction scheme in Australia. Climatic Change, 109(3), 417–436. https://doi.org/10.1007/s10584-011-0034-8
- Almiron, N., Boykoff, M., Narberhaus, M., & Heras, F. (2020). Dominant counter-frames in influential climate contrarian European think tanks. Climatic Change, 162(4), 2003–2020. https://doi.org/10.1007/s10584-020-02820-4
- Anderson, A. A. (2017). Effects of social media use on climate change opinion, knowledge, and behavior. Oxford Research Encyclopedia of Climate Science. https://doi.org/10.1093/acrefore/9780190228620.013.369
- Anderson, A. A., Scheufele, D. A., Brossard, D., & Corley, E. A. (2012). The role of media and deference to scientific authority in cultivating trust in sources of information about emerging technologies. International Journal of Public Opinion Research, 24(2), 225–237. https://doi.org/10.1093/ijpor/edr032
- *Arlt, D., Hoppe, I., Schmitt, J. B., De Silva-Schmidt, F., & Brüggemann, M. (2018). Climate engagement in a digital age: Exploring the drivers of participation in climate discourse online in the context of COP21. Environmental Communication, 12(1), 84–98. https://doi.org/10.1080/17524032.2017.1394892
- *Arlt, D., Hoppe, I., & Wolling, J. (2011). Climate change and media usage: Effects on problem awareness and behavioural intentions. International Communication Gazette, 73(1–2), 45–63. https://doi.org/10.1177/1748048510386741
- Arnold, A., Böhm, G., Corner, A., Mays, C., Pidgeon, N., Poortinga, W., Poumadère, M., Scheer, D., Sonnberger, M., Steentjes, K., & Tvinnereim, E. (2016). European perceptions of climate change. Socio-political profiles to inform a cross-national survey in France, Germany, Norway and the UK. Climate Outreach.
- Baker, J. O., Perry, S. L., & Whitehead, A. L. (2020). Crusading for moral authority: Christian nationalism and opposition to science. Sociological Forum, 35(3), 587–607. https://doi.org/10.1111/socf.12619
- Balduzzi, S., Rücker, G., & Schwarzer, G. (2019). How to perform a meta-analysis with R: A practical tutorial. Evidence-Based Mental Health, 22(4), 153–160. https://doi.org/10.1136/ebmental-2019-300117
- Barnhart, M., & Mish, J. (2017). Hippies, hummer owners, and people like me: Stereotyping as a means of reconciling ethical consumption values with the DSP. Journal of Macromarketing, 37(1), 57–71. https://doi.org/10.1177/0276146715627493
- Baum, M. A., & Potter, P. B. K. (2008). The relationships between mass media, public opinion, and foreign policy: Toward a theoretical synthesis. Annual Review of Political Science, 11(1), 39–36. https://doi.org/10.1146/annurev.polisci.11.060406.214132
- Bayes, R., Bolsen, T., & Druckman, J. N. (2020). A research agenda for climate change communication and public opinion: The role of scientific consensus messaging and beyond. Environmental Communication, https://doi.org/10.1080/17524032.2020.1805343
- *Binder, A. R. (2010). Routes to attention or shortcuts to apathy? Exploring domain-specific communication pathways and their implications for public perceptions of controversial science. Science Communication, 32(3), 383–411. https://doi.org/10.1177/1075547009345471
- Bloomfield, E. F., & Tillery, D. (2019). The circulation of climate change denial online: Rhetorical and networking strategies on Facebook. Environmental Communication, 13(1), 23–34. https://doi.org/10.1080/17524032.2018.1527378
- *Bolin, J. L., & Hamilton, L. C. (2018). The news you choose: News media preferences amplify views on climate change. Environmental Politics, 27(3), 455–476. https://doi.org/10.1080/09644016.2018.1423909
- Borenstein, M. (2022). In a meta-analysis, the I-squared statistic does not tell us how much the effect size varies. Journal of Clinical Epidemiology, https://doi.org/10.1016/j.jclinepi.2022.10.003
- Borenstein, M., Hedges, L. V., Higgins, J. P. T., & Rothstein, H. R. (2009). Introduction to meta-analysis. John Wiley. ISBN:9780470057247.
- Boykoff, M. T. (2008). Media and scientific communication: A case of climate change. Communicating Environmental Geoscience, Geological Society, London, Special Publications, 305(1), 11–18. https://doi.org/10.1144/SP305.3
- Boykoff, M. T., & Boykoff, J. M. (2004). Balance as bias: Global warming and the US prestige press. Global Environmental Change, 14(2), 125–136. https://doi.org/10.1016/j.gloenvcha.2003.10.001
- Boykoff, M. T., & Boykoff, J. M. (2007). Climate change and journalistic norms: A case-study of US mass-media coverage. Geoforum; Journal of Physical, Human, and Regional Geosciences, 38(6), 1190–1204. https://doi.org/10.1016/j.geoforum.2007.01.008
- Brewer, P. R., & Ley, B. L. (2013). Whose science do you believe? Explaining trust in sources of scientific information about the environment. Science Communication, 35(1), 115–137. https://doi.org/10.1177/1075547012441691
- Brick, C., Bosshard, A., & Whitmarsh, L. (2021). Motivation and climate change: A review. Current Opinion in Psychology, 42, 82–88. https://doi.org/10.1016/j.copsyc.2021.04.001
- Brossard, D. (2013). Science and new media landscapes. Proceedings of the National Academy of Sciences Aug, 110(3), 14096–14101. https://doi.org/10.1073/pnas.1212744110
- Brossard, D., & Nisbet, M. C. (2006). Deference to scientific authority among a low information public: Understanding U.S. Opinion on Agricultural Biotechnology, International Journal of Public Opinion Research, 19(1), 24–52. https://doi.org/10.1093/ijpor/edl003
- Brown, M. J., & Havstad, J. C. (2016). The disconnect problem, scientific authority, and climate policy. Perspectives on Science, 25(1), 67–94. https://doi.org/10.1162/POSC_a_00235
- Brownlee, M. T., Powell, R. B., & Hallo, J. C. (2013). A review of the foundational processes that influence beliefs in climate change: Opportunities for environmental education research. Environmental Education Research, 19(1), 1–20. https://doi.org/10.1080/13504622.2012.683389
- Brüggemann, M., & Engesser, S. (2017). Beyond false balance: How interpretive journalism shapes media coverage of climate change. Global Environmental Change, 42, 58–67. https://doi.org/10.1016/j.gloenvcha.2016.11.004
- Brulle, R. J. (2018). The climate lobby: A sectoral analysis of lobbying spending on climate change in the USA, 2000 to 2016. Climatic Change, 149(3-4), 289–303. https://doi.org/10.1007/s10584-018-2241-z
- Brulle, R. J., Carmichael, J., & Jenkins, J. G. (2012). Shifting public opinion on climate change: An empirical assessment of factors influencing concern over climate change in the U.S., 2002–2010. Climatic Change, 114(2), 169–188. https://doi.org/10.1007/s10584-012-0403-y
- Bruns, A. (2019). Filter bubble. Internet Policy Review, 8(4), https://doi.org/10.14763/2019.4.1426
- Capstick, S., Whitmarsh, L., Poortinga, W., Pidgeon, N., & Upham, P. (2015). International trends in public perceptions of climate change over the past quarter century. WIRES Climate Change, 6(4), 435–435. https://doi.org/10.1002/wcc.343
- Carmichael, J. T., & Brulle, R. J. (2017). Elite cues, media coverage, and public concern: An integrated path analysis of public opinion on climate change, 2001–2013. Environmental Politics, 26(2), 232–252. https://doi.org/10.1080/09644016.2016.1263433
- Carvalho, A. (2007). Ideological cultures and media discourses on scientific knowledge: Re-reading news on climate change. Public Understanding of Science, 16(2), 223–243. https://doi.org/10.1177/0963662506066775
- *Chang, J. J. C., Kim, S., Shim, J. C., & Ma, D. H. (2016). Who is responsible for climate change? Attribution of responsibility, news media, and south Koreans’ perceived risk of climate change. Mass Communication and Society, 19(5), 566–584. https://doi.org/10.1080/15205436.2016.1180395
- Chen, S., Xiao, L., & Mao, J. (2021). Persuasion strategies of misinformation-containing posts in the social media. Information Processing & Management, 58(5), https://doi.org/10.1016/j.ipm.2021.102665
- *Chung, M. G., Kang, H., Dietz, T., Jaimes, P., & Liu, J. (2019). Activating values for encouraging pro-environmental behavior: The role of religious fundamentalism and willingness to sacrifice. Journal of Environmental Studies and Sciences, 9(4), https://doi.org/10.1007/s13412-019-00562-z
- Clayton, S. (2019). Psychology and climate change. Current Biology, 29(19), R942–R995. https://doi.org/10.1016/j.cub.2019.09.020
- Coburn, K., & Vevea, J. L. (2019). Weightr: Estimating weight-function models for publication bias. https://CRAN.R-project. org/package=weightr.
- Cook, J., Nuccitelli, D., Green, S. A., Richardson, M., Winkler, B., Painting, R., Way, R., Jacobs, P., & Skuce, A. (2013). Quantifying the consensus on anthropogenic global warming in the scientific literature. Environmental Research Letters, 8(2), https://doi.org/10.1088/1748-9326/8/2/024024
- Cook, J., Oreskes, N., Doran, P., Anderegg, W., Verheggen, B., Maibach, E., Carlton, J., Lewandowsky, S., Skuce, A., Green, S., Nuccitelli, D., Jacobs, P., Richardson, M., Winkler, B., Painting, R., & Rice, K. (2016). Consensus on consensus: A synthesis of consensus estimates on human-caused global warming. Environmental Research Letters, 11(4)), https://doi.org/10.1088/1748-9326/11/4/048002
- Cook, J., van der Linden, S., Maibach, E., & Lewandowsky, S. (2018). . The consensus handbook. https://doi.org/10.13021/G8MM6P.
- *Diamond, E., Bernauer, T., & Mayer, F. (2020). Does providing scientific information affect climate change and GMO policy preferences of the mass public? Insights from survey experiments in Germany and the United States. Environmental Politics, 29(7), 1199–1218. https://doi.org/10.1080/09644016.2020.1740547
- *Diehl, T., Huber, B., Gil de Zúñiga, H., & Liu, J. (2019). Social media and beliefs about climate change: A cross-national analysis of news use, political ideology, and trust in science. International Journal of Public Opinion Research, 33(2), 197–213. https://doi.org/10.1093/ijpor/edz040
- DiFrancesco, A. D., & Young, N. (2011). Seeing climate change: The visual construction of global warming in Canadian national print media. Cultural Geographies, 18(4), 517–536. https://doi.org/10.1177/1474474010382072
- Ding, D., Maibach, E., Zhao, X., Roser-Renouf, C., & Leiserowitz, A. (2011). Support for climate policy and societal action are linked to perceptions of scientific agreement. Nature Climate Change, 1(9), 462–466. https://doi.org/10.1038/NCLIMATE1295
- Dixon, G. N., McKeever, B. W., Holton, A. E., Clarke, C., & Eosco, G. (2015). The power of a picture: Overcoming scientific misinformation by communicating weight-of-evidence information with visual exemplars. Journal of Communication, 65(4), 639–659. https://doi.org/10.1111/jcom.12159
- Doran, P. T., & Zimmerman, M. K. (2011). Examining the scientific consensus on climate change. Eos Transactions AGU, 90(3), 22–23. https://doi.org/10.1029/2009EO030002
- Dubois, E., & Blank, G. (2018). The echo chamber is overstated: The moderating effect of political interest and diverse media, information. Communication & Society, 21(5), 729–745. https://doi.org/10.1080/1369118X.2018.1428656
- Dunlap, R. E., & Brulle, R. J. (2020). Sources and amplifiers of climate change denial. In D. C. Holmes & L. M. Richardson (Eds.), Research handbook on communicating climate change (pp. 49–61). Edward Elgar. ISBN:9781789900392
- Duval, S., & Tweedie, R. (2000). A nonparametric “trim and fill” method of accounting for publication bias in meta-analysis. Journal of the American Statistical Association, 95(449), 89–98. https://doi.org/10.2307/2669529
- *Ecklund, E. H., Scheitle, C. P., Peifer, J., & Bolger, D. (2017). Examining links between religion, evolution views, and climate change skepticism. Environment and Behavior, 49(9), 985–1006. https://doi.org/10.1177/0013916516674246
- Edwards, D., & Cromwell, D. (2006). Guardians of power: The myth of the liberal media. Pluto Press. ISBN:9780745324821.
- Egger, M., Smith, G. D., Schneider, M., & Minder, C. (1997). Bias in meta-analysis detected by a simple, graphical test. The BMJ, 315(7109), 629–634. https://doi.org/10.1136/bmj.315.7109.629
- Eom, K., Papadakis, V., Sherman, D. K., & Kim, H. S. (2019). The psychology of proenvironmental support: In search of global solutions for a global problem. Current Directions in Psychological Science, 28(5), 490–495. https://doi.org/10.1177/0963721419854099
- Fairbrother, M., Johansson Sevä, I., & Kulin, J. (2019). Political trust and the relationship between climate change beliefs and support for fossil fuel taxes: Evidence from a survey of 23 European countries. Global Environmental Change, 59, 102003. https://doi.org/10.1016/j.gloenvcha.2019.102003
- Fawzi, N., Steindl, N., Obermaier, M., Prochazka, F., Arlt, D., Blöbaum, B., Dohle, M., Engelke, K. M., Hanitzsch, T., Jackob, N. & Jakobs, I. (2021). Concepts, causes and consequences of trust in news media – a literature review and framework. Annals of the International Communication Association, 45(2), 154–174. https://doi.org/10.1080/23808985.2021.1960181
- Feldman, L., Hart, P. S., & Milosevic, T. (2017). Polarizing news? Representations of threat and efficacy in leading US newspapers’ coverage of climate change. Public Understanding of Science, 26(4), 481–497. https://doi.org/10.1177/0963662515595348
- *Feldman, L., Myers, T. A., Hmielowski, J. D., & Leiserowitz, A. (2014). The mutual reinforcement of media selectivity and effects: Testing the reinforcing spirals framework in the context of global warming. Journal of Communication, 64(4), 590–611. https://doi.org/10.1111/jcom.12108
- Fisher, Z., Tipton, E., & Hou, Z. (2017). robumeta: Robust variance meta-regression (R package version 2.0) [computer software]. https://cran.r-project.org/ package=robumeta.
- Fiske, S. T., & Dupree, C. (2014). Gaining trust as well as respect in communicating to motivated audiences about science topics. PNAS, 111(4), 13593–13597. https://doi.org/10.1073/pnas.1317505111
- Funk, C. (2017). Mixed messages about public trust in science. Issues in Science and Technology, 34(1), 86–88. http://www.jstor.org/stable/44577388.
- Gauchat, G. (2011). The cultural authority of science: Public trust and acceptance of organized science. Public Understanding of Science, 20(6), 751–770. https://doi.org/10.1177/0963662510365246
- Gauchat, G. (2012). Politicization of science in the public sphere: A study of public trust in the United States, 1974 to 2010. American Sociological Review, 77(2), 167–187. https://doi.org/10.1177/0003122412438225
- Gauchat, G. (2015). The political context of science in the United States: Public acceptance of evidence-based policy and science funding. Social Forces, 94(2), 723–746. https://doi.org/10.1093/sf/sov040
- Graham, N., Carroll, W. K., & Chen, D. (2020). Carbon capital’s political reach: A network analysis of federal lobbying by the fossil fuel sector from Harper to Trudeau. Canadian Political Science Review, 14(1), 1–31. ISSN:1911-4125. https://doi.org/10.24124/c677/20201743
- Griffin, J. (2020). metapoweR: An R package for computing meta-analytic statistical power (R package version 0.2.1) [computer software]. https://CRAN.R-project.org/package=metapower.
- Hamilton, L. C. (2015). Conservative and liberal views of science, does trust depend on topic?. The Carsey School of Public Policy at the Scholars’ Repository 252. https://scholars.unh.edu/carsey/252.
- Hamilton, L. C., & Safford, T. G. (2020). Ideology Affects Trust in Science Agencies During a Pandemic. The Carsey School of Public Policy at the Scholars’ Repository 391. https://scholars.unh.edu/carsey/391.
- Hamilton, L. C., & Safford, T. G. (2021). Elite cues and the rapid decline in trust in science agencies on COVID-19. Sociological Perspectives, 64(5), 988–1011. https://doi.org/10.1177/07311214211022391
- Harrer, M., Cuijpers, P., Furukawa, T., & Ebert, D. D. (2019). dmetar: Companion R package for the guide “Doing meta-analysis in R”. (R package version 0.0.9000) [computer software]. https://github.com/MathiasHarrer/dmetar.
- Harrer, M., Cuijpers, P., Furukawa, T. A., & Ebert, D. D. (2021). Doing meta-analysis with R: A hands-on guide. Chapman & Hall/CRC Press. ISBN 978-0-367-61007-4.
- Harris, A. J. L., Sildmäe, O., Speekenbrink, M., & Hahn, U. (2018). The potential power of experience in communications of expert consensus levels. Journal of Risk Research, 22(5), 593–609. https://doi.org/10.1080/13669877.2018.1440416
- Herman, E. S., & Chomsky, N. (1988). Manufacturing consent: The political economy of the mass media. Pantheon Books.
- Higgins, J. P. T., & Green, S. (2020). Cochrane handbook for systematic reviews of interventions (Version 6.1). The Cochrane Collaboration. https://training.cochrane.org/handbook.
- *Hmielowski, J. D., Feldman, L., Myers, T. A., Leiserowitz, A., & Maibach, E. (2014). An attack on science? Media use, trust in scientists, and perceptions of global warming. Public Understanding of Science, 23(7), 866–883. https://doi.org/10.1177/0963662513480091
- *Hmielowski, J. D., & Nisbet, E. C. (2016). “Maybe yes, maybe no?”: Testing the indirect relationship of news use through ambivalence and strength of policy position on public engagement with climate change. Mass Communication and Society, 19(5), 650–670. https://doi.org/10.1080/15205436.2016.1183029
- Hornsey, M., Harris, E., Bain, P., & Fielding, K. S. (2016). Meta-analyses of the determinants and outcomes of belief in climate change. Nature Climate Change, 6(6), 622–626. https://doi.org/10.1038/nclimate2943
- Hornsey, M. J., & Lewandowsky, S. (2022). A toolkit for understanding and addressing climate scepticism. Nature Human Behaviour, 6(11), 1454–1464. https://doi.org/10.1038/s41562-022-01463
- Howell, E. L., Wirz, C. D., Scheufele, D. A., Brossard, D., & Xenos, M. A. (2020). Deference and decision-making in science and society: How deference to scientific authority goes beyond confidence in science and scientists to become authoritarianism. Public Understanding of Science, 29(8), 800–818. https://doi.org/10.1177/0963662520962741
- Huber, B., Barnidge, M., Gil de Zúñiga, H., & Liu, J. (2019). Fostering public trust in science: The role of social media. Public Understanding of Science, 28(7), 759–777. https://doi.org/10.1177/0963662519869097
- *Hula, R., Bowers, M., Whitley, C., & Isaac, W. (2017). Science, politics and policy: How Michiganders think about the risks facing the great lakes. Human Ecology, 45(4), https://doi.org/10.1007/s10745-017-9943-0
- Jackson, D., & Turner, R. (2017). Power analysis for random-effects meta-analysis. Research Synthesis Methods, 8(3), 290–302. https://doi.org/10.1002/jrsm.1240
- Jarreau, P. B., & Porter, L. (2018). Science in the social media age: Profiles of science blog readers. Journalism & Mass Communication Quarterly, 95(1), 142–168. https://doi.org/10.1177/1077699016685558
- Jones, C., Hine, D. W., & Marks, A. D. G. (2017). The future is now: Reducing psychological distance to increase public engagement with climate change. Risk Analysis, 37(2), 331–341. https://doi.org/10.1111/risa.12601
- *Kahlor, L., & Rosenthal, S. (2009). If we seek, do we learn?: Predicting knowledge of global warming. Science Communication, 30(3), 380–414. https://doi.org/10.1177/1075547008328798
- Kantartzis, S., & Molineux, M. (2011). The influence of Western society’s construction of a healthy daily life on the conceptualisation of occupation. Journal of Occupational Science, 18(1), 62–80. https://doi.org/10.1080/14427591.2011.566917
- *Kellstedt, P. M., Zahran, S., & Vedlitz, A. (2008). Personal efficacy, the information environment, and attitudes toward global warming and climate change in the United States. Risk Analysis, 28(1), 113–126. https://doi.org/10.1111/j.1539-6924.2008.01010.x
- King, B., & Short, M. (2017). Science: How the status quo harms its cultural authority. BioEssays, 39(12), 1–3. https://doi.org/10.1002/bies.201700154
- KNAW (Koninklijke Nederlandse Akademie van Wetenschappen). (2013). Vertrouwen in wetenschap. Adviescommissie integriteit, beleid en vertrouwen in wetenschap. ISBN:9789069846699.
- Koehler, D. (2016). Can journalistic “false balance” distort public perception of consensus in expert opinion? Journal of Experimental Psychology: Applied, 22(1), https://doi.org/10.1037/xap0000073
- Kozyreva, A., Lewandowsky, S., & Hertwig, R. (2020). Citizens versus the internet: Confronting digital challenges with cognitive tools. Psychological Science in the Public Interest, 21(3), 103–156. https://doi.org/10.1177/1529100620946707
- Krause, N. M., Brossard, D., Scheufele, D. A., Xenos, M. A., & Franke, K. (2019). The polls – trends Americans’ trust in science and scientists. Public Opinion Quarterly, 83(4), 817–836. https://doi.org/10.1093/poq/nfz041
- Kruse, L. (2011). Psychological aspects of sustainability communication. In J. Godemann & G. Michelsen (Eds.), Sustainability communication. Springer. https://doi.org/10.1007/978-94-007-1697-1_6
- Kulin, J., Johansson Sevä, I., & Dunlap, R. E. (2021). Nationalist ideology, rightwing populism, and public views about climate change in Europe. Environmental Politics, 30(7), 1111–1134. https://doi.org/10.1080/09644016.2021.1898879
- Lang, J. T., & Hallman, W. K. (2005). Who does the public trust? The case of genetically modified food in the United States. Risk Analysis, 25(5), 1241–1252. https://doi.org/10.1111/j.1539-6924.2005.00668.x
- Lee, C., & Scheufele, D. A. (2006). The influence of knowledge and deference toward scientific authority: A media effects model for public attitudes toward nanotechnology. J&MC Quarterly, 83(4), 819–834. https://doi.org/10.1177/107769900608300406
- Leiserowitz, A. A. (2005). American risk perceptions: Is climate change dangerous? Risk Analysis, 25(6), 1433–1442. https://doi.org/10.1111/j.1540-6261.2005.00690.x
- Lewandowsky, S. (2021). Climate change, disinformation, and how to combat it. Annual Review of Public Health, 42(1), 1–21. https://doi.org/10.1146/annurev-publhealth-090419-102409
- Lewandowsky, S., Cook, J., Fay, N., & Gignac, G. E. (2019). Science by social media: Attitudes towards climate change are mediated by perceived social consensus. Memory & Cognition, 47(8), 1445–1456. https://doi.org/10.3758/s13421-019-00948-y
- Lewandowsky, S., Gignac, G. E., & Vaughan, S. (2012). The pivotal role of perceived scientific consensus in acceptance of science. Nature Climate Change, 3(4), 399–404. https://doi.org/10.1038/nclimate1720
- Lewandowsky, S., & Van der Linden, S. (2021). Countering misinformation and fake news through inoculation and prebunking. European Review of Social Psychology, 32(2), 348–384. https://doi.org/10.1080/10463283.2021.1876983
- Linden, A. H., & Hönekopp, J. (2021). Heterogeneity of research results: A new perspective from which to assess and promote progress in psychological science. Perspectives on Psychological Science, 16(2), 358–376. https://doi.org/10.1177/1745691620964193
- Lipsey, M. W., & Wilson, D. B. (2001). Practical meta-analysis. Volume: 49 series: Applied social research methods. Sage Publications, INC. ISBN:9780761921684.
- Litfin, K. (2000). Environment, wealth, and authority: Global climate change and emerging modes of legitimation. International Studies Review, 2(2), 119–148. https://doi.org/10.1111/1521-9488.00207
- Liu, H., & Priest, S. (2009). Understanding public support for stem cell research: Media communication, interpersonal communication and trust in key actors. Public Understanding of Science, 18(6), 704–718. https://doi.org/10.1177/0963662508097625
- Lynas, M., Houlton, B., & Simon, P. (2021). Greater than 99% consensus on human caused climate change in the peer-reviewed scientific literature. Environmental Research Letters, 16(11), 114005. https://doi.org/10.1088/1748-9326/ac2966
- *MacInnis, B., &Krosnick, J. (2016). Chapter 13: Trust in scientists’ statements about the environment and American public opinion on global warming. In J. A. Krosnick (Ed.), Political psychology. Psychology Press. ISBN:9781315445687
- *Malka, A., Krosnick, J. A., & Langer, G. (2009). The association of knowledge with concern about global warming: Trusted information sources shape public thinking. Risk Analysis, 29(5), 633–647. https://doi.org/10.1111/j.1539-6924.2009.01220.x
- McCright, A. M., Dentzman, K., Charters, M., & Dietz, T. (2013). The influence of political ideology on trust in science. Environmental Research Letters, 8(4), 044029. https://doi.org/10.1088/1748-9326/8/4/044029
- McGuinness, L. A., & Higgins, J. P. T. (2020). Risk-of-bias VISualization (robvis): An R package and Shiny web app for visualizing risk-of-bias assessments. Research Synthesis Methods, 12(1), 55–61. https://doi.org/10.1002/jrsm.1411
- Merkley, E., & Stecula, D. (2021). Party cues in the news: Democratic elites, Republican Backlash, and the dynamics of climate skepticism. British Journal of Political Science, 51(4), 1439–1456. https://doi.org/10.1017/S0007123420000113
- *Metag, J., Füchslin, T., & Schäfer, M. S. (2017). Global warming’s five Germanys: A typology of Germans’ views on climate change and patterns of media use and information. Public Understanding of Science, 26(4), 434–451. https://doi.org/10.1177/0963662515592558
- Moser, S. C. (2010). Communicating climate change: History, challenges, process and future directions. WIRES Climate Change, 1(1), 31–53. https://doi.org/10.1002/wcc.011
- Moser, S. C. (2016). Reflections on climate change communication research and practice in the second decade of the 21st century: What more is there to say? WIRES Climate Change, 7(3), 345–369. https://doi.org/10.1002/wcc.403
- Myers, T. A., Maibach, E. W., Roser-Renouf, C., Akerlof, K., & Leiserowitz, A. A. (2012). The relationship between personal experience and belief in the reality of global warming. Nature Climate Change, 3(4), 343–347. https://doi.org/10.1038/nclimate1754
- Nakagawa, S., Lagisz, M., O’Dea, R. E., Rutkowska, J., Yang, Y., Noble, D. W. A., & Senior, A. M. (2021). The orchard plot: Cultivating a forest plot for use in ecology, evolution, and beyond. Research Synthesis Methods, 12(1), 4–12. https://doi.org/10.1002/jrsm.1424
- Nerlich, B., Koteyko, N., & Brown, B. (2010). Theory and language of climate change communication. WIRES Climate Change, 1(1), 97–110. https://doi.org/10.1002/wcc.2
- Nisbet, M. C. (2014). Framing science: A new paradigm in public engagement. In L. Kahlor& P. A. Stout (Eds.), Communicating science (pp. 40–67). Routledge.
- O’Brien, T. L. (2012). Scientific authority in policy contexts: Public attitudes about environmental scientists, medical researchers, and economists. Public Understanding of Science, 22(7), 799–816. https://doi.org/10.1177/0963662511435054
- Olkin, I., Dahabreh, I. J., & Trikalinos, T. A. (2012). GOSH – a graphical display of study heterogeneity. Research Synthesis Methods, 3(3), 214–223. https://doi.org/10.1002/jrsm.1053
- O’Neill, S. J., & Boykoff, M. (2010). The role of new media in engaging the public with climate change. In L. Whitmarsh & I. Lorenzoni (Eds.), Engaging the public with climate change (pp. 233–251). Routhledge. ISBN:9781849775243
- Oreskes, N. (2004). The scientific consensus on climate change. Science, 306(5702), 1686. https://doi.org/10.1126/science.1103618
- Ouzzani, M., Hammady, H., Fedorowicz, Z., & Elmagarmid, A. (2016). Rayyan – a web and mobile app for systematic reviews. Systematic Review, 5(210), https://doi.org/10.1186/s13643-016-0384-4
- Page, M. J., McKenzie, J. E., Bossuyt, P. M., Boutron, I., Hoffmann, T. C., Mulrow, C. D., Shamseer, L., Tetzlaff, J. M., Akl, E. A., Brennan, S.E. & Chou, R. (2021). The PRISMA 2020 statement: An updated guideline for reporting systematic reviews. BMJ, 372. https://doi.org/10.1136/bmj.n71
- Pastor, D. A., & Lazowski, R. A. (2018). On the multilevel nature of meta-analysis: A tutorial, comparison of software programs, and discussion of analytic choices. Multivariate Behavioral Research, 53(1), 74–89. https://doi.org/10.1080/00273171.2017.1365684
- Pechar, E., Bernauer, T., & Mayer, F. (2018). Beyond political ideology: The impact of attitudes towards government and corporations on trust in science. Science Communication, 40(3), 291–313. https://doi.org/10.1177/1075547018763970
- Peck, J., & Tickell, A. (2002). Neoliberalizing space. Antipode, 34(3), 380–404. https://doi.org/10.1111/1467-8330.00247
- Petersen, A. M., Vincent, E. M., & Westerling, A. L. (2019). Discrepancy in scientific authority and media visibility of climate change scientists and contrarians. Nature Communications, 10(3966), https://doi.org/10.1038/s41467-019-12061-4
- Peterson, R. A., & Brown, S. P. (2005). On the use of beta coefficients in meta-analysis. Journal of Applied Psychology, 90(1), 175–181. https://doi.org/10.1037/0021-9010.90.1.175
- Prior, M. (2005). News vs. Entertainment: How increasing media choice widens gaps in political knowledge and turnout. American Journal of Political Science, 49(3), 577–592. https://doi.org/10.2307/3647733
- Pustejovsky, J. (2022). clubSandwich: Cluster-robust (sandwich) variance estimators with small-sample corrections (R package version 0.5.6) [computer software]. https://github.com/jepusto/clubSandwich.
- Rainie, L., Funk, C., & Page, D. (2015). Public and Scientists’ Views on Science and Society. Pew Research Centre.
- Ratter, B. M. W., Philipp, K. H. I., & Von Storch, H. (2012). Between hype and decline: Recent trends in public perception of climate change. Environmental Science & Policy, 18, 3–8. https://doi.org/10.1016/j.envsci.2011.12.007
- Revelle, W. (2022). psych: Procedures for Psychological, Psychometric, and Personality Research (R package version 2.2.9) [computer software]. https://CRAN.R-project.org/package=psych.
- *Rhodes, E., Axsen, J., & Jaccard, M. (2017). Exploring citizen support for different types of climate policy. Ecological Economics, 137, 56–69. https://doi.org/10.1016/j.ecolecon.2017.02.027
- Rosenthal, S. (2022). Information sources, perceived personal experience, and climate change beliefs. Journal of Environmental Psychology, 81, https://doi.org/10.1016/j.jenvp.2022.101796
- Roser-Renouf, C., Maibach, E., & Leiserowitz, A. (2009). Global Warming’s Six Americas: An Audience Segmentation Analysis. Yale Project on Climate Change and the George Mason University Center for Climate Change Communication.
- Roth, P. L., Le, H., Oh, I., Van Iddekinge, C. H., & Bobko, P. (2017). Using beta coefficients to impute missing correlation coefficients in meta-analytic research: Reasons for caution. Journal of Applied Psychology, 103(6), 644–658. https://doi.org/10.1037/apl0000293
- Schäfer, M. S. (2012). Online communication on climate change and climate politics: A literature review. Wiley Interdisciplinary Reviews: Climate Change, 3(6), https://doi.org/10.1002/wcc.191
- Schäfer, M. S., & Schlichting, I. (2014). Media representations of climate change: A meta-analysis of the research field. Environmental Communication, 8(2), 142–160. https://doi.org/10.1080/17524032.2014.914050
- Schäfer, T., & Schwarz, M. A. (2019). The meaningfulness of effect sizes in psychological research: Differences between Sub-disciplines and the impact of potential biases. Frontiers in Psychology, 10, 813. https://doi.org/10.3389/fpsyg.2019.00813
- Schmid-Petri, H., Adam, S., Schmucki, I., & Häussler, T. (2017). A changing climate of skepticism: The factors shaping climate change coverage in the US press. Public Understanding of Science, 26(4), 498–513. https://doi.org/10.1177/0963662515612276
- Sewpaul, V. (2015). Neoliberalism. In J. D. Wright (Ed.), International encyclopedia of the social & behavioral sciences (pp. 462–468). Elsevier. https://doi.org/10.1016/B978-0-08-097086-8.28062-8
- Shackley, S., & Wynne, B. (1996). Representing uncertainty in global climate change science and policy: Boundary-ordering devices and authority. Science, Technology & Human Values, 21(3), 275–302. https://doi.org/10.1177/016224399602100302
- Slater, M. D. (2007). Reinforcing spirals: The mutual influence of media selectivity and media effects and their impact on individual behavior and social identity. Communication Theory, 17(3), 281–303. https://doi.org/10.1111/j.1468-2885.2007.00296.x
- Spence, A., Poortinga, W., & Pidgeon, N. (2012). The psychological distance of climate change. Risk Analysis, 32(6), 957–972. https://doi.org/10.1111/j.1539-6924.2011.01695.x
- *Stamm, K. R., Clark, F., & Eblacas, P. R. (2000). Mass communication and public understanding of environmental problems: The case of global warming. Public Understanding of Science, 9(3), 219–237. https://doi.org/10.1088/0963-6625/9/3/302
- Stanley, T. D., & Doucouliagos, H. (2014). Meta-regression approximations to reduce publication selection bias. Research Synthesis Methods, 5(1), 60–78. https://doi.org/10.1002/jrsm.1095
- Stecula, D. A., & Merkley, E. (2019). Framing climate change: Economics, ideology, and uncertainty in American news media content from 1988 to 2014. Frontiers in Communication, 4, 6. https://doi.org/10.3389/fcomm.2019.00006
- Strömbäck, J., Tsfati, Y., Boomgaarden, H., Damstra, A., Lindgren, E., Vliegenthart, R., & Lindholm, T. (2020). News media trust and its impact on media use: Toward a framework for future research. Annals of the International Communication Association, 44(2), 139–156. https://doi.org/10.1080/23808985.2020.1755338
- Su, L. Y.-F., Akin, H., Brossard, D., Scheufele, D. A., & Xenos, M. A. (2015). Science news consumption patterns and their implications for public understanding of science. Journalism & Mass Communication Quarterly, 92(3), 597–616. https://doi.org/10.1177/1077699015586415
- *Taddicken, M. (2013). Climate change from the user’s perspective: The impact of mass media and internet use and individual and moderating variables on knowledge and attitudes. Journal of Media Psychology: Theories, Methods, and Applications, 25(1), 39–52. https://doi.org/10.1027/1864-1105/a000080
- *Taddicken, M., & Reif, A. (2016). Who participates in the climate change online discourse? A typology of Germans’ online engagement. Communications, 41(3), 315–337. https://doi.org/10.1515/commun-2016-0012
- *Thaker, J., Zhao, X., & Leiserowitz, A. (2017). Media use and public perceptions of global warming in India. Environmental Communication, 11(3), 353–369. https://doi.org/10.1080/17524032.2016.1269824
- Tsfati, Y., Strömbäck, J., Lindgren, E., Damstra, A., Boomgaarden, H. G., & Vliegenthart, R. (2022). Going beyond general media trust: An analysis of topical media trust, its antecedents and effects on issue (mis)perceptions. International Journal of Public Opinion Research, 34(2), https://doi.org/10.1093/ijpor/edac010
- Tuitjer, L., & Dirksmeier, P. (2021). Social media and perceived climate change efficacy: A European comparison. Digital Geography and Society, 2, https://doi.org/10.1016/j.diggeo.2021.100018
- Van der Linden, S. L. (2021). The Gateway Belief Model (GBM): A review and research agenda for communicating the scientific consensus on climate change. Current Opinion in Psychology, 42, 7–12. https://doi.org/10.1016/j.copsyc.2021.01.005
- Van der Linden, S. L., Leiserowitz, A. A., Feinberg, G. D., & Maibach, E. W. (2015). The scientific consensus on climate change as a Gateway Belief: Experimental evidence. PLoS One, 10(2), e0118489. https://doi.org/10.1371/journal.pone.0118489
- Vevea, J. L., & Hedges, L. V. (1995). A general linear model for estimating effect size in the presence of publication bias. Psychometrika, 60(3), 419–435. https://doi.org/10.1007/BF02294384
- Victor, D. G. (2009). The politics of fossil-fuel subsidies. International Institute for Sustainable Development, https://doi.org/10.2139/ssrn.1520984
- Viechtbauer, W. (2010). Conducting meta-analyses in R with the metafor package. Journal of Statistical Software, 36(3), 1–48. https://doi.org/10.18637/jss.v036.i03
- *Visschers, V. H. M. (2018). Public perception of uncertainties within climate change science. Risk Analysis, 38(1), 43–55. https://doi.org/10.1111/risa.12818
- Vraga, E. K., Kim, S. C., & Cook, J. (2019). Testing logic-based and humor-based corrections for science, health, and political misinformation on social media. Journal of Broadcasting & Electronic Media, 63(3), 393–414. https://doi.org/10.1080/08838151.2019.1653102
- Wang, S., Corner, A., Chapman, D., & Markowitz, E. (2017). Public engagement with climate imagery in a changing digital landscape. WIRES Climate Change, 9(509), https://doi.org/10.1002/wcc.509
- *Wang, X. (2017). Understanding climate change risk perceptions in China: Media use, personal experience, and cultural worldviews. Science Communication, 39(3), 291–312. https://doi.org/10.1177/1075547017707320
- Wickham, H., Averick, M., Bryan, J., Chang, W., McGowan, L. D., François, R., Grolemund, G., Hayes, A., Henry, L., Hester, J., Kuhn, M., Pedersen, T. L., Miller, E., Bache, S. M., Müller, K., Ooms, J., Robinson, D., Seidel, D. P., Spinu, V., … Yutani, H. (2019). Welcome to the tidyverse. Journal of Open Source Software, 4(43), 1686. https://doi.org/10.21105/joss.01686
- Wickham, H., Hester, J., & Bryan, J. (2022). readr: Read Rectangular Text Data (R package version 2.1.3) [computer software]. https://github.com/tidyverse/readr.
- *Williams, A. E. (2011). Media evolution and public understanding of climate science. Politics and the Life Sciences, 30(2), 20–30. https://doi.org/10.2990/30_2_20. PMID: 22702422.
- Wynne, B. (2006). Public engagement as a means of restoring public trust in science – hitting the notes, but missing the music? Community Genetics, 9, 211–220. https://doi.org/10.1159/000092659
- *Yang, X., Shirley, S., & Ho, S. S. (2017). Decreasing the knowledge gap among different socioeconomic status groups on the issue of climate change. Environmental Hazards, 16(3), 276–290. https://doi.org/10.1080/17477891.2017.1279999
- Zerva, A., Grigoroudis, E., Karasmanaki, E., & Tsantopoulos, G. (2021). Multiple criteria analysis of citizens’ information and trust in climate change actions. Environment Development and Sustainability, 23(5), 7706–7727. https://doi.org/10.1007/s10668-020-00942-4
- *Zhao, X. (2009). Media use and global warming perceptions: A snapshot of the reinforcing spirals. Communication Research, 36(5), 698–723. https://doi.org/10.1177/0093650209338911
List of appendices
Appendix 1 – Power analysis
Appendix 2 – Paper collection: database searches and results, included and excluded papers, reasons for exclusion
Appendix 3 – Results Risk of Bias and SciteAI analysis
Appendix 4 – Results and plots GOSH analysis
Appendix 5 – Results P-curve analysis, Baujat plot, outlier and leave-one-out analysis
Appendix 6 – PRISMA checklist Abstract
Appendix 7 – PRISMA checklist Manuscript
The appendices are available at https://osf.io/w3syv/x.