Abstract
The seriousness of losses caused by disaster dependent on the hazard degree of environment, vulnerability of hazard-affected bodies, and emergency response capacity of the region is studied in this article. The study on hazard-affected bodies is of importance to disaster risk management, regional hazard prevention, reduction, and investment in disaster insurance. With summarizing of various assessment methods of vulnerability of hazard-affected bodies, this paper presents a refined Spatial Quantification Model of regional vulnerability which combines refined spatial geographic data and land-use type data. A quantitative study on regional vulnerability was carried out by defining fine spatial grid as the basic evaluation unit based on GIS.
Introduction
Disaster means a calamity brought to human beings and their society due to mutation of environmental factors (Bo and Bo Citation2008). The natural hazard and hazard-affected bodies are interrelated and complementary. The seriousness of losses caused by disaster depends on the dangerous degree of environment, vulnerability of hazard-affected bodies, and emergency response capacity of the region (Shi Citation2002). A large number of cases of disasters show that the vulnerability of hazard-affected bodies is an important reason for the enormous losses from disasters. Determining the pre-disaster vulnerability of hazard-affected bodies becomes the primary task of a regional mitigation. Vulnerability assessment of the hazard-affected body will help to guide macro-adjustment of the regional planning so that disaster losses can be minimized.
The vulnerability of hazard-affected bodies means the inherent sensitivity of various hazard-affected bodies responding to the strike of hazard. The extent of losses suffered from a disaster in a region can be assessed by the vulnerability of hazard-affected bodies (Chen and Liang Citation2008). At present, the vulnerability assessment is still mainly focused on qualitative studies. However, with the in-depth research, the study on the vulnerability will be shifted from the sociological qualitative analysis to the quantitative assessment with a combination of natural sciences and sociology (Shang Citation2000). For a specific type of disaster, the population can be divided into different groups of vulnerability according to their income (such as rich, middle-income, and poor), age, and health status. Then the extent of disaster suffered by the different groups of people in a disaster and the impact of local social, political, economic, legal, cultural, and market systems on these people can be analyzed qualitatively (Alexander Citation1993). In fact, the occurrence of any disaster has its own location, frequency, quasi-periodic, and strength. And the extent of the disaster complicatedly interacts with the various resources, socio-economic status and legal rights, and interests possessed by the population in the disaster-affected region. Qualitative analysis can indeed provide a certain basis for social rescue and policy-making for the disaster, but there are shortcomings. The authors believe that the quantitative assessment of vulnerability is able to grasp the regional distribution patterns, rules and mechanisms, reaction model, and response of the vulnerability of various disasters, and it will have better application in the regional mitigation practices.
At present, there are four main methods for vulnerability evaluation. First is the composite index method which adopts the mathematical statistics techniques, such as the weighted sum (average) method (Moss and Malone Citation2002), principal component analysis (Cutter and Boruf Citation2003), analytic hierarchy process (AHP; Thirumalaivasan et al. Citation2003), fuzzy comprehensive evaluation method (Dixon Citation2005), etc. The composite index method is being extensively applied because it is simple and easy to operate. However, this method lacks the systematic evaluation of the vulnerability, ignoring the interaction mechanisms between the various components of the vulnerability. The second is the layer overlay method which is based on GIS technology. According to the evaluation objectives, the stacked layers comprise of the various components of the vulnerability or different disturbances to the vulnerability (O'Brien et al. Citation2004). This method provides an approach to the vulnerability evaluation in the context of multiple disturbances (natural and economic), but it does not consider the risks of various disturbances and their different impact on vulnerability of the overall system. The third is vulnerability function model evaluation method. In this method, quantitative evaluation of all the constituent elements of the vulnerability is first made. The vulnerability assessment model is then established from the interactions between the constituent elements of the vulnerability (Metzger et al. Citation2005). As this method is able to reflect interaction mechanisms between the constituent elements of the vulnerability, this will help to explain the origins and characteristics of vulnerability and the evaluation results are able to reflect the overall vulnerability of the whole system and constituent elements of vulnerability. However, there is no common agreement on the definition, constituent elements of vulnerability and their interaction mechanism, and it is difficult to quantitatively describe the constituent elements of the vulnerability. All of these difficulties make the current development of this evaluation method slow. Nevertheless, this method is attracting more concerns about the vulnerability assessment studies. The last method is the fuzzy matter-element evaluation method in which the relative vulnerability of the study area can be determined by the degree of similarity relative to a selected reference state (the highest or lowest vulnerability) (Chen and Wang Citation2007). However, the evaluation results from this method reflect relatively small amounts of information, only showing the relative vulnerability of the study area. It is difficult to identify the determinants of spatial differences and characteristics of vulnerability.
In this paper, a fine Spatial Quantitative Model of the vulnerability of hazard-affected bodies has been proposed to study the vulnerability of hazard-affected bodies by combining the refined spatial geographic data and land-use types of data, and defining fine spatial grid as the basic evaluation unit. At present, in vulnerability assessment, GIS application of the majority is also limited to data management and evaluation of the results of the mapping (Pang and Dondchen Citation2008). Powerful GIS spatial analysis, geographic analysis, statistical analysis, and other functions, and there is no real effect; hence, the establishment of a fine spatial GIS model to quantify the vulnerability of space provides a good quantitative technique. Based on this model, quantification of relatively simple data was first carried out to achieve an overall knowledge on the vulnerability of the region. Then an in-depth and detailed evaluation and analysis was performed by introduction of a more precise geographical and population factors data as well as more sophisticated methods of evaluation.
Fine spatial quantification model
Evaluation index and evaluation unit
In natural hazard, the regional vulnerability of hazard-affected bodies is characterized by the degree of vulnerability of hazard, regional economic and population. According to this, the refined data of land-use types are introduced and an evaluation index system is established. The index system of regional vulnerability of hazard-affected bodies is shown in .
Table 1. The index system of regional vulnerability of hazard-affected bodies.
The present three index factors, land use, socio-economic, and distribution of population, can be replaced by annual income to some extent. It only can reflect the vulnerability of disaster generally but not the different vulnerabilities among various disasters. The introduction of land-use type reflects precisely different vulnerabilities to different disasters of a region which reinforces the sensitivities of the constituent elements of vulnerability.
Evaluation unit is the basic unit used for quantifying the vulnerability of hazard-affected bodies. At present, the evaluation unit of the vulnerability is the vector unit of the main administrative divisions. This paper presents an evaluation unit based on elements – raster vector hybrid model, which takes the spatial distribution of geographical features into consideration by integrating the vector unit of the geographical features and regular grid cells. The Spatial Quantitative Models are typically applied in county-level areas, according to the principle that the climatic conditions and disasters feature in the same evaluation unit being basically the same and also the limitation on calculating the amount of grids; the study area is divided into the grid mesh with an area of 100×100 m which satisfies the above requirement, basically.
Spatial Quantification Model
Spatial quantification of vulnerability of land-use type
Different types of land use largely determine the extent of their vulnerability. For a certain type of land use in a designated region, the maximum and minimum potential vulnerability to a particular disaster are defined to be 1 and 0, respectively. The actual weight of the potential vulnerability to a particular disaster and land-use type needs to be determined first. There are two main methods for determining the weight. The first is based on experts’ experiences and subjective judgments, such as the weight of experience, expert scoring, etc. The second is based on various mathematical methods, such as statistical average method, indicator value method, the adjacent target comparative law, flexible preference matrix method, stepwise regression method, gray correlation method, principal component analysis, AHP, fuzzy variable weighting method, etc. Mathematical methods are widely used because of their relatively less subjectivity, though the section of model and data in the mathematical methods also has certain subjectivity. For instance, the data in ‘Typhoon’ column is calculated by the following steps. Firstly, to get the weight of vulnerability of each kind of land-use type (residential, town, water, dry land, woodland, grassplot, and paddy field) to typhoon according to the AHP. Secondly, adjust the factor according to the situations of disasters in this area historically and then acquire the final calculated data. The obtained factors are shown in .
Table 2. Potential vulnerability factors of different land-use types and disasters.
The study area is divided into the grid mesh with an area of 100×100 m for each grid based on GIS technology. Each grid is a basic evaluation unit. The vulnerability of each evaluation unit can be calculated according to the proposed spatial quantization models (this evaluation unit is used to quantify the vulnerability of land-use types for a certain disaster). The study area presented in can be divided into several polygonal regions according to land-use types. It is seen from that very often more than one type of land use exists within one grid. Under this situation, an area-weighted interpolation method is adopted to derive the weighted vulnerability factor for that evaluation unit. This method according to the rules grid within the region occupied area of the source region to determine the percentage of grid area property values. The main steps are as follows: (1) to identify all types of land use within each grid unit; (2) to determine the area of all types of land use within the grid, and calculate their area percentages of grid size; (3) to allocate property values in proportion to the area percentages.
The specific algorithm is shown in . shows an evaluation unit with four types of land use within a grid, namely A, B, C, and D. For a specific type of natural disater, the potential vulnerability factors of different land-use types is different, this can be obtained from . The potential vulnerability A, B, C, and D, are I A, I B, I C, and I D, respectively. The area of A, B, C, and D in the grid are S A, S B, S C, and S D, respectively. I 1, the weighted value of vulnerability of land-use types in this evaluation unit, can be expressed as:
Spatial quantification of the vulnerability of socio-economic factors and population factors
When taking into account the socio-economic factors and population factors, vulnerability assessment in the past was usually evaluated based on administrative divisions as evaluation units. The results often show significant deficiencies in the accuracy and continuity in the spatial distribution. For example, within an administrative region, when the economic and population distributions in urban areas and rural regions are significantly different, it is not advisable to put them in the same unit for evaluation. According to the economic contribution rate of various types of land use, the spatial quantification of social–economic data in each administrative region can be reasonably determined based on the refined geographical data and land-use types. Similarly, for the demographic factors, the spatial distribution of the population can be reasonably calculated according to the distribution of urban areas and residential regions. Similar to the above-mentioned method, the value of vulnerability of the socio-economic I 2 and the value of vulnerability of the population factor I 3 can be derived.
Overall evaluation
Bringing all the above discussed factors together, spatial quantitative value of the vulnerability of hazard-affected bodies in a region is I.
Case study
The above-mentioned ideas and algorithms have been applied in a regional prevention. The fine geographical elements, such as township population data, economic data of various sectors, buildings, roads, lifelines, and refined data of land-use types from the years 2007 and 2008 were selected. and present the distribution of the vulnerability of socio-economic factors and population elements, respectively. There are 21 types of land use, including 21 woodland, 22 shrub forest, 23 sparse, 24 other wooded, 31 high-coverage grassland, 32 in the coverage of grass, 33 low-coverage grassland, 41 canals, 42 lakes, 43 reservoirs, 51 urban land use, 52 residential areas, 53 industrial and communications construction land, 66 bare rock, 111 mountain paddy fields, 112 hills paddy fields, 113 plains, 121 mountains in arid, 122 dry hills, and 123 arid plains (the number before each type is the type code). Based on the classification criteria of land-use type as well as the actual situation in the region, these 21 types of land use can be grouped into seven main types, i.e. residential areas (52, 53), urban land use (51), waters (41, 42, 43), dry land (121, 122, 123), woodland (21, 22, 23, 24), grasslands (31, 32, 33), paddy (111, 112, 113). shows the distribution of seven types of land-use data in the same region.
Taking the typhoon disasters for example, an assessment of the vulnerability of hazard-affected bodies in the region was carried out according to the method introduced in Spatial Qualification Model and the spatial distribution of the vulnerability was then obtained as shown in .
Figure 6. The distribution of the vulnerability of hazard-affected bodies in regional typhoon disasters.
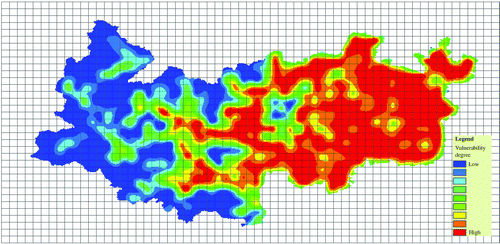
A paragraph of how these calculations have found entry points to the decision-making and how authorities make use of them has been added in the last part of the Section ‘Case study.’ Using the method described above the Spatial Quantitative distribution of various vulnerabilities of hazard-affected bodies under different disasters in a region is obtained, according to which the local mitigation department can get the risk distribution and stricken index factors of various disasters locally. The government can take firm structural measures in vulnerable areas normally and ensure effective warning and emergency response according to the risk distribution and stricken index factors during the disaster and even evaluate the stricken situation rationally according to the spatial quantitative distribution of vulnerabilities in recovery. This provides scientific assistance to decision-making for governments on practices of disaster reduction and prevention.
Conclusion
In this paper, a fine Spatial Quantitative Model has been proposed to study the vulnerability of hazard-affected bodies by combining the refined spatial geographic data, land-use types of data, and defining fine spatial grid as the basic evaluation unit. Meanwhile, a new approach for evaluation of socio-economic factors and demographic elements was also adopted in which the spatial distribution of social–economic was determined by the contribution rate of various types of land use to the economics. According to the distribution of urban land and residential land, the spatial distribution of population was reasonably calculated. This method reinforces the interaction of the constituent elements of vulnerability and has obvious advantages in reflecting the spatial differences of vulnerability and the sensitivity of the constituent elements of vulnerability. These advantages are more prominent for evaluation of the vulnerability when the data is sufficiently precise. It can produce more objective and accurate values of the vulnerability and its spatial distribution. In addition, it is believed that vulnerability assessment of hazard-affected bodies should start from the study of a single disaster. Based on the precise understanding of the vulnerability of hazard-affected bodies in each type of disaster, complex evaluation methods can then be selected to perform a general analysis. The proposed method was successfully applied in practical disaster planning for Deqing County and Jiaxing City, in Zhejiang Province, China.
Notes on contributors
Zhang Bin is a PhD student of Tsinghua University. His current research interests center on risk and disaster assessment, and public safety emergency resource demand forecast.
Yuan Hongyong is Professor of Center for Public Safety Research of Tsinghua University. He received his doctorate from WuHan University in Photogrammetry and Remote Sensing in 1994. He is the deputy director of Center for Public Safety Research. He is the author of some 50 scientific papers and four books. His current research interests center on fire detection and smart fighting, public safety, and emergency.
Huang Quanyi is Professor of Center for Public Safety Research of Tsinghua University. He received his doctor degree from WuHan University in GIS. His current research interests center on 3S technology application in the field of public safety.
Wen Renqiang is a PhD student of Tsinghua University. His current research interests center on emergency resource scheduling and allocation.
Gu Junqiang is a Senior Engineer of Zhejiang Research Institute of Meteological Science. He received his undergraduate degree from NanJin University in climate change impact assessment. His current research interests center on climate change impact assessment and disaster emergency management.
Acknowledgements
The authors thank two anonymous reviewers for providing valuable comments on this study. This research was partly funded by the Science and Technology project of the Meteorological Bureau of Zhejiang Province in 2010 (No. 2010ZD05) and Science and Technology projects in Zhejiang Province (No. 2007C33062).
References
- Alexander , D. , 1993 . Natural disasters . New York : Chapman and Hall
- Bo , L. Bo , L. , 2008 . An approach to synthetic management model of disasters and accidents in large cities Legn System and Society 122 134
- Chen , G. Liang , T. , 2008 . Evaluation model for urban-regional disaster bearing capacity and its application . Journal of Safety and Environment , 8 2 , 651 661 .
- Chen , H. and Wang , N. 2007 . Application of fuzzy matter element model in evaluation of water security based on Euclid approach degree . Journal of Xi'an University of Technology , 23 ( 1 ) : 37 – 42 .
- Cutter , S.L. and Boruf , B.J. 2003 . Social vulnerability to environmental hazards . Social Science Quarterly , 84 ( 2 ) : 242 – 261 .
- Dixon , B. 2005 . Groundwater vulnerability mapping: a GIS and fuzzy rule based integrated tool . Applied Geography , 25 ( 4 ) : 327 – 347 .
- Metzger , M.J. , Leemans , R. and Schroter , D. 2005 . A multidisciplinary multi-scale framework for assessing vulnerabilities to global change . International Journal of Applied Earth Observation and Geoinformation , 7 : 253 – 267 .
- Moss , R.H. Malone , E.L. , 2002 . Vulnerability to climate change: a quantitative approach .: Prepared for the US Department of Energy . Available from: http://www.globalchange.umd.edu/cgi-bin/Details.pl?sref=PNNL-13765
- O'Brien , K. , Leichenkob , R. and Kelkar , U. 2004 . Mapping vulnerability to multiple stressors climate change and globalization in India . Global Environmental Change , 14 ( 4 ) : 303 – 313 .
- Pang , X. Dondchen , E. , 2008 . GIS-based assessment of eco-environmental vulnerability of ice-free areas in Antarctica . Geomatics and Information Science of Wuhan University , 33 11 , 1174 1177 .
- Shang , Y. 2000 . Vulnerability study – the new development of synthesized study on natural disasters . Areal Research and Development , 19 ( 2 ) : 73 – 77 .
- Shi , P. 2002 . Theory on disaster science and disaster dynamics . Journal of Natural Disasters , 11 ( 3 ) : 1 – 9 .
- Thirumalaivasan , D. , Karmegam , M. and Venugopal , K. 2003 . AHP-DRASTIC: software for specific aquifer vulnerability assessment using DRASTIC model and GIS . Environmental Modelling & Software , 18 ( 7 ) : 645 – 665 .