Abstract
Geomorphologic and hydrologic research heavily depends on digital elevation models (DEM) which are currently being prepared from digital contours. The present study examines the use and applicability of freely available global elevation data source (3 arc seconds finished Shuttle Radar Topography Mission (SRTM)) in landform characterisation, geomorphometry, river basin studies and other allied scientific applications in comparison with contour elevation data derived from the surveyed topographical sheets. The relief data extracted from a conventionally digitised geo-information science dataset of topographic contours (1:50,000) are compared with the SRTM-DEM and the variations are analysed. The automated geomorphometric and landform parameters derived from the contour DEM and the computed statistical properties of those parameters have substantial agreement with the same parameters derived from the SRTM-DEM. At the same time, localised variations also exist in some spatial domains. Derivative landscape analysis outputs from the SRTM-DEM suggest the wide acceptability and applicability of the freely available SRTM data source, especially in the regional scale applications related to hydrological modelling, terrain characterisation, disaster management and land degradation studies.
Introduction
Mountain environments are highly susceptible to changes and cause complex environmental alterations, which are difficult to deduce and manage. These changes are caused mainly by the influence of altitude and relative relief characteristics of mountains on the dynamism and power of the biogeochemical processes with the spatial distribution and interaction of physical, biological, anthropogenic and aesthetic elements present in mountain environments (Gerrard Citation1990). Geomorphometric analysis of landforms provides a quantitative description of the shape and characteristics of terrains and is derived using a combination of mathematics, engineering and computer sciences (Blaszczynski Citation1997, Pike Citation2000, Alan et al. Citation2008, Tagil and Jenness Citation2008, Thomas et al. Citation2009). Geomorphometric parameters have been measured manually from topographic maps for decades (Chapman Citation1952, Strahler Citation1952, Pike and Wilson Citation1971, Zevenbergen and Thorne Citation1987) to assess and to evaluate the terrain characteristics of the area, as topography has great influence on hydrology, microclimate characteristics, geological structures, mineral deposits, landform dynamics and vegetation cover properties (Pike Citation2000).
Automatic extraction of topographic parameters based on digital elevation models (DEM) and geographical information systems (GIS) are increasingly being used in the geomorphological and hydrologic research covering a wide range of scales, from global to local (Florinsky Citation1998b, Toutin Citation2001, Lee et al. Citation2003, Gerstenecker et al. Citation2005, Nikolarkopoulos et al. Citation2006, Demirkesen Citation2008, Klingseisen et al. Citation2008, El-Magd and Hillman Citation2009). Geospatial information describing the elevation of the land surface above a common datum plane is defined as DEM. Digital terrain models (DTM) represent segments of spatial data bases related to terrain features and landforms, and offer the most common method for extracting vital topographic informations (Desmet and Govers Citation1996, Kamp et al. Citation2005, Singh et al. Citation2007). During the last two decades, the availability of DEM/DTM data has been continuously growing, data accuracy has improved, and additional algorithms have been developed and implemented through the industry standard and stand-alone GIS software programmes (Florinsky Citation1998a, Kamp et al. Citation2005, Zhang et al. Citation2010). In practice, DEMs are often derived from stereophotos or stereoscopic satellite imagery or from digitised topographic contour, which are time-consuming and laborious. The release of Shuttle Radar Topography Mapping Mission (SRTM) data having a grid resolution of 90 m for the whole world made the direct application of the DEM data in terrain analysis with the existing GIS resources and is an efficient and cost-effective method for acquiring accurate terrain information.
In the present study, DEM from topographical contours of 20 m interval and SRTM image of 90 m resolution was used for deriving the geomorphometric parameters and for the landform characterisation of a region, forming a part of the Western Ghats, in south India. The emphasis of this paper is placed on the comparison of the terrain derivatives from contour DEM and SRTM-DEM to assess the utility of the latter in the regional scale hydrologic/disaster management studies.
Study region
Comparison of the results of geomorphometric parameters derived from the two sources is attempted in a mountain area with high variability in elevation and terrain nature. The Munnar Forest Division falling within north latitudes 09°52′–10°18′ and east longitudes 76°42′–77°18′, represents a region of bioclimatic diversity located within the Western Ghats of Kerala, and covers an area of about 1341.18 km2 ().
Geologically, the study area falls within the high-grade granulite terrain (Soman Citation2002) and the predominant rock types in the area are granitic gneiss, migmatite (hornblende–biotite gneiss), intrusive granite bodies, calc-granulite and minor quartzite patches. The rocks are highly sheared and fractured at places. Pegmatites, quartz veins and basic intrusives cut across the older host rocks (Thampi et al. Citation1993). Locally, the rock grades into typical biotite or hornblende–biotite gneiss. The granitic gneiss is medium grained, pinkish in colour, and foliated. Foliation is expressed by parallel planar arrangement of flakes of biotite, prisms of hornblende and lenticular flattened quartz grains. Granite is seen exposed as WNW–ESE trending linear body with irregular outline surrounded by granitic gneiss, migmatite and calc-granulite (Nair et al. Citation1984). The foliation trends indicate the presence of a major synclinal–axial trace, contained mostly in the hornblende–biotite gneiss (Thomas et al. Citation2010). Joint systems are well-developed and the major lineaments in the area show NNE–SSW and NW–SE trends. The subaerial relief of the area, vegetated with plantations crops, forest and grassland, ranges from 40 to 2640 m at the highest mountain peaks. The study area exhibits a subtropical climate with minor variations due to the influence of high altitude hill ranges and receives an annual average rainfall of 660 cm from the south-west and north-west monsoons.
Methodology
Shuttle Radar Topography Mission (SRTM) data
The SRTM, involving a consortium of agencies in the USA, Germany and Italy, is aimed to produce a topographic map of the earth's land surface between 60° North and 56° South latitude using C- and X-band interferometric SARs, covering about 80% of earth's land mass. The SRTM-DEM products are being distributed mainly under three forms, the SRTM 1’, SRTM 3’ and SRTM-GTOPO30, with spatial resolution of 1 arc second (30 m), 3 arc seconds (90 m) and 30 arc seconds (1 km), respectively (Global Land Cover Facilities website Citation2005). The first is available only for the USA, while the latter is freely distributed for the rest of the globe. According to mission specifications, SRTM was expected to generate DEMs with a vertical RMSE of 16 m and the horizontal circular error of 20 m at the 90% confidence level (Farr and Kobrick Citation2000, Rabus et al. Citation2003, Rodriguez et al. Citation2006, Farr et al. 2007). Generally, any SRTM-DEM is a digital surface model (DSM). That means, the elevation data are with respect to the reflective surface, which may be vegetation, man-made features or bare earth, and are given above the WGS84 geoid (asl).
Input data processing
Topographical maps of scale 1:50,000 (prepared by Survey of India (SoI)) and SRTM data were used for the study. The 20 m contours extracted from the SoI topographical maps were digitised manually using ArcGIS 9.3 software. Then the digitally captured contour data were compiled to generate a raster contour DEM of the area to derive various terrain attributes. All studies employing DEMs make use of planar coordinates to have the same measurement units for both (x, y) and elevation. The SRTM data were provided in UTM WGS84 and therefore it was necessary to reproject the SRTM-derived DEM to the polyconic projection because the contour DEM was produced with respect to the projection of the SoI topographical maps. When dealing with multi-source and multi-resolution datasets, there is often a need for data sampled at one scale to be generalised to other scales. The pixel size of the contour DEM was 20 m, whereas the SRTM-derived DEM had a pixel dimension of 90 m, as provided by the Global Land Cover Facility (Global Land Cover Facilities website 2005). More details about the SRTM datasets, its errors and accuracy can be found at Farr et al. (Citation2007). In order to compare the two datasets, the contour DEM was scaled up to a pixel size, matching the dimensions of the SRTM. The GIS processing of these various analyses was performed using spatial analyst and 3D analyst extensions, topographic tool models for ArcGIS 9.3 and SAGA 2.0.
Selection and generation of geomorphometric attributes
The surface geomorphometry is a fundamental feature to describe the topographic character of a point on a land surface and the exact information about the earth's surface is of fundamental importance while describing the topographical features. The DEM is an important source of information of topography and permits a more detailed analysis of topographic data structure than is possible from conventional paper topographic contour maps. Geomorphometric parameters, derived from DEMs, can give valuable information on the characteristics of surface element. In order to assess and demonstrate the use of SRTM elevation data over the contour DEM at regional scale applications in the study area and other parts of India, the important geomorphometric parameters were extracted by reviewing the various parameters developed by various researchers (Pennock et al. Citation1987, Blaszczynski Citation1997, Shary et al. Citation2002, Zomer et al. Citation2002, Bolongaro-Crevenna et al. Citation2005, Guth Citation2006, Maathuis Citation2006, Tagil and Jenness Citation2008, Thomas et al. Citation2010). The parameters include relief/hillshade, slope, aspect, plan curvature, profile curvature, relative relief, length and steepness (LS) factor, wetness index, terrain convergence, topographical position index (TPI), slope position classification and landform classification.
Comparison of contour and SRTM-DEM derivatives
Contour DEM and SRTM-DEM are used in different GIS software packages and models for landform characterisation and to derive the most important geomorphometric parameters of the study area. The basic statistical parameters of the two DEMs and derived local geomorphometric parameters were examined and are given in .
Table 1. Comparison of estimated spatial morphometric parameters from contour and SRTM-DEM.
Among the two DEMs analysed, the contour DEM gives a minimum value of 40 m while that of the SRTM gives 30 m. The DEM from the digitised contours gives a maximum value of 2620 m while the respective higher value for the SRTM-DEM is 2635 m. The mean value of the two DEMs shows a minor difference of 5.32 only. The standard deviation of both the DEMs shows similar values. A series of derived map products and landscape analyses were carried out based on contour and SRTM-DEMs. These results were evaluated both in terms of their comparative accuracy and the usefulness of the respective DEMs for the purposes of that analysis and are discussed in detail in the following sections.
Relief and hillshade
Relief is one of the major factors that heavily influence landscape development. The DEM is an excellent source to derive relief or topographic attributes to assess the terrain characteristics and classify the region accordingly. A DEM at a spatial resolution corresponding to pixel size 90×90 m has been generated using the 3D analyst option of the ArcGIS software using the contours (20 m interval) and elevation points digitised from the topographic maps. The elevation surface thus generated has shown a range in elevation varying from 40 to 2620 m. At the same time, the elevation surface of SRTM data was showing value ranges between 33 and 2635 m. Cross-sectional profiles were generated for the study area using 90 m resolution DEM (both contour DEM and SRTM). The elevation profile of the contour DEM shows maximum match with the SRTM-DEM (). At the same time, the contour DEM shows more smoothened appearance than the SRTM-DEM.
Figure 2. Comparative relief profiles between selected points (X–Y) in contour and SRTM-derived DEM.
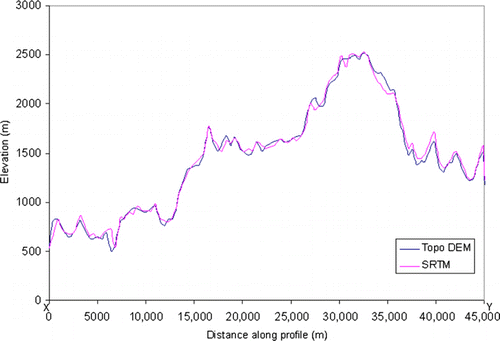
Hypsometric analysis describes the elevation distribution across an area of land surface. It is an important tool to assess and compare the geomorphic evolution of various landforms irrespective of the factor that may be responsible for it. The major factors governing the evolution of landscape are tectonics and/or climate and the variation in lithology (Hurtrez et al. Citation1999, Awasthi et al. Citation2002). Hypsometric curves are usually interpreted as youthful (convex upward curves), mature (S-shaped curves) and peneplain or old age (concave upwards curves) stages of landscape evolution. Convex hypsometric curves are more likely typical of a plateau with little erosion, which can evolve into an S shape, while concave hypsometric curves indicate greater erosion (Hurtrez et al. Citation1999). In the present study, a comparison between the contour and SRTM-DEM-based hypsometric analysis was carried out and it was found that both the DEMs have shown a similar pattern in the hypsometric curve with an area under curve/hypsometric integral of 19.70%. These also suggest the suitability of SRTM-DEM in the hypsometric analysis of the basin.
The hillshade image for the study area is generated to enhance the aesthetic qualities of the elevation grid and to help quick identification of hills and valleys ((a) and (b)). The hillshade function obtains the hypothetical illumination of a surface by determining illumination values for each cell in a raster and makes it a useful estimate of insolation. Both the DEMs with settings for sun location as 295° azimuth and 45° elevation have produced hillshades with grid cell values ranging from 0 to 255 based on how directly the grid cell faces the sun.
Slope and aspect
Surface slope and aspect are two of the most important characteristics of a terrain surface and are the two most commonly used products of an elevation surface. These play a crucial role in a number of morphological, ecological and hydrological processes. Slope is a controlling factor in earth surface processes as it influences surface flow, soil properties, water content and the erosion potential of an area. It is of great significance in hydrology and geomorphology as the means by which gravity induces stress in rocks, flux of water and other materials. The most important factor controlling the stability of slopes is the slope angle. In the present study, the surface steepness of slope is calculated in degrees for the DEMs. The slope map derived from the contour DEM and SRTM-DEM shows good similarity in basic statistics with a minimum value of 0 and a maximum of 66° with a mean of 15.39 for contour DEM and 16.26 for SRTM-DEM and standard deviation of 9.44 ((c) and (d)).
Aspect generally refers to the direction to which a mountain slope faces and is expressed in degrees, generally clockwise from −1 to 359°. An aspect value of −1 is frequently assigned to flat areas. Aspect distributes incident sunlight. Depending on the orientation of the earth surface in respect of the cardinal points (and slope), energy and moisture are redistributed, generating a wide range of processes that affect their course and duration. Solar radiation, the dominant component of the surface energy balance, influences ecologically critical factors of microclimate, including near-surface temperatures, evaporative demand and soil moisture content. The aspect image thus produced has a value ranging from −1 to 359° with a mean of 166.60 and 183.60 and standard deviation of 110.86 and 102.35, respectively, for contour DEM and SRTM-DEM.
Plan and profile curvatures
The curvature attributes are based on second derivatives: the rate of change of a first derivative such as slope or aspect, usually in a particular direction. The two curvatures, most frequently computed, are plan and profile curvatures. Plan curvature measures the rate of change of slope perpendicular to the down slope direction, indicating topographic convergence and divergence and hence the propensity of water to converge as it flows across the land. Profile curvature measures the rate of change of slope in the down slope direction and hence is important for characterising changes in flow velocity and sediment transport processes.
Plan and profile curvature classifies the terrain based on the shape of slope as convex (erosional), concave (probably depositional) or flat (erosional or depositional-depending on slope). Generally, the values of planar and profile curvature don't show rate of erosion, but both can indicate areas of dominant erosion or deposition by its convexity and concavity. Plan curvature generated for the study area shows lowest values −2.37 and −5.75 (concave out), respectively, for contour DEM and SRTM-DEM indicating deposition areas, as well as values near zero with flat curvature. But the highest values indicate dispersion areas (convex out) with more erosion and the respective values of contour DEM and SRTM-DEM (3.94 and 5.20, respectively). Similarly for the profile curvatures, the negative values (−4.10 and −4.06) indicate deposition areas (concave up). The positive values above 0.001 with the maximum values of 2.86 and 4.03, respectively, for contour DEM and SRTM-DEM indicate highly convex terrain (convex up) with high erosion potential. Flat areas are represented by a range of values between −0.001 and 0.001.
Relative relief and length and steepness (LS) factor
Elevation is useful to classify the local relief and locate points of maximum and minimum heights within terrains. Relative relief portrays the difference in elevation at a given point (Vijith and Madhu Citation2007). Runoff is higher and infiltration is lower in areas of steeper topography. The relative relief map was generated from the DEM using the neighbourhood range function available in the Spatial Analyst extension of ArcGIS. The generated relative relief map of both contour DEM and SRTM-DEM doesn't show much variation with each other. But the general statistics of the layers show slight variation in the maximum value (985 and 994), mean (243.83 and 234.87) and standard deviation (132.54 and 129.54) for contour DEM and SRTM-DEM.
The effect of topography on erosion is accounted for by the significant slope-related parameter, slope LS factor in RUSEL/USLE, which combines the effects of a hillslope-length factor (L), and a hillslope-gradient factor (S). Generally, as hillslope length and hillslope gradient increase, soil loss increases. As hillslope length increases, total soil loss and soil loss per unit area increase due to the progressive accumulation of runoff in the downslope direction. As the hillslope gradient increases, the velocity and erosivity of runoff increases (Moore and Willson Citation1992, Renard et al. Citation1997, Lastoria et al. Citation2008). In the case of contour DEM, values for LS factor range from 0 to 46.71 with mean and standard deviation of 4.06 and 3.88, respectively. At the same time, the SRTM-DEM derived LS factor shows a maximum value of 41.15 with a mean of 3.69 and standard deviation of 3.72. The high LS values are characteristic of areas with high elevation and slope, concentrated in the central and north-eastern part of the study area ((a) and (b)). It has been demonstrated that increase in LS can produce higher overland flow velocities and corresponding higher erosion.
Wetness index
Wetness index represents a theoretical measure of the accumulation of flow at any point within a river basin. It is defined as the natural logarithm of specific catchment area divided by the tangent of the slope based on the assumption that topography controls the movement of water in sloping terrain and thus the spatial pattern of soil moisture (Pradhan et al. Citation2006). The wetness index has a major role in determining a catchment's susceptibility to water-driven soil degradation and is generally used to represent the geomorphic processes associated with soil moisture and its spatial distribution in the landscape. Values of wetness index, generated from contour DEM and SRTM-DEM, do not show much variations in the lower and upper limit of the values (3.92–22.80 and 3.22–22.64, respectively, for contour and SRTM-DEMs), but the distribution pattern shows more spatial differences. The high values of wetness index are found in converging, flat terrains, whereas low values are typical for steep, diverging areas.
Topographical position index (TPI) based slope position classification and landform classification
TPI is the difference between the elevation at a cell and the average elevation in a neighbourhood surrounding that cell (Weiss Citation2001, Iampietro and Kvitek Citation2002, Iampietro et al. Citation2005, Jenness Citation2006). TPI is the simplification of the Landscape position described by Fels and Zobel (Citation1995) and was developed in detail by Weiss (Citation2001). The TPI values provide a simple and powerful means to classify the landscape into morphological classes. The derivation involves evaluating elevation differences between a focal point and the mean elevation of the surrounding cells within a user-defined rectangle, annulus or circle. Before processing to derive the TPIs from DEM, two important factors, namely, scale of the source data and landscape context must be considered. In the present analysis, circular neighbourhood of varying size (90, 250, 500, 750, 1000 and 1250 m) was used to derive TPI, the only one neighbourhood able to give good results (without loss of definition or structures) was the 1250 m. It is probable that while using a large radius some features might have been missed or dissolved into larger categories, while a small radius has given back wrong results because interpolation errors may be mistaken for distinct features. The resultant TPI images have shown values ranging from negative to positive. Positive TPI values represent locations that are higher than the average of their surroundings (ridges), as defined by the neighbourhood. Negative TPI values represent locations that are lower than their surroundings (valleys). TPI values near zero are either flat areas (where the slope is near zero) or areas of constant slope (where the slope of the point is significantly greater than zero).
Slope position classification
Landscapes can be classified into discrete slope position classes (valley, lower slope, flat, mid-slope, upper slope and hill top/ridges) using TPI values at a given scale by continuous thresholding and checking the slope for values near zero. Positive values mean the cell is higher than its surroundings while negative values mean it is lower. The extent to which it is higher or lower along with the slope of the cell, helps to classify the cell into slope position. If it is significantly higher than the surrounding neighbourhood, then quite likely it is at or near the top of a hill or ridge. Similarly, significantly low values suggest the cell is at or near the bottom of a valley. TPI values near zero could mean either a flat area or a mid-slope area and so the cell slope can be used to distinguish the two. In the present study, the two TPIs of different neighbourhood size (i.e. TPI 250 and 1250 m) were generated from contour and SRTM-DEM to derive slope position classification grids and are showing variation in spatial dimension slope position classes ((a) and (b)).
Landform classification
The classification of a topographical surface into different landforms is necessary, for landscape evaluation, suitability studies, erosion studies, hazard prediction and various fields of landscape and regional planning or land system inventories. The conventional process of landform classification by field investigation and aerial photo interpretation is both time- and money-consuming and the accuracy of the derived landscape map depends on the subjective skills of the surveyor/analyst. Recent advances in geocomputations enable the users to classify and describe the topographical characteristics by assessing the relationship between topography, process and landforms.
Landform category can be determined by classifying the landscape by combining TPIs at small- and large-scale grids at different scales. As a general rule, because elevation tends to be spatially autocorrelated, the range of TPI values increases with scale. A high TPI value in a small neighbourhood, combined with a low TPI value in a large neighbourhood, would be classified as a local ridge or hill in a larger valley, while a low small-neighbourhood TPI plus a high large-neighbourhood TPI would be classified as an upland drainage or depression. As used here, a landform is a natural physical feature on the earth's surface having a characteristic shape at a given scale, and produced by natural processes. Two TPI grids of neighbourhood size, TPI 100 and 1000 m, along with slope derived from the contour DEM and SRTM-DEM were used to classify the terrains into 10 discrete landform units. The landform classification is based on the criteria defined by Weiss (Citation2001) and the landform classes are deeply incised valley, mid-slope/shallow valley, upland drains, U-shaped valleys, small plains, open slope, upper slope, hills in valleys (local ridges), small hills in plain (mid-ridges) and ridges ((a) and b)).
Discussion and conclusion
The paper is mainly intended to compare landform classes and local geomorphometric parameters derived from the topographic contour and SRTM data for the purpose of evaluating the applicability and acceptability of SRTM-DEM for regional scale studies. The comparison of simple statistical parameters of both DEMs has shown only very minor differences in the minimum (33 and 40 m) and maximum (2635 and 2620 m) values, respectively, for the SRTM and contour DEM. To assess the usability of SRTM-DEM in the field of geomorphology and geosciences, important geomorphometric parameters were derived and landform classifications were performed using the available GIS softwares for both the DEMs. The parameters include relief/hillshade, slope, aspect, plan curvature, profile curvature, relative relief, LS factor, wetness index, terrain convergence, TPI, slope position classification and landform classification. The statistical and spatial comparisons were performed for parameters derived from both the DEMs and it was observed that the derivative geomorphometric parameters and landform analysis outputs compare well with results based upon the conventional dataset (contour DEM).
The SRTM dataset provides an unprecedented view of the topography of most of the land surface. Its elevation distribution closely mirrors that of the traditional contour derived DEM. Once the SRTM data are ready for use, the number of possible applications is quite large and it shows high reliability at 90 m spatial resolution. As demonstrated here, in the absence of other available and acceptable elevation datasets, the SRTM-DEM will provide a timely and cost-effective way to assess the geomorphometric and landform characteristics of mountainous terrain, even under conditions of extreme topographic relief. In general, the SRTM-DEM offers a consistent representation of topography on a wide range of scales and it provides a faster and promising new means to obtain important terrain parameters which are relevant in geomorphologic and geoscience researches.
Notes on contributors
Dr. V. Prasannakumar is Professor of Geology at the University of Kerala and currently heads the Centre for Geo-Information Science and Technology (CGIST). He had his graduation in Geology from the University College, Trivandrum, and Post graduation and Doctoral degree from the Department of Geology, University of Kerala. His areas of research include microtectonics and shear zone kinematics and he did research in microtectonics at the Leeds University, UK, as a Commonwealth Academic Staff Fellow and in microstructures at the University of Oulu, Finland, as an Exchange Fellow. His research career focuses on the kinematic evolution of south Indian shear zones, application of remote sensing and GIS in earth system sciences and web enabled spatial data services.
R. Shiny worked as Junior Research Fellow in Centre for Geoinformation Science and Technology, University of Kerala. She was awarded the MSc in Environmental Sciences from University of Kerala. The broad goal of her contemporary research is to understand the influence of various environmental variables on landscape degradation through the application of remote sensing and GIS.
N. Geetha is currently working as Junior Research Fellow in Centre for Geoinformation Science and Technology, University of Kerala. She was awarded the MSc in Geoinformatics from Annamalai University, Tamil Nadu. Her research interests involve the assessment of spatial dynamics of land use/landcover and groundwater modelling.
Dr. H. Vijith is presently working as the Project Associate, Centre for Geoinformation Science and Technology, University of Kerala. He took his M.Sc. in Geology, PhD in Environmental Science from Mahatma Gandhi University, Kerala and MTech in Remote Sensing from Bharathidasan University, Tamil Nadu. His research programmes centre on the application of remote sensing and GIS in natural disaster management, land cover dynamics and fluvial geomorphology, data modelling and web enabled spatial data services.
Acknowledgements
This paper is a contribution of the Centre for Geoinformation Science and Technology, a joint venture of the University of Kerala, Department of Science and Technology, Government of India and Kerala State Council for Science Technology and Environment. Financial support from DST and KSCSTE is gratefully acknowledged. The authors are thankful to the anonymous reviewers for constructive comments and suggestions.
References
- Alan , Y. 2008 . Preliminary results for a semi-automated quantification of site effects using geomorphometry and ASTER satellite data for Mozambique, Pakistan and Turkey . Journal of Earth System Sciences , 117 ( S2 ) : 797 – 808 .
- Awasthi , K.D. 2002 . Land-use change in two Nepalese watersheds: GIS and geomorphometric analysis . Land Degradation and Development , 13 : 495 – 513 .
- Blaszczynski , J.S. 1997 . Landform characterization with geographic information systems . Photogrammetric Engineering and Remote Sensing , 63 ( 2 ) : 183 – 191 .
- Bolongaro-Crevenna , A. 2005 . Geomorphometric analysis for characterizing landforms in Morelos State, Mexico . Geomorphology , 67 : 407 – 422 .
- Chapman , C.A. 1952 . A new quantitative method of topographic analysis . American Journal of Science , 250 ( 6 ) : 428 – 452 .
- Demirkesen , A.C. 2008 . Digital terrain analysis using Landsat-7 ETM+ imagery and SRTM DEM: a case study of Nevsehir province (Cappadocia), Turkey . International Journal of Remote Sensing , 29 ( 14 ) : 4173 – 4188 .
- Desmet , P.J.J. and Govers , G. 1996 . Comparison of routing algorithms for digital elevation models and their implications for predicting ephemeral gullies . International Journal of GIS , 10 : 311 – 331 .
- El-Magd , I.A. and Hillman , P.F. 2009 . Geomorphological monitoring of a highly dynamic estuary using oblique aerial photographs . International Journal of Digital Earth , 2 ( 2 ) : 1 – 13 .
- Farr , T.G. and Kobrick , M. 2000 . Shuttle Radar Topography Mission produces a wealth of data . Atmospherics Geophysical Union EOS , 81 : 583 – 585 .
- Farr, T.G., et al., 2007. The Shuttle Radar Topography Mission. Reviews of Geophysics, 45. RG 2004. doi: 1029/2005RG000183
- Fels , J.E. and Zobel , R. , 1995 . Landscape position and classified land type mapping for state wide DRASTIC mapping project . North Carolina State University, Technical report , Raleigh, , North Carolina VEL. 95.1 to the North Carolina Department of Enivronment, Health, and Natural Resources, Division of Enivronmental Management
- Florinsky , I.V. 1998a . Accuracy of local topographic variables derived from digital elevation models . International Journal of Geographical Information Science , 12 ( 1 ) : 47 – 61 .
- Florinsky , I.V. 1998b . Combined analysis of digital terrain models and remotely sensed data in landscape investigations . Reports on Progress in Physical Geography , 22 : 33 – 60 .
- Gerrard , A.J. 1990 . Mountain environments: an examination of the physical geography of mountain , London : Belhaven Press .
- Gerstenecker , C. 2005 . Validation of digital elevation models around Merapi Volcano, Java, Indonesia . Natural Hazards and Earth System Sciences , 5 : 863 – 876 .
- Global Land Cover Facilities website , 2005 . Available from: http://glcfapp.umiacs.umd.edu/index.shtml [Accessed 10 March 2009] .
- Guth , P.L. 2006 . Geomorphometry from SRTM: comparison to NED . Photogrammetric Engineering & Remote Sensing , 72 ( 3 ) : 269 – 277 .
- Hurtrez , J.E. , Sol , C. and Lucazeau , F. 1999 . Effect of drainage area on the hypsometry from an analysis of small-scale drainage basins in the Siwalik hills (central Nepal) . Earth Surface Processes and Landforms , 24 : 799 – 808 .
- Iampietro , P. , and Kvitek , R.G. , 2002 . Quantitative seafloor habitat classification using GIS terrain analysis: effects of data density, resolution, and scale . In: Proceedings of the 22nd Annual ESRI User Conference , 8–12 July, San Deigo, CA.
- Iampietro , P. , Kvitek , R.G. and Morris , E. 2005 . Recent advances in automated genus-specific marine habitat mapping enabled by high-resolution multibeam bathymetry . Marine Technology Society , 39 ( 3 ) : 83 – 93 .
- Jenness , J. , 2006 . Topographic position index (tpi_jen.avx) extension for ArcView 3.x, v. 1.3a . Jenness Enterprises . Available from: http://www.jennessent.com/arcview/tpi.htm [Accessed 18 July 2009].
- Kamp , U. , Tobias , B. and Jeffrey , O. 2005 . Geomorphometry of Cerro Sillajhuay (Andes, Chile/Bolivia): comparison of digital elevation models (DEMs) from ASTER remote sensing data and contour maps . Geocarto International , 20 ( 1 ) : 23 – 33 .
- Klingseisen , B. , Metternicht , G. and Paulus , G. 2008 . Geomorphometric landscape analysis using a semi-automated GIS-approach . Environmental Modelling and Software , 23 ( 1 ) : 109 – 121 .
- Lastoria , B. 2008 . An estimated erosion map for the Aterno-Pescara river Basin . European Water , 21 ( 22 ) : 29 – 39 .
- Lee , H.Y. 2003 . Extraction of digital elevation models from satellite stereo images through stereo matching based on epipolarity and scene geometry . Image and Vision Computing , 21 : 789 – 796 .
- Maathuis , B.H.P. 2006 . Digital elevation model based hydro-processing . Geocarto International , 21 ( 1 ) : 21 – 26 .
- Moore , I.D. and Willson , J.P. 1992 . Length-slope factors for the revised universal soil loss equation: simplified method of estimation . Journal Soil and Water Conservation , 475 : 423 – 428 .
- Nair , N.G.K. , Santhosh , M. and Thampi , P.K. 1984 . Alkali granite-syenite – carbonatite association in Munnar Kerala, India, implications for rifting, alkaline magmatism and liquid immiscibility . Journal of Earth System Science , 99 ( 2 ) : 149 – 158 .
- Nikolarkopoulos , K.G. , Kamartakis , E.K. and Chrysoulakis , N. 2006 . SRTM vs ASTER elevation products. Comparison for two regions in Crete, Greece . International Journal of Remote Sensing , 27 ( 21 ) : 4819 – 4838 .
- Pennock , D.J. , Zebarth , B.J. and De Jong , E. 1987 . Landform classification and soil distribution in hummocky terrain . Geoderma , 40 : 297 – 315 .
- Pike , R.J. 2000 . Geomorphometry-diversity in quantitative surface analysis . Progress in Physical Geography , 24 ( 1 ) : 1 – 20 .
- Pike , R.J. and Wilson , S.E. 1971 . Elevation-relief ratio, hypsometric integral and geomorphic area-altitude analysis . Geological Society of America Bulletin , 82 ( 4 ) : 1079 – 1084 .
- Pradhan , N.R. , Tachikawa , Y. and Takara , K. 2006 . A downscaling method of topographic index distribution for matching the scales of model application and parameter identification . Hydrological Processes , 20 : 1385 – 1405 .
- Rabus , B. 2003 . The Shuttle Radar Topography Mission – a new class of digital elevation models acquired by space bourne radar . ISPRS Journal of Photogrammetry and Remote Sensing , 57 : 241 – 262 .
- Renard , K.G. 1997 . Predicting soil erosion by water: a guide to conservation planning with the Revised Universal Soil Loss Equation (RUSLE) . Agriculture Handbook, US Department of Agriculture , 703 : 1 – 251 .
- Rodriguez , E. , Morris , C.S. and Belz , J.E. 2006 . A global assessment of the SRTM performance . Photogrammetric Engineering and Remote Sensing , 72 : 249 – 260 .
- Shary , P.A. , Sharaya , L.S. and Mitusov , A.V. 2002 . Fundamental quantitative methods of land surface analysis . Geoderma , 107 : 1 – 32 .
- Singh , A.K. , Parkash , B. and Choudhury , P.R. 2007 . Integrated use of SRM, Landsat ETM+ data and 3D perspective views to identify the tectonic geomorphology of Dehradun valley, India . International Journal of Remote Sensing , 28 ( 11 ) : 2403 – 2414 .
- Soman , K. 2002 . Geology of Kerala , Bangalore, , India : Geological Survey of India .
- Strahler , A.N. 1952 . Hypsometric (area-altitude) analysis of erosional topography . Geological Society of America Bulletin , 63 ( 11 ) : 1117 – 1142 .
- Tagil , S. and Jenness , J. 2008 . GIS based automated landform classification and topographic, landcover and geologic attributes of land from around the Yazoren Polje, Turkey . Journal of Applied Sciences , 8 ( 6 ) : 910 – 921 .
- Thampi , P.K. , Nair , P.K.R. and Balasubramonian , G. 1993 . Petrochemistry and tectonic evolution of Munnar Granite, Idukki district, Kerala . Current Science , 64 ( 4 ) : 238 – 243 .
- Thomas , B.C. , Kuriakose , S.L. and Jayadev , S.K. 2009 . A method for groundwater prospect zonation in data poor areas using remote sensing and GIS: a case study in Kalikavu Panchayath of Malappuram district, Kerala, India . International Journal of Digital Earth , 2 ( 2 ) : 155 – 170 .
- Thomas , J. , Joseph , S. and Thrivikramaji , K.P. 2010 . Morphometric aspects of a small tropical mountain river system, the southern Western Ghats, India . International Journal of Digital Earth , 3 ( 2 ) : 135 – 156 .
- Toutin , T. 2001 . Elevation modelling from satellite VIR data: a review . International Journal of Remote Sensing , 22 : 1097 – 1125 .
- Vijith , H. and Madhu , G. 2007 . Application of GIS and frequency ratio model in mapping the potential surface failure sites in the Poonjar subwatershed of Meenachil river in Western Ghats of Kerala . Journal Indian Society of Remote Sensing , 33 ( 3 ) : 275 – 285 .
- Weiss , A. 2001 . Topographic position and landforms analysis . Geomorphology , 21 : 251 – 264 .
- Zevenbergen , L.W. and Thorne , C.R. 1987 . Quantitative analysis of land surface topography . Earth Surface Processes and Landforms , 12 : 47 – 56 .
- Zhang , T. 2010 . Ability to detect and locate gross errors on DEM matching algorithm . International Journal of Digital Earth , 3 ( 1 ) : 72 – 82 .
- Zomer , R. , Ustin , S. and Ives , J. 2002 . Using satellite remote sensing for DEM extraction in complex mountainous terrain: landscape analysis of the Makalu Barun National Park of Eastern Nepal . International Journal of Remote Sensing , 23 ( 1 ) : 125 – 143 .