Abstract
This study employs visible-near infrared and short wave infrared datasets of Advanced Spaceborne Thermal Emission and Reflection Radiometer (ASTER) to map salt diapirs and salt diapir-affected areas using Multi-Layer Perceptron (MLP) in the Zagros Folded Belt, Iran, and introduces the role of earth observation technology and a type of digital earth processing in lithological mapping and geo-environmental impact assessment. MLP neural network model with several learning rates between 0.01 and 0.1 was carried out on ASTER L1B data, and the results were compared using confusion matrices. The most appropriate classification image for L1B input to MLP was produced by learning rate of 0.01 with Kappa coefficient of 0.90 and overall accuracy of 92.54%. The MLP result of input data set mapped lithological units of salt diapirs and demonstrated affected areas at the southern and western parts of the Konarsiah and Jahani diapirs, respectively. Field observations and X-ray diffraction analyses of field samples confirmed the dominant mineral phases identified remotely. It is concluded that MLP is an efficient approach for mapping salt diapirs and salt-affected areas.
1. Introduction
Digital earth is a highly interdisciplinary field with offshoots extending into almost all scientific disciplines (Ehlers Citation2008, Foresman Citation2008, Gruen Citation2008). The application potential of digital earth stems from their unique advantages such as access to satellite and digital images (Gruen Citation2008) and providing the opportunity to detect and understand lithology and geo-environmental impacts. Mapping lithology of salt diapirs, especially salt rock exposures by earth observation technology, satellite-borne data processing, and digital image classification is the most important endeavor in environmental impact assessment and defining salt diapir-affected areas. Salt rock exposures and salinization of adjacent plains caused by this rock type is an environmental phenomenon that changes the chemical composition of water resources (rivers and groundwater), decreases the water quality, and consequently affects the agricultural productions. This affection is a major threat in semi-arid and arid regions such as Iran. Therefore, information on the extent of the salt rocks and salt diapir-affected areas is required for effective environmental planning and sustainable water resources management.
Exposure of more than 130 salt diapirs at the south eastern Zagros Folded Belt (ZFB), southern Iran, (Kent Citation1970) presents excellent opportunities for studying Infra-Cambrian salt diapirs and their prospective mineral resources, particularly potash and hematite. However, they pose considerable impact on the adjacent environments. Two of these salt diapirs, namely Konarsiah and Jahani, are exposed at SE Shiraz, Southern Firouzabad (). Assessing the spread of salinity by salt diapirs has traditionally been implemented by geochemical (Zadneek Citation2008), hydrological (Sabouki Citation1996, Zarei and Raeisi Citation2010), and geophysical methods (Ghanbarian Citation2007, Dehghan Ghasr Ahmadi Citation2008) that commonly require the collection and laboratory analysis of numerous samples, which could be challenging, time-consuming, and costly.
Figure 1. Geological map of the study area, southern Firouzabad, SE Shiraz, Iran (This modified map is part of the 1:100,000 Farrashband map from Sedaghat and Dabaghian Nezhad (1996).
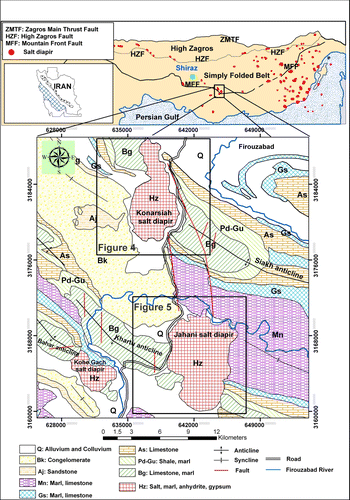
At the present time, remote sensing can provide the opportunity for mapping lithology, mineralogy, altered rocks, and environmental pollution, and is a useful tool for acquiring basic information, particularly on a regional scale (Sabins Citation1997). Many remote sensing techniques and datasets have already been used to map various minerals and lithologies (Rowan et al. Citation1977, Kruse et al. Citation2003, Rowan and Mars Citation2003, Tangestani et al. Citation2008, Bedini Citation2009) as well as salt-affected areas (Hick and Russell Citation1990, Mougenot et al. Citation1993, Rao et al. Citation1995, Metternicht Citation2001, Ben-Dor et al. Citation2002, Metternicht and Zink Citation2003, Khan et al. Citation2005, Farifteh et al. Citation2006, Citation2007). However, there is a lack of publication focusing on the application of remote sensing in mapping lithological units and detecting impact areas of salt diapirs. An unpublished work of Tavakkoli (Citation2008) used the Advanced Spaceborne Thermal Emission and Reflection Radiometer (ASTER) and Landsat-TM data, integrated with Principal Components Analysis (PCA) and Spectral Angle Mapper methods for enhancing the lithological units of the Jahani and Konarsiah salt diapirs, without assessing the extent of the salt diapir-affected areas in the adjacent plains.
To gain a better understanding of the salt diapir lithology and detecting salt diapir-affected areas, we used an integration of ASTER data and Multi-Layer Perceptron (MLP) neural network approach. The major objectives of this study was assessing the efficacy of MLP neural network to map lithology of the salt diapirs and salt rock exposures as well as detecting the salt diapir-affected districts in the study area.
2. Study area
The study area (52° 16′–52°33′E; 28°31′–28°53′N) is located in the ZFB, southeastern Shiraz, Iran, about 25km southwestern Firouzabad ().
The Hormoz Formation (Salt diapirs) was deposited in an evaporate basin during the late Precambrian-early Cambrian (Motiei Citation2001). In the Fars domain of the eastern ZFB, this basal formation is overlaid by 7–9 km of sedimentary rocks (Kent Citation1979, Talbot Citation1998). Many Hormoz diapirs had reached the surface by the Triassic time (Ala Citation1974), and others were outcropped during the Mio-Pliocene Zagros orogenic events (Talbot Citation1979). Central and northern parts of the study area are characterized by three salt diapirs that dominantly consist of gypsum, salt rock, marl and shale, red salty marl with oligist crystals, and black to dark gray crystalline limestone and anhydrite (Sedaghat and Dabaghian Nezhad Citation1996). The diapirs are surrounded by sedimentary rocks, which dominantly consist of limestone, marl, shale, and sandstone.
Jahani diapir () is one of the largest extrusions of salt currently active in the Zagros mountains (Talbot Citation1998). The maximum elevation of this diapir is about 1470 m above sea level. The maximum length and width of the Jahani are 9 and 6 km, respectively. The Konarsiah () is a complex diapir, consisting of two independent northern and southern vents (Zarei and Raeisi Citation2010). The maximum elevation of this diapir is about 1530 m above sea level, and its dimensions are 10.5 by 4.5 km. Sinkholes are the most significant karstic features of the Konarsiah (Zarei and Raeisi Citation2010) and Jahani salt diapirs.
3. Material and method
Visible-near infrared (VNIR) and short wave infrared (SWIR) datasets of ASTER were used to map lithology of salt diapirs and to detect salt diapir-affected areas using MLP neural network model. ASTER instrument measures reflected radiation in three bands between 0.52 and 0.86 µm (VNIR) and six bands from 1.6 to 2.43 µm (SWIR), with 15- and 30-m resolution, respectively (Fujisada Citation1995). The ASTER Level 1B data used in this study, acquired on 24 March 2001, was provided by the Land Processes Distributed Active Center (LP DAAC).
The following steps describe data processing and analysis of the ASTER bands: (1) spatial registration of the 30-m SWIR data to the 15-m VNIR data in order to producing images with 15×15 m pixels; (2) geometric correction of data using 1:25,000 topographic maps; (3) selection of training areas using PCA (Richards Citation1984) imagery, knowledge obtained from geological map, and the field works. PCA was performed on the data set to reduce the number of correlated image bands to form a small number of independent components to represent most of the variability carried by the multiple image bands, and to increase the interpretability of the components as combinations of multiple bands (Jing and Panahi Citation2006). PCA output results were used to create RGB color composite images to reduce the information included in the raw data into two or three bands without losing significant information (Monger Citation2002).
PCA statistics were accounted to select components with the highest information to be used in selecting training areas. shows the eigenvalues, variances, and total cumulative variances for the nine PC images of ASTER. It is evident from the table that the first three components represent 98.7% variances of the image data, while the components 4–9 contain only 1.3% of the information. So, the first three components were used to generate a color composite image which was applied for selecting the training areas. To do this, several regions of interest were extracted from ASTER imagery; (4) carrying out MLP neural network model with several learning rates between 0.01 and 0.1 on L1B input and creating hard classification images; and (5) accuracy assessment using confusion matrices. The flowchart of processes is shown in .
Figure 2. The flowchart of process steps. After preprocessing, training areas are selected by generating PCA color composite image, geological map, and field observation, L1B data set is input into the MLP neural network, hard classification images are generated for inputs. Then, most appropriate classification image is selected using accuracy assessment.
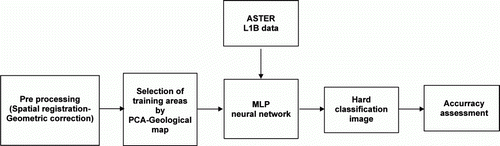
Table 1. PCA statistics of VNIR-SWIR ASTER bands of study area.
3.1. Multi-Layer Perceptron (MLP)
The MLP (Rumelhart and MacClelland Citation1986) is by far the most well known and most popular artificial neural network among all the existing neural network paradigms (Carvalho Citation2001, Hen Hu and Neng Hwang Citation2002) and offers a powerful method for analyzing complex relationships between variables without making assumptions about the data. ANNs are also capable of handling nonnormality, nonlinearity, and co-linearity in a system (Haykin Citation1994).
There are several examples of successful MLP applications on the hyperspectral and multispectral data in various disciplines such as agricultural remote sensing (Liu et al. Citation2001, Roosta et al. Citation2008), land cover classification (Kavzoglu and Mather Citation2003), image classification (Verbeke et al. Citation2004), and impervious surface estimation (Chormanski et al. Citation2008, Hu and Weng Citation2009). However, it is widely recognized that MLPs are sensitive to many operational factors including the size and quality of the training dataset, network architecture, training parameters, and over-fitting problems (Kavzoglu and Mather Citation2003, Yuan et al. Citation2009). The MLP parameters have to be set-up properly to find the global minimum of error function instead of a local minimum (Hu and Weng Citation2009). Global minimum is the lowest value of a function over the entire range of its input parameters, whereas local minimum is the minimum of a function over a limited range of input values (Demuth and Beale Citation2003).
A MLP neural network model used in the back-propagation learning algorithm has a sigmoid function and contains several neurons (nodes), each having several inputs. These neurons are organized in layers, labeled as the hidden layer 1, hidden layer 2, and the output layer. The number of the hidden layer nodes is estimated by Equation (Equation1);
where;
N h , N i , and N o are the number of hidden, input, and output layer nodes, respectively. During training, which is a key step in classification processes, the input that a single node receives is weighted using Equation (Equation2);
where;
w ij represents the weight between node i and j, and o i is output from node i. The output from given node j is then computed by the use of Equation (Equation3);
The function f is usually a nonlinear sigmoidal function that is applied to the weighted sum of inputs before the signal passes to the next layer. Once the forward pass is finished, the activities of the output nodes are compared with their expected activities. Each node in the output layer is associated with a class. When a pattern is presented to the network, each output node will generate a value that indicates the similarity between the input pattern and the corresponding class. Except in very unusual circumstances, the actual output will differ from the desired outcome; the difference is associated with error in the network. This error is then propagated backward with weights for relevant connections corrected via a relation known as the delta rule, which is presented in Equation (Equation4);
where;
η is the learning rate, α is the momentum factor, and ▵ is the computed error. The forward and backward passes continue until the network has ‘learned’ the characteristics of all the classes (Civco Citation1993, Atkinson and Tatnall Citation1997, Richards and Jia Citation1999, Chan et al. Citation2001).
3.2. Accuracy assessment
The accuracy of lithological units and salt diapir – affected areas were assessed by using confusion matrices approach (Richards Citation1994).
The overall accuracy is the percentage of correctly classified pixels and is calculated by summing the number of pixels classified correctly, divided by the total number of pixels. The pixels classified correctly are found along the diagonal of the confusion matrix table which lists the number of pixels that were classified into the correct ground truth class (Richards Citation1994). The overall accuracy (OA) is calculated by using Equation (Equation5);
where;
A is the number of pixels assigned to the correct class and B is the number of pixels that actually belong to that class.
The Kappa coefficient (K) (Cohen Citation1960) is a statistical measure of the agreement, beyond chance between two predicted rock units and test data (Chen et al. Citation2007), and is calculated by the use of Equation (Equation6);
where;
N is the total number of pixels in all the testing samples, Σ k X kk is the sum of the confusion matrix diagonal (X kk ), X kΣ is the sum of the testing samples in class k, and X Σk is the sum of the predicted pixels in class k (Cohen Citation1960). The Kappa coefficient is always in range of less than or equal to 1, where the latter indicates complete agreement, and is often multiplied by 100 to give a percentage measure of classification accuracy. The Kappa values are characterized into three groupings: values >0.80 (80%) represent strong agreement; values between 0.40 and 0.80 (40–80%) represents moderate agreement, while the values below 0.40 (40%) represent poor agreement (Congalton Citation1996). In this study, the test data were selected independently from the training data, but still were based on the geological map and field observations.
4. Results and discussion
The MLP was carried out on ASTER L1B data as the first layer (the input layer) with nine neurons (nine bands). Based on PC1, PC2, and PC3 color composite, existing geological map, and field observations, five training sites which represented the lithological units of salt diapirs and surrounding sedimentary rocks, including salt rock/salt affected-area, limestone, shale, gypsiferous soil, and sandstone were selected. Meanwhile, due to the presence of vegetation cover, mixed with rocks on the salt diapirs, ‘vegetation class’ was also selected and considered as a training area in classification. Fifty-seven pixels were used as training and testing areas for both implementing the MLP network and validating its results. Seven neurons were calculated to be used in the hidden layer and the six output layer nodes were defined based on the number of training categories. For balance between training time and overall error reduction, learning rates between 0.01 and 0.1 were used, and to reduce the oscillatory problems, momentum factor 0.5 was applied. To terminate the training process, the accuracy rate was set to 100% and iteration to 10,000.
The accuracy results of the MLP classification of the L1B data with several learning rates between 0.01 and 0.1 are displayed in . These results based on Congalton (Citation1996) category represent strong agreement for learning rates between 0.01 and 0.05, 0.07, and 0.08, and moderate agreement for learning rates 0.06, 0.09, and 0.1. The results also indicated that operating MLP with learning rate of 0.01, has the highest OA of 92.54% and a Kappa coefficient of 0.90 (), so the classification image produced by this learning rate () was used to map lithological units and salt diapir-affected areas.
Table 2. Kappa coefficient for the classification images.
illuminates the lithological units extracted from the L1B ASTER image by MLP neural network. The result shows good identification of salt rock/salt affected-area. However, some misclassifications occur due to the spectral similarities between salt rock/salt affected-area, limestone, sandstone, gypsiferous soil, and shale as well as alluvial or colluvial deposits (). Misclassification increases for limestone, shale, and in particular for sandstone, whose enhanced pixels generally correspond the alluvial or colluvial deposits. Misclassification of sandstone with limestone and shale is the major source of classification errors.
The confusion matrix of L1B input with learning rate of 0.01 to the MLP () shows good accuracy for salt rock/salt affected areas. For example, errors of commission and omission for salt rock/salt affected areas were 0.11 and 0.00. The salt rock has error of commission with shale and gypsiferous soil which means that 11% of the pixels that should be included as shale and gypsiferous soil are assigned to salt rock. In this classification image, errors of commission and omission for limestone were 0.07 and 0.00, respectively. However, shale class showed a decrease in error of commission and increase in error of omission. The gypsiferous soil has 14% error of omission with salt rock/salt affected areas, limestone, shale and sandstone, and 25% error of commission with vegetation and sandstone. The confusion matrix also indicates that sandstone has 0.14 and 0.38 errors of commission and omission, respectively.
Table 3. Confusion matrix for the most accurate classification image of L1B input.
Classification image of the study area shows Konarsiah salt diapir in elliptical shape (). The salt rocks are exposed only in limited parts of the steep margins of the salt diapir and on the bottom and walls of some of the sinkholes (photographs b and c in ). The cap rocks of this salt diapir mainly consist of gypsiferous soil, limestone, and sandstone (photograph a in ). Salt rocks mainly occur at the western, southern, and southeastern parts of the Konarsiah salt diaper, and a salt-affected area is present at the southern part of this salt diapir ().
Figure 4. Hard classification image of Konarsiah salt diapir. Red boxes illustrate the locations of limestone (Photograph a), salt rocks (Photographs b and c), and salt-affected area (Photograph c).
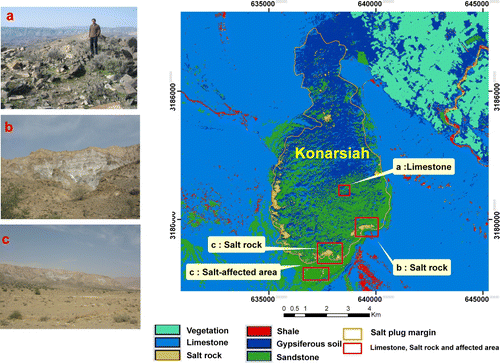
Exposures of salt rock for the Jahani occur mainly at the center, eastern, and northeastern parts of the salt diapir (). Moreover, some salt rock exposures were mapped at the western steep margins of the Jahani (photograph a in ). Salt rock exposures at northeastern part of the salt diapir can have an important role in pollution of the Firouzabad River (). In general, the most distinctive lithological units at the salt diapir are cap rocks including the gypsiferous soil, limestone, and sandstone. In addition to the salt rock exposure, a salt-affected area is present at the western part of the Jahani salt diapir (photographs b and c in ).
Figure 5. Hard classification image of Jahani salt diapir. Red boxes illustrate the locations of salt rock (Photograph a) and salt-affected area (Photographs b and c).
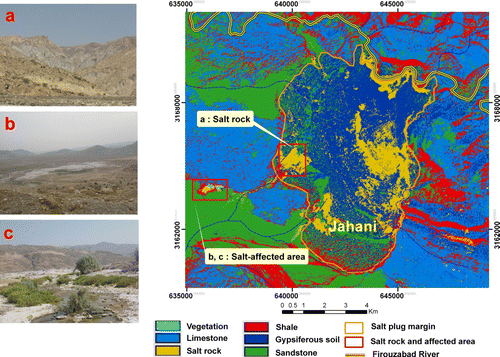
The salt diapirs and salt diapir-affected areas were field checked and ground data were collected and used to evaluate the accuracy of mapping results and to provide sufficient information about the mineralogy of affected-areas. Field check of salt-affected areas showed that soil and vegetation have been severely affected by the water-soluble salts. Forty-three rock, soil, and sediment samples were collected from salt diapirs, salt-affected areas and stream channels in the surrounding areas, and were tested by X-ray diffraction analysis. Results showed halite, calcite, dolomite, and quartz as major, and quartz, dolomite, albite, muscovite, and orthoclase as minor phases.
5. Conclusion
This study used an integration of satellite digital images and a type of digital earth processing to map lithological units of salt diapir and detect salt diapir-affected areas in the South eastern Shiraz, Iran. The target areas were mapped using the L1B input to the MLP approach.
Results showed that the salt rocks are exposed only in limited steep margins of the Konarsiah and Jahani salt diapirs and on the bottom and walls of some of the sinkholes. It was also revealed that the salt diapirs mainly consist of gypsiferous soil, limestone, and sandstone at the surface. In addition, some affected areas were discriminated by MLP neural network at the southern and western parts of the Konarsiah and Jahani diapirs, respectively. Field check showed that soil and vegetation in western part of the Jahani have been severely affected by the water-soluble salts. It seems that as water flows over and through the Jahani salt diapir, it picks up and carries salt toward the outlet of the basin. Therefore, it is necessary to plan reducing its impact on the water quality. It is concluded that MLP approach is effective for mapping salt diapirs and salt-affected areas at the study area. However, it is sensitive to operational factors such as learning rate and is time consuming.
While this method can identify salt rock exposures and also help discriminate the lithological units of salt diapirs, it is somewhat difficult to delineate the actual salt diapirs in the study area without prior knowledge of their spatial extent. In order to identify and truly delineate salt diapirs, particularly those that are unmapped, it may also be necessary to consider integrating topographic information into the mapping approach.
Notes on contributors
Mohammad Hassan Tayebi is a Ph.D. student at the Department of Earth Sciences, Shiraz University, Iran. He holds a B.Sc in Geology from Shahid Bahonar University, Iran and an M.Sc in Tectonic from Shiraz University, Iran. He is interested in research works on the applications of remote sensing and GIS in geological mapping, mineral exploration.
Majid H. Tangestani is an associate professor at the Department of Earth Sciences, and the director of the ‘Remote Sensing and GIS Research Center’ of the Shiraz University, Iran. He holds an M.Sc in Geology from Kerman University, Iran and a Ph.D. in application of Remote Sensing and GIS in porphyry copper exploration from the Shiraz University, Iran. His research area is applications of remote sensing and GIS in geosciences.
Hasan Roosta is a lecturer at the Department of Surveying Engineering and the director of Map Explorer institute in Shiraz, Iran. He holds a B.Sc in Surveying Engineering from Amir Kabir University of Technology, Iran and an M.Sc in application of Remote Sensing from Tehran University, Iran. His research area is applications of remote sensing and image processing in geosciences.
Acknowledgements
The ASTER data were provided by the land Processes Distributed Active Center (LP DAAC), located at the US Geological Survey (USGS) Earth Resources Observation and Science (EROS) Center. The authors thankfully acknowledge the constructive suggestions received from the IJDE's editor, Huadong Guo, and two anonymous reviewers.
References
- Ala , M.A. 1974 . Salt diapirism in south Iran . AAPG , 58 : 1758 – 1770 .
- Atkinson , P.M. and Tatnall , A.R.L. 1997 . Neural networks in remote sensing – introduction . International Journal of Remote Sensing , 18 ( 4 ) : 699 – 709 . doi: 10.1080/014311697218700
- Bedini , E. 2009 . Mapping lithology of the Sarfartoq carbonatite complex, southern West Greenland, using HyMap imaging spectrometer data . Remote Sensing of Environment , 113 : 1208 – 1219 . doi: 10.1016/j.rse.2009.02.007
- Ben-Dor , E. 2002 . Mapping of several soil properties using DAIS-7915 hyperspectral scanner data: a case study over clayey soils in Israel . International Journal of Remote Sensing , 23 ( 6 ) : 1043 – 1062 . doi: 10.1080/01431160010006962
- Carvalho , L.M.T. , 2001 . Mapping and monitoring forest remnants: a multiscale analysis of spatio-temporal data . Thesis (PhD). Wageningen University , Wageningen , The Netherlands .
- Chan , J.C. , Chan , K.P. and Yeh , A.G.O. 2001 . Detecting the nature of change in an urban environment: a comparison of machine learning algorithms . Photogrammetric Engineering and Remote Sensing , 67 ( 2 ) : 213 – 225 .
- Chen , X. , Warner , T.A. and Campagna , D.J. 2007 . Integrating visible, near infrared and short wave infrared hyperspectral and multispectral thermal imagery for geologic mapping: simulated data . International Journal of Remote Sensing , 28 ( 11 ) : 2415 – 2430 . doi: 10.1080/01431160600702624
- Chormanski , J. 2008 . Improving distributed runoff prediction in urbanized catchments with remote sensing based estimates of impervious surface cover . Sensors , 8 : 910 – 932 . doi: 10.3390/s8020910
- Civco , D.L. 1993 . Artificial neural networks for land cover classification and mapping . International Journal of Geographic Information Systems , 7 ( 2 ) : 173 – 186 . doi: 10.1080/02693799308901949
- Cohen , J. , 1960 . A coefficient of agreement for nominal scales . Educational and Psychological Measurement , 20 , 37 – 44 . doi: 10.1177/001316446002000104
- Congalton , R.G. , 1996 . Accuracy assessment: a critical component of land cover mapping . Proceedings of the American Society for Photogrammetry and Remote Sensing/Gap Analysis Program Symposium , 27 February – 2 March 1995 , Charlotte, NC .
- Dehghan Ghasr Ahmadi , M. , 2008 . Geological investigation of Sarvestan salt dome and it's influence on the groundwater . Thesis (MSc). Department of Earth Sciences, Shiraz University , Iran .
- Demuth , H. and Beale , M. , . Neural network toolbox for use with MATLAB, User's guide, Version 4.1 2003 Natrick: The Mathworks Press , New York , USA .
- Ehlers , M. 2008 . Geoinformatics and digital earth initiatives: a German perspective . International Journal of Digital Earth , 1 ( 1 ) : 17 – 30 . doi: 10.1080/17538940701781975
- Farifteh , J. , Farshad , A. and George , R.J. 2006 . Assessing salt-affected soils using remote sensing, solute modelling and geophysics . Geoderma , 130 : 191 – 206 . doi: 10.1016/j.geoderma.2005.02.003
- Farifteh , J. , Van Der Meer , F. and Carranza , E.J.M. 2007 . Similarity measures for spectral discrimination of salt-affected soils . International Journal of Remote Sensing , 28 ( 23 ) : 5273 – 5293 . doi: 10.1080/01431160701227604
- Foresman , T.W. 2008 . Evolution and implementation of the digital earth vision, technology and society . International Journal of Digital Earth , 1 ( 1 ) : 4 – 16 . doi: 10.1080/17538940701782502
- Fujisada , H. , 1995 . Design and performance of ASTER instrument . In : H. Fujisads and M.N. Sweeting , Proceedings of SPIE (International Society for Optical Engineering) , 25–28 September 1995 , Paris , France , 2583 , 16 – 25 .
- Ghanbarian , M.A. , 2007 . Tectonic and geoelectrical studies of salt domes in southern fars with emphasis on groundwater quality . MSc Thesis (MSc). Department of Earth Sciences, Shiraz University , Iran .
- Gruen , A. 2008 . Reality-based generation of virtual environments for digital earth . International Journal of Digital Earth , 1 ( 1 ) : 88 – 106 . doi: 10.1080/17538940701782585
- Haykin , S. , 1994 . Neural networks: a comprehensive foundation . Upper Saddle River, NJ7 Prentice Hall .
- Hen Hu , Y. and Neng Hwang , J. , 2002 . Hand book of neural network signal processing . Boca Raton: CRC Press .
- Hick , P.T. and Russell , W.G.R. 1990 . Some spectral considerations for remote sensing of soil salinity . Australian Journal of Soil Research , 28 : 417 – 431 . doi: 10.1071/SR9900417
- Hu , X. and Weng , Q. 2009 . Estimating impervious surfaces from medium spatial resolution imagery using the self-organizing map and multi-layer perceptron neural networks . Remote Sensing of Environment , 113 : 2089 – 2102 . doi: 10.1016/j.rse.2009.05.014
- Jing , Q.C.L. and Panahi , A. 2006 . Principal component analysis with optimum order sample correlation coefficient for image enhancement . International Journal of Remote Sensing , 27 ( 16 ) : 3387 – 3401 . doi: 10.1080/01431160600606882
- Kavzoglu , T. and Mather , P.M. 2003 . The uses of backpropagating artificial neural networks in land cover classification . International Journal of Remote Sensing , 24 ( 23 ) : 4907 – 4938 . doi: 10.1080/0143116031000114851
- Kent , P.E. 1970 . The salt of the Persian Gulf region . Transactions of the Leicester Literary & Philosophical Society , 64 : 56 – 88 .
- Kent , P.E. 1979 . The emergent Hormuz salt plugs of southern Iran . Journal of Petrolium Geology , 2 : 117 – 144 . doi: 10.1111/j.1747-5457.1979.tb00698.x
- Khan , M. 2005 . Assessment of hydrosaline land degradation by using a simple approach of remote sensing indicators . Agriculture Water Management , 77 : 66 – 109 . doi: 10.1016/j.agwat.2004.09.038
- Kruse , F.A. , Boardman , J.W. and Huntington , J.F. 2003 . Evaluation and validation of EO-1 Hyperion for mineral mapping . IEEE Transactions on Geoscience & Remote Sensing , 41 : 1388 – 1400 . doi: 10.1109/TGRS.2003.812908
- Liu , J. , Goering , C.E. and Tian , L. 2001 . A neural network for setting target corn yields . Transactions of the ASAE , 44 ( 3 ) : 705 – 713 .
- Metternicht , G.I. 2001 . Assessing temporal and spatial changes of salinity using fuzzy logic, remote sensing and GIS. Foundations of an expert system . Ecological Modelling , 144 : 163 – 179 . doi: 10.1016/S0304-3800(01)00371-4
- Metternicht , G.I. and Zink , J.A. 2003 . Remote sensing of soil salinity: potential and constraints . Remote Sensing of Environment , 85 : 1 – 20 . doi: 10.1016/S0034-4257(02)00188-8
- Monger , M. 2002 . Soil classification in arid lands with Thematic Mapper data . Terra , 20 ( 2 ) : 89 – 100 .
- Motiei , H. , 2001 . Simplified table of rock unit in south west Iran. Keyhan Exploration and Production Services, Tehran .
- Mougenot , B. , Pouget , M. and Epema , G.F. 1993 . Remote sensing of salt-affected soils . Remote Sensing Reviews , 7 : 241 – 259 . doi: 10.1080/02757259309532180
- Rao , B.R.M. 1995 . Spectral behaviour of salt-affected soils . International Journal of Remote Sensing , 16 ( 12 ) : 2125 – 2136 . doi: 10.1080/01431169508954546
- Richards , J.A. 1984 . Thematic mapping from multitemporal image data using principal components transformation . Remote Sensing of Environment , 16 : 35 – 46 . doi: 10.1016/0034-4257(84)90025-7
- Richards , J.A. 1994 . Remote sensing digital image analysis – an introduction , New York : Springer-Verlag .
- Richards , J.A. and Jia , X. 1999 . Remote sensing digital image analysis – an introduction , 3rd ed , Berlin : Springer-Verlag .
- Roosta , H. , Farhudi , R. , and Afifi , M. , 2008 . Comparison between sub-pixel classifications of MODIS images: linear mixture model and neural network model . WSEAS Transactions on Environment and Development , 4 2 , 161 – 168
- Rowan , L.C. , Goetz , A.F.H. and Ashley , R.P. 1977 . Discrimination of hydrothermally altered and unaltered rocks in visible and near infrared multispectral images . Geophysics , 42 ( 3 ) : 522 – 535 . doi: 10.1190/1.1440723
- Rowan , L.C. and Mars , J.C. 2003 . Lithologic mapping in the Mountain Pass, California, area using Advanced Spaceborne Thermal Emission and Reflectance Radiometer (ASTER) data . Remote Sensing of Environment , 84 : 350 – 366 . doi: 10.1016/S0034-4257(02)00127-X
- Rumelhart , D.E. and MacClelland , J.L. 1986 . Parallel distributed processing. Explorations in the Microstructure of Cognition , Vol. I , Cambridge , MA : MIT Press .
- Sabins , F.F. 1997 . Remote sensing, principles and interpretation , 3rd edn , New York : Freeman .
- Sabouki , A. , 1996 . Hydrogeology of Sarvestan salt diapir . Thesis (MSc). Department of Earth Sciences, Shiraz University , Iran .
- Sedaghat , M.E. and Dabaghian Nezhad , A. 1996 . Geological map of the Farrashband, scale: 1:100,000 , Tehran , , Iran : Geological Survey of Iran .
- Talbot , C.J. 1979 . Fold trains in a glacier of salt in southern Iran . Journal of Structural Geology , 1 : 5 – 18 . doi: 10.1016/0191-8141(79)90017-8
- Talbot , C.J. 1998 . Extrusions of Hormuz salt in Iran. In: D.J. Blundell and A.C. Scott, eds. Lyell: the past is the key to the present. London: Geological Society. Special Publications . 143 : 315 – 334 .
- Tangestani , M.H. 2008 . Evaluating Advanced Spaceborne Thermal Emission and Reflection Radiometer (ASTER) data for alteration zone enhancement in a semiarid area, northern Shahr-e-Babak, SE Iran . International Journal of Remote Sensing , 29 : 2833 – 2850 . doi: 10.1080/01431160701422239
- Tavakkoli , H. , 2008 . Comparison of TM and ASTER datasets for enhancement of lithological units of salt domes, a case study from Firouzabad area . Thesis (MSc). Department of Earth Sciences, Shiraz University , Iran .
- Verbeke , L.P.C. , Vancoillie , F.M.B. and De Wulf , R.R. 2004 . Reusing back-propagation artificial neural networks for land cover classification in tropical savannahs . International Journal of Remote Sensing , 25 : 2747 – 2771 . doi: 10.1080/01431160310001652385
- Yuan , H. , Van Der Wiele , C.F. and Khorram , S. 2009 . An automated artificial neural network system for land use/land cover classification from Landsat TM imagery . Remote Sensing , 1 : 243 – 265 . doi: 10.3390/rs1030243
- Zadneek , T. , 2008 . Environmental effects of Konarsiah salt dome on pollution of water resource of Firouzabad area Thesis (MSc). Department of Earth Sciences, Shiraz University , Iran .
- Zarei , M. and Raeisi , E. 2010 . Karst development and hydrogeology of Konarsiah salt diapir, south of Iran . Carbonates Evaporites , 25 : 217 – 229 . doi: 10.1007/s13146-010-0027-0