Abstract
Because the removal of topographic effects is one the most important pre-processing steps when extracting information from satellite images in digital Earth applications, the problem of differential terrain illumination on satellite imagery has been investigated for at least 20 years. As there is no superior topographic correction method applicable to all areas and all images, a comparison of topographic normalization methods in different regions and images is necessary. In this study, common topographic correction methods were applied on an ALOS AVNIR-2 image of a rugged forest area, and the results were evaluated through different criteria. The results show that the simple correction methods [Cosine, Sun-Canopy-sensor (SCS), and Minnaert correction] are inefficient in exceptionally rough forests. Among the improved correction methods (SCS+C, modified Minnaert, and pixel-based Minnaert), the best result was achieved using a pixel-based Minnaert approach in which a separate correction factor in various slope angles is used. Thus, this method should be considered for topographic correction, especially in forests with severe topography.
1. Introduction
Topographic effects have long been a time problem in classification and image interpretation when extracting useful information from satellite imagery in digital Earth applications (Wylie et al. Citation2008, Fadhil Citation2010, Panagos et al. Citation2011), especially in mountainous areas because of the large variations in the Sun incident angle and ground slope. In such regions, and for the same surface coverage (i.e., the same vegetation cover), orientation away from and toward the sun will cause the reflectance to appear darker or brighter than expected. This behavior causes problems in image classification and thematic scene interpretation, so topographic correction has always been a vital step in image processing in rugged areas.
In recent studies, a wide range of topographic correction methods has been applied to different images (especially TM and Système Probatoire d'Observation de la Terre (SPOT)) to eliminate or reduce these effects (Justice et al. Citation1981, Teillet et al. Citation1982, Civco Citation1989, Sandmeier and Itten Citation1997, Richter Citation1998, Riano et al. Citation2003, Shepherd and Dymond Citation2003, Richter et al. Citation2009). Some papers used Lambertian normalization methods (Justice et al. Citation1981, Teillet et al. Citation1982, Sandmeier and Itten Citation1997), while others employ Sun-sensor viewing geometry (Richter 1999, Riano et al. Citation2003).
The most common topographic correction methods have been compared based on the accuracy of the classification of vegetation cover in Landsat TM images, and the C correction approach was ranked the best (Riano et al. Citation2003). The three most frequently used topographic correction methods (C correction, Gamma, and Minnaert methods) have been applied to two different areas (Israel and Switzerland) in Landsat and SPOT images, and the modified Minnaert approach produced the best results (Richter et al. Citation2009). Although each paper yielded good results in the investigated region, there is no clear consensus on a method that is generally applicable to all images and areas; this lack of consensus is the main difficulty in performing topographic normalization (Riano et al. Citation2003).
A comparison of different topographic corrections in all geographical areas and on different images would be beneficial to future studies but is outside of the scope of a single paper. Some studies have already been conducted in this field, and this paper is a continuation of previous researches. In this study, the most commonly used topographic correction methods, including Cosine correction, C correction, SCS (Sun-Canopy-sensor), SCS+C, Minnaert, modified Minnaert, and pixel-based Minnaert were performed to reduce topographic effects on the ALOS AVNIR-2 data of a rugged forested area, and the results have been evaluated and compared.
2. Study area and dataset
2.1. Study area
The forest area chosen for this study () was the Kheyroud Kenar forest (51°33′12′′E and 51°39′56′′E longitude and 36°32′08′′N and 36°36′45′′N latitude) which is located about 7 km to the east of Noshahr in Mazandaran province, Iran. It is a portion of Hyrcanian ancient forests of Iran located at the northern slopes of Alborz Mountains with an area about 8000 ha. The terrain in this area is severely rough, ranging from a highest elevation from 2500 m along the ridges to 100 m in the foot of the valleys. The majority of slopes are 50% with an average value of 20 degrees.
Figure 1. Study area (a) on the map, (b) ALOS AVNIR image, and (c) digital elevation model (DEM) of the study area.
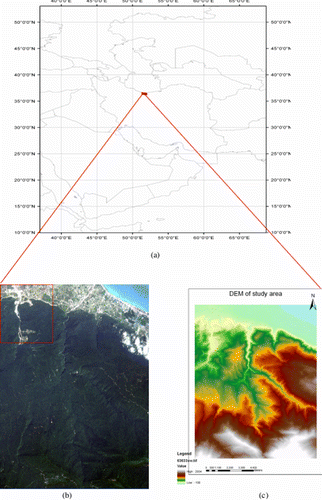
The density of vegetation cover of the area is between 75% and 100%. The main species of the trees in this region in order of importance are beech (Fagus sylvatica), hornbeam (Carpinus betulus), alder (Alnus Glutinousa), maple (Acer Velotonia), and elm (Ulmus). Because of the differences in elevation, humidity, and terrain condition, the distribution and density of trees vary greatly. At higher elevations the species like Fagus and Carpinus are dominant while in the flat areas the main species are mixed.
The study area is a mixed landscape including forest, agriculture, built up, and water, but the assessments are performed on the forest part of the landscape. The complex topography of the forest part of the study area is ideal for exploring the topographic correction methods to reduce the topography effects in rugged forests.
2.2. Dataset
The study was performed using a mid-summer ALOS AVNIR-2 scene data acquired on 6 June 2008. The image is cloud free and has a high sun elevation angle (70°) which provides good illumination conditions and minimizes topographic effects. Digital elevation model (DEM) with 10 m spatial resolution which was produced based on the 1:25,000 topographic map of the study area with an altimetric precision <6 m and vegetation map of the area produced by the Forest, Range and Watershed Organization of Iran are also used.
The ortho-rectification process was performed based on the set of 35 ground control points extracted from 1:25,000 scale map of the study area. The geometric correction was assessed using some check points which were also extracted from the 1:25,000 scale map, and the consequence calculated error was about half of a pixel (x=0.45, y=0.34). The nearest neighborhood resampling method was also used (Riano et al. Citation2003). The atmospheric correction based on the dark object subtraction method was also applied (Chavez Citation1988, Citation1996).
3. Methodology
The ALOS AVNIR-2 image of the study area was subject to the test of common topographic correction methods. The Cosine correction method (with the Lambertian assumption) has been compared with improved normalization approaches (C, SCS, and SCS+C) and semi-empirical correction methods (Minnaert, modified Minnaert, and pixel-based Minnaert).
In the first step, the results of the correction methods were compared by the visual quality of the image after performing the correction and the best methods have been selected. In the next step, an accuracy assessment was performed according to three different criteria (spectral coherency, class homogeneity, and classification accuracy). Finally, the best method was selected according to the accuracy assessment results. A summary of the methodology of this study is presented in .
3.1. Topographic correction methods
This section offers a brief survey of the most common topographic correction methods used in this study. Topographic normalization approaches can be divided into two groups. The first group is based on the band ratio, which assumes that the reflectance changes proportionally in all bands so that the ratio between them compensates for the topographic effects (Jansa Citation1998). This assumption is not accurate for the diffuse component of light because it is different in each band (Conese et al. 1993, Gu and Gillespie Citation1998).
Another problem of using these methods is the loss of spectral resolution, especially when performing classification on multispectral images (Riano et al. Citation2003). The second group relies on DEMs to normalize topographic effects. These methods use DEMs to calculate the local illumination angle, which is defined as the angle between the normal to the pixel surface and the solar zenith direction (Civco Citation1989).
θ s , θ n , ϕ s , and ϕ n denote the solar zenith angle, the terrain slope, the solar azimuth, and the topographical azimuth, respectively. The local illumination angle (β) can be calculated using the slope angle and aspect and the Sun geometry as follows:
where x, y indicate the pixel coordinate of the image.
The most widely used and the simplest method is Cosine correction, which assumes the ground is a Lambertian surface and that reflectance is independent of the incidence angle (Teillet et al. Citation1982, Civco Citation1989). If ρ H and ρ T represent the reflectance of horizontal and inclined terrain, respectively, then the Cosine correction method is defined as below:
It should be noted that when cos β tends toward 0 (in low illumination areas), the corrections would be too large, so these parts of the image are overcorrected (Justice et al. Citation1981). As many of the previous researches have shown, the Lambertian assumption is simple to perform; however, it is unrealistic because in most areas, the terrain is rugged and exhibits non-Lambertian behavior. Therefore, the Cosine correction usually does not produce accurate results.
A modification made to the Cosine correction method is called C correction, which is defined as follows (Teillet et al. Citation1982):
where the parameter C k is the slope of the regression line between the surface reflectance (ρ T ) and the local illumination angle (β). The C k is calculated for each channel separately, and the k index indicates the band number. Applying the parameter C k prevents overcorrection in low illumination areas.
Another modification of the Cosine correction is called the SCS correction, which was proposed to reduce the topographic effect in vegetated areas (Gu and Gillespie Citation1998, Citation1999, Dymond and Shepard Citation1999). This method is equivalent to projecting the digital number (DN) values of pixels from the sloped surface to the horizontal surface in the direction of illumination (Soenen et al. Citation2005). The SCS correction method is defined as follows:
This method preserves the geometry of the Sun, sensor, and canopy, and it improves the Cosine correction. However, this method neglects the diffuse component of light, so it may lead to overcorrection in faintly illuminated areas (Soenen et al. Citation2005).
The cause of overcorrection in the SCS correction is similar to that of the Cosine method. The SCS correction was improved (Soenen et al. Citation2005), and a correction factor was added to compensate for the neglect of diffuse light in the Cosine correction. Similar to the C correction, the C parameter was used. The SCS+C correction method is defined as follows:
where exponent k usually between 0 and 1 represents the extent in which a surface is non-Lambertian. The value of Minnaert constant (k) is calculated from Equation (Equation6) as
The above equation shows that the value of k can be calculated based on regression analysis and should be computed for each band before performing a correction.
The Minnaert method has improved the accuracy of the results compared with the Cosine correction in many of the past studies (Justice et al. Citation1981, Itten and Meyer Citation1992, Sandmeier and Itten Citation1997, Richter et al. Citation2009). However, in weakly illuminated areas (when cos β tends to 0), the Minnaert method causes an overcorrection similar to the Cosine method. Different methods have been developed for computing the k value. One simple method is to use a single global k value for an entire image, based on the assumption that the reflectance is homogenous over the study area.
However, this assumption is not valid because of differences in topographic impacts in areas with different slopes and aspects (Lu et al. Citation2008). The second approach for k computation is related to land cover classes which assumes that land covers have a strong influence on reflectance (Bishop and Colby Citation2002, Bishop et al. Citation2003). A similar approach for the computation of multiple k values is based on the stratification of such zones as relatively flat and mountainous regions (Belsius and Weirich Citation2005).
In this study, the forest cover part of the image has been stratified into different areas based on the slope ranges, and for each group the k value has been calculated. This method has been tested on Landsat ETM+ images of a mountainous region without vegetation cover in China (Lu et al. Citation2008).
The samples are extracted for every 5 pixels from the entire image. After removing pixels with slopes <1 degree, the samples are separated into 11 groups based on the slope values. The k values were then computed for each group in each band separately based on the selected sample data. The specific k values for the slope groups are presented in .
Table 1. The specific k values for each slope groups in pixel-based Minnaert method.
Another modification of the Minnaert method was employed based on some empirical rules (Richter 1999). This modified method has been tested on many different areas with severe topography, and the results were satisfying (Richter 1999, Citation2009). As a first step in this method, the local illumination angle is computed, and if it exceeds a threshold (β T ), then the corrected reflectance is computed as
To determine the value of β T and exponent b, the following rules are used:
• | if θ s < 45 then β T =θ s +20 | ||||
• | if 45 ≤ θ s ≤ 55 then β T =θ s +15 | ||||
• | if 55<θ s then β T =θ s +10 | ||||
• | exponent b=1/2 for non-vegetation | ||||
• | exponent b=3/4 for vegetation in the visible spectrum(λ< 720 nm) | ||||
• | exponent b=1/4 for vegetation if λ> 720 nm |
Additionally, when cos β is smaller than cos β T , no correction is needed and when (cos β/cos β T ) b is smaller than 0.25 (the solar zenith angle is much higher than the threshold) the value of ρ H is set to 0.25 to prevent a high correction factor.
In this study, the above-mentioned methods have been applied to the ALOS AVNIR data acquired over the study area (a rugged forest area).
3.2. Accuracy assessment methods
Several authors have offered suggestions for accuracy assessments of topographic correction methods (Civco Citation1989, Colby Citation1991, Conese et al. Citation1993, Lu et al. Citation2008, Richter Citation2009). Ideally, changes in the spectral characteristics of processed images should be low after correction; otherwise they indicate over- or under-correction (Civco Citation1989).
Therefore, the spectral homogeneity should increase after performing the correction, and it could then be used as the first criteria for the evaluation of correction methods.
The second approach is to conduct a graphical analysis between ρ H and ρ T versus the local illumination angle (β). ρ T changes with β, but after correction ρ H should remain constant versus the β changes (Meyer et al. Citation1993, Ekstrand Citation1996).
Third, the standard deviation (SD) of each class should decrease after applying a correction, and the homogeneity of each surface coverage class should increase (Civco Citation1989, Richter Citation2009).
Finally, the classification accuracy can be a criterion to assess the accuracy of topographic correction methods (Conese et al. Citation1993, Riano et al. Citation2003, Richter Citation2009, Richter et al. Citation2009). In this study, (1) the spectral coherency, (2) the classification accuracy, and (3) the class homogeneity were calculated before and after applying correction methods to evaluate the results (Riano et al. Citation2003).
In the first step, all methods mentioned were applied on the selected image, then the results were visually compared to eliminate methods that caused overcorrections leading to the worst image quality.
In the second step, the selected correction methods were applied to the image's bands, and the changes in the SD and mean of each band after correction were calculated. The best method should maintain the mean of the original bands while decreasing the SD (Civco Citation1989, Riano et al. Citation2003).
The second accuracy assessment criterion is class homogeneity. After performing topographic correction methods, class homogeneity should increase in the classification process (Conese et al. Citation1993, Riano et al. Citation2003, Richter et al. Citation2009). First, the image over the study area was classified into five main classes based on the vegetation map which includes dominant species in the vegetation of this area. The training samples were selected using a vegetation map of the study area.
A description of the vegetation classes used to analyze the result of the topographic correction methods is presented in .
Table 2. The changes in mean and SD of each image band after applying correction methods. The negative values indicate increasing in SD or mean after correction.
This performance was realized in two steps. In the first step, different supervised classification algorithms, including parallel piped, maximum likelihood, Spectral Angle Mapper (Kruse et al. Citation1993), and neural network, were tested.
The classification accuracy was assessed using samples defined as regions of interest based on the vegetation map of the study area.
Assessment of the accuracy of topographic correction methods is also possible by reducing the SD of reflectance of a surface class. The coefficient of variation (CV) was used to assess the accuracy of each topographic correction method (Richter et al. Citation2009). CV is defined as
where σ represents the SD and mean represents the mean of the reflectance value of each class.
The topographic correction increases spectral homogeneity within each surface coverage class so that the CV decreases after applying the corrections (Civco Citation1989, Bishop et al. Citation2003, Riano et al. Citation2003, Richter et al. Citation2009). The CV metric was calculated for each class before and after applying the correction.
4. Results and discussion
The results of applying the different topographic correction methods are depicted in .
According to , the worst image quality was obtained after applying the Cosine, SCS, and modified Minnaert correction methods. The Cosine, SCS, and traditional Minnaert correction methods were eliminated from further analysis because of the overcorrection on the image after performing these methods. Therefore, in the further analysis, only the C correction, SCS+C, pixel-based Minnaert, and modified Minnaert methods were taken into account.
4.1. Spectral coherency
The results of calculating the mean and SD of each band are summarized in .
Table 3. Description of each vegetation class in the study area.
From , it can be concluded that the SCS+C correction and pixel-based Minnaert produced the lowest changes in the mean of the original bands while the modified Minnaert method yields the maximum changes. The pixel-based Minnaert method and the modified Minnaert method caused a reduction and an increase of the SD, respectively (note that the SD and mean values before the correction minus these values after correction have been calculated).
Based on these results, the pixel-based Minnaert method was chosen as the best approach, and the modified Minnaert method was selected as the worst in terms of using spectral coherency as a criterion for accuracy assessment. The results of the C correction are in between. The C correction method performed better and worse than the modified Minnaert and pixel-based Minnaert methods, respectively.
4.2. Classification accuracy
The accuracy of the performed classification algorithms is presented in .
Table 4. The Kappa coefficient classification accuracy before and after topographic correction.
As shown in , the best result was achieved using the neural network algorithm. Therefore, the neural network algorithm was selected to classify the image. Dozens of network architectures were tested to obtain the best network design.
Finally, a network including one input layer, one hidden layer, and one output layer was selected. The input layer used a linear function, while the hidden layer had 10 neurons and used a sigmoid transfer function for each neuron. Furthermore, the training was realized by setting the learning rate as 0.75 and the momentum as 0.45.
Comparing the total accuracy and the Kappa coefficient of the image classification revealed that the best accuracy was reached after performing the pixel-based Minnaert method and the least accurate result was obtained from the Modified Minnaert method ().
Table 5. The Kappa coefficient classification accuracy before and after topographic correction using neural network algorithm.
4.3. Class homogeneity
The changes of the CV metric calculated before and after applying the corrections are summarized in .
Table 6. The coefficient of variation (CV) changes after applying correction respective to uncorrected image. Negative values indicate classes where CV increased after applying correction. The most changes are bold.
According to , in classes 1 and 5, which have little topographic distortion, the SCS+C and C corrections produced better results than the pixel-based Minnaert.
In class 1, in which the topographic effects are slightly smaller than class 5, the C correction method had the best result; in class 5, however, the SCS+C method performed better. In classes 2, 3, and 4, which have extreme topographic impacts, the pixel-based Minnaert yielded the best results. The SCS+C correction method produced the second-rank performance.
According to these results observation, we concluded that the C correction would be suitable for areas with slight topographic effects, while the modified Minnaert presented less accurate results.
4.4. Discussion
An evaluation of the topographic correction result is often required to understand the performance of the methods used for reducing topographic effects (Lu et al. Citation2008). Therefore, the selected methods have been evaluated based on three different criteria (spectral coherency, classification accuracy, and class homogeneity).
All tested methods assume that the spectral homogeneity should increase after performing corrections, which means there is one spectral class in the image (Riano et al. Citation2003). However, the different vegetation types are mixed in many regions.
Some authors claim it is impossible to perform topographic correction without knowing the vegetation types in advance, because the topographic effect is vegetation-dependent (Leprieur et al. Citation1988, Thomson and Jones Citation1990).
In our case (and for similar areas), the spectral differences between the vegetation types are not considerable, so the increasing or decreasing spectral homogeneity of all the vegetated areas on the image after applying the topographic correction is similar (Shataee Joibary and Darvishsefat Citation2004, Shataee Joibary et al. Citation2007, Mohammadi et al. Citation2010). If performing an accurate classification were possible before applying the topographic correction, the increasing homogeneity within each vegetation class should be considered as an accuracy assessment criterion.
Most of the previous research used a comparison of classification accuracies before and after correction to evaluate the topographic correction methods. Some research has indicated that topographic correction could improve classification accuracy (Civco Citation1989, Meyer et al. Citation1993, Tokola et al. Citation2001).
However, other studies have not shown the capability of improving the classification accuracy (Belsius and Weirich Citation2005). In fact, the classification accuracy depends on many factors so the classification accuracy may not be a good indicator for evaluating topographic correction methods in some regions (Bishop and Colby Citation2002).
In this study, the classification accuracy was improved after performing topographic correction; however, this improvement was not considerable because of the complex vegetation mixture in the study area. It is critical to concentrate on improving classification methods and identifying suitable images for the stratification of different vegetation types in this area and in similar regions.
The spectral coherency and interclass homogeneity increased after performing the topographic correction, as previous studies have concluded (Riano et al. Citation2003, Lu et al. Citation2008, Richter et al. Citation2009). A summary of all assessments is presented in .
Table 7. The summary of all evaluation criteria.
Based on the presented results, the C correction method produced a more accurate corrected image than the SCS approach due to the application of a correction factor (C k ) to consider the impacts of the diffuse component of light (Riano et al. Citation2003, Richter et al. Citation2009). Thus, this factor is only effective in flat areas or areas with slight topography. After applying C k to the SCS correction to account for residual effects and to prevent overcorrection (SCS+C method), better results were yielded than the other physically based methods (Riano et al. Citation2003).
Semi-empirical models such as Minnaert normalization have shown good results in previous studies (Justice et al. Citation1981, Sandmeier and Itten Citation1997, Bishop and Colby Citation2002, Richter et al. Citation2009), but it has been proven that using a global correction factor for all areas covered by an image is not acceptable and leads to over- or under-correction (Lu et al. Citation2008). Some modifications have been made to the Minnaert approach to overcome this problem (Richter Citation2009, Richter et al. Citation2009).
Improvement of the results of Minnaert methods in many areas by adding empirical correction factors has been reported in some studies (Richter Citation2009); however, it did not yield satisfying results in our study area.
Using specific k values for various areas with different topographical conditions on an image can solve the problem of the Minnaert method (Lu et al. Citation2008). As can be seen in , the pixel-based Minnaert method achieved the best results in our study area.
Simple correction methods, such as the Cosine method, lead to overcorrection in low illumination areas, especially in areas with dense vegetation cover. Physically based approaches, such as SCS and C correction, which account for vegetation structure, are more suitable for forested areas (Riano et al. Citation2003, Soenen et al. Citation2005).
However, these methods also cause overcorrection in areas with severe topography. Physically based approaches (C, SCS+C) are recommended for vegetated areas, but in areas with extreme topographical changes, the pixel-based Minnaert method yields the best results. In line with these facts, our findings improved the suitability of the pixel-based Minnaert method in rugged forests, as Lu et al. (Citation2008) previously showed the capability of this method in mountainous areas without vegetation cover.
5. Conclusion
Image interpretation and the extraction of useful information are vital in many fields of digital Earth applications. Therefore, the pre-processing step and removing the effects of topography are important in the accuracy of extracted information. The accuracy of frequently used topographic correction methods has been tested through different criteria over a forest area using a mid-summer ALOS AVNIR-2 image.
Based on the accuracy assessment results, the best accuracy was achieved by the pixel-based Minnaert method. For the vegetation classes with low topographic effects, the SCS+C and C correction methods were more accurate than the pixel-based Minnaert method in increasing interclass homogeneity.
However, further investigation is needed to determine whether the pixel-based Minnaert correction method is the most suitable model in all seasonal and topographic conditions as there is still no superior correction model. Also, further research should be done to test the different methods with other images of the same study area under worse illumination conditions (lower Sun elevation angle).
It is also recommended that the effect of topographic correction methods on increasing classification accuracy be examined, especially in areas with mixed species and complex structure.
Notes on contributors
Nafiseh Ghasemi received the B.Sc. degree in Geodesy & Geomatics Engineering from K.N. Toosi University of Technology, Tehran, Iran, in 2009. She also received the M.Sc. degree with a concentration on biomass mapping using optical and SAR images from the K.N. Toosi University of Technology in 2011. Her research interests are in SAR data processing algorithms, polarimetry and interferometry techniques with application in forestry and environmental modeling.
Dr. Ali Mohammadzadeh received the B.Sc. and M.Sc. degrees in Geodesy & Geomatics Engineering from K.N. Toosi University of Technology, Tehran, Iran, in 2000 and 2002, respectively. He received his Ph.D. in Remote Sensing in 2009. His areas of research interest include remote sensing image analysis, artificial intelligence, lidar image analysis, and feature extraction. He has over 30 published conference papers and 12 published journal papers. Since January 2010, he is assistant professor of K.N. Toosi University of Techology, Tehran, Iran and responsible for the university courses entitled ‘Management of Remote Sensing Data’ and ‘Artificial Intelligence’ taught at respective University. http://wp.kntu.ac.ir/a_mohammadzadeh/
Dr. Mahmod Reza Sahebi received the B.Sc. degree in Civil Engineering from Amirkabir University of Technology, Tehran, Iran, in 1991. He entered the graduate program at the Université de Sherbrooke, Sherbrooke, Canada in 1994 and received the M.Sc. A degree in Civil Engineering in 1996 and the Ph.D. degree in remote sensing in 2003. From 2003 to 2006 he worked as post-doctoral fellow in Canadian space agency. He is presently an Assistance professor and head of remote sensing research center in the Geodesy & Geomatics Engineering of the K.N. Toosi University of Technology, Tehran, Iran. His research interests include Applications of SAR data (polarimetry and interferometry), hyperspectral image analysis, and remote sensing in land-use and land-cover change analysis.
Acknowledgements
The authors would like to thank the ESA (European Space Agency) for providing ALOS AVNIR-2 data (based on the Category 2 program) as well as the Iran Forest and Watershed Management Organization for providing digital elevation data.
References
- Belsius , L. and Weirich , F. , 2005 . The use of the Minnaert correction for land-cover classification in mountainous terrain . International Journal of Remote Sensing , 26 , 3831 – 3851 .
- Bishop , M.P. and Colby , J.D. 2002 . Anisotropic reflectance correction of SPOT-3 HRV imagery . International Journal of Remote Sensing , 23 : 2125 – 2131 .
- Bishop , M.P. , Shroder , J.F. Jr and Colby , J.D. 2003 . Remote sensing and geomorphometry for studying relief production in high mountains . Geomorphology , 55 : 345 – 361 .
- Chavez , P.S. , 1988 . An improved dark-object subtraction technique for atmospheric scattering correction of multispectral data . Remote Sensing of Environment , 24 , 459 – 479 .
- Chavez , P.S. , 1996 . Image-based atmospheric corrections – revisited and improved . Photogrammetric Engineering and Remote Sensing , 62 , 1025 – 1036 .
- Civco , D. , 1989 . Topographic normalization of Landsat Thematic Mapper digital imagery . Photogrammetric Engineering and Remote Sensing , 55 , 1303 – 1309 .
- Colby , J.D. , 1991 . Topographic normalization in rugged terrain . Photogrammetric Engineering and Remote Sensing , 57 , 531 – 537 .
- Conese , C. et al. , 1993 . Topographic normalization of TM scenes through the use of an atmospheric correction method and digital terrain models . Photogrammetric Engineering and Remote Sensing , 59 , 1745 – 1753.
- Dymond , J.R. and Shepard , J.D. , 1999 . Comment on ‘Topographic normalization of Landsat TM images of forest based on subpixel sun canopy-sensor geometry’ by Gu and Gillespie . Remote Sensing of Environment , 194 , 166 – 175 .
- Ekstrand , S. , 1996 . Landsat-TM based forest damage assessment: correction for topographic effects . Photogrammetric Engineering and Remote Sensing , 62 , 151 – 161 .
- Fadhil , A.M. 2010 . Drought mapping using Geoinformation technology for some sites in the Iraqi Kurdistan region . International Journal of Digital Earth , 4 : 239 – 257 .
- Gu , D. and Gillespie , A. , 1998 . Topographic normalization of Landsat TM images of forest based on subpixel Sun-Canopy-sensor geometry . Remote Sensing of Environment , 64 , 166 – 175 .
- Gu , D. and Gillespie , A. 1999 . Response to Dymond and Shepard's comment on ‘Topographic normalization of Landsat TM images of forest based on subpixel Sun-Canopy-sensor geometry . Remote Sensing of Environment , 69 , 195 – 196 .
- Itten , K.I. and Meyer , P. , 1992 . Geometric and radiometric correction of TM data of mountainous forested areas . IEEE Transactions on Geoscience and Remote Sensing , 16 , 764 – 770 .
- Jansa , J. , 1998 . A global topographic normalization algorithm for satellite images . In : Proceedings of the ‘ISPRS Commission VII Symposium on Resource and Environmental Monitoring’ , 1–4 September , Budapest , , Hungary. Hamburg : CENSIS .
- Justice , C. , Wharton , S. , and Holben , B. , 1981 . Application of digital terrain data to quantify and reduce the topographic effect on Landsat data . International Journal of Remote Sensing , 2 , 213 – 230 .
- Kruse , F.A. 1993 . The spectral image processing system (SIPS) – interactive visualization and analysis of imaging spectrometer data . Remote Sensing of Environment , 44 : 145 – 163 .
- Leprieur , C. , Durand , J.M. and Peyron , J.L. 1988 . Influence of topography on forest reflectance using Landsat Thematic Mapper and digital terrain data . Photogrammetric Engineering and Remote Sensing , 54 : 491 – 496 .
- Lu , D. 2008 . Pixel-based Minnaert correction method for reducing topographic effects on a Landsat 7 ETM+ image . Photogrammetric Engineering and Remote Sensing , 74 : 1343 – 1350 .
- Meyer , P. , et al. , 1993 . Radiometric corrections of topographically induced effects on Landsat TM data in an alpine environment . ISPRS Journal of Photogrammetry and Remote Sensing , 48 , 17 – 28 .
- Mohammadi , J. , et al. , 2010 . Modelling forest stand volume and tree density using Landsat ETM+ data . International Journal of Remote Sensing , 31 , 2959 – 2975 .
- Panagos , P. 2011 . Monthly soil erosion monitoring based on remotely sensed biophysical parameters: a case study in Strymonas river basin towards a functional pan-European service . International Journal of Digital Earth , 2 : 196 – 220 .
- Riano , D. , et al. , 2003 . Assessment of different topographic corrections in Landsat TM data for mapping vegetation types . IEEE Transactions on Geoscience and Remote Sensing , 41 , 1056 – 1061 .
- Richter , R. , 1998 . Correction of satellite imagery over mountainous terrain . Applied Optics , 37 , 4004 – 4015 .
- Richter , R. , 2009 . Report DLR-IB 565-01/09 [online]. Available from Atmospheric/Topographic Correction for Satellite Imagery: ftp://ftp.dfd.dlr.de/put/richter/ATCOR/ [Accessed 17 September 2010] .
- Richter , R. , Kellenberger , T. , and Kaufmann , H. , 2009 . Comparison of topographic correction methods . Remote Sensing , 1 , 184 – 196 .
- Sandmeier , S. and Itten , K. , 1997 . A physically-based model to correct atmospheric and illumination effects in optical satellite data of rugged terrain . IEEE Transactions on Geoscience and Remote Sensing , 35 , 708 – 717 .
- Shataee Joibary , S. , and Darvishsefat , A.A. , 2004 . Forest type classification improvement using spatial predictive distribution models . Proceedings of the “21st International Symposium on Remote Sensing of Environment” , 25–29 April . St Petersburg , , Russia. Michigan : Erim Intl Inc .
- Shataee Joibary , S. , Darvishsefat , A.A. , and Kellenberger , T.W. , 2007 . Forest type mapping using incorporation of spatial models and ETM+ data . Pakistan Journal of Biological Sciences , 10 , 2292 – 2299 .
- Shepherd , J. and Dymond , J. , 2003 . Correcting satellite imagery for the variance of reflectance and illumination with topography . International Journal of Remote Sensing , 24 , 3503 – 3514 .
- Soenen , S.A. , Peddle , D.R. , and Coburn , C.A. , 2005 . SCS+C: a modified Sun-Canopy-sensor topographic correction in forested terrain . IEEE Transactions on Geoscience and Remote Sensing , 43 , 2148 – 2159 .
- Teillet , P. , Guindon , B. , and Goodenough , D. , 1982 . On the slope-aspect correction of multispectral scanner data . Canadian Journal of Remote Sensing , 8 , 84 – 106 .
- Thomson , A.G. and Jones , C. 1990 . Effects of topography on radiance from upland vegetation in North Wales . International Journal of Remote Sensing , 11 : 829 – 840 .
- Tokola , T. , Sarkeala , J. , and van der Linden , M. , 2001 . Use of topographic correction in Landsat TM-based forest interpretation in Nepal . International Journal of Remote Sensing , 22 , 551 – 563 .
- Wylie , B. et al. , 2008 . Integrating modelling and remote sensing to identify ecosystem performance anomalies in the boreal forest, Yukon River Basin, Alaska . International Journal of Digital Earth , 1 , 1 – 27 .