Abstract
The aim of this study was to develop a straightforward approach for flood area mapping in a transboundary riverbed using Geographic Object-Based Image Analysis. Weak bilateral/multilateral cooperation among neighboring countries hampers effective disaster management and mitigation activities over transboundary areas and strengthens the demand for reliable remote-sensing-derived information. Three object-based classification approaches using ENVISAT/ASAR and multi-temporal LANDSAT TM data were developed and validated for flood area delineation. The accuracy assessment of the classification results was based on oblique air photo interpretation and an area-based comparison with the official flood map. The bi-level object-based model using the Normalized Difference Water Index and the original post-flood TM bands attained 92.67% overall accuracy in inundated-areas detection, while the ENVISAT/ASAR classification was the least accurate (85.33%), probably due to the lower spatial resolution of the Synthetic Aperture Radar image. A strong agreement (92.14%) was found between the LANDSAT flood extent and the official flood map, suggesting that the proposed method has the potential to be employed in the future as a standard part of a flood crisis management process.
1. Introduction
Natural disasters such as floods, wildfires, earthquakes, tsunamis, and volcanic eruptions usually result in significant human losses and environmental and economical degradation. Among the many natural hazards of the world, floods are probably the most devastating, widespread, and frequent (Sanyal and Lu Citation2004). During the last few decades, floods in Europe have become a growing topic of concern for citizens, authorities, insurance companies, and policy-makers, given that during the second half of the twentieth century, the number of floods in European river basins rose from 11 to 64 per decade (Van Alphen et al. Citation2009).
Floods generated from heavy or continuous rainfall exceeding the absorptive capacity of soil, and the flow capacity of rivers or streams cause a watercourse to overflow its bank onto adjacent lands (Amini Citation2010), resulting in human losses, crop and infrastructure damage, and siltation of reservoirs (Sanyal and Lu Citation2004).
To minimize the consequences of these catastrophic events, disaster managers and national authorities are in need of accurate information regarding the geographic extent of the areas at risk to apply comprehensive and innovative risk-management measures (Gitas et al. Citation2008, Mayer et al. Citation2008). Digital earth technologies can play a very important role in the provision of geo-information that could reduce risks and vulnerability, mitigate the effects of natural disasters, and improve rescue operations (Guo Citation2010). Remote sensing, which is increasingly being recognized as a powerful tool to support decision-making in disaster management and mitigation (Gillespie et al. Citation2007, Guo Citation2010), along with GIS that allows input, management, processing, spatial analysis, cartographic modeling, and visualization of complex and multi-faceted physical phenomena (Kalabokidis et al. Citation2007), can be applied in all four phases of the flood management process, namely in pre-flood planning, flood emergency management, post-flood recovery, and disaster mitigation (Goodchild and Glennon Citation2010, Seppänen and Virrantaus Citation2010).
Accurate flood mapping is an essential task for flood-hazard and flood-damage assessment (Amini Citation2010). Aerial photography has been efficiently used in the past for determining limits of the flooded zones as well as water-depth assessment, over small- and mid-sized flooded areas (Puech and Raclot Citation2002). However, there are certain advantages to using satellite remote sensing in flood mapping, such as (Gianinetto et al. Citation2006):
easy availability of the data (especially for the optical data where large archives are often available),
lower cost and extended coverage area, and
robustness and efficiency of the data-processing techniques.
Medium/low spatial resolution optical data with short revisiting time has been used to provide timely and frequently updated situation reports that are required particularly by the local authorities during the emergency phase to locate and identify the affected areas and to consequently organize rescue and damage-mitigation actions (Gitas et al. Citation2008, Lacava et al. Citation2010). Information from medium/high spatial and spectral resolution optical imagery has been employed for flood risk mapping as well as for the production of accurate post-flood maps of the inundated areas. However, the use of optical satellite imagery during or immediately after a flood event is often limited by the presence of clouds (Gianinetto et al. Citation2006). Remote sensing sensors such as the Synthetic Aperture Radar (SAR) have the capability to penetrate clouds and as a result can provide views of the extent of inundation, devoid of cloud cover (Kiage et al. Citation2005, Henry et al. Citation2006, Evans et al. Citation2010). In addition, the dielectric constant of free water (about 70 at C-band) is very high compared to dry soil or vegetation, providing SAR sensors the capability to detect small changes in the water content of soil or plant targets (Brisco et al. Citation2008).
In recent years, a shift from the adoption of the pixel as a unit of classification to Geographic Object-Based Image Analysis (GEOBIA) methods has been observed due to recognition of limitations in the use of pixel-based image approaches, existence of the high-resolution scene model for most applications, environmental monitoring requirements, ecosystem-oriented natural resources management, and software and hardware developments, among other drivers (Hay and Castilla Citation2008, Blaschke Citation2010). GEOBIA, which has been defined by Hay and Castilla (Citation2008) as ‘a discipline that will primarily develop theory, methods and tools that will replicate and/or exceed experienced human interpretation of RS images, resulting in more accurate and repeatable information, less subjectivity and reduced labour and time costs,’ could evolve to be a paradigm that fosters and promotes the visions of Digital Earth. The geo-intelligence that could be generated through GEOBIA (Hay and Blaschke Citation2010) could be used in many of the applications fields of Digital Earth such as: climate change, natural disaster prevention and response, new energy-source development, agricultural and food security, and urban planning and management (Guo et al. Citation2010).
Geographic Object-Based Image Analysis is also attractive for SAR imagery classification where the radiometric signal of each target must be approximated by averaging backscattering across a neighborhood of pixels to decrease the effects of speckle (Evans et al. Citation2010).
The aim of this study was to develop a straightforward and reliable approach for flood mapping and damage evaluation in a transboundary river plain with restricted access by using GEOBIA and medium spatial resolution optical and SAR data.
The objectives of the study included the development and evaluation of three object-based classification approaches, using:
an ENVISAT ASAR image acquired during the peak of a major flood event in the Evros river along the Greek-Turkish borders in winter 2010,
multi-temporal LANDSAT TM images and multi-date post-classification comparisons, and
a single spectral index – differential Normalized Difference Water Index (dNDWI).
2. Study area
The Evros river – Maritsa in Bulgarian and Meriç in Turkish – is the second largest river in Eastern Europe after the Danube. Its total length is 515 km, while the drainage basin covers an area of 52,900 km2, divided between Bulgaria (34,900 km2, i.e. 66%), Turkey (14,550 km2, i.e. 27.5%) and Greece (3450 km2, i.e. 6.5%). Parts of the Evros riverbed serve as the state border between Greece and Turkey ().
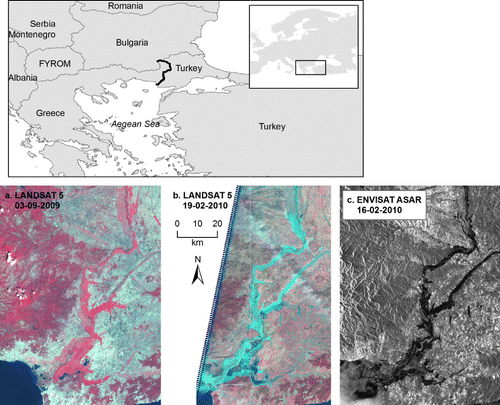
There are currently 18 major dams and a number of smaller dams in operation in Bulgaria; 8 dams and 59 reservoirs, for irrigation purposes, in Turkey; and 1 irrigation dam in Greece (Kyprinos), irrigating 25,000 ha of arable land (Kanellopoulos et al. Citation2008). After the construction of embankments on the Greek and the Turkish side of the Evros river in the last two decades, floods are observed either by overflow or by fracture of the embankments, when the discharge of the river exceeds 2500 m3/s. It is not clear yet if these flood phenomenona are due to extreme climatic changes or a result of the management of the Bulgarian dams after 1994. Despite the magnitude and the frequency of the flood disasters, accurate flood maps do not exist yet due to the low level of transboundary cooperation (Angelidis et al. Citation2009). On 11 February 2010, the water of the Evros river reached flood alert level as a result of snowmelt in the Bulgarian part of the drainage basin. The water level continued to rise reaching its maximum height on 15 February.
3. Materials and methods
3.1. Outline of the methodology
Information extraction from the ENVISAT/ASAR and LANDSAT TM imagery relied on the use of the GEOBIA concept (Hay and Castilla Citation2008). First, the perennial riverbed was delineated using the Normalized Difference Water Index (NDWI) calculated from a summer LANDSAT TM image. To assess the extent of the flood damage using optical data we evaluated two alternative options, considering:
a univariate image differencing approach, and more specifically the use of a single differential spectral index (dNDWI) and
the use of the post-NDWI spectral index along with bands of the original post-flood TM image followed by post-classification comparisons with the perennial riverbed extracted from the pre-flood TM image.
3.2. Remote sensing data and pre-processing
3.2.1. SAR imagery
The Advanced Synthetic Aperture Radar (ASAR) onboard ENVISAT, operating at C-band (5.3 GHz), is an advanced version of SAR instruments onboard the ERS-1 and ERS-2 satellites, featuring enhanced capability in terms of coverage, range of incidence angles, polarization, and modes of operation (European Space Agency [ESA], Citation2007). One of the key new features of the ASAR processor is its ability to generate medium spatial resolution (150 m) but high radiometric resolution products for the Single (IM) and Alternating (AP) Image polarization modes, in long strips (100 km) without geometric or radiometric discontinuity. Such an Image Mode Medium Image-resolution image with VV polarization acquired 16 February 2010 was obtained a day later by the ESA following a simple fast registration process available through ESA's Earthnet Online.
3.2.2. Optical imagery and pre-processing
Two LANDSAT-5 TM images (path/row 182/031 and 182/032) acquired in early September 2009 were used to detect perennial water bodies and riverbed prior to the inundation, while another set of LANDSAT TM images acquired on 19 February 2010 (path/row 181/031 and 181/032) was used for post-flood damage evaluation.
The Next ESA SAR Toolbox (NEST) and the ERDAS IMAGINE were used for the geometric correction and the co-registration of the ASAR images to the TM data-sets using a 30-meter resolution DEM and GCPs identified on large-scale orthophotographs. Image mosaics were subsequently produced for each acquisition date.
An absolute atmospheric correction procedure based on the dark object subtraction approach was applied to correct the effects caused by the solar zenith angle, solar radiance, and atmospheric scattering. One advantage of this image-based approach is that it is relatively simple to apply and does not require any in situ measurements and atmospheric parameters (Chavez Citation1996). First, recorded DN values were converted to radiance at sensor L (i) for each band of the Landsat TM image:
Second, radiance at sensor was converted to surface reflectance by applying the following equation:
The surface reflectance was used to calculate a spectral water index – an arithmetic operation that not only enhances the spectral signals by contrasting the reflectance between different wavelengths but also cancels out a large portion of the noise components such as sensor calibration and changing radiation conditions caused by illumination, soil, topography, and atmospheric conditions that are common in different wavelength regions (Ji et al. Citation2009). The NDWI was estimated using the following formula (Gao Citation1996):
Furthermore, a differential spectral index has been calculated by the subtraction of the pre-flood from the post-flood NDWI values:
3.3. Image analysis
The object-based image analysis approach adopted in our study included the generation of single-level and multi-level object hierarchies through image segmentation, both for SAR and LANDSAT TM imagery followed by fuzzy rule-based classification.
We used a multi-resolution bottom-up segmentation algorithm, where individual image pixels are perceived as the initial regions and are sequentially merged pairwise into larger ones with the intent of minimizing the heterogeneity of the resulting objects. Within this approach, the sequence of the merging objects, as well as the size and shape of the resulting objects are empirically determined by the user (Mallinis et al. Citation2008a, Gao et al. Citation2009).
For the fuzzy rule-based classification of the segments, samples were selected and we visually determined fuzzy thresholds in the case of the dNDWI and SAR approaches. In the case of the post-flood LANDSAT/NWDI approach, where more variables (bands) were available, a feature selection process was adopted based on the use of Classification and Regression Trees (Breiman Citation1984) which have been shown to couple well with GEOBIA (Chubey et al. Citation2006, Mallinis et al. Citation2008a, Citation2008b).
In the classification process, we also explored the use of variables related to the anaglyph such as the digital elevation model, the slope, the aspect, and the cosine of the solar incidence angle computed by the following formula (Mallinis et al. Citation2004):
3.4. Post-classification analysis
To determine multi-temporal changes in land-cover/use types, a multi-date post-classification comparison is perhaps the most common approach to change detection (Jensen Citation2000). Therefore, areas inundated were identified based on the subtraction of the post-flood SAR and LANDSAT/NWDI-derived maps and the perennial water areas identified on the pre-flood LANDSAT imagery.
3.5. Accuracy assessment
A number of ground truth points (GPS measurements) were gathered during the flood event along the road network, going parallel to the border line and the riverbed. The ground observations were coupled with oblique digital aerial photographs acquired during the flood event from the local branch of the Greek Civil Protection Agency, along with the official flood extent map, and were used to verify the accuracy of the flooded/not flooded areas on the derived inundation maps.
4. Results and discussion
4.1. Segmentation and classification
For the identification of the perennial river course and water bodies we introduced a fuzzy rule based on NDWI values of the pre-flood LANDSAT TM image. Following a classification-based fusion procedure (merging of adjacent water segments), we further introduced a fuzzy rule based on DEM values for eliminating erroneously classified segments as water objects on mountainous areas covered with cloud shadows. A post-classification refinement based on manual editing corrected minor misclassifications.
As for the dNDWI approach, fine scale segments were generated solely upon the information content of the differential spectral index. Originally, the use of a fuzzy-classification rule based on dNDWI values produced an overestimation of the flooded areas, especially on north-facing, abrupt relief areas, as a result of the low sun elevation and the satellite's position during the post-flood acquisition. Therefore, we introduced the cosine of the solar incidence angle as an indicator of the topographic context to minimize erroneously identified flooded areas over shaded areas.
In the case of the LANDSAT/NWDI approach, a detailed segmentation level was generated based on information from the post-NDWI image. The aim of this level was to delineate small segments, uniformly covered with water. In addition, a second upper level was generated by information from the near-infrared and mid-infrared bands of the post-flood LANDSAT imagery. Segments of this level represented patches not totally submerged by water but with an inner pattern characterized by small, dispersed water spots. These small water segments, not always immediately adjacent to the main riverbed, corresponded to individual agricultural fields that due to their vicinity to the extensive irrigation network canals connected to the river, and the local anaglyph are the first to be inundated following a major flood and among the last areas remain covered by water after the event. The rationale behind the delineation of these ‘semi-flooded’ areas in our classification is two-fold: First, as we observed from the official flood extent during the training phase, disaster managers consider these areas as flooded likely because of the risk associated in accessing these partially flooded areas during the crisis. Second, as we mentioned earlier identification of such areas could be used for flood delineation using imagery acquired after several days of the flood event. In the latter case, these partially flooded areas would be characterized by scattered water spots and high soil/vegetation moisture.
Finally, for the classification of the segments in the lower detailed level, NDWI and TM7 were identified as the most appropriate bands for the well inundated areas, and TM5 and TM7 were selected for the classification of areas appearing to be covered by shallower waters. Frazier and Page (Citation2000) have also noted that in areas with turbid water, an increase in the upper range of values in the VIR part of the spectrum is more evident than in the mid-infrared bands. In the second/upper level, two flood classes were discriminated: the first one accounted for objects totally covered by water and the second one with partially flooded objects, containing small sub-objects classified as water. The former class was classified upon hierarchical information from the classified sub-level (existence of sub objects classified as water) while the objects with dispersed water segments within them, were identified by the number and the relative area of water sub-objects and TM5 and TM3 bands.
For the ENVISAT ASAR image a single level of segments was generated, followed by a classification procedure based on fuzzy thresholding of backscattering signal.
4.2. Accuracy assessment results
The post-NDWI/LANDSAT approach considering only the lower level of the model (uni-level) achieved the highest overall accuracy (92.67%) among the three approaches, while the ENVISAT/ASAR classification was less accurate (85.33%) than the dNDWI approach (91.33%). In addition, the post-NDWI/LANDSAT approach was most successful in terms of presenting the most balanced omission/commission errors.
Area-based comparisons of the official flood extent map with the four classification maps confirmed the findings of the accuracy assessment procedure. The ENVISAT/ASAR and the dNDWI approach underestimated significantly (169.44 and 121.71 km2, respectively) the flooded area as was expected from the low producer's accuracy observed in these classification approaches (large commission errors) in .
Table 1. Confusion matrices and accuracy measures of the three classification approaches.
However, remote sensing estimates showing best agreement with the official flood extent map were obtained from the bi-level post-NDWI/LANDSAT approach, which successfully identified 92.14% of the flood extent recorded by the aerial survey. This approach was the only one producing larger commission than omission errors ( and ).
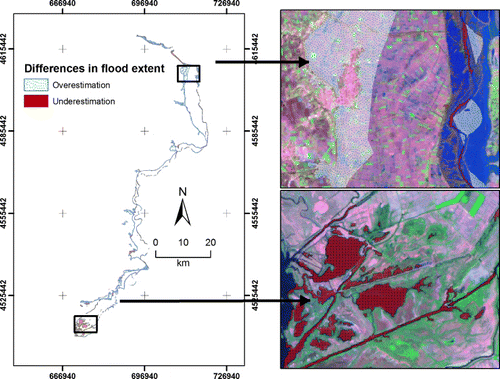
Table 2. Area-based comparison and agreement of the uni-level Geographic Object-Based Image Analysis (GEOBIA) post-NDWI/LANDSAT, dNDWI, ENVISAT and bi-level GEOBIA post-NDWI/LANDSAT classifications with the official flood map.
An underestimation (according to the official records) of the flooded area using the LANDSAT imagery is evident in the lower delta area of the Evros river covered with wetlands and subsaline lakes (). It is likely that the aerial sketch of the flood extent produced without the use of a multi-temporal image data-set mistakenly identifies these areas as flooded. Some discrepancies are also noted along the main riverbed probably due to geometric inaccuracies of the aerial sketch approach. A significant part of the areas wrongly identified as flooded from the GEOBIA approach, according to the officially reported inundation extent, are probably linked to the five-day difference between the aerial flight and LANDSAT images acquisition. As shown in adjacent areas between the erroneously classified objects and the main riverbed present the same pattern of scattered water spots and have been designated as flooded in the official reported map.
Both the accuracy in water areas detection and the agreement with official flood extent were higher in the case of the bi-temporal classification approach of the LANDSAT imagery compared to the SAR image classification. A possible reason could be the polarization mode of the radar image use. Henry et al. (Citation2006), in a comparison study between different polarization modes of the ASAR sensor using 12.5 meter spatial resolution images, concluded that the HH polarization provides a more suitable discrimination of the flooded areas than VV or HV. A further constraint in achieving a higher water detection rate is the coarse resolution of the radar image that prohibits the detection of scattered water-covered segments.
The accuracy achieved in water detection from both approaches using LANDSAT imagery was above 92%. The dNDWI approach was slightly less accurate than the post-NDWI/LANDSAT bands approach. An advantage of the differential spectral index approach lies in its simplicity and straightforward implementation as opposed to the most elaborative feature and threshold selection approach needed in the case of the post-NDWI/LANDSAT bands approach. Additionally, in the case of the post-classification comparisons apart from the misregistration errors, problems due to the misclassification of the pre-flood imagery might occur contrary to the univariate image differencing based on dNDWI (Coppin et al. Citation2004).
Yet, an advantage of the post-NDWI/LANDSAT bands classification is that information from other parts of the electromagnetic spectrum is available and can be introduced in the classification process for delineating more complex classification schemes. In our study this information eased the introduction of a second upper level for delineating segments partially covered by water.
5. Concluding remarks
In this work, processing of ENVISAT/ASAR and multi-temporal LANDSAT TM data within a GEOBIA framework for flood area delineation in a transboundary river system with restricted access produced satisfactory results. The post-NDWI/LANDSAT approach achieved the highest overall accuracy, while the ENVISAT/ASAR classification was the least accurate. Differences in the accuracies between the object-based classification approaches are primarily related to the source of information that is RS data, used. Potential and weaknesses associated with the use of SAR and optical remote sensing imagery in flood area delineation have been well demonstrated and discussed in several studies (Brivio et al. Citation2002, Wang et al. Citation2002, Sanyal and Lu Citation2004, Wang Citation2004, Jain et al. Citation2005). The GEOBIA approach used in our study provided satisfactory overall results for mapping the extent of the flood, especially in reference to the local authorities' official map. Strength of the GEOBIA approach in flood mapping, as in other earth system studies, is the ability to incorporate semantic knowledge in the classification process, thus restricting limitations resulting from imagery characteristics and temporal availability. As Hay and Castilla (Citation2008) note, a fundamental component of GEOBIA is its multi-scale contextual model, which attempts to emulate humans' interpretation of remote sensing images. Therefore, the information produced through the GEOBIA framework can be more easily integrated into social understanding and political decision-making, promoting, and implementing the Digital Earth vision.
Acknowledgements
The Landsat Data were available from the US Geological Survey. The ENVISAT/ASAR Data were provided by the European Space Agency.
References
- Amini, J., 2010. A method for generating floodplain maps using ikonos images and dems. International Journal of Remote Sensing, 31 (10), 2441–2456.
- Angelidis, P., Kotsikas, M., and Kotsovinos, N., 2009. Management of upstream dams and flood protection of the transboundary river Evros/Maritza. Water Resources Management, 24 (11), 1–18.
- Blaschke, T., 2010. Object based image analysis for remote sensing. ISPRS Journal of Photogrammetry and Remote Sensing, 65 (1), 2–16.
- Breiman, L., 1984. Classification and regression trees. Belmont, California: Wadsworth International Group.
- Brisco, B., et al., 2008. Water resource applications with RADARSAT-2 – a preview. International Journal of Digital Earth, 1 (1), 130–147.
- Brivio, P.A., et al., 2002. Integration of remote sensing data and GIS for accurate mapping of flooded areas. International Journal of Remote Sensing, 23 (3), 429–441.
- Chavez, P.S. Jr 1996. Image-based atmospheric corrections – Revisited and improved. Photogrammetric Engineering and Remote Sensing, 62 (9), 1025–1036.
- Chubey, M.S., Franklin, S.E., and Wulder, M.A., 2006. Object-based analysis of ikonos-2 imagery for extraction of forest inventory parameters. Photogrammetric Engineering and Remote Sensing, 72 (4), 383–394.
- Coppin, P., et al., 2004. Digital change detection methods in ecosystem monitoring: a review. International Journal of Remote Sensing, 25 (9), 1565–1596.
- ESA, 2007. ASAR Product Handbook, issue 2.2 [online]. ESA Earthnet Online. Available from: http://envisat.esa.int/handbooks/asar/ [Accessed 5 December 2011].
- Evans, T.L., et al., 2010. Using ALOS/PALSAR and RADARSAT-2 to Map Land Cover and seasonal inundation in the Brazilian Pantanal. Selected Topics in Applied Earth Observations and Remote Sensing, IEEE Journal of, 3 (4), 560–575.
- FLAPP, 2007. Flood awareness and prevention policy in border areas network. In: N. Morisson, ed. Final report. The Netherlands: INTERREG IIIC network FLAPP, p. 53.
- Frazier, P.S. and Page, K.J., 2000. Water body detection and delineation with Landsat TM data. Photogrammetric Engineering and Remote Sensing, 66 (12), 1461–1467.
- Gao, B.-c., 1996. NDWI–A normalized difference water index for remote sensing of vegetation liquid water from space. Remote Sensing of Environment, 58 (3), 257–266.
- Gao, Y., Mas, J.F., and Navarrete, A., 2009. The improvement of an object-oriented classification using multi-temporal MODIS EVI satellite data. International Journal of Digital Earth, 2 (3), 219–236.
- Gianinetto, M., Villa, P., and Lechi, G., 2006. Postflood damage evaluation using Landsat TM and ETM+ data integrated with DEM. IEEE Transactions on Geoscience and Remote Sensing, 44 (1), 236–243.
- Gillespie, T.W., et al., 2007. Assessment and prediction of natural hazards from satellite imagery. Progress in Physical Geography, 31 (5), 459–470.
- Gitas, I.Z., et al., 2008. Contribution of remote sensing to disaster management activities: a case study of the large fires in the Peloponnese, Greece. International Journal of Remote Sensing, 29 (6), 1847–1853.
- Goodchild, M.F. and Glennon, J.A., 2010. Crowdsourcing geographic information for disaster response: a research frontier. International Journal of Digital Earth, 3 (3), 231–241.
- Guo, H., 2010. Understanding global natural disasters and the role of earth observation. International Journal of Digital Earth, 3 (3), 221–230.
- Guo, H.D., Liu, Z., and Zhu, L.W., 2010. Digital Earth: decadal experiences and some thoughts. International Journal of Digital Earth, 3 (1), 31–46.
- Hay, G.J. and Blaschke, T., 2010. Special issue: geographic object-based image analysis (GEOBIA). Photogrammetric Engineering and Remote Sensing, 76 (2), 121–122.
- Hay, G.J. and Castilla, G., 2008. Geographic object-based image analysis (GEOBIA): a new name for a new discipline. In: T. Blaschke, S. Lang, and G.J. Hay, eds. Object based image analysis. Springer Berlin Heidelberg, 75–89.
- Henry, J.B., et al., 2006. Envisat multi-polarized ASAR data for flood mapping. International Journal of Remote Sensing, 27 (10), 1921–1929.
- Jain, S.K., et al., 2005. Delineation of flood-prone areas using remote sensing techniques. Water Resources Management, 19 (4), 333–347.
- Jensen, J.R., 2000. Remote sensing of the environment: an earth resource perspective. Upper Saddle River, N.J.: Prentice Hall.
- Ji, L., Zhang, L., and Wylie, B., 2009. Analysis of dynamic thresholds for the normalized difference water index. Photogrammetric Engineering and Remote Sensing, 75 (11), 1307–1317.
- Kalabokidis, K.D., et al., 2007. Multivariate analysis of landscape wildfire dynamics in a Mediterranean ecosystem of Greece. Area, 39 (3), 392–402.
- Kanellopoulos, T.D., et al., 2008. The influence of the Evros River on the recent sedimentation of the inner shelf of the NE Aegean Sea. Environmental Geology, 53 (7), 1455–1464.
- Kiage, L.M., et al., 2005. Applications of Radarsat-1 synthetic aperture radar imagery to assess hurricane-related flooding of coastal Louisiana. International Journal of Remote Sensing, 26 (24), 5359–5380.
- Lacava, T., et al., 2010. Improving flood monitoring by the Robust AVHRR technique (RAT) approach: the case of the April 2000 Hungary flood. International Journal of Remote Sensing, 31 (8), 2043–2062.
- Mallinis, G., et al., 2004. Forest parameters estimation in a European Mediterranean landscape using remotely sensed data. Forest Science, 50 (4), 450–460.
- Mallinis, G., et al., 2008a. Object-based classification using Quickbird imagery for delineating forest vegetation polygons in a Mediterranean test site. ISPRS Journal of Photogrammetry and Remote Sensing, 63 (2), 237–250.
- Mallinis, G., et al., 2008b. Local-scale fuel-type mapping and fire behavior prediction by employing high-resolution satellite imagery. Ieee Journal of Selected Topics in Applied Earth Observations and Remote Sensing, 1 (4), 230–239.
- Mayer, R., et al., 2008. MONITOR: hazard monitoring for risk assessment and risk communication. Georisk, 2 (4), 193–220.
- Puech, C. and Raclot, D., 2002. Using geographical information systems and aerial photographs to determine water levels during floods. Hydrological Processes, 16 (8), 1593–1602.
- Sanyal, J. and Lu, X.X., 2004. Application of remote sensing in flood management with special reference to monsoon Asia: a review. Natural Hazards, 33 (2), 283–301.
- Seppänen, H. and Virrantaus, K., 2010. The role of GI-supported methods in crisis management. International Journal of Digital Earth, 3 (4), 340–354.
- Van Alphen, J., et al., 2009. Flood risk mapping in Europe, experiences and best practices. Journal of Flood Risk Management, 2 (4), 285–292.
- Wang, Y., 2004. Using Landsat 7 TM data acquired days after a flood event to delineate the maximum flood extent on a coastal floodplain. International Journal of Remote Sensing, 25 (5), 959–974.
- Wang, Y., Colby, J.D., and Mulcahy, K.A., 2002. An efficient method for mapping flood extent in a coastal floodplain using Landsat TM and DEM data. International Journal of Remote Sensing, 23 (18), 3681–3696.