Abstract
Penman–Monteith (PM) theory has been successfully applied to calculate land surface evapotranspiration (ET) for regional and global scales. However, soil surface resistance, related to soil moisture, is always difficult to determine over a large region, especially in arid or semiarid areas. In this study, we developed an ET estimation algorithm by incorporating soil moisture control, a soil moisture index (SMI) derived from the surface temperature and vegetation index space. We denoted this ET algorithm as the PM-SMI. The PM-SMI algorithm was compared with several other algorithms that calculated soil evaporation using relative humidity, and validated with Bowen ratio measurements at seven sites in the Southern Great Plain (SGP) that were covered by grassland and cropland with low vegetation cover, as well as at three eddy covariance sites from AmeriFlux covered by forest with high vegetation cover. The results show that in comparison with the other methods examined, the PM-SMI algorithm significantly improved the daily ET estimates at SGP sites with a root mean square error (RMSE) of 0.91 mm/d, bias of 0.33 mm/d, and R2 of 0.77. For three forest sites, the PM-SMI ET estimates are closer to the ET measurements during the non-growing season when compared with the other three algorithms. At all the 10 validation sites, the PM-SMI algorithm performed the best. PM-SMI 8-day ET estimates were also compared with MODIS 8-day ET products (MOD16A2), and the latter showed negligible bias at SGP sites. In contrast, most of the PM-SMI 8-day ET estimates are around the 1:1 line.
Keywords:
1. Introduction
Evapotranspiration (ET), which includes soil evaporation and vegetation transpiration, is a major component of the global water cycle, and plays a central role in climate and meteorology, plant community dynamics, and carbon and nutrient biogeochemistry (Vörösmarty, Federer, and Schloss Citation1998). ET returns 60% of the precipitation to the atmosphere (Oki and Kanae Citation2006) and imposes an important constraint on the water supply. In addition, more than half of the absorbed solar energy is utilized for ET (Trenberth, Fasullo, and Kiehl Citation2009). Accordingly, accurate estimation of ET is extremely valuable for better irrigation management, especially in semiarid areas.
Remote sensing has been recognized as the most feasible means to provide a spatial distribution of land surface ET, as it can be used to estimate the land surface parameters required for estimation of ET, such as Normalized Difference Vegetation Index (NDVI), Leaf Area Index (LAI), albedo, and land surface temperature (Ts). Remote sensing-based methods can be divided into four types: (1) empirical statistical methods (Bella, Rebella, and Paruelo Citation2000; Carlson, Capehart, and Gillies Citation1995; Wang and Liang Citation2008); (2) residual methods based on the residual of surface energy balance through thermal infrared data (Anderson et al. Citation1997; Bastiaanssen et al. Citation1998; Norman, Kustas, and Humes Citation1995; Su Citation2002); (3) triangle/trapezoid methods linked with vegetation index and Ts (Jiang and Islam Citation1999; Jiang and Islam Citation2001; Moran et al. Citation1994; Stisen et al. Citation2008; Tang, Li, and Tang Citation2010; Wang, Li, and Cribb Citation2006; Zhang et al. Citation2005); and (4) Penman–Monteith or Priestley–Taylor methods (Cleugh et al. Citation2007; Fisher, Tu, and Baldocchi Citation2008; Leuning et al. Citation2008; Mu et al. Citation2007a; Yuan et al. Citation2010; Zhang et al. Citation2010).
The empirical methods relate ET to vegetation parameters and key environmental control factors. These methods are naturally empirical, but their applications in other areas are limited because these methods do not provide an explicit formula to follow (Lu and Zhuang Citation2010). Some methods have proposed a universal empirical method that is suitable for different land cover (Wang and Liang Citation2008; Wang et al. Citation2010). However, experiments show that the composition of tree species strongly influence ET. Land cover dependent coefficients may be a better choice than a universal formulation (Wang and Dickinson Citation2012).
For the residual method, surface temperature (Ts), air temperature (Ta), and aerodynamic resistance (ra) are first critically used to calculate sensible heat flux. Land surface temperature retrieved from thermal infrared data is strongly affected by clouds, resulting in discontinuous valid data with an error of about 1 K (Wan et al. Citation2002). Air temperature may have an unacceptable error depending on the elevation and number of distributed stations, if the ground-station measured values are interpolated spatially (Hamann and Wang Citation2005; Stahl et al. Citation2006). The difference between aerodynamic temperature and surface temperature results in the introduction of KB-1, which is a function of the structural characteristics of the vegetation, level of water stress, and climatic conditions (Lhomme et al. Citation1997), and is difficult to estimate precisely, especially on a regional or global scale. In addition, remote sensing can only directly capture instantaneous information, which may not meet the requirements of all applications (Li et al. Citation2009). The residual method may not be suitable for the estimation of period-averaged ET because of the difficulties in using 8- or 16-day composites of once-daily measurements of radiative surface temperature (Cleugh et al. Citation2007).
Although triangle/trapezoid methods are simple, and require only Ts and the vegetation index, they have the following potential limitations: (1) determination of the dry and wet edges requires a certain degree of subjectivity; (2) a large number of pixels over a flat area with a wide range of soil wetness and fractional vegetation cover (Fv) are required to ensure that dry and wet limits exist in the triangle/trapezoid space (Sun et al. Citation2012). Zhang et al. (2005, 2008) determined the true dry edge based on an energy balance equation to overcome the limitations mentioned above. However, the estimated ET is still an instantaneous value.
The main challenge in the practical application of the Penman–Monteith or Priestley–Taylor equation is to determinate the surface resistance, which is related to LAI, soil moisture status, leaf physiological attributes, and soil attributes. Based on some definitions and assumptions, Kelliher et al. (Citation1995) derived an expression for surface resistance that considered the effects of soil and canopy. Leuning et al. (Citation2008) introduced a factor in the surface resistance model that indicates the ratio of the actual ET to the potential ET. Cleugh et al. (Citation2007) adopted the Penman–Monteith equation to estimate the 16-day ET based on a canopy resistance sub-model driven by MODIS-derived vegetation data and daily surface meteorological inputs including incoming solar radiation, surface air temperature, and vapor pressure deficit (VPD). Mu et al. (Citation2007a) further modified the model developed by Cleugh et al. to estimate the global ET, and its partitioning into soil evaporation and vegetation transpiration. In their soil evaporation sub-model, soil resistance is controlled by air relative humidity, assuming that it reflects soil moisture status (Fisher, Tu, and Baldocchi Citation2008). Based on the model described by Mu et al. (Citation2007a), Zhang et al. (Citation2010) developed a model to estimate ET using remotely sensed NDVI data. Yuan et al. (Citation2010) modified Mu et al.'s (Citation2007a) model by adding the constraint of air temperature to stomatal conductance and calculating the vegetation cover fraction using LAI instead of EVI. Vinukollu et al. (Citation2011) pointed out that Mu et al.'s (Citation2007a) model underestimated ET for grassland, cropland, and woody savanna. Mu et al. (Citation2011) improved Mu et al.'s (Citation2007a) model by adding a nighttime ET component and wet soil surface component to enhance the ET estimates.
Soil resistance is largely controlled by soil moisture. However, directly monitoring large-scale soil moisture is always a challenge for remote sensing. Passive microwave sensors, such as TRMM and AMSR-E, have 1–1.5 passes per day but a coarse spatial resolution (25 km) that can only be used to estimate global or large-area soil moisture. Conversely, active microwave sensors (SAR: Synthetic Aperture Radar) have higher spatial resolution (10–30 m), but low repeat intervals (once in 16–25 days). Optical–thermal infrared sensors, such as MODIS and AVHRR/NOAA, have moderate resolution (250 m to 1 km) and one or two overpasses per day, which is suitable for regional- or large-scale applications. In addition, the triangle/trapezoid method formed by land surface temperature and vegetation index data is considered as a simple and suitable method to monitor soil moisture, and has been applied by many researchers (Gao et al. Citation2011; Mallick, Bhattacharya, and Patel Citation2009; Sandholt, Rasmussen, and Andersen Citation2002; Wang et al. Citation2004). However, this method has the same disadvantages as the triangle ET method. To reduce the uncertainties associated with the determination of the dry edge, Zhang et al. (Citation2008) developed the theoretical dry edge based on the energy balance equation. Then, Sun et al. (Citation2012) showed that the theoretical dry edge could monitor soil moisture better than the traditional dry edge, which is fitted by scatterplots.
Mu et al. (Citation2007b) have pointed out that the use of VPD alone is not enough to indicate the effects of the environmental water stress on plant growth for the arid and semiarid areas. For the similar reason, the VPD alone should not be enough to represent the water stress on ET especially in the arid and semiarid regions. In this study, we incorporated a soil moisture index (SMI), extracted from Ts–Fv space, into the Penman–Monteith equation to estimate ET over semiarid areas, which is abbreviated as the PM-SMI method. We then compared this method with those developed by Mu et al. (Citation2007a), Mu et al. (Citation2011), and Yuan et al. (Citation2010), which are abbreviated as PM-Mu2007, PM-Yuan, and PM-Mu2011 below, in which soil evaporation is controlled by relative humidity.
2. ET algorithms
Soil evaporation in PM-Mu2007, PM-Mu2011, and PM-Yuan is limited by relative humidity, assuming that VPD (saturation vapor pressure deficit) can be used as an indicator of environmental water stress (Fisher, Tu, and Baldocchi Citation2008; Granger and Gray Citation1989). The PM-SMI algorithm is revised from PM-Mu2007, and we used SMI instead of the relative humidity, as soil moisture control.
2.1. PM-Mu2007 algorithm
Net radiation (Rn) is linearly partitioned between the canopy and the soil surface using Fv,
2.1.1. Vegetation transpiration
Vegetation transpiration is calculated as:
2.1.2. Soil evaporation
Relative humidity is used as a constraint for potential soil evaporation to calculate actual soil evaporation:
2.2. PM-SMI algorithm
In the PM-SMI algorithm, vegetation transpiration is calculated the same way as PM-Mu2007. Soil evaporation is estimated by incorporating soil moisture control as follows:
shows the logic behind the PM-SMI ET algorithm for estimating daily ET.
2.3. PM-Mu2011 algorithm
Mu et al. (Citation2011) added two items to improve ET estimation, evaporation from the wet canopy surface (λEwet) and nighttime ET. The total λE is expressed as:
2.4. PM-Yuan algorithm
PM-Yuan adopted the same equations as PM-Mu2007, except for m(Tmin), which follows the equation detailed by Fisher, Tu, and Baldocchi (Citation2008):
3. Data
To compare the performance of ET algorithms applied to different vegetation cover conditions, we used two data-sets. One data-set included seven sites in the Southern Great Plain (SGP) collected from 2004. The SGP is located in the middle of the USA, and primarily covered by grassland and cropland with low vegetation cover. The other data-set included three sites of the AmeriFlux network sampled in 2005. These sites are located in eastern USA, and primarily covered by forest with high vegetation cover.
3.1. SGP
The SGP site was the first field measurement site established by the Atmospheric Radiation Measurement Program. More than 30 instrument clusters have been placed around the SGP site, at the Central Facility and at Boundary, Extended, and Intermediate Facilities. The locations for the instruments were selected so that the measurements reflect conditions over the typical distribution of land uses within the site. The SGP covers most of Oklahoma and the southern part of Kansas, which is a heterogeneous land cover area characterized by mixed farming, interrupted forest, and tall and short grass. Major soil types are silt loam, loamy sand, and loam. The region produces much of the nation's grain and fiber, including more than 60% of the wheat and 36% of the cotton. Water supplied as irrigation in the region amounts to billions of cubic meters (2×1010 m3 in 1990) annually and is governed by the determination of ET in the region. An area including seven study sites (), was selected in this study, and the Energy Balance Bowen Ratio (EBBR) system was placed to obtain ET measurements.
Table 1. Location, soil type and land cover of study sites.
EBBR produces 30-minute estimates of the vertical fluxes of sensible and latent heat at the local surface. Flux estimates are calculated from the observations of net radiation, soil surface heat flux, and the vertical gradients of temperature and relative humidity. Meteorological data collected by the EBBR are used to calculate the bulk aerodynamic fluxes, which are used in the Bulk Aerodynamic Technique EBBR value-added product to replace sunrise and sunset spikes in the flux data. A unique aspect of the system is the automatic exchange mechanism which helps to reduce errors from instrument offset drift.
λE is estimated as a function of the Bowen ratio β:
3.2. AmeriFlux sites
An area in the eastern USA including three flux towers, Duke Forest Hardwoods, North Carolina Loblolly Pine, and Walker Branch, were selected. Detailed information regarding these sites is listed in .
The ET at the AmeriFlux sites was measured by the eddy covariance (EC) method (http://public.ornl.gov/ameriflux/data-get.cfm). The eddy covariance method is accepted as the best method for direct measurement of heat fluxes and is widely used in global measurement experiments (Baldocchi et al. Citation2001). Unfortunately, it suffers from an energy imbalance problem, defining the energy closure ratio (ECR) as:
3.3. Remote sensing data
Four MODIS products, including the 16-day vegetation indices at 1-km resolution (MOD13A2), daily Ts products at 1-km resolution (MOD11A1), 8-day albedo products at 1-km resolution (MCD43B3), and 8-day ET products (MOD16A2) at 1-km resolution were used. These data were acquired through the EOS data gateway (https://wist.echo.nasa.gov), except for the MOD16A2 which were downloaded from frp://ftp.ntsg.umt.edu. Two algorithms were used to retrieve Ts from the MODIS thermal and middle infrared spectral regions: the generalized split window algorithm (Wan and Dozier Citation1996) and the MODIS day/night land surface temperature algorithm (Wan and Li Citation1997). The accuracy of the MODIS Ts product is better than 1 K (Wan et al. Citation2002; Wan et al. Citation2004). The 16-day NDVI extracted from MOD13A2 was time series smoothed by the locally adjusted cubic spline capping (LACC) method (Chen, Feng, and Chen Citation2006), which can produce flexible and mathematically smooth capping curves and fit rapid seasonal changes, for the purpose of identifying the atmosphere-contaminated data points and their replacements through temporal interpolation. The data were then linear interpolated to give the daily value. The albedo product consists of black sky and white sky albedo. To derive the overall surface albedo, we followed the method described by Su et al. (Citation2007) by averaging the above two estimates. The MOD16 ET data-sets were estimated using the ET algorithm described by Mu et al. (Citation2011). The MOD16 ET algorithm uses NASA's MERRA GMAO (GEOS-5) daily meteorological reanalysis data, collection 4 MOD12Q1, collection 5 MCD43B2/B3 and MOD15A2 as input. The 8-day ET is the sum of ET during these 8-day time periods. All these products described above were converted to UTM projection using the Modis Reprojection Tool.
Two study regions were selected for this study. One is in the middle of the USA, containing seven SGP sites. The selected window size for the remote sensing image was 562×526 km, extended in longitude from 95.3°W to 99.5°W and in latitude from 34.5°N to 38.5°N, which is the spatial subset from tile H10V05 of 2004 and covered most of the SGP. Another is in eastern USA, containing three AmeriFlux sites, for which the selected window size of the remote sensing image was 884×592 km. This area extended in longitude from 75.1°W to 85.4°W and in latitude from 34.5°N to 39.8°N, which is the spatial subset from tile H11V05 of 2005.
3.4. Methodology
In this study, although SMI distribution could be estimated based on Ts–Fv space, we use in situ meteorological data such as Ta, RH, and Rn. Therefore, only in situ ET is estimated using measured meteorological data and 3×3 1-km pixels of remote sensing data surrounding each site. Below is the description of each step.
Deriving the dry edge using instantaneous value of air temperature, vapor pressure (e0), solar radiation (S0), Ts, albedo, and NDVI;
Calculating the SMI distribution based on the improved Ts–Fv space and extracting 3×3 pixels value of SMI, NDVI, and LAI images surrounding each study site;
Estimating daily ET using in situ daily value of air temperature, relative humidity (RH), Rn, and each pixel value of SMI, NDVI, and LAI;
The PM-SMI ET average over the 3×3 pixels was validated with tower ET observations;
Compared to the other three ET algorithms with the same input measurement data.
4. Results and discussion
shows the mean NDVI, mean LAI, max NDVI, max LAI, and mean soil water content for each site. The vegetation cover is low for SGP sites, which were covered by grassland and cropland. There is a mix of good density improved pasture in site E7; therefore, the value of NDVI and LAI in this site is relatively high (max NDVI=0.75, max LAI=3.1). The vegetation cover is high for the three forest sites. The soil type of E8 and E15 is sandy; therefore, the mean soil water content is lower than 0.15 m3/m3.
Table 2. The mean NDVI, mean LAI, max NDVI, max LAI and mean soil water content for each site. The mean and max values of NDVI and LAI were obtained from the MODIS NDVI and LAI products. The mean soil water content was obtained from surface soil moisture measurements taken at 5 cm. The data from SGP sites was collected from 2004 and the data from AmeriFlux sites was collected from 2005.
Since Ts is affected by clouds, the results of the PM-SMI algorithm were only available for less than half of the year. As shown in , the PM-SMI algorithm significantly enhanced the ET estimates for all of the SGP sites, which were covered by grassland and cropland, while the other three ET algorithms had relatively large errors. For example, at site E9, the root mean square error (RMSE) of PM-Mu2007, PM-Mu2011, PM-Yuan, and PM-SMI is 1.69, 1.64, 1.67, and 0.39 mm, respectively, while the bias is –1.49, –1.34, –1.45, and –0.03 mm, respectively, and the R2 is 0.94, 0.87, 0.95, and 0.95, respectively. Although the R2 values of the PM-SMI ET estimates are close to those of the PM-Mu2007 and PM-Yuan ET estimates, the RMSE and bias were greatly reduced, indicating that PM-SMI ET estimates capture the magnitude of ET measurements better than the other three algorithms. For all the seven SGP sites, the RMSE and bias of PM-Mu2011 (1.37 mm and –0.93 mm) are lower than that of PM-Mu2007 (1.4 mm and –1.06 mm). However, the R2 of PM-Mu2011 (0.64) is lower than the PM-Mu2007 (0.69). The RMSE, bias, and R2 of PM-Yuan fall between those of PM-2007 and PM-2011. Although PM-Mu2011 improved the ET estimates when compared with PM-Mu2007 at grasslands and croplands as reported by Mu et al. (2011), results of this study indicate that PM-Mu2011 still underestimates ET.
Table 3. Available days, root mean square error (RMSE: mm/day), absolute bias (bias: mm/day), and square of correlation coefficient (R2) of four ET algorithms for each site. (1) PM-Mu2007; (2) PM-Mu2011; (3) PM-Yuan; and (4) PM-SMI.
Among the forest sites, in Duke Forest Hardwoods (Mixed forest), North Carolina Loblolly Pine (Mixed forest) and Walker Branch (Deciduous Broad-leaf forest), there were no large differences among ET estimates of PM-Mu2007, PM-Yuan, and PM-SMI. Although PM-Mu2011 showed the lowest RMSE and bias and the highest R2 in Walker Branch, PM-Mu2011 showed the lowest correlation and highest RMSE and bias across all the three forest sites.
Across all 10 sites, the PM-SMI algorithm also had the best performance, with an RMSE of 0.88 mm, bias of 0.24 mm and R2 of 0.78. PM-Yuan ET estimates had a slightly lower RMSE and bias (1.28 mm, –0.78 mm), and higher R2 (0.66) than PM-Mu2007 (1.31 mm, –0.82 mm and 0.66) and PM-Mu2011 (1.33 mm, –0.8 mm and 0.64). PM-Mu2011 had a lower bias than PM-Mu2007, but the RMSE was higher and the R2 was lower. Mu et al. (2011) also indicated that PM-Mu2011 had a reduced bias relative to PM-Mu2007, although it had a slightly increased RMSE and decreased R2.
shows the scatterplots between the observed and the estimated ET with four algorithms. PM-Mu2007, PM-Mu2011, and PM-Yuan significantly underestimate ET and PM-SMI performs the best among the four ET algorithms. shows comparisons of the ET measurements and the ET estimates with four algorithms. The PM-SMI ET estimates match well across the annual variation for all SGP sites. PM-Mu2007, PM-Mu2011, and PM-Yuan algorithms obviously underestimated ET across SGP sites, where soil evaporation plays a dominant role. However, for E7, E20, and E27, which have relatively good vegetation condition (), these three algorithms' ET estimates are closer to the ET measurements, with a lower bias (). No significant difference was observed among the four algorithms' ET estimates for the three forest sites during the growing season. However, the PM-SMI ET estimates are closer to the ET measurements in the non-growing season when compared with the other three algorithms.
Figure 3. The ET measurements (gray line) and ET estimates with PM-Mu2007 (cyan line), PM-Mu2011 (blue line), PM-Yuan (yellow line), and PM-SMI algorithms (red line) for 10 sites.shows comparisons of the 8-day ET measurements with the PM-SMI 8-day ET estimates and MODIS 8-day ET product. For the PM-SMI ET estimates, it was difficult to obtain continuous daily ET owing to the lack of continuous Ts. Therefore, the valid PM-SMI 8-day ET was combined for every 8-day period, during which there are more than six valid daily ET values. The MODIS 8-day ET was extracted directly from the MOD16A2 product. As shown in , the MODIS 8-day ET was significantly lower than the ET measurements for the SGP sites, with R2, RMSE, and bias values of 0.51, 10.58 mm, and –6.72 mm, respectively. In contrast, most of the PM-SMI 8-day ET estimates were around the 1:1 line. However, the PM-SMI 8-day ET estimates were slightly larger than the ET measurements, with a bias of 4.02 mm. The PM-SMI daily ET was estimated based on clear day conditions with no clouds, resulting in larger ET values when compared to those obtained for cloudy days. Therefore, it is reasonable that the PM-SMI 8-day ET estimates combined from the cloudless daily ET were slightly larger than the measurements. The R2 and RMSE of the PM-SMI 8-day ET estimates were 0.68 and 7.82 mm, respectively.
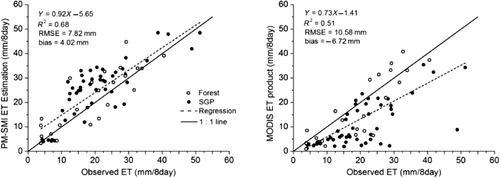
However, relatively large differences between PM-SMI ET estimates and ET measurements still exist for a few sites, including E8, E13, E15, and Walker Branch. These discrepancies may be explained by the following:
Estimation error of soil heat flux. Surface available energy used in the Penman–Monteith equation is estimated from net radiation minus soil heat flux. However, soil heat flux, which is correlated with soil properties, soil moisture, and vegetation condition, has high spatial variability. The ratio between G and Rn over bare soil ranges from 0.20 to 0.50 depending on the soil moisture (Idso, Aase, and Jackson Citation1975), and from 0.05 to 0.30 depending on the vegetation cover (Clothier et al. Citation1986; Kustas and Daughtry Citation1990). Therefore, it is a challenge to estimate soil heat flux. In our study, relative bias of G ranged from –30% to 30%, which may result in some uncertainties associated with the ET estimates.
Algorithm limitations. Biophysical parameters (CL, VPDclose, and VPDopen) used in this study are the same as those used in the PM-Mu 2011 algorithm. However, Mu et al. (Citation2007a), Mu et al. (Citation2011), Yuan et al. (Citation2010), and Zhang et al. (Citation2010), all have recalibrated the biophysical parameters of Penman–Monteith ET algorithms based on their studies. The parameters such as a and b, which are used to compute soil resistance (rs) from SMI, are also the same as those used by Kustas, Zhan, and Schmugge (Citation1998). However, these values are a function of soil texture, which is heterogeneous in our study region, and consists of silt loam, sandy loam, clay loam, and loamy sand. Unfortunately, ground measurement sites for different biome types or soil textures are not sufficient for analysis. Therefore, it is not possible to refit these parameters.
SMI. The SMI incorporated in the PM-SMI algorithm is estimated based on improved Ts–Fv trapezoid space (Sun et al. Citation2012). The assumption of monitoring soil moisture based on Ts–Fv space is that meteorological parameters and land surface attributes are homogeneous (Friedl and Davis Citation1994; Lambin and Ehrlich Citation1995; Zhang et al. Citation2008). However, Sun et al. (Citation2012) showed that sites with sandy soil, such as E8 and E15, could result in an evident difference in heat property and relationships between Ts and soil moisture when compared to other soil types, which was likely a major cause of large errors at E8 and E15.
Inaccuracy of ET measurements. Surface energy flux data from the SGP sites measured by Bowen ratio do not have energy balance non-closure problem. However, data from three forest sites, measured by the eddy covariance flux towers, have lack of energy balance closure. Although latent heat flux was corrected using the method of Twine et al. (Citation2000), correcting error and reducing uncertainty in the ET measurements is still uncertain due to the closure error (Shuttleworth Citation2007). The large errors at the Walker Branch site may result from a low energy balance ratio, which is about 50% on most days.
Validation. We found that some research used one pixel containing the study site to validate (Yuan et al., Citation2010), some research used 3×3 pixels to validate (Mu et al. Citation2007a), and some research used footprint model to validate (Jia et al. Citation2012). To reduce the effect of heterogeneity of land surface, we used 3×3 pixels surrounding the study sites to validate the results. Actually, this is a simplified method while the area of these pixels could mostly represent the source area of flux measurements. The source area is always varying with the observation height, wind velocity and direction, atmospheric stability, and the roughness length of land surface. For the EC and EBBR measurements, which used in this study, the source area of them might either located in only one MODIS pixel or covered some parts of two to four pixels, with different relative contribution within the source area.
5. Conclusions
Penman–Monteith equation is a classical method used to calculate ET. However, surface resistance, which is related to soil moisture, is always difficult to obtain. In this study, we developed an ET algorithm by incorporating the SMI derived from an improved Ts–Fv space. The PM-Mu2007algorithm has been successfully applied on the global scale (Mu et al. Citation2007a; Mu et al. Citation2009), and was recently adjusted by Yuan et al. (Citation2010) and Zhang et al. (Citation2009). Mu et al. (Citation2011) pointed out that PM-Mu2007 underestimates ET for cropland, grassland, and woody savanna, which was also confirmed by other studies (Vinukollu et al. Citation2011). Mu et al. (Citation2011) improved this ET algorithm and enhanced the ET estimates most for woody savannas, grasslands, and croplands. Soil evaporation in these ET algorithms was controlled by VPD. However, the VPD alone is not enough to represent the water stress on ET especially in the arid and semiarid regions (Mu et al. Citation2007b). Generally in the arid and semiarid areas, the vegetation cover fraction is low, the plant transpiration is low as well, and hence the soil evaporation is the largest part of the ET components.
In this study, we tried to improve Mu et al.'s 2007 ET algorithm by incorporating the SMI to account for the soil moisture constraint on soil evaporation to develop the PM-SMI ET algorithm. ET estimates derived from PM-SMI algorithm were compared with PM-Mu2007, PM-Mu2011, and PM-Yuan algorithm and validated by seven SGP sites that were covered by cropland and grassland with low vegetation cover, as well as three AmeriFlux sites covered by forest with high vegetation cover. The results showed that the PM-SMI algorithm significantly improved daily ET estimates at SGP sites with an RMSE of 0.91 mm, bias of 0.33 mm, and R2 of 0.77. PM-Mu2011 reduced the RMSE and bias of ET estimates when compared with PM-Mu2007, but still underestimated ET. For the three forest sites, no large difference was observed among the ET estimates produced by these four algorithms during the growing season. However, the PM-SMI ET estimates are closer to the ET measurements in the non-growing season when compared with the other three algorithms. For all 10 validation sites, PM-SMI also had the best performance, followed by PM-Yuan.
PM-SMI 8-day ET estimates were also compared with MODIS 8-day ET product (MOD16A2). MODIS 8-day ET was significantly lower than the ET measurements for the SGP sites, with R2, RMSE, and bias values of 0.51, 10.58 mm, and –6.72 mm, respectively. In contrast, most PM-SMI 8-day ET estimates were around the 1:1 line, with R 2, RMSE, and bias values of 0.68, 7.82 mm, and 4.02 mm, respectively.
ET distribution is necessary in many applications, and the method proposed in this manuscript would be a meaningful attempt to get the ET maps. Unfortunately, the input meteorological data, such as net radiation (Rn) was only collected from study sites. We could not get a good product of Rn distribution of the study area. We will try to make some analysis about the ET distribution in the future. The application of PM-SMI is limited by land surface temperature, which is used to obtain the SMI, since surface temperature is largely affected by clouds and it is difficult to capture consistent and valid data. Passive microwave sensors, such as AMSR-E, which have all-weather surface sensing capability, have been used to retrieve soil water content (Bindlish et al. Citation2003; Njoku et al. Citation2003). However, the application of this technique is limited by its coarse spatial resolution (25 km). In the future, we plan to downscale the soil moisture product derived from AMSR-E to fine scales matching MODIS 1 km resolution.
Acknowledgements
This work was funded by the High-Tech Research and Development Program of China (No. 2009AA122100) and the National Science and Technology Ministry (2012BAH29B02). We thank Dr Tongren Xu for providing the measurement data. SGP data of the Atmospheric Radiation Measurement (ARM) Project of the US Department of Energy is downloaded from http://www.arm.gov/. Eddy covariance flux tower sites are part of the AmeriFlux. We gratefully acknowledge the efforts of researchers at these sites. Research at the Duke Forest Hardwoods, North Carolina Loblolly Pine and Walker Branch sites was led by Ram Oren, Asko Noormets and Tilden Meyers.
References
- Anderson, M. C., J. M. Norman, G. R. Diak, W. P. Kustas, and J. R. Mecikalski. 1997. “A Two-Source Time-Integrated Model for Estimating Surface Fluxes using Thermal Infrared Remote Sensing.” Remote Sensing of Environment 60 (2): 195–216. doi:10.1016/S0034-4257(96)00215-5.
- Baldocchi, D., E. Falge, L. Gu, R. Olson, D. Hollinger, S. Running, P. Anthoni, et al. 2001. “FLUXNET: A New Tool to Study the Temporal and Spatial Variability of Ecosystem-Scale Carbon Dioxide, Water Vapor, and Energy Flux Densities.” Bulletin of the American Meteorological Society 82: 2415–2434. doi:10.1175/1520-0477(2001)082<2415:FANTTS>2.3.CO;2.
- Bastiaanssen, W. G. M., M. Menenti, R. A. Feddes, and A. A. M. Holtslag. 1998. “A Remote Sensing Surface Energy Balance Algorithm for Land (SEBAL). 1. Formulation.” Journal of Hydrology 212–213: 198–212. doi:10.1016/S0022-1694(98)00253-4.
- Bella, C. M., C. M. Rebella, and J. M. Paruelo. 2000. “Evapotranspiration Estimates using NOAA AVHRR Imagery in the Pampa Region of Argentina.” International Journal of Remote Sensing 21 (4): 791–797. doi:10.1080/014311600210579.
- Bindlish, R., T. J. Jackson, E. Wood, H. Gao, P. Starks, D. Bosch, and V. Lakshmi. 2003. “Soil Moisture Estimates from TRMM Microwave Imager Observations over the Southern United States.” Remote Sensing of Environment 85 (4): 507–515. doi:10.1016/S0034-4257(03)00052-X.
- Brutsaert, W. 1975. “On a Derivable Formula for Long-Wave Radiation from Clear Skies.” Water Resources Research 11 (5): 742–744. doi:10.1029/WR011i005p00742.
- Carlson, T. N., W. J. Capehart, and R. R. Gillies. 1995. “A New Look at the Simplified Method for Remote Sensing of Daily Evapotranspiration.” Remote Sensing of Environment 54 (2): 161–167. doi:10.1016/0034-4257(95)00139-R.
- Chen, J., D. Feng, and M. Chen. 2006. “Locally Adjusted Cubic-Spline Capping for Reconstructing Seasonal Trajectories of a Satellite-Derived Surface Parameter.” IEEE Transactions on Geoscience and Remote Sensing 44 (8): 2230–2238. doi:10.1109/TGRS.2006.872089.
- Cleugh, H., R. Leuning, Q. Mu, and S. Running. 2007. “Regional Evaporation Estimates from Flux Tower and MODIS Satellite Data.” Remote Sensing of Environment 106 (3): 285–304. doi:10.1016/j.rse.2006.07.007.
- Clothier, B. E., K. L. Clawson, P. J. Pinter, M. S. Moran, R. J. Reginato, and R. D. Jackson. 1986. “Estimation of Soil Heat Flux from Net Radiation during the Growth of Alfalfa.” Agricultural and Forest Meteorology 37 (4): 319–329. doi:10.1016/0168-1923(86)90069-9.
- Fisher, J. B., K. P. Tu, and D. D. Baldocchi. 2008. “Global Estimates of the Land–Atmosphere Water Flux based on Monthly AVHRR and ISLSCP-II Data, Validated at 16 FLUXNET Sites.” Remote Sensing of Environment 112 (3): 901–919. doi:10.1016/j.rse.2007.06.025.
- Friedl, M. A., and F. W. Davis. 1994. “Sources of Variation in Radiometric Surface Temperature over a Tallgrass Prairie.” Remote Sensing of Environment 48 (1): 1–17. doi:10.1016/0034-4257(94)90109-0.
- Gao, Z. 2005. “Determination of Soil Heat Flux in a Tibetan Short-Grass Prairie.” Boundary-Layer Meteorology 114 (1): 165–178. doi:10.1007/s10546-004-8661-5.
- Gao, Z., W. Gao, and N.-B. Chang. 2011. “Integrating Temperature Vegetation Dryness Index (TVDI) and Regional Water Stress Index (RWSI) for Drought Assessment with the Aid of LANDSAT TM/ETM+ Images.” International Journal of Applied Earth Observation and Geoinformation 13 (3): 495–503. doi:10.1016/j.jag.2010.10.005.
- Granger, R. J., and D. M. Gray. 1989. “Evaporation from Natural Nonsaturated Surfaces.” Journal of Hydrology 111 (1–4): 21–29. doi:10.1016/0022-1694(89)90249-7.
- Halliwell, D. H., and W. R. Rouse. 1987. “Soil Heat Flux in Permafrost: Characteristics and Accuracy of Measurement.” Journal of Climatology 7 (6): 571–584. doi:10.1002/joc.3370070605.
- Hamann, A., and T. L. Wang. 2005. “Models of Climatic Normals for Genecology and Climate Change Studies in British Columbia.” Agricultural and Forest Meteorology 128 (3–4): 211–221. doi:10.1016/j.agrformet.2004.10.004.
- Idso, S. B., J. K. Aase, and R. D. Jackson. 1975. “Net Radiation — Soil Heat Flux Relations as Influenced by Soil Water Content Variations.” Boundary-Layer Meteorology 9 (1): 113–122. doi:10.1007/BF00232257.
- Jacobsen, A. 1999. “Estimation of the Soil Heat Flux/Net Radiation Ratio Based on Spectral Vegetation Indexes in High-Latitude Arctic Areas.” International Journal of Remote Sensing 20 (2): 445–461. doi:10.1080/014311699213532.
- Jia, Z., S. Liu, Z. Xu, Y. Chen, and M. Zhu. 2012. “Validation of Remotely Sensed Evapotranspiration over the Hai River Basin, China.” Journal of Geophysical Research: Atmospheres 117 (D13): D13113. doi:10.1029/2011JD017037.
- Jiang, L., and S. Islam. 1999. “A Methodology for Estimation of Surface Evapotranspiration over Large Areas using Remote Sensing Observations.” Geophysical Research Letters 26 (17): 2773–2776. doi:10.1029/1999GL006049.
- Jiang, L., and S. Islam. 2001. “Estimation of Surface Evaporation Map over Southern Great Plains using Remote Sensing Data.” Water Resources Research 37 (2): 329–340. doi:10.1029/2000WR900255.
- Kelliher, F. M., R. Leuning, M. R. Raupach, and E. D. Schulze. 1995. “Maximum Conductances for Evaporation from Global Vegetation Types.” Agricultural and Forest Meteorology 73 (1–2): 1–16. doi:10.1016/0168-1923(94)02178-M.
- Kustas, W. P., and C. S. T. Daughtry. 1990. “Estimation of the Soil Heat Flux/Net Radiation Ratio from Spectral Data.” Agricultural and Forest Meteorology 49 (3): 205–223. doi:10.1016/0168-1923(90)90033-3.
- Kustas, W. P., X. Zhan, and T. J. Schmugge. 1998. “Combining Optical and Microwave Remote Sensing for Mapping Energy Fluxes in a Semiarid Watershed.” Agricultural and Forest Meteorology 64: 116–131.
- Lambin, E. F., and D. Ehrlich. 1995. “Combining Vegetation Indices and Surface Temperature for Land-Cover Mapping at Broad Spatial Scales.” International Journal of Remote Sensing 16 (3): 573–579. doi:10.1080/01431169508954423.
- Leuning, R., Y. Q. Zhang, A. Rajaud, H. Cleugh, and K. Tu. 2008. “A Simple Surface Conductance Model to Estimate Regional Evaporation using MODIS Leaf Area Index and the Penman–Monteith Equation.” Water Resources Research 44: 10. doi:10.1029/2007WR006562.
- Lhomme, J. P., D. Troufleau, B. Monteny, A. Chehbouni, and S. Bauduin. 1997. “Sensible Heat Flux and Radiometric Surface Temperature over Sparse Sahelian Vegetation II. A Model for the kB-1 Parameter.” Journal of Hydrology 188–189: 839–854. doi:10.1016/S0022-1694(96)03173-3.
- Li, Z., R. Tang, Z. Wan, Y. Bi, C. Zhou, B. Tang, G. Yan, and X. Zhang. 2009. “A Review of Current Methodologies for Regional Evapotranspiration Estimation from Remotely Sensed Data.” Sensors 9 (5): 3801–3853. doi:10.3390/s90503801.
- Lu, X., and Q. Zhuang. 2010. “Evaluating Evapotranspiration and Water-Use Efficiency of Terrestrial Ecosystems in the Conterminous United States using MODIS and AmeriFlux Data.” Remote Sensing of Environment 114 (9): 1924–1939. doi:10.1016/j.rse.2010.04.001.
- Mallick, K., B. K. Bhattacharya, and N. K. Patel. 2009. “Estimating Volumetric Surface Moisture Content for Cropped Soils using a Soil Wetness Index Based on Surface Temperature and NDVI.” Agricultural and Forest Meteorology 149 (8): 1327–1342. doi:10.1016/j.agrformet.2009.03.004.
- Monteith, J. L., ed. 1973. Principles of Environmental Physics. London: Edward Arnold.
- Moran, M. S., T. R. Clarke, Y. Inoue, and A. Vidal. 1994. “Estimating Crop Water Deficit using the Relation between Surface-Air Temperature and Spectral Vegetation Index.” Remote Sensing of Environment 49 (3): 246–263. doi:10.1016/0034-4257(94)90020-5.
- Mu, Q., F. A. Heinsch, M. Zhao, and S. W. Running. 2007a. “Development of a Global Evapotranspiration Algorithm Based on MODIS and Global Meteorology Data.” Remote Sensing of Environment 111 (4): 519–536. doi:10.1016/j.rse.2007.04.015.
- Mu, Q., L. A. Jones, J. S. Kimball, K. C. McDonald, and S. W. Running. 2009. “Satellite Assessment of Land Surface Evapotranspiration for the Pan-Arctic Domain.” Water Resources Research 45 (9): W09420. doi:10.1029/2008WR007189.
- Mu, Q., M. Zhao, F. A. Heinsch, M. Liu, H. Tian, and S. W. Running. 2007b. “Evaluating Water Stress Controls on Primary Production in Biogeochemical and Remote Sensing Based Models.” Journal of Geophysical Research: Biogeosciences 112: G01012. doi:10.1029/2006JG000179.
- Mu, Q., M. Zhao, and S. W. Running. 2011. “Improvements to a MODIS Global Terrestrial Evapotranspiration Algorithm.” Remote Sensing of Environment 115 (8): 1781–1800. doi:10.1016/j.rse.2011.02.019.
- Mueller, R. W., K. F. Dagestad, P. Ineichen, M. Schroedter-Homscheidt, S. Cros, D. Dumortier, R. Kuhlemann, et al. 2004. “Rethinking Satellite-Based Solar Irradiance Modelling: The SOLIS Clear-Sky Module.” Remote Sensing of Environment 91 (2): 160–174. doi:10.1016/j.rse.2004.02.009.
- Njoku, E. G., T. J. Jackson, V. Lakshmi, T. K. Chan, and S. V. Nghiem. 2003. “Soil Moisture Retrieval from AMSR-E.” IEEE Transactions on Geosciences and Remote Sensing 41: 215–229. doi:10.1109/TGRS.2002.808243.
- Norman, J. M., W. P. Kustas, and K. S. Humes. 1995. “Source Approach for Estimating Soil and Vegetation Energy Fluxes in Observations of Directional Radiometric Surface Temperature.” Agricultural and Forest Meteorology 77 (3–4): 263–293. doi:10.1016/0168-1923(95)02265-Y.
- Oki, T., and S. Kanae. 2006. “Global Hydrological Cycles and World Water Resources.” Science 313 (5790): 1068–1072. doi:10.1126/science.1128845.
- Rouse, W. R. 1984. “Microclimate at Arctic Tree Line 3. The Effects of Regional Advection on the Surface Energy Balance of Upland Tundra.” Water Resources Research 20 (1): 74–78. doi:10.1029/WR020i001p00074.
- Sandholt, I., K. Rasmussen, and J. Andersen. 2002. “A Simple Interpretation of the Surface Temperature/Vegetation Index Space for Assessment of Surface Moisture Status.” Remote Sensing of Environment 79 (2–3): 213–224. doi:10.1016/S0034-4257(01)00274-7.
- Sellers, P. J., M. D. Heiser, and F. G. Hall. 1992. “Relations between Surface Conductance and Spectral Vegetation Indices at Intermediate (100 m2 to 15 km2) Length Scales.” Journal of Geophysical Research 97 (D17): 19033–19059. doi:10.1029/92JD01096.
- Shuttleworth, W. J. 2007. “Putting the “Vap” into Evaporation.” Hydrology and Earth System Sciences 11 (1): 210–244. doi:10.5194/hess-11-210-2007.
- Stahl, K., R. D. Moore, J. A. Floyer, M. G. Asplin, and I. G. McKendry. 2006. “Comparison of Approaches for Spatial Interpolation of Daily Air Temperature in a Large Region with Complex Topography and Highly Variable Station Density.” Agricultural and Forest Meteorology 139 (3–4): 224–236. doi:10.1016/j.agrformet.2006.07.004.
- Stisen, S., I. Sandholt, A. Nørgaard, R. Fensholt, and K. H. Jensen. 2008. “Combining the Triangle Method with Thermal Inertia to Estimate Regional Evapotranspiration – Applied to MSG-SEVIRI Data in the Senegal River Basin.” Remote Sensing of Environment 112 (3): 1242–1255. doi:10.1016/j.rse.2007.08.013.
- Su, Z. 2002. “The Surface Energy Balance System SEBS for Estimation of Turbulent Heat Fluxes.” Hydrology and Earth System Sciences 6: 85–99. doi:10.5194/hess-6-85-2002.
- Su, H., E. F. Wood, M. F. Mccabe, and Z. Su. 2007. “Evaluation of Remotely Sensed Evapotranspiration over the CEOP EOP-1 Reference Sites.” Journal of the Meteorological Society of Japan 85A: 439–459. doi:10.2151/jmsj.85A.439.
- Sun, L., R. Sun, X. Li, S. Liang, and R. Zhang. 2012. “Monitoring surface soil moisture status based on remotely sensed surface temperature and vegetation index information.” Agricultural and Forest Meteorology 166–167 (0): 175–187. doi:10.1016/j.agrformet.2012.07.015.
- Tang, R., Z. Li, and B. Tang. 2010. “An Application of the Ts–VI Triangle Method with Enhanced Edges Determination for Evapotranspiration Estimation from MODIS Data in Arid and Semi-Arid Regions: Implementation and Validation.” Remote Sensing of Environment 114 (3): 540–551. doi:10.1016/j.rse.2009.10.012.
- Thornton, P. E. 1998. Regional Ecosystem Simulation: Combining Surface- and Satellite-based Observations to Study Linkages between Terrestrial Energy and Mass Budgets. Missoula: University of Montana.
- Trenberth, K. E., J. Fasullo, and J. Kiehl. 2009. “Earth's Global Energy Budget.” Bulletin of the American Meteorological Society 90 (3): 311–323. doi:10.1175/2008BAMS2634.1.
- Twine, T. E., W. P. Kustas, J. M. Norman, D. R. Cook, P. R. Houser, T. P. Meyers, J. H. Prueger, P. J. Starks, and M. L. Wesely. 2000. “Correcting Eddy-Covariance Flux Underestimates over a Grassland.” Agricultural and Forest Meteorology 103 (3): 279–300. doi:10.1016/S0168-1923(00)00123-4.
- Vörösmarty, C. J., C. A. Federer, and A. L. Schloss. 1998. “Potential Evaporation Functions Compared on US Watersheds: Possible Implications for Global-Scale Water Balance and Terrestrial Ecosystem Modeling.” Journal of Hydrology 207 (3–4): 147–169.
- Vinukollu, R. K., E. F. Wood, C. R. Ferguson, and J. B. Fisher. 2011. “Global Estimates of Evapotranspiration for Climate Studies using Multi-Sensor Remote Sensing Data: Evaluation of Three Process-Based Approaches.” Remote Sensing of Environment 115 (3): 801–823. doi:10.1016/j.rse.2010.11.006.
- Wan, Z., and J. Dozier. 1996. “A Generalized Split-Window Algorithm for Retrieving Land-Surface Temperature from Space.” IEEE Transactions on Geoscience and Remote Sensing 34 (4): 892–905. doi:10.1109/36.508406.
- Wan, Z., and Z. L. Li. 1997. “A Physics-Based Algorithm for Retrieving Land-surface Emissivity and Temperature from EOS/MODIS Data.” IEEE Transactions on Geoscience and Remote Sensing 35 (4): 980–996. doi:10.1109/36.602541.
- Wan, Z., Y. Zhang, Q. Zhang, and Z.-l. Li. 2002. “Validation of the Land-Surface Temperature Products Retrieved from Terra Moderate Resolution Imaging Spectroradiometer Data.” Remote Sensing of Environment 83 (1–2): 163–180. doi:10.1016/S0034-4257(02)00093-7.
- Wan, Z., Y. Zhang, Q. Zhang, and Z. Li. 2004. “Quality Assessment and Validation of the MODIS Global Land Surface Temperature.” International Journal of Remote Sensing 25 (1): 261–274. doi:10.1080/0143116031000116417.
- Wang, K., and R. E. Dickinson. 2012. “A Review of Global Terrestrial Evapotranspiration: Observation, Modeling, Climatology, and Climatic Variability.” Reviews of Geophysics 50 (2): G2005. doi:10.1029/2011RG000373.
- Wang, K., R. E. Dickinson, M. Wild, and S. Liang. 2010. “Evidence for Decadal Variation in Global Terrestrial Evapotranspiration between 1982 and 2002: 1. Model Development.” Journal of Geophysical Research 115 (D20): D20112. doi:10.1029/2009JD013671.
- Wang, K., Z. Li, and M. Cribb. 2006. “Estimation of Evaporative Fraction from a Combination of Day and Night Land Surface Temperatures and NDVI: A New Method to Determine the Priestley–Taylor Parameter.” Remote Sensing of Environment 102 (3–4): 293–305. doi:10.1016/j.rse.2006.02.007.
- Wang, K., and S. Liang. 2008. “An Improved Method for Estimating Global Evapotranspiration Based on Satellite Determination of Surface Net Radiation, Vegetation Index, Temperature, and Soil Moisture.” Journal of Hydrometeorology 9 (4): 712. doi:10.1175/2007JHM911.1.
- Wang, C., S. Qi, Z. Niu, and J. Wang. 2004. “Evaluating Soil Moisture Status in China using the Temperature–Vegetation Dryness Index (TVDI).” Canadian Journal of Remote Sensing 30 (5): 671–679. doi:10.5589/m04-029.
- Wilson, K., A. Goldstein, E. Falge, M. Aubinet, D. Baldocchi, P. Berbigier, C. Bernhofer, et al. 2002. “Energy Balance Closure at FLUXNET Sites.” Agricultural and Forest Meteorology 113 (1–4): 223–243. doi:10.1016/S0168-1923(02)00109-0.
- Yang, K., T. Koike, H. Ishikawa, and Y. Ma. 2004. “Analysis of the Surface Energy Budget at a Site of GAME/Tibet using a Single-Source Model.” Journal of the Meteorological Society of Japan 82: 131–153. doi:10.2151/jmsj.82.131.
- Yuan, W., S. Liu, G. Yu, J.-M. Bonnefond, J. Chen, K. Davis, A. R. Desai, A. H. Goldstein, D. Gianelle, and F. Rossi. 2010. “Global Estimates of Evapotranspiration and Gross Primary Production Based on MODIS and Global Meteorology Data.” Remote Sensing of Environment 114 (7): 1416–1431. doi:10.1016/j.rse.2010.01.022.
- Zaksek, K., and M. Schroedter-Homscheidt. 2009. “Parameterization of Air Temperature in High Temporal and Spatial Resolution from a Combination of the SEVIRI and MODIS Instruments.” ISPRS Journal of Photogrammetry and Remote Sensing 64 (4): 414–421. doi:10.1016/j.isprsjprs.2009.02.006.
- Zhang, K., J. S. Kimball, Q. Z. Mu, L. A. Jones, S. J. Goetz, and S. W. Running. 2009. “Satellite Based Analysis of Northern ET Trends and Associated Changes in the Regional Water Balance from 1983 to 2005.” Journal of Hydrology 379 (1–2): 92–110. doi:10.1016/j.jhydrol.2009.09.047.
- Zhang, K., J. S. Kimball, R. R. Nemani, and S. W. Running. 2010. “A Continuous Satellite-Derived Global Record of Land Surface Evapotranspiration from 1983 to 2006.” Water Resources Research 46 (9): W09522.
- Zhang, R., X. Sun, W. Wang, J. Xu, Z. Zhu, and J. Tian. 2005. “An Operational Two-Layer Remote Sensing Model to Estimate Surface Flux in Regional Scale: Physical Background.” Science in China (Earth Sciences) 48 (z1): 225–244.
- Zhang, R., J. Tian, H. Su, X. Sun, S. Chen, and J. Xia. 2008. “Two Improvements of an Operational Two-Layer Model for Terrestrial Surface Heat Flux Retrieval.” Sensors 8 (10): 6165–6187. doi:10.3390/s8106165.
Appendix A: Calculation of relative soil moisture based on Ts−Fv space.
Relative soil moisture index (SMI) is calculated as Equation (EquationA1) and determined from Ts−Fv space as shown in . In this Ts−Fv space, the dry edge is determined based on the energy balance equation (Zhang et al. Citation2008). Sun et al. (Citation2012) showed that the new dry edge could monitor soil moisture better than the traditional dry edge, which is fitted by scatterplots.
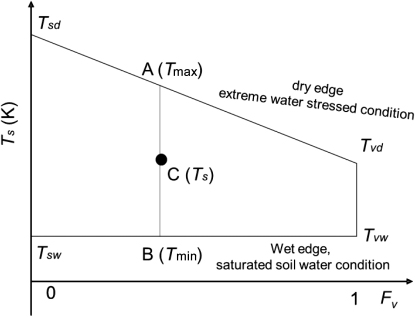
We adopt an empirical method proposed by Zaksek and Schroedter-Homscheidt (Citation2009) to estimate instantaneous Ta:
For αsd and αvd, we select the maximum albedo from the bare soil and full cover vegetation, corresponding to soil and vegetation dry conditions, respectively.
Assuming that there is not much variation for S0 on a regional scale (Mueller et al. Citation2004) for a clear sky, the averaged value of measured S0 is adopted as the input. The location of the theoretical dry edge is not sensitive to ea (Sun et al. Citation2012); thus, the averaged value of ea is also adopted as the input.