Abstract
Global Land Surface Satellite-downward surface shortwave radiation (GLASS-DSSR) products have been routinely produced from 2008–2010 based on an improved look-up table algorithm, which explicitly accounts for the variations of cloud optical depth, water vapor content, and elevation. In this study, we validated and assessed the accuracy of these products in arid and semiarid regions of China. Toward this goal, observation data-sets provided by the Arid and Semiarid Region Collaborative Observation Project as well as four other metrological sites were collected, chosen, and preprocessed for the final validation. Due to the possible effect of spatial collocation and the strong adjacency pixel effect in instantaneous products, we used a more sophisticated validating scheme in order to reduce the impacts from these effects as much as possible. Evidences indicate that the GLASS-DSSR products are considerably accurate over most parts of arid and semiarid regions in China, but in complex terrain areas the products might need further refinements. The R2 at all sites (except Naqu) was larger than 0.8 with a root mean square error (RMSE) range of about in 90–130 W/m2. Linear regression analyses suggest that GLASS-DSSR products tend to overestimate DSSR in the interval of low surface-measured values and symmetrically underestimate DSSR in the interval of high values. This systematic error may result from inappropriate assumptions about clouds and aerosol loadings over the regions in the operational algorithm.
1. Introduction
Accurate knowledge of the distribution of downward surface shortwave radiation (DSSR) is of particular importance for understanding climate processes and validating climate models. Due to the limited and inhomogeneous coverage of meteorological sites, retrieving DSSR from satellites is the only feasible way to obtain this information at regional and even global scales (Deneke et al. Citation2005).
As a basic land variable, DSSR constitutes the principal forcing that drives the energy and water exchange process and is needed by almost all land process models (Liang et al. Citation2010). Over the past few decades, a number of algorithms have been developed to obtain DSSR, and several reviews have also been given by Schmetz (Citation1989), Pinker, Frouin, and Li (Citation1995), and Liang et al. (Citation2010). Starting from the simple statistical approaches to link the observed reflectance at the top of the atmosphere (TOA) and the irradiance at the surface, currently many algorithms have become increasingly complicated and more physical processes of atmospheric radiative transfer are imported (Deneke et al. Citation2005; Liang et al. Citation2006; Deneke, Feijt, and Roebeling Citation2008; Huang et al. Citation2011). The algorithm adopted by Global Land Surface Satellite-downward surface shortwave radiation (GLASS-DSSR) products was also developed based on calculations of comprehensive radiative transfer modeling (Zhang et al. Citation2012). The algorithm potentially estimates cloud optical depth with narrowband geostationary meteorological satellite data, and subsequently calculates broadband atmospheric transmittance. In similar algorithms, the most critical part is how to design the most appropriate cloud field to acquire the relationship between TOA reflectance and atmospheric transmittance. However, natural cloud fields are extremely complicated and protean, and it is often unclear whether the ideal cloud field described by the radiative transfer model, which is used to link the TOA reflectance and atmospheric transmittance, is representative and appropriate for a specific climatic zone. Therefore, there is no guarantee that these more physical algorithms can perform better than the algorithms based on empirical relationships. Furthermore, to a certain extent, errors originating from the incomplete knowledge of the required inputs (especially information on clouds and aerosols) also constrain the physical algorithms in producing higher quality retrievals in actual operation.
At the same time, the validation of satellite-based instantaneous DSSR is another intricate problem. Unlike other land remote sensing products, such as surface temperature, due to the height of cloud tops and oblique solar light paths and satellite viewing angles, here it is not a simple point-area mismatch problem but a more troublesome three-dimensional (3D) problem. First, the effect of spatial collocation may be produced, and the shadow pixels influenced by certain cloud scenes would be misallocated into ‘apparent pixels’ (see for detailed explanations). Second, complex radiation field caused by the 3D structure of clouds would result in a strong adjacency pixel effect, especially for products with higher spatial resolution, and subsequently the independent pixel approximation (IPA), which most algorithms are based on, would be totally violated. The adjacency pixel effect ultimately leads to radiative smoothing and also makes satellite retrieved radiation products with higher spatial resolution (less than 1 km) lose their meaning (Wyser et al. Citation2002). Therefore, in order to reduce the impacts from the aforementioned effects, in this study we adopted a more sophisticated scheme to validate and assess GLASS-DSSR products based on the conclusions drawn by Wyser et al. (Citation2002; Wyser, O'Hirok, and Gautier Citation2005), Deneke et al. (Citation2005; Deneke, Knap, and Simmer Citation2009). Of course, we do not try to quantitatively solve these problems, but give some insights on potential difficulties and more or less reduce these impacts in the process of validating GLASS-DSSR products. In addition, it should be noted that for surface shortwave radiation products with longer periods, such as daily, monthly, or yearly, the above problems would be reduced automatically. The accuracy of products in these time scales are very high [e.g. the World Climate Research Program aims for an accuracy of 10 W/m2 for monthly mean radiation (Suttles and Ohring Citation1986)]; while for instantaneous irradiance, generally the root mean square errors (RMSEs) are 60–150 W/m2. It is very doubtful that the higher accuracy occasionally reported in the literature would be attained.
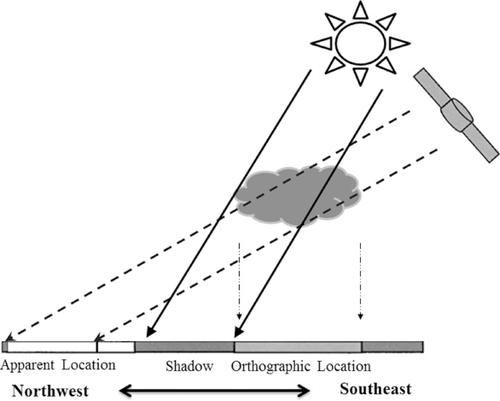
The outline of this paper is as follows. In Section 2, the GLASS-DSSR products as well as the algorithm and meteorological data we used are simply summarized. In Section 3, we describe in detail the validating scheme with Daxing site as an example. Section 4 gives the overall accuracy evaluation and individual accuracy at each site. A summary and a brief discussion are presented in Section 5.
2. Data
2.1. GLASS-DSSR products and algorithm
GLASS-DSSR products were produced using an improved lookup table algorithm developed by simulating atmospheric radiative transfer processes with Moderate Resolution Atmospheric Transmission (MODTRAN) software. In calculations, the aerosol is represented by the built-in MODTRAN rural aerosol type, and the built-in MODTRAN altostratus cloud type is considered as the ideal cloud field. In order to obtain varied cloud optical depth, cloud particle vertical extinction at 0.55 µm was changed in MODTRAN. Meanwhile, Moderate Resolution Imaging Spectroradiometer (MODIS) near-infrared precipitable water column products were utilized to perform water vapor correction. For different kinds of sensors, MODIS aboard polar-orbiting satellites and various imagers on different geostationary satellites, DSSR values were first produced separately, and then a simple fusion method was designed to combine them to obtain global surface shortwave irradiance (see Zhang et al. Citation2012 for details). At present, instantaneous global DSSR products have been completed and three-hour average global DSSR products are being urgently produced. Specific to our validation, Japan new generation of geostationary meteorology satellite, the Multifunctional Transport Satellite (MTSAT) sitting in geostationary orbit at 140°E or 145°E, was exploited to calculate DSSR over China. The spatial resolution of the DSSR products is approximately 5 km.
2.2. Pyranometer data
There are two kinds of data sources in the pyranometer data we collected. One category of data originates from the measured data-sets of 18 meteorological sites in the summer of 2008 and/or 2009 provided by the Arid and Semi-arid Region Collaborative Observation Project (hereafter termed as ASRCOP data-sets). For the 18 meteorological sites, all the instruments were collectively calibrated by CRCOP in June 2008. Nevertheless, the measurements at site of Zhangye and Xinglong still seemed to be questionable. Meanwhile, at Linze and another three sites, the measured data were very scarce and discontinuous. Therefore, these six sites would be excluded and only the data from the retained 12 sites were utilized. Another category of data stems from the complete data-sets throughout 2008 from sites at Miyun, Yingke, A'rou, and Naqu. At these four sites, the surface shortwave irradiance was all measured with second-class pyranometers (most were CMP3 developed by Kipp & Zone Corporation) and the expected daily accuracy was within 5%. Observed data at the aforementioned sites (total 16 sites) were recorded with a temporal resolution of 10 or 30 minutes. After a series of simple processes (as mentioned in Section 3), they were used in the following validation. The detailed information on these sites can be found in .
Table 1. Basic summary of the meteorological sites used to validate GLASS-DSSR products.
3. Validation approach
The philosophy why effect of spatial collocation emerges in instantaneous satellite-based shortwave irradiance products can be explained by . To a certain extent, it is relevant to the viewing characteristics of satellite sensors. In the presence of clouds, the mismatch will be inevitably produced if the observations are imaged from a TOA location. It is expected that this effect is relatively strong in GLASS-DSSR products over China due to the large viewing zenith angles of MTSAT. MTSAT sits at E140° or E145° (MTSAT1R is E140° and MTSAT2R is E145°) above the Equator and China is far from the nadir. Therefore, over China, especially western China, the observing paths incline severely. In the following section, we use the Daxing site as an example to analyze the effect of spatial collocation.
According to the research of Li et al. (Citation1993), the relationship of TOA reflectance and atmospheric transmittance is approximately linear and highly anticorrelated with the stable surface reflectance. If a linear model is used to relate the two quantities, the determination coefficient (R 2) represents the explained variance proportion. Perfect inversion is based on that satellite observed TOA reflectance can completely explain the fluctuation of atmospheric transmittance, with the determination coefficient reaching 1.0. Therefore, we can use the determination coefficient of TOA reflectance and atmospheric transmittance to quantify the effect of spatial collocation. By means of the multiresolution analysis on the collocated time series of TOA reflectance and atmospheric transmittance, Deneke, Knap, and Simmer (Citation2009) recommended using 40- to 80-minute measurements to assess instantaneous satellite products because the periods in this range can remove high-frequency variations in atmospheric transmittance and result in a better agreement between satellite TOA reflectance and atmospheric transmittance time series. Here data from each collected site were preprocessed into 40- or 60-minute mean irradiance depending on the original recording type of 10 or 30 minutes, and subsequently atmospheric transmittance series were calculated. In order to make sure, the surface optical properties did not change dramatically, the data at every site were divided into different groups according to the seasons (that is, three-month period was considered as a validating cell), and every group would be analyzed and validated separately following the same procedures. Meanwhile, cases with a solar zenith angle exceeding 80° were excluded because at low solar elevations large errors were expected for both the radiative modeling and measurements. Only the processed data were used in the following validation. Additionally, the necessary TOA reflectance series were extracted from original MTSAT images.
gives the determination coefficient contour/intensity plot centered around the site of Daxing. It was calculated based on the two time series of MTSAT TOA reflectance and atmospheric transmittance derived from ground pyranometer data. As expected, the pixel with the maximum explained variance appeared in the northwestern direction of the ground site. This finding is approximately consistent with the conclusions of Deneke, Knap, and Simmer (Citation2009), Greuell and Roebeling (Citation2009), and Schutgens and Roebeling (Citation2009), but they have some slight differences in direction and amplitude. This can be explained by a different satellite-surface relative positional relationship. Compared with the observations of the METeorological SATellite (METEOSAT)-Spinning Enhanced Visible and Infrared Imager (SEVIRI) satellite imager over Western Europe (discussed in the three aforementioned studies), MTSAT images over China contain larger longitude differences spacing between satellite and surface targets. Consequently, the pixel with maximum explained variance inclines toward not only the north but also the west, relative to the referenced ground site. The magnitude of three pixels shifting from observing site seems to be so large that an approximately 3-km cloud top height of Altostratus cloud in MODTRAN does not explain the cause. In actual validation, the shift of two pixels to the north and one pixel to the west was deemed ‘the most appropriate validating pixel’. Similar contour/intensity plots can also be seen at the other sites, except Maqu and Naqu. At these two sites, the contour/intensity plots were complicated and in disorder. This might be because of nonnegligible elevation changes in their vicinity.
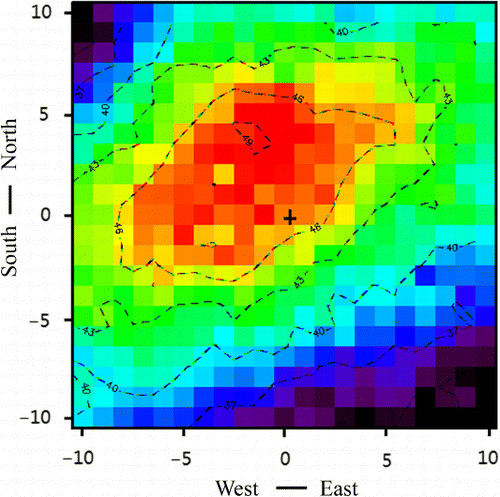
The adjacency pixel effect originates from the inhomogeneous cloud fields. The scattering from cloud particles removes part of the radiation from an atmospheric column and distributes it to neighboring pixels, and subsequently affects the distribution of radiation in a region larger than the resolution of an individual pixel. If a natural cloud field is not homogeneous (always so), the IPA is no longer justified and a more complex surface radiative field is produced. Wyser et al. (Citation2002; Wyser, O'Hirok, and Gautier Citation2005) suggested the adjacency pixel effect results in the enhancement of clear-sky irradiance close to cloud edges and radiative smoothing in cloudy pixels. We adopted their method through averaging and optimizing the size of superpixel to remove adjacency pixel effects as much as possible. According to the above discussions, we analyzed the relationship of atmospheric transmittance and TOA reflectance of 1×1, 3×3, 5×5, 7×7, and 9×9 pixels windows (centered around ‘the most appropriate validating pixel’), and found the corresponding determination coefficient would increase at the beginning, and then rapidly toward stability (still using the site of Daxing as an example, the range of R 2 was 0.48–0.59). The proper windows were 7×7 and 5×5 pixels. Here we selected the 5×5 pixels window for validating GLASS-DSSR products. That is, in this 5×5 pixels window, the determination coefficient for every pixel was deemed the weight to calculate the weighted DSSR. And finally the weighted DSSR was compared with preprocessed surface measured data.
Lastly, it should be kept in mind that, in essence, both of the above effects need to be corrected and removed in instantaneous satellite radiation products not in validation since these problems result from the restriction of the current 1D theory framework. However, since the heights as well as 3D structures of clouds are difficult to obtain in actual operations, it is very challenging and nearly impossible to completely and finely resolve these problems.
4. Validation results
Using the approach described in the above section and the observation data-sets introduced in Section 2, we validated the accuracy of GLASS-DSSR products and evaluated whether they could be used in a scientific study or application relating to understanding of a geophysical quantity or process in arid and semiarid regions of China.
4.1. Validating GLASS-DSSR products with ASRCOP data-sets
The frequency scatterplot in shows the overall comparison of GLASS-DSSR products and the 12 ASRCOP sites’ data-sets. The color of each ordered pair (measured DSSR vs. GLASS-DSSR, with 10×10 W/m2 increment) represents the number in such matchups. Each axis ranges from 0 to 1200 W/m2. The dashed and solid lines are the linear regression and 1–1 line, respectively, and some statistics are also given in the figure. A small magnitude of bias indicates the overall systematic deviation is negligible. GLASS-DSSR products slightly underestimating the surface shortwave irradiance could possibly be caused by inappropriate assumptions about aerosol information as well as pollution from some sites, such as Maqu. Nevertheless, it is clear that GLASS-DSSR products overestimate DSSR at a low-value range and underestimate DSSR at a high-value range (in view of the results at the following individual sites, it is nearly true at every site).
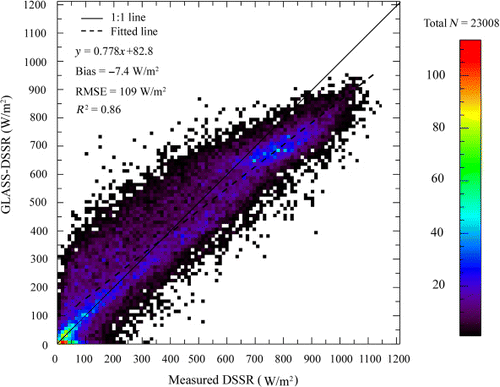
describes the validation results at six sites in temperate continental climate and the first part of lists the corresponding statistics. GLASS-DSSR products show a very high accuracy at the Neiman site, while the performance is relatively disappointing in Yuzhong and Dingxi. At an interval larger than 700 W/m2, which generally indicates that skies are clear, a very obvious underestimation was found nearly at every site. On the contrary, at an interval less than 200 W/m2, where skies are very likely to be cloudy, overestimation was seen. In order to draw a more detailed conclusion, we further distinguished the clear and cloudy conditions by comparing the clearness index (the ratio between surface incident solar irradiance and extraterrestrial solar irradiance) and the atmospheric transmittances of clear and cloudy skies. When the clearness index is larger than the atmospheric transmittance of clear sky (the aerosol optical depth at 0.55 µm is assumed into 0.32), a clear case is indicated, whereas when the clearness index is less than the atmospheric transmittance of cloudy sky (the cloud optical depth at 0.55 µm is assumed into 6), a cloudy case is implied. Then, most clear conditions and cloudy conditions can be extracted by analyzing the in situ measurements. As a result, about 40% of the validation results at these sites are grouped into clear conditions, 32% of the validation results are separated into cloudy conditions, and the remaining ones are considered as ‘undetermined’ cases. For the clear conditions, the DSSR is underestimated by ~15% on average by GLASS-DSSR products (the corresponding bias is −88.5W/m2), whereas for cloudy conditions the DSSR is averagely overestimated by ~48% by GLASS-DSSR products (the corresponding bias is −94.1W/m2). Therefore, we can conclude that there are systematic underestimations under clear conditions and overestimations under cloudy conditions in GLASS-DSSR products in summer. In the operational algorithm of GLASS-DSSR, aerosol optical depth is always regarded as a fixed default value (the surface meteorological range is set into 23 km, approximately the aerosol optical depth at 0.55 µm corresponds to 0.32). Obviously here this fixed value was set too large to represent the actual aerosol conditions in this region. This might be one of the main reasons that GLASS-DSSR products have consistent underestimations on clear days. As for the systematic overestimations on cloudy days, the inappropriate cloud field could be the most important factor. It seems that altostratus clouds in MODTRAN are not very suitable or representative for this region.
Table 2. Statistics of the comparison between GLASS DSSR and observed DSSR at individual site during summer of 2008 and/or 2009.
compares results at the six other sites. The first five sites are in temperate monsoon climate, and the last one is in plateau cold climate. The related statistical summaries can be found in the second and third parts of . Compared to the aforementioned six sites in temperate continental climate, the results of the five sites in monsoon climate are clearly better; only at Daxing were a number of cases in the interval of low values obviously overestimated. The accuracy at Maqu was worse than that of any other site. Not only were there a lot of underestimated cases, but there was also more scattering in the plot. Therefore, overall, the accuracy of GLASS-DSSR products dropped dramatically with increasing elevation. Moreover, in validation we also found a few excessively high measurements in surface observation data (the corresponding atmospheric transmittance was even larger than 1.0), especially for sites at high altitudes. A plausible explanation is that horizontal photon transport from inhomogeneous small-scale cloud edges significantly increased the surface incident shortwave radiation.
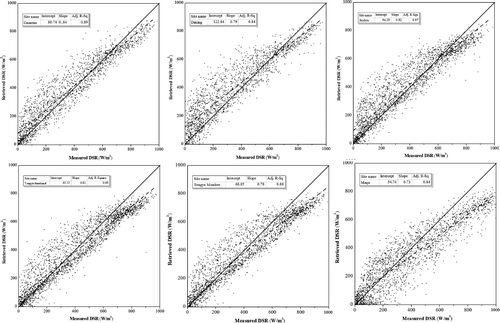
Furthermore, we also conducted a simple inter-comparison with other satellite-based downward surface shortwave irradiance products to comprehensively validate the GLASS-DSSR products. Currently, there are three available global DSSR data-sets: International Satellite Cloud Climatology Project-Flux Data (ISCCP-FD), Global Energy and Water cycle Experiment-Surface Radiation Budget (GEWEX-SRB), and Clouds and the Earth's Radiant Energy System-Monthly Gridded Radiative Fluxes and Clouds (CERES-FSW). Unfortunately, however, the corresponding DSSR products from latter two data-sets in 2008 and later years have not yet been produced or at least not provided by their websites. Therefore, we had to only use the ISCCP-FD surface radiative flux to perform the inter-comparison. The native three hourly (UTC = 0, 3 … 21) ISCCP-FD surface shortwave irradiances for all skies with 280-km spatial resolution were extracted and also the corresponding data from GLASS-DSSR products were collated again to facilitate the comparisons. At the six sites in temperate continental climate, we found that the R 2 between in situ measurements and ISCCP-FD data, and GLASS-DSSR data respectively were 0.78 and 0.87. If surface observed data were regarded as the referenced surface ‘true’ DSSR, for ISCCP-FD products the bias and RMSE respectively were −30.1 W/m2 and 130.7 W/m2, whereas for GLASS-DSSR products the bias and RMSE respectively dropped to −11.4 W/m2 and 107.4 W/m2. At the five sites in temperate monsoon climate, we saw that the R 2, bias and RMSE between ISCCP-FD data and in situ measurements were 0.85, −35.6 W/m2 and 102.9 W/m2, respectively. Correspondingly, the R 2, bias, and RMSE between ISCCP-FD data and in situ measurements were 0.87, 3.7 W/m2, and 88.8 W/m2, respectively. Likewise, the inter-comparison was conducted at Maqu in Plateau cold climate, too. For ISCCP-FD products the R 2, bias, and RMSE, respectively, were 0.77, −65.7 W/m2 and 155.7 W/m2, meanwhile for GLASS-DSSR products the R 2, bias, and RMSE, respectively, were 0.86, −67.2 W/m2, and 135.5 W/m2. More intuitive visual inter-comparisons can be found in . These simple inter-comparisons at these sites all indicate that the GLASS-DSSR products clearly outperform ISCCP-FD products. In view of its low spatiotemporal resolution, the DSSR from ISCCP-FD products are not a very appropriate choice for many applications (such as estimating regional evapotranspiration) over these regions and GLASS-DSSR products can act as a strong substitute.
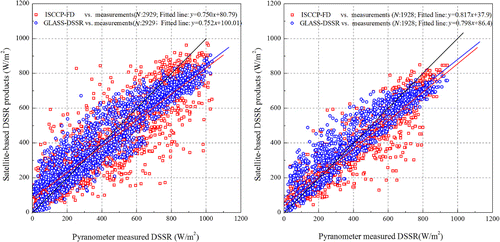
4.2. Assessing GLASS-DSSR products using one-year measured data
Because only summer observation data are provided by ASRCOP, the above comparison at any site cannot be considered a comprehensive and full validation. Numerous other studies have reported that the retrieval quality in a summer half year and a winter half year might be clearly distinct (Deneke, Knap, and Simmer Citation2009; Huang et al. Citation2011). Therefore, we collected one-year data from four other sites to completely evaluate the GLASS-DSSR products again.
The same method was implemented to assess GLASS-DSSR products at these sites. describes the histograms of the residuals’ distributions at the four sites. The statistical information and linear regression analyses are tabulated in . It was found that the retrieval qualities at Miyun and Yingke were high, A'rou was worse, and Naqu was poorest. Obviously a similar conclusion was again reached, that the accuracy of GLASS-DSSR products dramatically worsens with increasing altitude. Accumulative percentiles of relative errors in show that for Miyun and Yingke about 80% of relative errors were less than 40%, for A'rou it dropped to 74%, and for Naqu it was only 70%. More than half of the relative errors at Miyun and Yingke fell into the interval of 0–15%, also confirming that GLASS-DSSR products at these two sites are very accurate. describes a similar frequency scatterplot using the validation results from Miyun and Yingke. Comparing these results to , we find that the two kinds of results are basically consistent. That is, the accuracy of a whole year of GLASS-DSSR products does not significantly differ from the accuracy of summer. Therefore, the conclusions in Section 4.2 can all be further confirmed here.
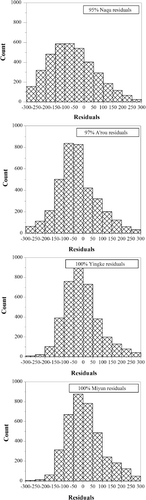
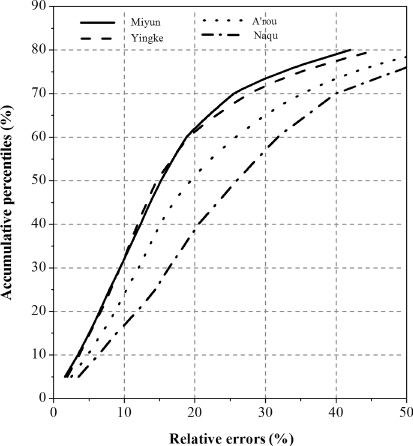
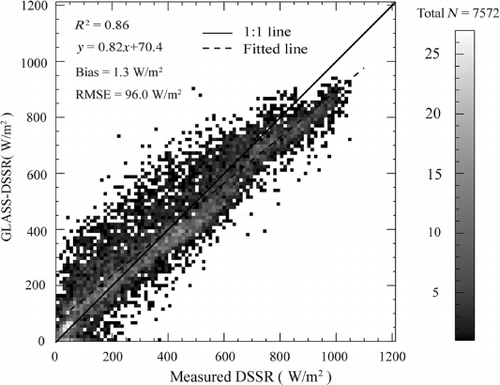
Table 3. Assessment of GLASS instantaneous DSSR using 2008 measurements at Miyun, Yingke A'rou, and Naqu sites.
5. Summary and discussion
Because instantaneous surface shortwave irradiance includes uncertainties caused by cloud inhomogeneity and partial cover, a significant part of discrepancies between surface observed and retrieved irradiance cannot be attributed to the shortcomings of the algorithm. Therefore, we adopted a more sophisticated validating scheme to more or less reduce the impacts from the spatial collocation effect and adjacency pixel effect. Our primary purpose was to validate and assess the application of GLASS-DSSR products in arid and semiarid regions of China, and provide feedback to the algorithm developing team to further refine the algorithm of GLASS-DSSR products. For this purpose, validations were carried out first using the summer data-sets provided by the ASRCOP, and then for a more comprehensive evaluation, a whole year of 2008 measured data from sites at Miyun, Yingke, A'rou, and Naqu were used. Through these validations, we can draw the following conclusions.
In the arid and semiarid regions of China, the GLASS-DSSR products are considerably accurate over most parts. From some statistical summaries reported by other researchers (Gui et al. Citation2010), overall the GLASS-DSSR products have roughly the same accuracy as GEWEX-SRB data and ISCCP-FD data, and higher accuracy than CERES-FSW data. But our preliminary inter-comparisons with ISCCP-FD data showed that the accuracy of GLASS-DSSR was obviously better than that of ISCCP-FD DSSR products in these regions. Considering their lower temporal and spatial resolution (>3 hours and > 1°), GLASS-DSSR products may be more promising for the applications in some regions. Consequently, the GLASS-DSSR products can be used in most scientific studies and applications in these regions, such as hydrological process simulation and estimation of surface evapotranspiration (Zhou et al. Citation2011; Jia et al. Citation2012).
In validations, a notable feature is that the accuracy of GLASS-DSSR products drops with increasing elevation. It is not a novel characteristic because numerous studies have reported and confirmed the same phenomenon. This problem also appears in other satellite radiation data-sets, such as GEWEX-SRB, ISCCP-FD as well as CERES-FSW. Therefore, GLASS-DSSR products give a relatively low accuracy in the Tibetan Plateau. The results at the sites of Maqu, Naqu, and even A'rou, were evidently worse than other sites. Nevertheless, GLASS-DSSR products can still be considered as the quality-guaranteed products because other satellite radiation data-sets are very likely to perform worse on the Tibetan Plateau (Yang et al. Citation2006; Yang et al. Citation2008; Gui et al. Citation2010).
The linear regression analysis showed that at nearly every site the intercept was positive and the slope was less than 1.0. This means GLASS-DSSR products tend to overestimate DSSR in conditions of low measurements and underestimate DSSR in conditions of high measurements. Further analysis points out there may be systematic underestimations under clear skies and overestimations under cloudy skies. This feature is relevant to the settings of aerosol and cloud optical properties in the algorithm. The default aerosol optical depth in the algorithm of GLASS-DSSR products seems to be excessively larger than the true conditions in arid and semi-arid regions of China. Besides this, using altostratus clouds in MODTRAN is also not very appropriate in this region. The cloud base height of 2.4 km for altostratus clouds in MODTRAN is not very suitable for most regions of China. From the sensitivity analysis of Van Laake and Sanchez-Azofeifa (Citation2004), we know that the influence of cloud height on surface shortwave irradiance is approximately as strong as aerosol loading. Therefore, a more representative cloud type is necessary to be imported over this region in the future. We expect these problems will be taken seriously and partly addressed in the future second-generation algorithm.
Finally we want to point out that the accuracy of GLASS-DSSR products in different climate regions is different. The accuracy in temperate monsoon climate in China is slightly higher than in temperate continental climate, and much better than in plateau cold climate.
Acknowledgments
The authors would like to thank the Arid and Semi-arid Region Collaborative Observation Project (ASRCOP) and Watershed Allied Telemetry Experimental Research (WATER) program, which provided in situ measurements for our validation. This work was supported by Key Project of National Natural Science Foundation of China (grant 91125002), National Natural Science Foundation of China (grant 41101389), and Chinese Academy of Sciences Action Plan for West Development Program (grant KZCX2-XB3-15-4).
References
- Deneke, H. M., A. J. Feijt, and R. A. Roebeling. 2008. “Estimating Surface Solar Irradiance from METEOSAT SEVIRI-Derived Cloud Properties.” Remote Sensing of Environment 112 (6): 3131–3141. doi:10.1016/j.rse.2008.03.012.
- Deneke, H., A. Feijt, A. van Lammeren, and C. Simmer. 2005. “Validation of a Physical Retrieval Scheme of Solar Surface Irradiances from Narrowband Satellite Radiances.” Journal of Applied Meteorology 44 (9): 1453–1466. doi:10.1175/JAM2290.1.
- Deneke, H. M., W. H. Knap, and C. Simmer. 2009. “Multiresolution Analysis of the Temporal Variance and Correlation of Transmittance and Reflectance of an Atmospheric Column.” Journal of Geophysical Research Atmospheres 114 (D17): D17206. doi:10.1029/2008JD011680.
- Greuell, W., and R. A. Roebeling. 2009. “Toward a Standard Procedure for Validation of Satellite-Derived Cloud Liquid Water Path: A Study with SEVIRI Data.” Journal of Applied Meteorology and Climatology 48 (8): 1575–1590. doi:10.1175/2009JAMC2112.1.
- Gui, S., S. Liang, K. Wang, L. Li, and X. Zhang. 2010. “Assessment of Three Satellite-Estimated Land Surface Downwelling Shortwave Irradiance Data Sets.” IEEE Geoscience and Remote Sensing Letters 7 (4): 776–780. doi:10.1109/LGRS.2010.2048196.
- Huang, G., M. Ma, S. Liang, S. Liu, and X. Li. 2011. “A Lut-Based Approach to Estimate Surface Solar Irradiance by Combining MODIS and MTSAT Data.” Journal of Geophysical Research Atmospheres 116 (D22): D22201. doi:10.1029/2011JD016120.
- Jia, Z., S. Liu, Z. Xu, Y. Chen, and M. Zhu. 2012. “Validation of Remotely Sensed Evapotranspiration over the Hai River Basin, China.” Journal of Geophysical Research Atmospheres 117 (D13): D13113. doi:10.1029/2011JD017037.
- Li, Z., H. G. Leighton, K. Masuda, and T. Takashima. 1993. “Estimation of SW Flux Absorbed at the Surface from TOA Reflected Flux.” Journal of Climate 6 (2): 317–330. doi:10.1175/1520-0442(1993)006<0317:EOSFAA>2.0.CO;2.
- Liang, S., K. Wang, X. Zhang, and M. Wild. 2010. “Review on Estimation of Land Surface Radiation and Energy Budgets from Ground Measurement, Remote Sensing and Model Simulations.” IEEE Journal of Selected Topics in Applied Earth Observations and Remote Sensing 3 (3): 225–240. doi:10.1109/JSTARS.2010.2048556.
- Liang, S., T. Zheng, R. Liu, H. Fang, S.-C. Tsay, and S. Running. 2006. “Estimation of Incident Photosynthetically Active Radiation from Moderate Resolution Imaging Spectrometer Data.” Journal of Geophysical Research Atmospheres 111 (D15): D15208. doi:10.1029/2005JD006730.
- Pinker, R. T., R. Frouin, and Z. Li. 1995. “A Review of Satellite Methods to Derive Surface Shortwave Irradiance.” Remote Sensing of Environment 51 (1): 108–124. doi:10.1016/0034-4257(94)00069-Y.
- Schmetz, J. 1989. “Towards a Surface Radiation Climatology: Retrieval of Downward Irradiances from Satellites.” Atmospheric Research 23 (3–4): 287–321. doi:10.1016/0169-8095(89)90023-9.
- Schutgens, N. A. J., and R. A. Roebeling. 2009. “Validating the Validation: The Influence of Liquid Water Distribution in Clouds on the Intercomparison of Satellite and Surface Observations.” Journal of Atmospheric and Oceanic Technology 26 (8): 1457–1474. doi:10.1175/2009JTECHA1226.1.
- Suttles, J., and G. Ohring. 1986. Report of the Workshop on Surface Radiation Budget for Climate Applications. Columbia, MA: WCRP WC119, World Meteorological Organization Technique Document.
- Van Laake, P. E., and G. A. Sanchez-Azofeifa. 2004. “Simplified Atmospheric Radiative Transfer Modelling for Estimating Incident PAR Using MODIS Atmosphere Products.” Remote Sensing of Environment 91 (1): 98–113. doi:10.1016/j.rse.2004.03.002.
- Wyser, K., W. O'Hirok, and C. Gautier. 2005. “A Simple Method for Removing 3-D Radiative Effects in Satellite Retrievals of Surface Irradiance.” Remote Sensing of Environment 94 (3): 335–342. doi:10.1016/j.rse.2004.10.003.
- Wyser, K., W. O'Hirok, C. Gautier, and C. Jones. 2002. “Remote Sensing of Surface Solar Irradiance with Corrections for 3-D Cloud Effects.” Remote Sensing of Environment 80 (2): 272–284. doi:10.1016/S0034-4257(01)00309-1.
- Yang, K., T. Koike, P. Stackhouse, C. Mikovitz, and S. J. Cox. 2006. “An Assessment of Satellite Surface Radiation Products for Highlands with Tibet Instrumental Data.” Geophysical Research Letters 33 (22): L22403. doi:10.1029/2006GL027640.
- Yang, K., R. T. Pinker, Y. Ma, T. Koike, M. M. Wonsick, S. J. Cox, Y. Zhang, and P. Stackhouse. 2008. “Evaluation of Satellite Estimates of Downward Shortwave Radiation over the Tibetan Plateau.” Journal of Geophysical Research-Atmospheres 113 (D17): D17204. doi:10.1029/2007JD009736.
- Zhang, X., S. Liang, G. Zhou, H. Wu, and X. Zhao. 2012. “Mapping Global Incident Downward Shortwave Radiation and Photosynthetically Active Radiation over Land Surfaces Using Multiple Satellite Data.” Remote Sensing Environment.
- Zhou, J., B. X. Hu, G. Cheng, G. Wang, and X. Li. 2011. “Development of a Three-Dimensional Watershed Modelling System for Water Cycle in the Middle Part of the Heihe Rivershed, in the West of China.” Hydrological Processes 25 (12): 1964–1978. doi:10.1002/hyp.7952.