Abstract
Implementation of land management policies influences land use and hence causes environmental change. Taking the Ordos rangelands in China as a case study, this paper explores the potential of remote sensing to assess in dryland areas the impacts of policies on the environment. Thirteen Landsat images of the period 1978–2010 were acquired and those corresponding to the starting dates of implementation of different policies were selected for land-cover change analysis; others were used to check the detected change and track the normalized difference vegetation index (NDVI) trajectory matched with time series of meteorological data for calibration of natural response of rangelands to rainfall. The results indicate that policy impacts are complex and include both positive and negative aspects depending on the locality in space. On one hand, policies have aroused the enthusiasm of people in agricultural production and sand-control leading to the recovery of about 2618 km2 of desertified rangeland and sandy land, and economic growth, on the other hand, provoked vegetation degradation with an accumulated area of 2439 km2 when policies cannot reconcile the conflict between environmental protection and the interest of rural people. However, degradation is not absolute and can be mitigated by the implementation of rational policies.
1. Introduction
In recent decades, remote sensing has been widely applied in land-cover change and land condition assessments, land degradation and desertification studies in the dry areas (Hellden Citation1988; Lambin and Strahlers Citation1994; Diouf and Lambin Citation2001; Runnstrom Citation2003; Li et al. Citation2004; Wu Citation2003a, Citation2003b; Wessels et al. Citation2007; Hill et al. Citation2008; Zhou, Li, and Kurban Citation2008; Wu Citation2009; Del Barrio et al. Citation2010), and a number of publications have dealt with the linkages between environmental change and human activity (e.g. Geoghegan et al. Citation1998; Mertens and Lambin Citation2000; Veldkamp et al. Citation2001; Verburg et al. Citation2002; Wu, Lambin, and Courel Citation2002; Lambin, Geist, and Lepers Citation2003; Geist and Lambin Citation2004; Aspinall Citation2004; Xie et al. Citation2005). Yet, few studies have focused specifically on the cause/effect relationships between the observed changes in land use/cover and land management policies probably because of the intricate interlinkage between policies and the difficulty to distinguish and quantify the effect of one from the others. In fact, the implementation of policies has been increasingly recognized as one of the major drivers of land surface change since they can directly or indirectly influence the land resources exploitation, creation of markets, and economic opportunities. Responding to land use policies, the new socioeconomic activities may lead to further environmental change and new policy-making. Therefore, the analysis of policy impact is important and essential not only for the policy-makers but also for scientists. It allows them to better understand the role of policies in socioecological systems and to work out better strategies for sustainable land use and mitigation of land degradation in dryland ecosystems.
In the Ordos region, as in other provinces of China, different national and local land management policies have been implemented, especially in recent decades. This provides us with an opportunity to analyze the impacts of these policies on the environment in space and time. Although diagnostic studies of land-cover change and land degradation have been undertaken in Ordos (e.g. Jia Citation1997; Gao and Zha Citation2001; Li et al. Citation2004; Runnstrom Citation2003; Wu, Zucca, and Enne Citation2005) and Wu and De Pauw (Citation2010) have attempted to reveal the linkage between policies and land degradation, there is an urgent need to have a complete view of policy impacts on the Ordos rangeland environment in order to provide useful reference for promulgation of more rational and sustainable land management policies in future.
Hence, the main objective of this case study in Ordos is to assess how remote sensing technology can assist land use planning by analyzing the impacts and implementation effectiveness of the past land management policies on the environment. This will be achieved by matching time series of remote sensing data with meteorological data, and socioeconomic information. Several remote sensing processing methods exist for analyzing changes in land use/land cover, such as change vector analysis, post-classification and differencing-and-thresholding techniques (Singh Citation1989; Lambin and Strahlers Citation1994; Mas Citation1999; Lillesand and Kiefer Citation2000; Wu Citation2003b; Coppin et al. Citation2004; Wu Citation2007, Citation2009). In order to be able to attribute the detected changes to human activity, temporary changes related to rainfall variations have to be taken into consideration. For this purpose, meteorological data have to be incorporated for calibration against the biophysical indicators such as NDVI (Rouse et al. Citation1973), as studied by Evans and Geerken (Citation2004) and Wessels et al. (Citation2007).
The most recent historical period, from the early 1950s to present, offers tremendous opportunities to study the effects of policy on the environment in Ordos. Since no remote sensing imagery is available for the period before the 1970s, our study is confined to the post-1973 period for which both satellite images and policy documents were available.
2. Materials and methods
2.1. The study area
2.1.1. Ordos region
The Ordos region is located in Inner Mongolia and is adjacent to the Shaanxi and Ningxia Provinces in China (). Geomorphologically it is a part of the Ordos Plateau bordering the Loess Plateau on the south. The region is mainly covered with rangelands and situated in the transitional belt between the arid grasslands and deserts. The natural vegetation cover consists mainly of shrubs, perennial herbs, and, very locally, forests (Wu, Zucca, and Enne Citation2005). The soil types are entisols (sandy soils) in sandy land and deserts, mollisols in meadows and grasslands, gleysols in depressions or marshland, fluvisols in the alluvial plain of the Yellow River in the north, brown calcareous soils and loess in the hilly-and-gully area in the east. The average annual precipitation is around 317 mm. The eastern part of the region, e.g. Yulin, Ejihoro, and Dongsheng, receives the highest annual rainfall with an average of about 335–409 mm. It gradually declines in the west and northwest, where the average annual rainfall is about 195–224 mm in Yinchuan, Shizuishan, and Linhe (see location in ). With a summer rainfall pattern, 70% of the annual rainfall is concentrated in the period July–September. Winds from the northwest blow on average 230 days per year and may exceed Beaufort Scale Force-8 (speed >17 m s−1) during more than 40 days. The concentrated rainfall and strong winds provoke soil erosion and water loss (Xu Citation2006, Citation1999). The study area, covering a territory of 17,000 km2, is located in the southwest part of the Ordos region, and more specifically, an area including a part of the two Banners namely Otog and Otog Front, and Wuhai City in Inner Mongolia, and Taole County in Ningxia ().
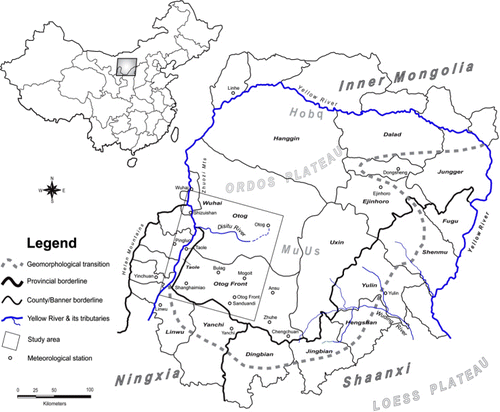
In terms of natural resource endowment, the Ordos region has abundant good-quality coal resources as well as natural gas and oil reserves. In recent years, Ordos has become one of the national energy bases under the Chinese Government's mid-to-long-term national strategy ‘To Develop the West’. Significant land use/cover change and land degradation have taken place in Ordos owing to long-time human activity in grazing, deforestation, and land reclamation for agriculture driven by a number of local and national policies (Jiang et al. Citation1995; Jia Citation1997; Chen and Gao Citation2001; Zhang and Wang Citation2001; Wu Citation2003a; Xu Citation2006).
2.1.2. Land reclamation and degradation cycles through history
According to the studies of Jiang et al. (Citation1995), Chen and Gao (Citation2001), and Zhang and Wang (Citation2001), cultivation in Ordos dates back to the late Zhou Dynasty (474–221 BC). Policy-driven land reclamation started in the Han Dynasty (206 BC–220 AD), when Emperor Wu issued an order in 120 BC ‘Migration for consolidating the border areas’. Hence, about 700,000 peasants emigrated from the interior of the country to the Ordos and Loess Plateaus for land reclamation, and at the same time consolidating the border areas (Enkhee Citation2003). Widespread forest and grasslands were hence reclaimed for agriculture. In the Tang Dynasty (618–907AD), a similar policy was implemented in the period 707–820 AD, leading to forest-clearing for construction of the frontier fortresses and land reclamation for crop production which gradually resulted in land degradation – a series of small incursions of sandy land – the initial stages of the Hobq Desert and Mu Us sandy land (Enkhee Citation2003). In the Qing Dynasty (1644–1911 AD), again under the similar policy, deforestation for the construction of temples and government houses and land reclamation for cropland took place (Zhang and Wang Citation2001). In recent decades, three peaks of land reclamation from grassland to cropland occurred again in 1955–1956, 1958–1962, and 1971–1973 under new policies such as ‘Giving the prominence to food production’ (Chen and Gao Citation2001). Owing to the low productivity of these converted rangelands (annual rainfall <250 mm), the fields were abandoned after two to three years' cultivation and became degraded by topsoil loss due to exposure to the strong winds and water erosion by concentrated rainfall. This loss of land necessitated new reclamation in the grasslands elsewhere.
After 1973, several periods can be differentiated with respect to how national and local government land policies have influenced land use in the Ordos rangelands.
2.1.2.1. Period 1 (1979–1985)
This period refers to the nationwide implementation of Deng Xiaoping's ‘Household land tenure policy’ in 1979–1984, and the promulgation of the first law concerning grassland use, the ‘Decree of grasslands’, in 1985. The main objective of the first policy was to stimulate people toward agricultural production in order to minimize the risk of famine in the country after the ‘Cultural Revolution’ (1966–1976). Deng's land tenure policy was fully implemented in all agricultural provinces, and food production was much increased in the whole country. In Ordos, an agropastoral region, this policy transferred the tenure of agricultural land and ownership of production to individual farmers, resulting in new land reclamation and boosts of food production. For example, in the Banner Otog Front, food production increased by 163% while the population grew only by 15.5% from 1980 to 1989 (Statistic Yearbook of Otog Front, 2009). On the other hand, the Grasslands Decree of 1985 kept the grasslands as a collective resource, whereas livestock was private (Erdenzhab Citation2002; Gai Citation2007).
2.1.2.2. Period 2 (1987–1992)
After the countrywide success of the agricultural reform, Deng further proposed a national ‘Open and reform policy’ and issued the ‘Decree on legalization of the private economy’ under the strategy named ‘Invigorating the domestic economy and opening to the outside world’ in 1987–1988 (In memory of Deng Xiaoping: http://cpc.people.com.cn/GB/69112/69113/69683/index.html). However, nationwide implementation of these policies was not conducted until February 1992 when Deng paid an official visit to the southern regions of the country. These governmental actions promoted the privatization of the collective or national enterprises and encouraged the development of the private economy. In response to these new policies, countless local private companies were set up in Ordos after 1992 and started to bring into the national economy local products such as coal, oil, gas, kaoline, as well as wool, and medicinal and edible wild herbs (Ephedra sinica, Glycyrrhiza uralensis, Nostoc commune var. flagelliforme). At the same time, self-organized teams and family-based companies, composed of a great number of peasants and herdsmen, started to invest in the control of land degradation and to increase the economic value of the land, with the encouragement of existing governmental agencies for combating desertification. As expected, the economy in Ordos developed rapidly in the 1990s. Taking the same example of the Banner Otog Front, the Gross Domestic Product (GDP) from 1989 to 1999 increased by 614%, and the annual income of rural people augmented by 628%. The drawback of these two policies was their exclusive focus on pursuing economic development and profit, and neglect of environmental protection. As a result, overexploitation of land resources (e.g. coal mining and oil exploitation without protection measure, overgrazing, excessive collection of medicinal, and edible herbs) lead to widespread degradation of the fragile vegetation cover.
2.1.2.3. Period 3 (2000–2001)
Aware that as the primary vocation for Ordos, the region had become to serve as one of the national energy bases due to its abundant fossil fuel and gas resources, and that earlier agricultural land use policies had led to severe land degradation, the Chinese Government issued new legislation for the area. The Central Government promulgated in 2000 a decree ‘Prohibiting the collection of the medicinal and edible herbs (e.g. licorice and ephedra) in grasslands’. In 2001, on top of this national order, the local governments of Otog and Otog Front published ‘Grazing ban and rotation policy with subsidy system’ as well as the call ‘To return cultivated land to grassland’. Government subsidies encouraged herdsmen to utilize, in the areas affected by the grazing ban, some highly productive land to cultivate aridity- and cold-resistant forage grasses, such as alfalfa (Medicago sativa) and Astragalus adsurgens pall for breeding animals and preserving large surfaces of grassland. The previously open grazing system was then converted into a system based on indoor dry-lot feeding. Other areas were designated for rotational grazing.
2.2. Data
Multi-temporal Landsat MSS, TM, ETM+ images from 1978 to 2010 were obtained (). The images of 1978, 1989, and 1999 were considered as the initial states of different policy implementation periods (marked bold in ). To represent the current state, the image of 2007 was used since the 2009 ETM+ image contains a defect due to the Scan Line Corrector (SLC-Off) problem, and there is considerable cloud cover (>33%) in the TM 2010 image. However, both images could still be used with others for projecting the NDVI trajectory of the test sites.
Table 1. Multi-temporal satellite images used in this study.
Annual rainfall data for the years 1991, 1996, 1999, and recent years 2005–2009 were collected for 16 stations within the Ordos region, especially, in the Banners of Otog and Otog Front and Yinchuan region in Ningxia from the Statistic Yearbooks of Ningxia and of the Banner Otog Front. Monthly rainfall data series of Otog and eight adjacent stations (Dongsheng, Linhe, Ejinhoro, Yanchi, Yinchuan, Yongning, Yan'an, and Yulin) from the 1960s to 2010 were acquired from the China Meteorological Data Sharing Service (see for location).
Together with the acquisition dates of the different satellite images, the implementation onset dates of different land use policies are shown in .
2.3. Approaches to discern land surface change
Generally, classification-related approaches are most frequently applied to assessing land-cover change. However, as many authors have argued, these approaches may produce huge errors unless each class is accurately classified and validated (Lambin and Strahlers Citation1994). With the advances in classification techniques, a number of successful studies have been reported (Mas Citation1999; Lillesand and Kiefer Citation2000; Wu Citation2009). Another practical approach is the differencing-and-thresholding techniques (Jensen and Toll Citation1982; Lambin and Strahlers Citation1994; Lillesand and Kiefer Citation2000; Wu Citation2003b; Coppin et al. Citation2004; Wu Citation2009), which can identify accurate changes in space and time. Therefore, these two methods have been jointly employed for land-cover change detection in this study. The processing steps include the following sections.
2.3.1. Preprocessing
The Landsat ETM+ image dated 12 August 1999 was geometrically corrected using topographic maps on the scale of 1/200,000 and GPS points obtained during the field investigation in August 2000 and June 2002 (for a total of 121 ground-control points) in the WGS84 datum and projection UTM Zone 48N using the third-order polynomial model and nearest neighborhood resampling. This image was used as a reference to correct the other images with RMS error 0.23–0.58 pixels. Some recent images (2002, 2006, 2007, 2009, and 2010) obtained from USGS are already well-georeferenced. The correction procedure was then avoided.
An image-based approach, the COST model proposed by Chavez (Citation1996), was introduced in this study to correct the additive and multiplicative atmospheric effects in the Landsat MSS, TM, and ETM+ images; the dark-object subtract (DOS) method (Chavez Citation1988) was used to determine the haze in each band.
The most frequently applied vegetation index, NDVI, was derived for land-cover change detection and trajectory analysis. It is expressed as:
The MSS image dated 1978 was resampled into a 30-m resolution using the nearest neighborhood resampling approach to have more comparability with others. Then, two MSS NDVIs from two different near infrared (NIR) bands (band 3: 0.70–0.80 µm and band 4: 0.80–1.1 µm) vs. the same red band (band 2: 0.60–0.70 µm) were produced and averaged to get the mean NDVI, which is more compatible with the NDVI of TM/ETM+ images.
2.3.2. Indicator differencing and thresholding
To distinguish a negative trend of vegetative greenness from a positive trend, a differencing was applied to the atmospherically corrected NDVI images between the periods 1978–1989, 1989–1999, and 1999–2007 followed by a thresholding technique. More specifically, the differencing procedure consists of subtracting the NDVI of the two different dates. Taking the period 1999–2007 for example, the differencing can be expressed as:
The same change detection technique was applied on the ΔNDVI for the periods 1989–1999 and 1978–1989. Some changes due to drying up of water bodies (NDVI also increases for change from water to land) were not real vegetation cover change; other changes related to agricultural rotations such as conversion from cropland to fallow land or vice versa were not the subject of this analysis. All these false change were excluded manually by mask operation.
The advantage of this change detection technique lies in the fact that it can exactly position the changes in space and reveal the variation in greenness extent, and can thus further assess the dynamics of biomass production. For this reason, the conversion of grasslands into croplands and pastures as well as grassland in rotation (fallow), can also be identified in each observation period. The disadvantage is its insensitivity to the change in very low greenness land cover where there is no significant difference in NDVI. For example, recent sand-control with very low greenness cannot be identified in the deserts.
2.3.3. Post-classification differencing
To avoid underestimation, another change detection technique ‘post-classification differencing’ (Wu Citation2009) was applied to the images of MSS 1978, TM 1989, ETM+ 1999, and TM 2007 as a complementary to the differencing-and-thresholding approach. Prior to the differencing step, a supervised classification using the Maximum Likelihood classifier was carried out in which the training areas were visually selected in the color composites using Google Earth as reference. Four major land-cover types were differentiated: sands (deserts), vegetation cover (VGT, including agricultural patches, pastures, and grasslands with low greenness), water bodies, and bare soils (including normal bare land, salty crust, or saline land occurring near rivers, lakes, and swamps). These classes were further aggregated into two major groups: sands and nonsands (VGT cover + water bodies + bare soils) after classification. The overall accuracy against the ground truth ‘regions of interest’ and kappa coefficient of each individual classification before aggregation is shown in .
Table 2. Overall accuracy of each individual classification.
Differencing on these classes at different times allowed us to assess whether a given class expanded or contracted during the study period. For example, where Sand1989–Sand1978 > 0, it was considered that the sand class had extended or previous grassland (or other nonsand areas) was covered with sands (deserts) for the given area, whereas a negative difference indicated that it had contracted or former desert was covered with vegetation (e.g. due to sand-control).
The advantage of this technique is that it can detect both subtle changes such as sand-control, desert extension as well as abrupt change (e.g. conversion from grassland into urban or croplands with significant difference in greenness). The disadvantage is that it cannot precisely quantify the change degree of the greenness.
The two approaches ‘differencing-and-thresholding’ and ‘post-classification differencing’ are complementary and their combined use leads to optimal differentiation of land-cover change and land degradation.
2.3.4. Identification of specific land-cover change types
To understand the spatial variation in land use/cover changes, density slicing on the differenced NDVIs and masking operation to the detected changes were applied to identify, against the original image composites, the specific change types in different periods such as extension of cropland and pasture, extension of coal mining area, sand-control, and vegetation degradation, etc.
2.4. Calibration with meteorological data
To calibrate the detected change, we have to first understand the natural response of ecosystem to rainfall, and then to distinguish the role of human intervention. Malo and Nicholson (Citation1990), Tucker, Dregne, and Newcomb (Citation1991), Davenport and Nicholson (Citation1993), Wang et al. (Citation2001), Wessels et al. (Citation2007), and Udelhoven et al. (Citation2009) analyzed the vegetation response to rainfall and concluded that monthly maximum NDVI is best correlated with the rainfall in the concurrent plus two antecedent months in Africa, the USA, and Spain; Celis, De Pauw, and Geerken (Citation2007) found that in the Fertile Crescent an excellent agreement between the average NDVI and a linear combination of the average annual rainfall and the minimum temperature of the coldest month; and Evans and Geerken (Citation2004) identified in Syria that the rainfall period is best correlated to the annual maximum NDVI (NDVImax) or peak NDVI (NDVIpeak) which represents the total annual green biomass production.
In light of the above knowledge, the following points were taken into consideration in our calibration:
Landsat images used for this land-cover change detection were acquired in August, corresponding to the major annual rangeland biomass production and rainfall period. Thus, the reflectance-based NDVI derived from these images is considered as the annual NDVImax, which is representative of the total green biomass (Evans and Geerken Citation2004).
The accumulated rainfall in the biomass production season (May–September) in the study area accounts for 85.3% of the total annual rainfall in the observed years. Both the growing season rainfall and annual rainfall can be used to calibrate the response of the NDVImax. We used the annual rainfall for this purpose due to the lack of monthly data from some stations.
The least-square regression model is applicable in such kind of calibration. Instead of using the residuals trends (Evans and Geerken Citation2004; Wessels et al. Citation2007) as human-induced indicator, we consider the correlation coefficient as a direct indicator. As argued before, high correlation between NDVImax and annual rainfall implies the natural response of vegetation to rainfall with little or without disturbance; however, low correlation is considered as a result of human intervention such as overgrazing or other kinds of land modification overlapping on the rainfall. In both cases, interannual variability of rainfall is already considered.
The calibration was conducted in two ways shown in the following sections.
2.4.1. Diachronous calibration
To analyze the biophysical response of ecosystem to rainfall and human intervention over time, six sites (A, B, C, D, E, and F) were selected on the basis of whether evident land-cover change had occurred or not during the three observation periods. Their location and features is shown in and .
Table 3. Features of the selected sites.
For each site, the averaged reflectance-based NDVI value of 13 years was respectively computed to compose the NDVI trajectories (a, b, c, d, e, and f in ). The annual rainfall data were spatially interpolated using the inverse distance weighting (IDW) approach to produce the annual rainfall raster layers from which the average rainfalls of each site were extracted for the observed years. The average NDVI values of each site were then plotted against the corresponding annual rainfall data () and a least-square regression analysis at the confidence interval of 95% was undertaken to reveal the relationship between NDVI trend and rainfall in these sites (a′, b′, c′, d′, e′, and f′ in ).
2.4.2. Cross-spatial analysis
This analysis was aimed to ascertain the spatial variation of the rangeland ecosystem in response to rainfall by relating the vegetation greenness (e.g. NDVI) to the rainfall distribution in space at one point of time (one year in this case). The NDVI images and their corresponding interpolated rainfall data (raster layer) of 1978, 1989, 1999, and 2007 were chosen for this purpose. In order to have spatial representativeness and quick assessment, 2000 points were randomly generated in the whole study area to cover all types of land cover. After excluding the points falling in the irrigated agricultural land and desert patches (in which the NDVI has theoretically no relationship with rainfall), 1485 points remained to which a linear least-square regression model was fitted.
3. Results and discussion
Through the above processing and analysis, the results obtained are presented here for discussion.
3.1. Diachronous calibration at test sites
The NDVI trajectory at site A () shows a decrease in the period 1978–1989, followed by an increase up to 2002. From the texture in the ETM+ images dated 1999 and 2002, it appears that site A and its surrounding area were controlled and managed zones from 1996 to 2002: the ‘network belts’ in which local people planted grasses and shrubs to combat desertification could be clearly discerned in both images. From 2004 to 2007, the NDVI value decreased again whereas annual precipitation increased. This ‘unnatural’ NDVI drop is most likely the result of human intervention, e.g. overgrazing.
Site B was selected in the natural grassland, protected and with little disturbance by human activity up to 2004, but opened for grazing after 2005/2006. Although the overall correlation coefficient between the NDVI trajectory and rainfall is low (R 2 = 0.405, ), if we consider only the observed years before 2004, the fluctuation in NDVI is well consistent with that of annual rainfall and the coefficient is rather high (R 2 = 0.592).
Site C, protected from grazing in the period 1996–2006, presents a similar pattern as Site B (). With the opening for grazing in 2007, NDVI declines, but no significant degradation was detected in this site.
Site D experienced degradation in the period 1989–1999, particularly in the years from 1996 to 1999. The NDVI trajectory also fluctuates with rainfall but the correlation is low (R 2 = 0.264). The recovery of the grasslands occurred in the period 2002–2007.
Site E was carefully selected grasslands where neither evident degradation nor conversion to pastures was observed. Hence, the NDVI trajectory and annual rainfall in site E shows the best correlation (R 2 = 0.664) among the all test sites.
Site F is similar to Site E, but some land management, e.g. conversion from grasslands into pastures in 2004 and low greenness in comparison with the rainfall in 2010 were discerned. For this reason, the correlation between the NDVI and rainfall is lower than that of Site E.
In summary, the diachronous calibration reveals that the correlation between NDVI and annual rainfall is generally weak. The R 2 values vary from 0.044 (no correlation) to 0.664 (moderately high correlation), depending on the extent of human intervention (grazing, overgrazing, conversion of rangeland into cropland, etc.). In –, the ‘unnatural’ points represent the occurrence of land use/cover change, an intervention of human activity.
3.2. Cross-spatial analysis
The linear regression analysis did not show any correlation between the NDVI and rainfall distributions at 95% confidence level in each observation year (R 2 = 0.002–0.111). This implies that in the study area the vegetation greenness, represented by NDVI, an important spatial indicator of rangeland condition (Del Barrio et al. Citation2010), cannot be explained by the distribution of rainfall only, and is therefore more influenced by land use practices, superimposed on the rainfall pattern.
3.3. Impacts of land use and rainfall on grasslands
One important result obtained through the above two calibration analyses is that the peak greenness of rangeland is a combined effect of both rainfall and human activity – land use. Without human intervention, the greenness expressed by the maximum NDVI, is positively correlated with the annual precipitation, in our case, R 2 = 0.664. However, the increased human intervention led to a lower correlation between the two factors. Hence, the NDVI–rainfall models provide a simple but effective view to assess the impacts of land use and management on rangelands.
3.4. Land-cover changes
The relevant land-cover changes and their change rates corresponding to the different policy implementation periods are shown in . In general, the rangelands in the study area have experienced two kinds of change in each period: one is vegetation greenness growth (VGT-G) and the other is vegetation degradation (VGT-D) as shown in .
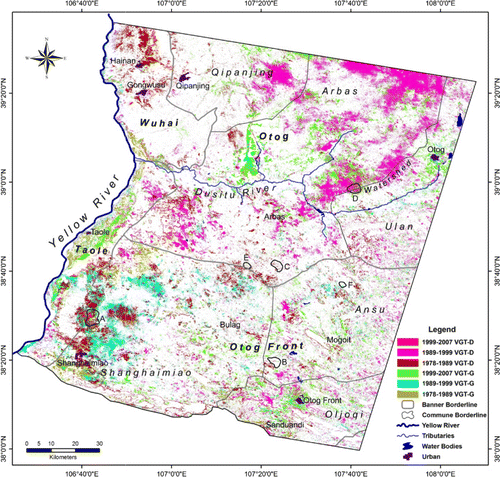
VGT-G, with a total surface area of about 2618 km2 or 15% of the total land area in the period 1978–2007, is attributed to different types of conversion of the grasslands into more productive land-cover categories, such as (1) croplands, pastures/forage land (e.g. areas planted with alfalfa) and cash crop plantation (planted with ephedra and licorice), with a total area of 557 km2 in the period 1978–2007 and irrigated by either the Yellow River (e.g. in Taole and Wuhai) and its tributary or by groundwater (e.g. in the communes of Arbas and Shanghaimiao); (2) sand-control by soil conservation methods, including afforestation (e.g. planting poplar, pine, and pinus sylvestris var. mongolica) and cultivation of drought-resistant shrubs and herbs (such as Hedysarum leave, Caragana korshinski, Artemisia sphaerocephala and Artemisia ordosica, sea-buckthorn) to combat desertification, improve land condition, and generate economic value; and (3) grasslands in fallow (in rotation without grazing) leading to a recovery of the rangelands.
VGT-D as shown in is characterized by a decrease in vegetation greenness in the rangelands due to (1) overgrazing in the permitted rotation grasslands, especially during the periods of droughts (the major degradation type); (2) overgrazing and trampling of animals around settlements and water points (secondary degradation type); (3) desert dynamics as expressed by mobile sand dunes in the western part of the study area (north of Taole, northwest of Shanghaimiao, southwest of Arbas), moving along the wind-blowing direction mainly from northwest to southeast at an annual rate of about 11–21 m depending on locations. In the southern parts of the study area this desert encroachment has been reduced or even stopped as a result of sand-control in the past decades; and (4) coal mining in Wuhai and Otog (Qipanjin) in the recent decade. These kinds of land degradation have caused the temporary loss of vegetation cover in 2439 km2 of rangelands (13% of the total land) in the period 1978–2007.
The change rates for each identified specific change in each policy implementation period are summarized in and ; the spatial extent of each specific change category is shown in .
Table 4. Land cover changes in the study area.
It was also noted that grassland degradation did not occur in the same place all the time but moved from one place to another in different periods as a result of the traditional grazing habit, and more recently linked to the implementation of the ‘Grazing ban and rotation policy’. In most cases, recovery occurred after degradation.
4. Discussion
As revealed in the above sections, about 28% of the total territory of the study area has experienced change corresponding to the implementation of different policies in the past three decades. A brief discussion on the interaction between the change in environment and policies is given below.
After the first period of new policy formulation (1979–1985), satellite images provide evidence that in the period 1978–1989, land reclamation had taken place at a rate of 7.45 km2 y−1 (see , and ), which coincided with a dramatic increase in food production (for example, 163% in the Banner Otog Front). The predominant biophysical change in this period is vegetation degradation, as a result of grazing activity in the collective grasslands in the western parts of the study area authorized by the Grasslands Decree in 1985 (see and , and ). Another factor leading to degradation is the fuel wood collection in the desert rangelands and mountainous slopes. It was reported that before the start of large-scale coal mining in Ordos in 1990s, collection of shrubs (Artemisia ordosica) as fuel wood for cooking and heating was widespread (Chen and Gao Citation2001; Wu Citation2003b). The global degradation rate during this period was estimated at about 60 km2 y−1 (see ).
During the second policy implementation period in 1990s, the local outcome of the burst in economic activity was mixed. Landsat images, dated 1989 and 1999, show an increase in NDVI (1989–1999 VGT-G in ), and indicate that some areas of degraded land and deserts in the southwest were controlled and that a considerable part was converted into croplands and cash crop plantations such as ephedra and licorice, especially in the Shanghaimiao and Arbas Communes ( and –). In the Banner Otog Front alone, food production was increased by 448.7% in the period from 1989 to 1999, which corroborates the evidence from remote sensing of the extension of cropland (see ). On the other hand, the incentives to improve family income and livelihoods and business opportunities offered by the new policy ‘Invigorating the domestic economy’, resulted in the widespread collection by local people of wild medicinal and edible herbs. This factor, combined with overgrazing and fuel wood collection during a period of rainfall reduction (e.g. 1999), can offer an explanation for the significant vegetation degradation observed by remote sensing (, , and ). Thus, the immense increase of the net income of the rural people by 628% and of the GDP by 614% from 1989 to 1999 in the Banner of Otog Front was to a large extent at the cost of grassland degradation, which was expanding at a rate of about 143 km2 y−1. In the meantime, coal mining by national and private companies started to be accelerated mainly in Wuhai and Qipanjing in the Banner of Otog ( and ).
Since 1999, thanks to the decrees ‘Prohibiting the collection of the medicinal and edible herbs in grasslands’ and the ‘Grazing ban and rotation policy with subsidy system’, the greenness in land cover was significantly increased in the whole study area (1999–2007 VGT-G in , –). The specific land management measures taken to combat degradation included rangeland recovery, pasture extension, and sand control, which were implemented at the rates of about 110 km2 y−1, 33 km2 y−1, and 52 km2 y−1, respectively. In comparison with the previous period 1989–1999, degradation has been significantly reduced, and its rate of growth was only about 14 km2 y−1. Meanwhile, from 1999 to 2009, the cattle numbers and food production did respectively increase in the two Banners Otog and Otog Front by around 51% and 106%, resulting in an increase of herdsmen's incomes by 267%.
In summary, remote sensing reveals that land use and management policies exerted both positive and negative impacts on the rangelands in the west Ordos region. The policies implemented in the three observed periods have clearly awakened the enthusiasm of peasants, improved the agricultural production, promoted the emergence of hundreds of private enterprises including the ‘sand-control teams’, lifted the regional economy as well as household incomes, and ameliorated the local environment. However, degradation could not be stopped when policies (e.g. those in 1985 and 1987–1988) were only aimed at keeping grassland tenure collective without considering the responsibility of herdsmen, or only at economic development without environmental protection measure or at environmental preservation without any benefit for rural people. Degradation just moved from one place to another following the loopholes in the legislative environment or weaknesses in the enforcement mechanisms. When the implementation of policy, even an enlightened one, could not be well monitored or enforced, or when it conflicted with the personal interests of local people (Erdenzhab Citation2002; Gai Citation2007), land degradation is inevitable since herdsmen would be more interested in increasing short-term benefit rather than protecting the rangelands from degradation.
5. Conclusion
This paper presents a cross-cutting study to assess the impacts of land use and management policies on the rangelands in Ordos by analyzing satellite images at times that correspond to the initial stages of the implementation of different policies. It was noted that in the study area land degradation, although it could be favored by natural factors such as drought and wind blowing, was basically the outcome of policies that did not take into consideration the fragility of the ecosystem, such as the land reclamation works in the early of 1970s (Zhang and Wang Citation2001; Enkhee Citation2003). Some activities under a rational but poorly implemented policy also caused local land degradation, e.g. overgrazing in the permitted areas under the ‘Grazing ban and rotation policy.’ At the same time, the history of the study area demonstrates that where sound policies and effective implementation go hand in hand, mitigation of land degradation and rangeland recovery are feasible. The legalization of the Chinese private economy in 1988 made the sand-control movement by individual families in the region possible and this in turn contributed to the growth in economy and significantly to the restoration of the sandy land and desert ecosystems. The grazing ban policy coupled to a subsidy system did restore and protect a large part of the grasslands and at the same time improve the income and livelihood of the rural people. Therefore, the impacts of policies are complex, depending on the extent, depth and duration of their implementation, but also on their ability to reconcile the desire for a healthy agropastoral ecosystem with the need to improve the livelihoods of poor people who depend on this resource base. If there is a contradiction, land degradation is unavoidable.
In conclusion, although of a reconnaissance nature, this study shows the great potential of remote sensing to evaluate the complex impacts of policy implementation on the rangeland ecosystem by monitoring the changes in biophysical features and land cover, and linking this information to the social and political environment. Interpreting the impacts of a single policy is difficult and very challenging, particularly, when its implementation overlaps with other policies promulgated in the same period. What can be revealed is the global effect of the major one or the joint effect of several ones. The proposed remote sensing methodology, consisting of an integrated processing procedure followed by a calibration with meteorological data, produced relevant results that revealed well the joint effect of policies in each period. Future research shall go in the direction of a more comprehensive and quantitative assessment with more reasonably integrated sets of assessment indicators (Sommer et al. Citation2011; Zucca et al. Citation2012), through a spatial analysis on the extent, depth and duration of the policy implementation linked to biomass and socioeconomic data at commune- or village-level.
Acknowledgements
A part of the Landsat images (1991, 2000, 2002, 2006, 2009 and 2010) were freely obtained from USGS data server (http://glovis.usgs.gov/). Historical meteorological data of different stations were obtained from China Meteorological Data Sharing Service System (http://cdc.cma.gov.cn/), and the recent ones and the socioeconomic data of the study area were from the Statistic Yearbooks of the Banner Otog, Front and of Ningxia dated 2005, 2006, 2007, 2008, 2009 and 2010.
References
- Aspinall, R. 2004. “Modelling Land Use Change with Generalized Linear Models—A Multi-Model Analysis of Change between 1860 and 2000 in Gallatin Valley, Montana.” Journal of Environmental Management 72 (1–2): 91–103. doi:10.1016/j.jenvman.2004.02.009.
- Celis, D., E. De Pauw, and R. Geerken. 2007. Assessment of Land Cover/Land Use in the CWANA Region Using AVHRR Imagery and Agroclimatic Data. Part 2: Hot Spots of Land Cover Change and Drought Vulnerability. Aleppo: International Center for Agricultural Research in the Dry Areas (ICARDA), ISBN 92-9127-195-X.
- Chavez, P. S. Jr. 1988. “An Improved Dark-Object Subtraction Technique for Atmospheric Scattering Correction of Multispectral Data.” Remote Sensing of Environment 24 (3): 459–479. doi:10.1016/0034-4257(88)90019-3.
- Chavez, P. S. Jr. 1996. “Image-Based Atmospheric Correction — Revisited and Improved.” Photogrammetric Engineering and Remote Sensing 62: 1025–1036. https://eserv.asprs.org/PERS/1996journal/sep/1996_sep_1025-1036.pdf.
- Chen, J., and S. Gao. 2001. “Root Causes, Development and Trend of Desertification in Yinjinhuoluo County, Inner Mongolia, China.” Research Report of UNDP Project: Construction of the Capacity to Implement the UNCCD in China. Accessed February 12, 2003. http://nic6.forestry.ac.cn/sts/cy/Root.html.
- Coppin, P., I. Jonckheere, K. Nackaerts, B. Muys, and E. Lambin. 2004. “Review Articledigital Change Detection Methods in Ecosystem Monitoring: A Review.” International Journal of Remote Sensing 25 (9): 1565–1596. doi:10.1080/0143116031000101675.
- Davenport, M. L., and S. E. Nicholson. 1993. “On the Relation Between Rainfall and the Normalized Difference Vegetation Index for Diverse Vegetation Types in East Africa.” International Journal of Remote Sensing 14 (12): 2369–2389. doi:10.1080/01431169308954042.
- Del Barrio, G., J. Puigdefabregas, M. E. Sanjuan, M. Stellmes, and A. Ruiz. 2010. “Assessment and Monitoring of Land Condition in the Iberian Peninsula, 1989–2000.” Remote Sensing of Environment 114 (8): 1817–1832. doi:10.1016/j.rse.2010.03.009.
- Diouf, A., and E. F. Lambin. 2001. “Monitoring Land-Cover Changes in Semi-Arid Regions: Remote Sensing Data and Field Observations in the Ferlo, Senegal”. Journal of Arid Environments 48 (2): 129–148. doi:10.1006/jare.2000.0744.
- Enkhee, J. 2003. “A Historical Rethink about The Grassland Desertification: The Cultural Dimension of Development (In Chinese with English Abstract).” Journal of Inner Mongolia University (Humanities and Social Sciences) 35 (2): 3–9. http://wenku.baidu.com/view/5e8b78a7f524ccbff1218491.html.
- Erdenzhab. 2002. “Reflections on Institutional Deficiency that Account for Grassland Desertification (In Chinese with English Abstract).” Journal of Inner Mongolia University (Humanities and Social Sciences) 34 (5): 8–12. http://caod.oriprobe.com/articles/23347969/Reflections_on_Institutional_Deficiency___That_Account_for_Grassland_D.htm.
- Evans, J., and R. Geerken. 2004. “Discrimination between Climate and Human-Induced Dryland Degradation.” Journal of Arid Environments 57 (4): 535–554. doi:10.1016/S0140-1963(03)00121-6.
- Gai, Z. 2007. “Grassland Property Right and Its Eco-Environmental Protection.” Globalization Forum (in Chinese). Accessed October, 2009. http://www.china-review.com/gath.asp?id=18883
- Gao, J., and Y. Zha. 2001. “Assessment of the Effectiveness of Desertification Rehabilitation Measures in Yulin, North-Western China Using Remote Sensing.” International Journal of Remote Sensing 22 (18): 3783–3795. doi:10.1080/01431160010030073.
- Geoghegan, J., L. Pritchard, Y. Ogneva-Himmelberger, R. R. Chowdhury, S. Sanderson, and B. L. Turner II. 1998. “‘Socializing the Pixels’ and ‘Pixelizing the Social’ in Land-Use and Land-Cover Change.” In People and Pixels, Linking Remote Sensing and Social Science, edited by D. Liverman, E. F. Moran, R. R. Rindfuss, and P. C. Stern, 51–69. Washington, DC: National Academy Press.
- Hellden, U. 1988. “Desertification Monitoring: Is the Desert Encroaching?” Desertification Control Bulletin 17: 8–12. http://www.ciesin.org/docs/002-178/002-178.html.
- Hill, J., M. Stellmes, T. Udelhoven, A. Röder, and S. Sommer. 2008. “Mediterranean Desertification and Land Degradation: Mapping Related Land Use Change Syndromes Based on Satellite Observations.” Global and Planetary Change 64 (3–4): 146–157. doi:10.1016/j.gloplacha.2008.10.005.
- Jensen, J. R., and D. R. Toll. 1982. “Detecting Residential Land Use Development at the Urban Fringe.” Photogrammetric Engineering and Remote Sensing 48 (4): 629–643. https://eserv.asprs.org/PERS/1982journal/apr/1982_apr_629-643.pdf.
- Jia, J. 1997. “Rangeland Degradation in Ordos Plateau, Its Nature and Assessment.” RALA Report, No. 200. Accessed March, 2012. http://www.rala.is/rade/ralareport/Jong.pdf.
- Jiang, H., P. Zhang, D. Zheng, and F. Wang. 1995. “The Ordos Plateau of China.” In Regions at Risk: Comparisons of Threatened Environments, edited by J. X. Kasperson, R. E. Kasperson, and B. L. Turner II, 420–459. Tokyo: United Nations University Press.
- Lambin, E. F., and A. H. Strahlers. 1994. “Change-Vector Analysis in Multitemporal Space: A Tool to Detect and Categorize Land Cover Change Processes Using High Temporal Resolution Satellite Data.” Remote Sensing of Environment 48 (2): 231–244. doi:10.1016/0034-4257(94)90144-9.
- Lambin, E. F., H. J. Geist, and E. Lepers. 2003. “Dynamics of Land-Use and Land-Cover Change in Tropical Regions.” Annual Review of Environment and Resources 28: 205–241. doi:10.1146/annurev.energy.28.050302.105459.
- Geist, H. J., and E. F. Lambin. 2004. “Dynamic Causal Patterns of Desertification.” BioScience 54 (9): 817–829. doi:10.1641/0006-3568(2004)054[0817:DCPOD]2.0.CO;2.
- Li, Z., X. Li, Y. Wang, A. Ma, and J. Wang. 2004. “Land-Use Change Analysis in Yulin Prefecture, Northwestern China Using Remote Sensing and GIS.” International Journal of Remote Sensing 25 (24): 5691–5703. doi:10.1080/01431160412331291206.
- Lillesand, T. M., and R. W. Kiefer. 2000. Remote Sensing and Image Interpretation. 4th ed, 739p. New York: John Wiley & Sons.
- Malo, A. R., and S. E. Nicholson. 1990. “A Study of Rainfall and Vegetation Dynamics in the African Sahel Using Normalized Difference Vegetation Index.” Journal of Arid Environment 19: 1–24.
- Mas, J.-F. 1999. “Monitoring Land-Cover Changes: A Comparison of Change Detection Techniques.” International Journal of Remote Sensing 20 (1): 139–152. doi:10.1080/014311699213659.
- Mertens, B., and E. F. Lambin. 2000. “Land Cover Change Trajectories in Southern Cameroon.” Annals of the Association of American Geographers 90 (3): 467–494. doi:10.1111/0004-5608.00205.
- Rouse, J. W., R. H. Haas, J. A. Schell, and D. W. Deering. 1973. Monitoring Vegetation Systems in the Great Plains with ERTS (Third ERTS Symposium), NASA SP-351 I, 309–317.
- Runnstrom, M. C. 2003. “Rangeland Development of the Mu Us Sandy Land in Semiarid China: An Analysis Using Landsat and NOAA Remote Sensing Data.” Land Degradation & Development 14 (2): 189–202. doi:10.1002/ldr.545.
- Singh, A. 1989. “Review Article Digital Change Detection Techniques Using Remotely-Sensed Data.” International Journal of Remote Sensing 10 (6): 989–1003. doi:10.1080/01431168908903939.
- Sommer S., C. Zucca, A. Grainger, M. Cherlet, R. Zougmore, Y. Sokona, J. Hill, R. Della Peruta, J. Roehrig, and G. Wang. 2011. “Application of Indicator Systems for Monitoring and Assessment of Desertification from National to Global Scales.” Land Degradation & Development 22 (2): 184–197. doi:10.1002/ldr.1084.
- Tucker, C. J., H. E. Dregne, and W. W. Newcomb. 1991. “Expansion and Contraction of Sahara Desert from 1980 to 1990.” Science 253 (5017): 299–300. doi:10.1126/science.253.5017.299.
- Udelhoven, T., M. Stellmes, G. Del Barrio, and J. Hill. 2009. “Assessment of Rainfall and NDVI Anomalies in Spain (1989–1999) Using Distributed Lag Models.” International Journal of Remote Sensing 30 (8): 1961–1976. doi:10.1080/01431160802546829.
- Veldkamp, A., P. H. Verburg, K. Kok, G. H. J. De Koning, J. Priess, and A. R. Bergsma. 2001. “The Need for Scale Sensitive Approaches in Spatially Explicit Land Use Change Modeling.” Environmental Modeling and Assessment 6 (2): 111–121. doi:10.1023/A:1011572301150.
- Verburg, P. H., W. Soepboer, A. Veldkamp, R. Limpiada, V. Espaldon, and S. A. Mastura, 2002. “Modelling the Spatial Dynamics of Regional Land Use: The CLUE-S Model.” Environmental Management 30 (3): 391–405. doi:10.1007/s00267-002-2630-x.
- Wang, J., K. P. Price, and P. M. Rich. 2001. “Spatial Patterns of NDVI in Response to Precipitation and Temperature in the Central Great Plains.” International Journal of Remote Sensing 22 (18): 3827–3844. doi:10.1080/01431160010007033.
- Wessels, K. J., S. D. Prince, J. Malherbe, J. Small, P. E. Frost, and D. Vanzyl. 2007. “Can Human-Induced Land Degradation Be Distinguished from the Effects of Rainfall Variability? A Case Study in South Africa.” Journal of Arid Environments 68 (2): 271–297. doi:10.1016/j.jaridenv.2006.05.015.
- Wu, W. 2003a. “Evaluation on Land Use and Land Cover Changes in North Shaanxi, China.” Photo-Interpretation, 39 (2): 15–29.
- Wu, W. 2003b. “Application de la géomatique au suivi de la dynamique de l'environnement en zones arides [Application of Geomatics to Monitoring the Environmental Dynamics in the Arid Regions].” PhD thesis, Université de Paris 1.
- Wu, W. 2007. “Coastline Evolution Monitoring and Estimation — A Case Study in the Region of Nouakchott, Mauritania.” International Journal of Remote Sensing 28 (24): 5461–5484. doi:10.1080/01431160701227612.
- Wu, W. 2009. “Monitoring Land Degradation in Drylands by Remote Sensing.” In: Desertification and Risk Analysis Using High and Medium Resolution Satellite Data, edited by A. Marini and M. Talbi, 157–169. Netherlands: Springer. http://link.springer.com/chapter/10.1007%2F978-1-4020-8937-4_14#.
- Wu, W., E. F. Lambin, and M.-F. Courel. 2002. “Land Use and Cover Change Detection and Modeling for North Ningxia, China.” In Proceedings of Map Asia 2002, Bangkok, Thailand, August 6–9. http://www.gisdevelopment.net/application/environment/overview/envo0008.htm.
- Wu, W., C. Zucca, and G. Enne. 2005. “Land Degradation Monitoring in the Ordos Region, China.” In Proceedings of the International Conference on Remote Sensing and Geoinformation Processing in the Assessment and Monitoring of Land Degradation and Desertification (RGLDD), September 7–9, Trier, Germany. 618–625. Trier: University of Trier.
- Wu, W., and E. De Pauw. 2010. “Policy Impacts on Land Degradation: Evidence Revealed by Remote Sensing in Western Ordos, China.” In: Land Degradation and Desertification: Assessment, Mitigation and Remediation, edited by P. Zdruli, M. Pagliai, S. Kapur, and A. F. Cano, 219–232. Springer Science. doi:10.1007/978-90-481-8657-0_17. http://link.springer.com/chapter/10.1007/978-90-481-8657-0_17.
- Xie, Y., Y. Mei, T. Guangjin, and X. Xuerong. 2005. “Socio-Economic Driving Forces of Arable Land Conversion: A Case Study of Wuxian City, China.” Global Environmental Change 15 (3): 238–252. doi:10.1016/j.gloenvcha.2005.03.002.
- Xu, J. 1999. “Erosion Caused by Hyperconcentrated Flow on the Loess Plateau of China.” CATENA 36 (1–2): 1–19. doi:10.1016/S0341-8162(99)00009-0.
- Xu, J. 2006. “Sand-Dust Storms in and around the Ordos Plateau of China as Influenced by Land Use Change and Desertification.” CATENA 65 (3): 279–284. doi:10.1016/j.catena.2005.12.006.
- Zhang, F., and L. Wang. 2001. “Analysis on Land Use Situation in the Banner Ejinhoro. Research Report of UNDP Project: Construction of the Capacity to Implement the UNCCD in China.” Accessed February 13, 2002. http://nic6.forestry.ac.cn/sts/tdly/tdly.html.
- Zhou, Q., B. Li, and A. Kurban. 2008. “Trajectory Analysis of Land Cover Change in Arid Environment of China.” International Journal of Remote Sensing 29 (4): 1093–1107. doi:10.1080/01431160701355256.
- Zucca, C., R. Della Peruta, R. Salvia, S. Sommer, and M. Cherlet. 2012. “Towards a World Desertification Atlas. Relating and Selecting Indicators and Data sets to Represent Complex Issues.” Ecological Indicators 15 (1): 157–170. doi:10.1016/j.ecolind.2011.09.012.