Abstract
3D desktop-based virtual environments provide a means for displaying quantitative data in context. Data that are inherently spatial in three dimensions may benefit from visual exploration and analysis in relation to the environment in which they were collected and to which they relate. We empirically evaluate how effectively and efficiently such data can be visually analyzed in relation to location and landform in 3D versus 2D visualizations. In two experiments, participants performed visual analysis tasks in 2D and 3D visualizations and reported insights and their confidence in them. The results showed only small differences between the 2D and 3D visualizations in the performance measures that we evaluated: task completion time, confidence, complexity, and insight plausibility. However, we found differences for different datasets and settings suggesting that 3D visualizations or 2D representations, respectively, may be more or less useful for particular datasets and contexts.
Introduction
Geovisual analytics considers spatial characteristics as an important dimension in making sense of data (Andrienko et al. Citation2010). Thus, data that are inherently spatial in three dimensions may benefit from being visually explored and analyzed in the context of the landscape or other three-dimensional aspects of the environment to which they relate. Earlier research (Bleisch, Dykes, and Nebiker Citation2008; Bleisch Citation2011) has shown some potential for displaying quantitative data as data graphics in 3D desktop-based virtual environments. The study presented here evaluated the performance of this 3D geovisualization technique for visual data analysis by integrating the results from two experiments employing quantitative data graphics with different datasets and tasks.
Background
3D desktop-based virtual environments are popular in science (e.g. Slingsby, Dykes, and Wood Citation2008), teaching (e.g. Patterson Citation2007), and for everyday use (e.g. Bartoschek and Schönig Citation2008). Software for the creation of virtual environments, such as Google Earth (http://www.google.com/earth/), is readily available and it is comparatively easy to integrate a range of spatial datasets and represent these graphically for exploratory visualization (Wood et al. Citation2007). However, evaluations on processes, for example, how visualizations are used to visually analyze data and to generate knowledge, are very rare (Lam et al. Citation2011) – and this is especially true for 3D geovisualizations. Similarly, research on how to most efficiently and effectively display quantitative data in 3D geovisualizations is sparse. Slocum et al. (Citation2009) list perspective height as a visual variable that they deem to be effective for representing numerical values but caution that occlusion and lack of north orientation may cause problems. Scientific visualizations in virtual environments (e.g. Butler Citation2006) display ‘curtains’ of data and tools like the Thematic Mapping Engine (Sandvik Citation2008) implement 2D thematic mapping symbology in 3D virtual environments. Some rare advice on how to best use the three dimensions of space is offered by Ware and Plumlee (Citation2005). They recommend using the x- and y-axes of the screen coordinates (orthogonal to the line of sight) to display information rather than the z-axis – the depth of the 3D environment (along the line of sight). The latter is deemed to be more difficult to interpret. Application of this recommendation may result in restricting navigation or using billboards – data graphics that always face the viewer (Bleisch Citation2011). Seipel and Carvalho (Citation2012) tested simple comparison tasks with bar charts in 2D and stereoscopic 3D displays finding no differences in time or accuracy. However, we still do not know how effective and efficient data display within 3D geovisualizations are in the context of data exploration, especially in relation to the environment in which data were collected. Gaining insight is a considerably more complex task than comparison and one for which geovisual analytics is considered a particularly appropriate approach (Andrienko et al. Citation2010).
Research aim
As Shepherd (Citation2008, 200) notes, we may need to overcome the ‘3D for 3D's sake’ tendency and consider when 3D is more appropriate than 2D in particular contexts. One such case could be when the data to be analyzed have a clear relation to the three-dimensional landscape in which they were collected. As this case has inherent three-dimensional spatial properties we may make use of a three-dimensional representation even though we are less effective at analyzing the depth dimension than the up-down or sideways dimensions of three-dimensional representations (Ware Citation2008). Any deficiencies may be mitigated by the ability to change viewpoint in 3D, perhaps at the cost of time spent making such changes. This study aims to help us better understand how well three-dimensional representations perform in comparison to more traditional 2D representations, with a particular emphasis on such three-dimensional patterns and relationships. The evaluation presented here is based on a comparison of quantitative and qualitative analytical task performance measures between different datasets (and the environment to which they relate) displayed in 2D representations and 3D desktop-based virtual environments. These conditions are termed ‘2D’ and ‘3D’ subsequently. North (Citation2006) claims that insight is the purpose of visualization. Consequently, the study described here follows North's (Citation2006) definition of qualitative, complex, and relevant insights for the qualitative task performance measures. We hypothesize that 3D representations facilitate data analysis in relation to the 3D landform and vice versa 2D representations facilitate data analysis in relation to location. Specifically, we want to evaluate whether exploratory data analysis tasks that have a direct reference to either location (a 2D concept) or altitude (including landforms and height differences) are more efficiently (time) and effectively (confidence, complexity, and plausibility) solved in the 2D or 3D representations, respectively.
Evaluation methods
We planned and conducted two experiments to evaluate the usefulness of data graphics in 3D desktop-based virtual environments – E1 and E2. The experiments used datasets and tasks of varying complexity to compare participants' task performance between the 2D and 3D environments. The data in both experiments were displayed as single bars (univariate data) or bar charts (multivariate data) without (2D environment) or with reference frames (3D environment) following earlier experimental results (Bleisch, Dykes, and Nebiker Citation2008). To facilitate broad participation at different spatial locations both experiments were administered through online questionnaires that could be completed remotely.
Visual analysis tasks
The definition of tasks is a complex issue in geovisualization evaluations (Tobon Citation2005). Interaction taxonomies (e.g. Yi et al. Citation2007) often combine navigation, data display manipulation, and analysis tasks. For 3D visualizations, which are interactive by nature, it is helpful to separate between the different types as we did not test or control for navigation tasks but focused solely on analysis. Additionally, the hypotheses stated required task definitions in which exploration and data analysis were performed in relation to the surrounding landscape – specifically those in which location and altitude/landform were considered with the source data either in independently or in combination. Frequently used data analysis tasks include identify, compare, or categorize as defined in task taxonomies by Wehrend and Lewis (Citation1990) or Zhou and Feiner (Citation1998). However, they do not intuitively support specific definitions of references for the analysis of data, which were core to the current hypotheses. The alternative functional view of data and tasks (Andrienko and Andrienko Citation2006) allows analysis tasks to be defined in terms of two components of data: characteristic and reference. Thus, tasks either referring to location and/or altitude could be defined. Additionally, the Andrienko and Andrienko (Citation2006) task framework allows a wide range of tasks to be defined at different levels of complexity (elementary and synoptic) as is relevant to data exploration. This framework was thus used in our experiments to define directed exploratory tasks to facilitate ideation (Marsh Citation2007).
Experiment E1
Experiment E1 evaluated whether typical users were able to explore and analyze data representations displayed in virtual environments and whether they were able to relate the data representations to altitude and landform. Eight univariate datasets of deer tracks recorded at different hours of the day during summer and winter months were used to prepare 16 visualizations in 3D (using Google Earth) and 2D (using HyperText Markup Language [HTML], Scalable Vector Graphics [SVG], and JavaScript). Both types allowed interaction such as zooming and panning. Viewpoint change was additionally enabled in 3D (). Using a within-subject design, 34 informed participants reported insights gained from completing seven tasks () and judged their confidence in each reported insight on a three-step scale: low, medium, and high (Rester et al. Citation2007). Participants were recruited from final year Geomatics courses and included academic staff from the Geomatics and Geography departments of several universities. Participants completed all tasks in each of two 2D representations and two 3D representations. The seven tasks () used were of varying complexity (elementary and synoptic) and had different reference sets (Andrienko and Andrienko Citation2006). Two tasks referred to location, three tasks referred to altitude, and two tasks referred to location and altitude in combination (). The visualizations were arranged in a balanced order in the different questionnaires to counter confounding carryover effects such as familiarization with a setting, getting used to the tasks or tiredness occurring toward the end of a series of several tasks. The questionnaires contained instructions, a test task to ensure Google Earth was installed on the system and that JavaScript was enabled, in addition to the different visualizations and tasks. They were implemented to be completed online with a current web browser. The questionnaires connected to a database and in total 932 insight reports were collected – 468 in the 2D condition, 464 in 3D. On an average each participant spent 45 minutes on the questionnaire, including reading the instructions, testing the installations, and completing the seven tasks in each of two 2D and two 3D representations.
![Figure 1. Quantitative data and map background (1:25000 © 2014 swisstopo [BA14010]) of the eight different settings (W1–W4, S1–S4) in the 2D (HTML, SVG, and JavaScript, left pairs) and 3D (Google Earth, right pairs) settings.](/cms/asset/fcd9cddf-469f-4923-9f36-a26583543ddd/tjde_a_927536_f0001_oc.jpg)
Table 1. Directed exploratory tasks for Experiment E1 (tasks E1.1–E1.7) and Experiment E2 (task E2.1) referring either to location (L) or, altitude (A), or a combination of location and altitude (LA).
Experiment E2
Experiment E2 extends Experiment E1 to multivariate data displays in virtual environments. Two datasets consisting of the aggregated four summer and four winter datasets from Experiment E1 were used to prepare four visualizations in 3D and 2D. Once again we used Google Earth for the 3D condition and HTML, SVG, and JavaScript for the 2D condition. Both conditions allowed interaction such as zooming and panning, and viewpoint change was available in the 3D case (). Data analysis showed that the seven tasks used in E1 strongly influenced the participants in their analysis as indicated, for example, by their use of words in the reported insights that were similar to those used in describing the task. Thus, Experiment E2 employed one synoptic exploratory task (referring to location, altitude, and time of the day, cf. , task E2.1) in which participants were asked to report all insights gained from visually analyzing the data (Rester et al. Citation2007) and thus resulting in a less guided data exploration session. In a within-subject design, 38 informed participants reported insights and judged their confidence in each insight when visually analyzing one 2D and one 3D representation. Participants were final year Geomatics students – from a different but comparable cohort to those who had participated in E1. The representations, together with experiment instructions and a test task to ensure that Google Earth and JavaScript were working, were implemented as online questionnaires that could be completed in a web browser. Once again, the questionnaires contained the representations in a balanced order to counter confounding factors. The questionnaires connected to a database and in total 522 insights were collected – 260 in the 2D case and 262 in the 3D case. On an average each participant spent 50 minutes completing the questionnaire, including reading the instructions, testing installations, and working on the task with the two datasets and representations.
![Figure 2. Quantitative data and map background (1:25000 © 2014 swisstopo [BA14010]) of the two different settings (W and S) either in 2D (HTML, SVG, and JavaScript) or 3D (Google Earth).](/cms/asset/7c5db907-915f-4c5b-adb1-4589fb585960/tjde_a_927536_f0002_oc.jpg)
The data used for the different settings in E1 and E2 were different subsets and aggregations of tracking data associated with a single deer (eight different settings in E1, cf. and two different settings in E2, cf. ). To account for the influence of different topographies on the analysis, the subset of the data collected during the winter months was spatially shifted to a nearby area that is also frequented by roaming deer. These settings are labeled ‘W’ (winter) in and , with settings in which the deer data are displayed in their original environment labeled ‘S’ (summer). This procedure could have resulted in the loss of some of the connection the deer tracks have with the environment. However, this influence is likely to be minimal as the area into which the data were shifted is also frequented by deer and their movements are generally more limited during wintertime.
Data analysis
The insights collected in Experiments E1 and E2 were analyzed through task completion times and participants' confidence ratings. Additionally, reported insights were qualitatively analyzed and coded. Data of quantitative or ordinal nature, such as recorded task times, confidence ratings, or categorical data from the qualitative analysis were analyzed using appropriate statistical tests. Judgment of significance was made at a level of 95%. As the experiments were administered through online questionnaires it is difficult to control the participants' precise actions and thus task completion times. A few task completion times from insights with comments like ‘I answered a phone call’ as well as three-extreme outliers (as compared to the rest of the values of the concerned participants) were removed from the dataset. The insights were coded by three-independent researchers for complexity and plausibility – both on a three-step scale: low, medium, and high (Rester et al. Citation2007) – and reference type – location (L), altitude (A), or a combination of both LA. The coding dictionary for reference types comprised two categories (location and altitude) with the same three subcategories each: datum, object, and relation/description (cf. ). Each reported insight was analyzed for words defined through the coding dictionary and the words used in reporting the insights coded accordingly. Coding insight is inherently subjective (North Citation2006) and objectivity is achieved through strict coding practices. Reliability of the manual coding process was ensured by comparison of repeated coding processes of the same researcher as well as comparison of the coding between researchers to check for intercoder reliability (Neuendorf Citation2002). No significant differences were found within and between the coders except for the coding of 2D complexity between two researchers (χ2 = 6.08, df = 2, p-value = 0.048). 2D complexity was then recoded after the coding guide had been studied once again by coders and no significant difference was found between coders in the recoded 2D complexity. At the end of both experiments, participants were able to comment generally on the experiment, an opportunity of which most participants took advantage. These comments were analyzed for their content and categorized. The results of this analysis were used in triangulation together with the results from the insight analysis.
Table 2. Word categories used to classify an answer/insight into either referring to location, altitude, or a combination of both.
Results
Comparing performance measures between 2D and 3D
Overall, comparing task times, confidence ratings, complexity, and plausibility measures between the 2D and 3D representations in both Experiments E1 and E2 did not reveal any significant difference between the 2D and 3D settings ().
Comparing performance measures between reference sets
Each task in E1, as well as each collected insight report in E1 and E2, had its own reference set. It referred either to altitude (A), location (L), or a combination of both LA based on the terms used in describing the task (see Data Analysis section). Thus, we were able to compare times, confidence ratings, complexity, and plausibility measures for different reference sets and to evaluate our hypotheses that the 3D representation facilitates tasks relating to altitude and that 2D representations facilitate tasks referring to location. The data analysis showed a statistically significant difference (at level 95%) in E1 for the plausibility ratings with the LA reference set (combination of location and altitude) between 2D and 3D (marked with * in ). The insights reported from the LA reference set in 3D were less often of high plausibility but more frequently of medium or low plausibility than in the 2D case. However, none of the other performance measure comparisons revealed a statistically significant difference between the different reference sets (). Overall, neither the 3D nor the 2D representations seemed to either facilitate or hinder data exploration in either the 2D or the 3D representation.
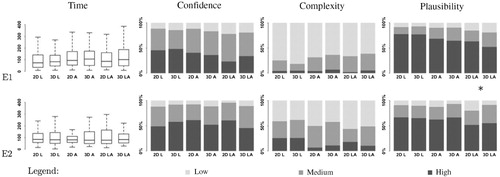
Comparing performance measures between experiments
and show some differences and trends when comparing the two Experiments E1 and E2. For example, participants were significantly more confident when reporting insights based on one generic task in E2 than reporting on the seven more specific tasks in E1 (2D: χ2 = 14.72, df = 2, p value = 0.001; 3D: χ2 = 18.53, df = 2, p-value < 0.001). Additionally, the synoptic task in E2 led to significantly more complex insights being reported (2D: χ2 = 93.74, df = 2, p-value < 0.001; 3D: χ2 = 79.56, df = 2, p-value < 0.001). Experiment E1 used seven tasks () to guide the participants in exploring the data in relation to location, altitude, or a combination of location and altitude. The single task in E2 did refer to location or altitude and was designed to give the participants more freedom in reporting their findings. This seems to have resulted in more complex insights being reported. As shows, participants were able to perform data analysis in relation to altitude (compare L, A, and LA reference sets of insight reports in E1). However, they reverted to reporting findings relating mainly to location when they were less strongly guided by the task (compare L, A, and LA reference sets of insights in E2 in ). This is also clearly shown in , which compares the task reference sets to the insight references in E1. Asking for analysis in relation to location, altitude, or a combination of both yielded insights with reference to location or altitude, respectively (). Additionally, the comments in E1 contained statements from participants explaining that they were not used to evaluating data in relation to the landform and that they found it difficult to do so. This is also shown in where the confidence ratings and also plausibility for the A and LA tasks in E1 are generally lower than for the L tasks. These findings may indicate that either the exploration of data in relation to the landform is not common or that the participants in this study were less ‘informed’ than we anticipated given their backgrounds.
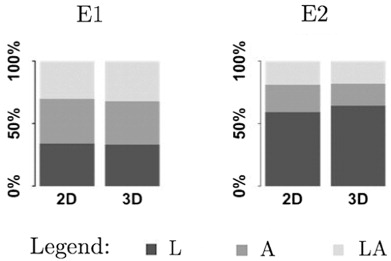
![Figure 6. Comparison of relative quantities of reference set usage (location L, altitude A, or both LA) in the insights reported in E1 compared to the task references (tasks referring to location [tL], tasks referring to altitude [tA], and tasks referring to a combination of location and altitude [tLA]).](/cms/asset/405ce2a1-5d2e-4733-a5a7-e52ecbd9d717/tjde_a_927536_f0006_b.jpg)
Comparing performance measures for different settings
All the settings in E1 and E2 used similar data – different subsets and aggregations of tracking data recorded by a single animal, as described in the Evaluation Methods section. However, comparing time, confidence, complexity, and plausibility measures between the settings for E1 and E2 () showed that there were some significant differences between 2D and 3D. The summer setting in E1 is a case in point, where more time was required in 3D than 2D (Z = −2.01, p-value = 0.044) and more insights of high plausibility were yielded in 2D than 3D (χ2 = 8.27, df = 2, p-value = 0.016). The winter setting in E2 also revealed differences between the 2D and 3D conditions as participants were more confident about the findings reported in the 3D case compared to 2D (χ2 = 10.57, df = 2, p-value = 0.005).
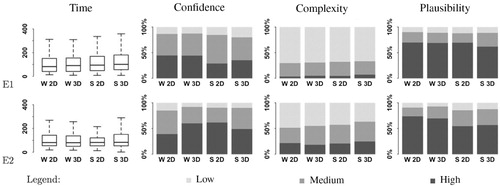
Looking for trends in the eight different datasets in E1 we found differences between 2D and 3D for the task performance measures for most datasets (). Small number of results in a few categories prevented statistical testing for significance as the research was not originally set up to detect differences in analysis between settings and datasets. Nevertheless, summarizing the trends in it seems that some settings and datasets may be better analyzed either in 2D or in 3D or using a combination of both representation types. In the case of just one setting and dataset (W2) analysis in 2D or 3D seem to be equally successful with regard to the evaluated performance measures.
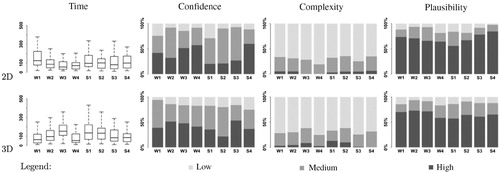
Setting W1: insights were reported more rapidly and with less variation in the times taken in 3D. Participants were more confident in their reported insights in 3D but the results for complexity and plausibility were similar for 2D and 3D. We conclude that setting W1 is faster and analyzed with more confidence in 3D.
Setting W2: the results for time, confidence, complexity, and plausibility were similar for the 2D and 3D conditions in setting W2. We conclude that setting W2 is equally successfully analyzed either in 2D or in 3D.
Setting W3: insights were reported more quickly and with less variation in completion time in 2D. The results for confidence and plausibility were similar for 2D and 3D. However, the 3D setting shows a trend for a higher number of insights of high and medium complexity. We conclude that setting W3 is analyzed more rapidly in 2D but may yield more complex insights in the 3D setting.
Setting W4: reporting time, complexity, and plausibility of insights in setting W4 were similar for 2D and 3D with some trends such as 3D being slightly quicker but yielding fewer highly plausible insights. Participants were more confident in their reported insights in 2D. We conclude that setting W4 may best be analyzed using a combination of 2D and 3D representations.
Setting S1: setting S1 was analyzed more quickly in 2D but with more confidence in the 3D case. A trend toward more complex insights in 3D was apparent. Plausibility was similar for 2D and 3D. We conclude that setting S1 may be analyzed most effectively using a combination of 2D and 3D representations.
Setting S2: insights were reported more quickly in 2D. The results for confidence, complexity, and plausibility were similar for 2D and 3D. We conclude that setting S2 is analyzed more rapidly in 2D.
Setting S3: the results showed similar analysis times and complexity for 2D and 3D. However, insights were reported with more confidence in 3D but higher plausibility in 2D. We conclude that setting S3 may best be analyzed using a combination of 2D and 3D representations.
Setting S4: the results of setting S4 showed less variation in insight reporting times in 3D. However, insights were reported with higher confidence, complexity, and plausibility in 2D. We conclude that setting S4 may be more successfully analyzed in 2D than 3D.
These trends may suggest that analysis in 2D, 3D, or a combination of both types of representation may be preferable dependent on the data and setting. Only setting W2 seems to be analyzed with comparable levels of success in either representation type. Relating these preferences back to specific characteristics of the settings, such as topography, and datasets (number, range, and distribution of the data values) used in these experiments proved difficult. We found no clear relationship between setting characteristics and improved performance in terms of the analysis of the data in one representation type or the other. However, this data dependent finding draws attention to the fact that open designs, in which a variety of options for display and interaction can be accessed quickly and smoothly, may well be important. We are, however, unable to make predictions based upon data characteristics as yet. In the context of Andrienko et al.'s (Citation2010, 1596) recommendation that we ‘develop appropriate design rules and guidelines for interactive displays of spatial and temporal information’ the guideline here given current knowledge may be to emphasize flexible and responsive interactions through which alternative representations can be accessed rather than data and context invariant rules for visual design. This is achievable in the kinds of dynamic environments used in geovisual analytics.
We note that differences between the settings would be expected. All seven tasks could not be equally well completed with all of the datasets. However, this does not explain the differences between 2D and 3D as the same tasks were attempted with the same dataset using the 2D and 3D visualizations.
Discussion and conclusions
In our two experiments a total of 72 different informed participants were able to work with the two types of representations (2D and 3D) to visually analyze the datasets based on different directed exploratory tasks (). Thousand four hundred and fifty-four insights, mostly of high plausibility, were collected. Consequently, we conclude that 2D bars or bar charts with reference frames on billboards are a viable option for displaying quantitative data in desktop-based 3D virtual environments for elementary and synoptic tasks in a range of settings. Participants were able to work with the displays and report insights based on exploratory tasks. This confirms earlier findings developed in more controlled settings (Bleisch, Dykes, and Nebiker Citation2008; Bleisch Citation2011) and suggests that these apply to use cases involving more complex datasets and tasks. Some evidence suggests that participants found analysis of data in relation to landform difficult even though they were able to do so. We may need to improve tools to help them if we deem these kinds of tasks to be important.
For more detailed understanding, especially of the potential of three-dimensional representations (as suggested by MacEachren and Kraak Citation2001), we then analyzed the data predominantly for differences between the two representation types – 2D and 3D. However, contrary to our expectation, we found no significant difference between the two types of representation for the evaluated performance measures (time, confidence, complexity, and plausibility) either in Experiment E1 or in E2. Additionally, analyzing the data for different reference sets (location, altitude, or both) required us to reject our initial hypotheses: we found no evidence that the 3D display of the landform and setting either helps or hinders the interpretation of altitude differences and landform through our experiments; similarly, the 2D display of landform and setting neither helps nor hinders the interpretation of positional aspects such as spatial distribution. However, the analysis of comments, participants reporting more insights in relation to location in E2 as well as the lower confidence ratings for altitude-related tasks in E1 indicate that visual analysis of quantitative datasets in relation to altitude and landform is not common. The fact that our participants were not used to analyzing data in relation to the landform may somewhat confound the results of this study. However, for the comparison between 2D and 3D this influence is minimized through the within-subject design, where participants were asked to perform exactly the same tasks with both representation types.
Generally, conclusions from empirical evaluations are somewhat limited to the datasets used in testing, the particular interface developed and the group of participants used. We sought informed users as participants in our experiments. Seventy-two students and staff from different universities and different ‘geo’-subjects ensured that the experiments were conducted with a large group of users who have interest in these types of interfaces and analysis tasks. Still, the results pertain to this user group and broad generalizations may need further research.
Besides the analysis of the collected data as reported above, the data were additionally analyzed to see whether carryover effects could be found. And indeed, analyzing the data according to ordering but across questionnaires (e.g. all first visualizations compared to all last visualizations) showed that participants generally completed tasks faster and were more confident in their findings later in the experiment. Balancing visualization orders in the different questionnaires employed in each of the experiments ensured that such effects were averaged and thus do not distort the overall results. But this does suggest that learning – in terms of software, data, and context – is likely to be important in geovisual analysis, and this seems particularly significant in contexts where complex tasks are being undertaken, such as those that our participants found difficult. These are the very tasks for which we consider geovisual analytics with 3D representations to be appropriate. This is an issue that should be considered by those designing experiments in which geovisual analytics is performed as well as those designing interfaces through which geovisual analytics is undertaken.
For both, 2D and 3D visualizations, the different datasets and the context or settings to which the data belong strongly influenced the results of the data analysis as shown through variations in the analyzed appropriateness measures (task completion time, complexity, plausibility, and confidence). It seems that for some datasets and settings a visual analysis in either 2D and 3D or a combination of both is preferable. It is difficult to derive advice on when to use 2D or 3D based on this study, as it was not specifically designed for this purpose. However, we have shown that based on the dataset the decision to use either 2D or 3D is reasonably likely to make a difference to the data analysis undertaken. Further research should include the option of combining 2D and 3D views as advocated and tested by Sedlmair et al. (Citation2009) in different contexts. Carefully designed combinations of juxtaposed (Gleicher et al. Citation2011) 2D and 3D views may minimize the increase in cognitive load as eye-movement is the fastest and easiest way to navigate (Ware Citation2008). Ware (Citation2008) states that zooming in 2D is almost always achieved at a lower-cognitive cost than viewpoint change in 3D. The results of this study did not find significant differences in task completion times between interactive 2D and 3D for the range of tasks that we evaluated. Additionally, linking the 2D and 3D views through brushing, as implemented but not evaluated by Bleisch and Nebiker (Citation2008), and commonly used in multi-view 2D information visualization interfaces (Keim Citation2002), could be helpful. Once again, further evaluation is needed.
The empirical data presented here show that in our particular context no significant difference between visual data analysis using either 2D or 3D representations was found. However, importantly there was a variation in results depending on datasets and settings. It suggests that future research in this area should focus on when (in terms of data characteristics and settings) 3D geovisualizations are more or less useful than other types of representations and supporting means of establishing this exploration with appropriate environments for visual analysis rather than more general comparisons of 2D versus 3D.
Acknowledgments
The authors thank the Swiss National Park, especially Dr. Ruedi Haller, for providing the deer data. The background map data of the experiments is reproduced with authorization of swisstopo (map data 1:25000 © 2014 swisstopo [BA14010]). We are very grateful to the participants for taking part in the experiments, investing their time, and thus facilitating this research. The authors also thank the two anonymous reviewers for their valuable comments and suggestions, which have been used to improve this article.
References
- Andrienko, G., N. Andrienko, U. Demsar, D. Dransch, J. Dykes, S. I. Fabrikant, M. Jern, M.-J. Kraak, H. Schumann, and C. Tominski. 2010. “Space, Time and Visual Analytics.” International Journal of Geographical Information Science 24 (10): 1577–1600. doi:10.1080/13658816.2010.508043.
- Andrienko, N., and G. Andrienko. 2006. Exploratory Analysis of Spatial and Temporal Data: A Systematic Approach. Berlin: Springer.
- Bartoschek, T., and J. Schönig. 2008. “Trends und Potenziale von virtuellen Globen in Schule, Lehramtsausbildung und Wissenschaft [Trends and Potential of Virtual Globes at School, in Teacher Education and Science].” GIS Science (4): 28–31.
- Bleisch, S. 2011. “Towards Appropriate Representations of Quantitative Data in Virtual Environments.” Cartographica 46 (4): 252–261. doi:10.3138/carto.46.4.252.
- Bleisch, S., J. Dykes, and S. Nebiker. 2008. “Evaluating the Effectiveness of Representing Numeric Information through Abstract Graphics in 3D Desktop Virtual Environments.” The Cartographic Journal 45 (3): 216–226. doi:10.1179/000870408X311404.
- Bleisch, S., and S. Nebiker. 2008. “Connected 2D and 3D Visualizations for the Interactive Exploration of Spatial Information.” Paper presented at the XXIst ISPRS Congress, Beijing, July 3–11.
- Butler, D. 2006. “Virtual Globes: The Web-wide World.” Nature 439 (7078): 776–778. doi:10.1038/439776a.
- Gleicher, M., D. Albers, R. Walker, I. Jusufi, C. D. Hansen, and J. C. Roberts. 2011. “Visual Comparison for Information Visualization.” Information Visualization 10 (4): 289–309. doi:10.1177/1473871611416549.
- Keim, D. A. 2002. “Information Visualization and Visual Data Mining.” IEEE Transactions on Visualization and Computer Graphics 7 (1): 100–107.
- Lam, H., E. Bertini, P. Isenberg, C. Plaisant, and S. Carpendale. 2011. “Empirical Studies in Information Visualization: Seven Scenarios.” IEEE Transactions on Visualization and Computer Graphics 18 (9): 1520–1536. doi:10.1109/TVCG.2011.279.
- MacEachren, A. M., and M.-J. Kraak. 2001. “Research Challenges in Geovisualization.” Cartography and Geographic Information Science 28 (1): 3–12. doi:10.1559/152304001782173970.
- Marsh, S. L. 2007. “Using and Evaluating HCI Techniques in Geovisualization: Applying Standard and Adapted Methods in Research and Education.” PhD diss., City University London.
- Neuendorf, K. A. 2002. The Content Analysis Guidebook. Thousand Oaks, CA: Sage.
- North, C. 2006. “Toward Measuring Visualization Insight.” IEEE Computer Graphics and Applications 26 (3): 6–9. doi:10.1109/MCG.2006.70.
- Patterson, T. C. 2007. “Google Earth as a (Not Just) Geography Education Tool.” Journal of Geography 106 (4): 145–152. doi:10.1080/00221340701678032.
- Rester, M., M. Pohl, S. Wiltner, K. Hinum, S. Miksch, C. Popow, and S. Ohmann. 2007. “Evaluating an InfoVis Technique Using Insight Reports.” In Information Visualization, 2007, 11th International Conference IV'07, edited by E. Banissi, R. A. Burkhard, G. Grinstein, U. Cvek, M. Trutschl, L. Stuart, T. G. Wyeld, G. Andrienko, J. Dykes, M. Jern, D. Groth, and A. Ursyn, 693–700. Zürich: IEEE Computer Society.
- Sandvik, B. 2008. “Using KML for Thematic Mapping.” MSc thesis, University of Edinburgh.
- Sedlmair, M., K. Ruhland, F. Hennecke, A. Butz, S. Bioletti, and C. O'Sullivan. 2009. “Towards the Big Picture: Enriching 3D Models with Information Visualisation and Vice Versa.” In Proceeding SG '09 Proceedings of the 10th International Symposium on Smart Graphics, edited by A. Butz, 27–39. Berlin, Heidelberg: Springer-Verlag.
- Seipel, S., and L. Carvalho. 2012. “Solving Combined Geospatial Tasks Using 2D and 3D Bar Charts.” In 2012 16th International Conference on Information Visualisation, edited by E. Banissi, S. Bertschi, C. Forsell, M. Johansson, S. Kenderdine, F. T. Marchese, M. Sarfraz et al., 157–163. Montpellier: IEEE.
- Shepherd, I. D. H. 2008. “Travails in the Third Dimension: A Critical Evaluation of Three-dimensional Geographical Visualization.” In Geographic Visualization: Concepts, Tools, and Applications, edited by M. Dodge, M. McDerby, and M. Turner, 199–222. Chichester: John Wiley & Sons.
- Slingsby, A., J. Dykes, and J. Wood. 2008. “A Guide to Getting your Data into Google Earth.” Accessed April 14. http://www.willisresearchnetwork.com/Lists/WRNNews/Attachments/8/An introduction to Getting your Data in Google Earth2.pdf.
- Slocum, T. A., R. B. McMaster, F. C. Kessler, and H. H. Howard. 2009. Thematic Cartography and Geovisualization. 3rd ed. Upper Saddle River, NJ: Pearson Prentice Hall.
- Tobon, C. 2005. “Evaluating Geographic Visualization Tools and Methods: An Approach and Experiment Based upon User Tasks.” In Exploring Geovisualization, edited by J. Dykes, A. M. MacEachren, and M.-J. Kraak, 645–666. Oxford: Elsevier.
- Ware, C. 2008. Visual Thinking for Design. Burlington, MA: Elsevier.
- Ware, C., and M. Plumlee. 2005. “3D Geovisualization and the Structure of Visual Space.” In Exploring Geovisualization, edited by J. Dykes, A. M. MacEachren, and M.-J. Kraak, 567–576. Oxford: Elsevier.
- Wehrend, S., and C. Lewis. 1990. “A Problem-oriented Classification of Visualization Techniques.” In Proceedings of the First IEEE Conference on Visualization, Visualization'90, edited by A. Kaufman, 139–143. San Francisco, CA: IEEE.
- Wood, J., J. Dykes, A. Slingsby, and K. Clarke. 2007. “Interactive Visual Exploration of a Large Spatio-temporal Dataset: Reflections on a Geovisualization Mashup.” IEEE Transactions on Visualization and Computer Graphics 13 (6): 1176–1183. doi:10.1109/TVCG.2007.70570.
- Yi, J. S., Y. Kang, J. T. Stasko, and J. A. Jacko. 2007. “Toward a Deeper Understanding of the Role of Interaction in Information Visualization.” IEEE Transactions on Visualization and Computer Graphics 13 (6): 1224–1231. doi:10.1109/TVCG.2007.70515.
- Zhou, M. X., and S. K. Feiner. 1998. “Visual Task Characterization for Automated Visual Discourse Synthesis.” In Proceedings of the SIGCHI Conference on Human Factors in Computing Systems CHI'98, edited by C.-M. Karat, A. Lund, J. Coutaz, and J. Karat, 392–399. Los Angeles, CA: ACM Press.