ABSTRACT
Computer-based evacuation simulations are important tools for emergency managers. These simulations vary in complexity and include 2D and 3D GIS-based network analyses, agent-based models, and sophisticated models built on documented human behaviour and particle dynamics. Despite the influential role of built environments in determining human movement, a disconnect often exists between the features of the real world and the way they are represented within these simulation environments. The proliferation of emergency management location-aware mobile devices, along with a recent infatuation for augmented reality (AR), has resulted in new wayfinding and hazard assessment tools that bridge this gap, allowing users to visualize geospatial information superimposed on the real world. In this paper, we report research and development that has produced AR geovisual analytical systems, enabling visual analysis of human dynamics in multi-level built environments with complex thoroughfare network infrastructure. We demonstrate prototypes that show how mixed reality visual analysis of intelligent human movement simulations built in virtual spaces can become part of real space. This research introduces a fundamentally new way to view and link simulations of people with the real-world context of the built environment: mixed reality crowd simulation in real space.
1. Introduction
Evacuation plans are an essential component of any establishment’s emergency management efforts. The evacuation plan dictates the necessary set of actions to be observed by evacuees and emergency response personnel in the event of a disaster. Those actions are dependent on the characteristics of the hazardous event, as well as those of the population that is impacted by it. Therefore, there is not a general evacuation plan for all people and places; the evacuation plan must be developed in accordance with the identified risks and corresponding scenarios that could impact the affected population (Nunes, Roberson, and Zamudio Citation2014). Context, that a unique set of circumstances that define the setting for a hazardous event, plays an extremely important role in the development of the evacuation plan, as the spatial and temporal characteristics preceding a disaster define the conditions and performance of the ensuing evacuation. The current suite of tools, including geographic information systems (GIS) and evacuation simulation software, that are used to evaluate and develop emergency evacuation plans captures a generalized or static snapshot of that context at a specific point in time. Current egress models, without sufficient supporting behavioural data to handle the complex interaction between evacuees and high-resolution models of the built environment, often simplify the overall process to provide a baseline indication of evacuation performance (Gwynne Citation2012). New methods of visualization, which connect these virtual spaces of simulation with the complex real-world spaces they analyse, could help enhance emergency evacuation cognizance in built environments.
Evacuation plans are often developed with the support of simulation software, GISs, prescriptive governmental guidelines, and published experiences. The evacuation routes delineated by these plans often define the movement of people based on specific constraints (i.e. building codes and government policy) more than the non-linear dynamics of the evacuation as defined by the context under which it occurs (Cepolina Citation2005). An evacuation route is more complex than the path defined by the shortest distance from one place to another, and a failure to account for the dynamic interactions between people and the environment represents a flawed foundation from which to prescribe evacuation procedures. Emergency egress software developers have evolved their models to include these interactions; however, their spatial and temporal context represents a static characterization of a dynamic environment that variably changes over time.
Recent research conducted by Lochhead and Hedley (Citation2017) revealed the differences in simulation outcomes when using a GIS, versus the artificial intelligence (AI) capabilities of 3D game engines, to analyse evacuation performance in multilevel built environments. That work revealed the limitations of a GIS to represent the complexity of 3D institutional spaces and pathways of movement (changing widths, topology, erroneous floor plans, nonlinearity of human behaviours, effects of furniture, collisions, pileups, and impedances of doors). In addition to the challenges of adequately modelling and simulating institutional spaces and human dynamics within them, the layout of the spaces themselves can often be multilevel, large-volume architectures that have evolved over time. This can result in corridor networks being buried deep within the architecture. GIS and architectural software have made some progress towards modelling the geometry of these often complex spaces (Thill, Dao, and Zhou Citation2011; Eaglin, Subramanian, and Payton Citation2013; Zhou et al. Citation2015), though there are still many issues to overcome, to move beyond floor-by-floor planar representation and analysis, to adequately represent and analyse institutional geometry and topology contiguously in three dimensions over time. The architectural community has arguably made more progress through building information modelling (BIM), which includes geometric and semantic building properties, inspiring BIM-oriented indoor data models for indoor navigation assessments (Isikdag, Zlatanova, and Underwood Citation2013). City Geography Markup Language (CityGML), which specifies the 3D spatial attributes and defining characteristics of 3D city models (Gröger and Plümer Citation2012), represents progress within the geospatial community towards BIM and GIS integration (Liu et al. Citation2017).
This paper introduces the research and development behind a workflow that helps close the gap between the virtual spaces where evacuation simulations are conducted and analysed, and the real-world spaces where the outcomes of these analyses need to be understood. There is a significant emerging opportunity for emergency managers to harness the power of situated visual analysis. Elsayed et al. (Citation2016) combined visual analytics with augmented reality (AR) for what they termed situated analytics, enabling analytical reasoning with virtual data in real-world spaces. The prototype interfaces presented in this paper complement recent research (Lochhead and Hedley Citation2017), which uses game engines and AI as a method for simulating evacuation behaviour in complex 3D spaces. Mixed reality (MR) and situated geovisual analytics, when applied to emergency management, connect the evacuation simulations developed in virtual spaces with the real-world spaces they aim to characterize. This connection provides a level of visual reasoning that is currently unavailable in evacuation analyses.
Regardless of how sophisticated the 3D visualizations or simulations of evacuation software such as EXODUS, SIMULEX, Pathfinder, or MassMotion may be, to date, the majority of these simulations have been implemented in desktop software environments. Implicitly, this assumes that the geometry, spatial relationships, and dynamics seen onscreen can be related to the real spaces they aim to represent and simulate. Harnessing the power of mobile technology and MR-based visualizations, we have developed a workflow that transforms the way evacuation simulations are experienced, and their connections to real-world spaces are realized. These prototype interfaces relocate game-engine-based evacuation simulations to the real world, enabling in situ visual analyses of computer-simulated human movement within real-world environments. The objective is to spatially contextualize 3D, AI-based evacuation simulations by superimposing virtual evacuees onto the real world, allowing emergency managers to identify the possible impedances to emergency egress that are missed, or are inadequately represented, using current methodologies.
2. Research context
The research presented in this paper is a continuation of the authors’ ongoing efforts to design and demonstrate the importance of spatially rigorous virtual spaces, for evacuation simulation and egress analysis, which capture the complex and dynamic nature of real-world built environments. Simulation environments, much like maps (Wright Citation1942), are a representation of objective reality predisposed to the subjectivity and implicit biases resultant from who created them, for what purpose, with what level of expertise or experience, and using which methods. Prioritization of feature importance, or the standards that are established to ensure the quality of their representation of reality, are choices made by analysts directly, or may reflect limited technical capacity or data asset options. These choices are not necessarily underhanded attempts to hide the truth but reflect the capabilities and requirements of the software, and the prescriptive nature of building codes and regulatory guidelines. However, simply quantifying how long it takes to get from one point to another is an extremely linear approach to what is a very non-linear problem. Evolving emergency scenarios, human decision-making, and unexpected circumstances in three-dimensional built environments are not well served by many conventional spatial analytical methods.
Assessing human crowd evacuation or tsunami inundation – simply by quantifying shortest path distances, characterizing positions of final crowd destinations, or computing the line of maximum inundation – only provides us with a limited analytical narrative. Revealing the dynamic properties of the space and the agents throughout the event may be more informative than the final geometry alone. An assumption that the geometric properties of the landscape are constant is risky, and relying on simulations based on static database representations makes analyses that include everyday circumstances impossible, such as temporary objects (such as stages and furniture), doors being locked or out of order, and movable objects being knocked over to create dynamic impedances. The Internet-of-Things (IoT), the capacity for real and virtual objects (things) to communicate with each other (i.e. their situation or location), could be beneficial to emergency management when integrated with virtual models of the built environment (Isikdag Citation2015). While IoT would provide real-time information beneficial to evacuation simulations, the technology only provides that information for IoT capable objects, leading to assumptions about the topology of indoor space. Zlatanova et al. (Citation2013), in their comprehensive review of indoor mapping and modelling, identified real-time data-acquisition, change detection, and model updating, as well as differences in data structure, real-time modelling, and 3D visualization as emerging problems.
While the visualization capabilities of GISs and simulation software have evolved to allow the user to see the process and not just the result, that process continues to be constrained by the hardware, the software, and the resolution of the spatial model. Virtual geographic environments, which allow for computer-aided geographic experiments and analyses of dynamic human and physical phenomena (Lin et al. Citation2013), provide an ideal platform for the simulation and visualization of human movement in built spaces. The following subsections present an overview of some of the tools currently available to emergency managers for evacuation assessments.
2.1. Emergency management
Emergency management is a multifaceted framework that is designed to help minimize the impact of natural and manmade disasters (Public Safety Canada Citation2011). It is a cyclical process consisting of four components: mitigation and prevention, preparedness, response, and recovery (Emergency Management British Columbia Citation2011; Public Safety Canada Citation2011). GIS-based analyses and evacuation models provide valuable contributions to all components of the cycle (Cova Citation1999).
A GIS is an important tool in the field of emergency management. These systems, and the science behind them, have been used to monitor and predict hazardous events, to form the foundation of incident command systems, to coordinate response efforts, and to communicate critical safety information to the public (Cutter Citation2003). GIS-based spatial analyses can be applied to evacuation assessments at all scales, from large-scale evacuations of entire cities to small-scale evacuations of multilevel buildings or the individual floors within them (Tang and Ren Citation2012). In a GIS, the complex transportation networks (i.e. roads or hallways) for network-based analyses are represented by an interconnected system of nodes, the defining details of which are contained within the associated attribute tables. GISs can be integrated with BIM and IoT to provide real-time geometric and semantic data about the built environment (Isikdag Citation2015). However, obstacles remain for GIS and BIM integration, including differences in spatial scale, granularity, and representation (Liu et al. Citation2017), and despite the help of IoT, there are some real-world changes to the transportation network (i.e. building maintenance or construction) that must be manually documented in the GIS for evacuation analyses to be accurate. Maintaining the attribute tables, as well as the algorithms which bring the evacuation model to life, is a major challenge for GIS-based evacuation analyses (Wilson and Cales Citation2008).
Accurate estimates of the time required to evacuate a building are critical in the design of new infrastructure that must meet specific code requirements. These performance-based analyses range from handwritten equations to complex computer-based models, all of which are focused on assessing the safety of the building (Kuligowski, Peacock, and Hoskins Citation2010). This assessment can be used to compare the safety of different architectural designs prior to construction, or to compare different egress strategies on pre-existing structures (Ronchi and Nilsson Citation2013). Regardless of the application, the defining element of these egress models is the accurate representation of human behaviour and not the specific characteristics of the building beyond the structure itself. Each model presents a simplified version of human behaviour that is based on the model developers’ level of knowledge and relies on their judgement as to what aspects of that behaviour are important (Gwynne, Hulse, and Kinsey Citation2016). Furthermore, these models often require that users input a significant amount of behavioural data themselves and that they construct the building manually or extract the structure from CAD drawings (Gwynne, Hulse, and Kinsey Citation2016). Evacuation modelling is therefore highly subjective, relying on the discretion of the developer as well as the user.
Videogame development software has emerged as a flexible platform from which to conduct evacuation modelling. While software such as Unity was not designed for evacuation analyses, researchers have validated the results from Unity-based simulations, confirming that they align with real-world tests and the evacuation data published in the research literature (Ribeiro et al. Citation2012; Chiu and Shiau Citation2016). These game-based environments can simulate human movement using built-in physics engines, navigation meshes, and AI-based agents. They are compatible with a wide range of 2D and 3D formats, including many of the outputs from a GIS, and can be used to create a wide range of visualizations. However, the ability to produce experiential virtual environments (VEs) that can be used to train evacuees and responders, as well as to plan emergency responses and evaluate their efficacy, provides an added level of value absent from GIS analyses and egress modelling. Researchers have developed game-based VEs that teach children the importance of fire safety and allow them to experience an immersive, head-mounted display (HMD)-based simulation of an evacuation from a structural fire (Smith and Ericson Citation2009), and have used surround displays and HMD-based environments to test wayfinding procedures and to evaluate the effectiveness of emergency signage (Tang, Wu, and Lin Citation2009; Meng and Zhang Citation2014). Advancements made with game-based environments are beginning to close the gap between the simulation space and the real world.
The formation of an evacuation plan is a scientific process that involves the assessment of risk and the careful consideration of potential life-threatening scenarios. Like maps, computer simulation spaces offer subjective representations of the real world. The aforementioned tools that are employed to help formulate the emergency plans for those spaces are attempting to replicate the constantly changing physical and human environments, which fluctuate not only over space and time but with the lens through which they are viewed (Torrens Citation2015). While game engines themselves cannot overcome the issues of subjective spatial representation, they provide an opportunity for additional human–computer (immersive) interaction and physics-enabled simulation environments, reducing the prescriptive nature typical of current egress models. Game engines also provide a platform for the development of new tools that change the way one visualizes the phenomena, effectively enabling situated analysis of simulated human movement in real space.
2.2. Geovisualization
Geovisualization is a multifaceted field involving the visual exploration, analysis, synthesis, and presentation of geospatial data (Slocum et al. Citation2001; Kraak and MacEachren Citation2005). Geovisualization combines the methodologies from cartography and GIScience with the technologies developed for GIS, image processing, computer graphics (including game development, animation, and simulation) (Bass and Denise Blanchard Citation2011), and mixed reality (Lonergan and Hedley Citation2015). The objective is to provide new ways to visualize geospatial problems, thereby revealing the complex structures of, and relationships between, geographic phenomena (MacEachren and Kraak Citation2001). As they relate to emergencies and hazards, geovisualizations have the potential to influence risk perception and the communication of risk and could improve disaster mitigation, preparedness, response, and recovery (Bass and Denise Blanchard Citation2011).
Geovisualizations can be applied to emergency preparedness using an array of interface technologies. Each of these interfaces is situated somewhere along the ‘Reality-Virtuality Continuum’ that was introduced by Milgram et al. (Citation1994) to help classify the relationships between an emerging collection of visual display systems (). At one end of the continuum, there are real environments (RE); any environment containing exclusively real-world objects that are viewed either in person or using some form of video display (Milgram et al. Citation1994). At the other end of the continuum, there are VEs; any environment that consists solely of virtual objects that are viewed using a video or immersive display system (Milgram et al. Citation1994). Between these two extremes lies mixed reality (MR); any environment containing a combination of real and virtual content. The MR environment can be further subdivided according to the proportion of real and virtual content; AR environments are primarily real spaces supplemented with virtual content, and augmented virtuality environments are primarily virtual with added real-world content (Lonergan and Hedley Citation2014).
Figure 1. A modified representation of the reality-virtuality continuum introduced by Milgram et al. (Citation1994).
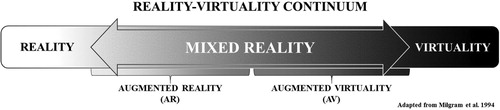
The application of these technological interfaces to emergency management has the potential to transform the way in which people prepare for emergencies; not for their novelty, but for what they allow the user to visualize, how they allow them to visualize it, and how they help the user comprehend the spatial phenomena at hand. New knowledge formation and sensemaking activities are supported by interactions with visualization tools (Pike et al. Citation2009; Ntuen, Park, and Gwang-Myung Citation2010), and a combination of visualizations provides greater opportunity for data exploration and comprehension (Koua, Maceachren, and Kraak Citation2006). By applying multiple methods of visualization to emergency management, the user is better equipped to appreciate the phenomena, perhaps shedding light on that which may be overlooked or exposing that which cannot be seen.
2.3. Situated geovisualizations
Geovisualizations are designed to improve the viewer’s comprehension of geospatial phenomena by creating a cognitive connection between abstract information and the real world. Metaphors are often used to facilitate that connection, as they help create a meaningful experience that users can connect to their experiences in real-world spaces (Slocum et al. Citation2001). However, emerging ‘natural’ interfaces – such as tangible and mobile AR – are demonstrating that interface metaphors are less necessary with certain AR applications, as these AR interfaces create a direct connection between geospatial information and the real world through strong proprioceptive cues and kinaesthetic feedback (Woolard et al. Citation2003; Shelton and Hedley Citation2004; Lonergan and Hedley Citation2014).
AR interfaces can be generally categorized as either image-based or location-based systems (Cheng and Tsai Citation2013). Image-based AR (also known as tangible AR or marker-based AR) uses computer vision software to recognize a specific image and then renders virtual objects on the display system according to the position and alignment of that image in space. Location-based AR (or mobile AR) uses GPS- or Wi-Fi-enabled locational awareness to pinpoint the location of the user and superimposes virtual information on the display system according to their position in space. Location-based AR facilitates the cognitive connection between the real world and virtual information without the metaphor, allowing the user to reify abstract phenomena in real-world space and in real time (Hedley Citation2008).
This real-time reification of geospatial information aids in the process of spatial knowledge transfer by allowing the user to connect the virtual information on the display to the real world, in situ (Hedley Citation2008, Citation2017). The situated nature of location-based AR generates a tacit learning environment, where users draw on cognitive strategies to make sense out of complex, and often abstract, phenomena (Dunleavy, Dede, and Mitchell Citation2009). Location-based AR holds the ability to provide spatial context, which when missing, often hinders one’s capacity to connect abstract information to real-world phenomena.
2.4. Applied MR
2.4.1. Novel applications
Modern applications of MR have received plenty of attention recently. Perhaps the most widely recognized example of mainstream MR is Pokémon Go, a location-based AR application for mobile devices that superimposes Pokémon characters on real-world landscapes and tasks players with their capture. This is just one of a number of recent applications of AR to gain notoriety; however, the concept behind this technology is not new – computer-generated AR was first introduced in the 1960s by Ivan Sutherland (Shelton and Hedley Citation2004; Billinghurst, Clark, and Lee Citation2015), while the term AR was not coined until the 1990s (Cheng and Tsai Citation2013). The recent media attention towards and public adoption of this technology is primarily the result of obtainability, as powerful mobile technologies and a collection of HMDs have become more affordable. With mainstream acceptance will come a greater number of AR applications for education, business, healthcare, entertainment, and much more.
2.4.2. Geographic applications
Geographic applications are particularly well suited for MR interfaces, as the scale, complexity, and dynamic nature of many phenomena makes them difficult to study in situ, and translating concepts from print to the real world can be cognitively demanding. AR has been used to augment paper maps with additional information (Paelke and Sester Citation2010), to view and interact with 3D topographic models (Hedley et al. Citation2002), to assist with indoor and outdoor navigation (Dünser et al. Citation2012; Torres-Sospedra et al. Citation2015), to reveal underground infrastructure (Schall et al. Citation2009), and even to simulate and watch virtual rain (Lonergan and Hedley Citation2014) or virtual tsunamis (Lonergan and Hedley Citation2015) interact with real-world landscapes.
2.4.3. Emergency management applications
The number of AR interfaces that have been designed specifically for emergency management activities is limited. Some aid post-disaster building damage assessment (Kim, Kerle, and Gerke Citation2016), while others help with navigation and hazard avoidance (Tsai et al. Citation2012; Tsai and Yau Citation2013). Hazards may not always be visible or apparent, and AR can help to raise awareness and reduce risk through situated visualization. Hedley and Chen (Citation2012) demonstrated the potential of AR to superimpose illuminated risk-encoded evacuation routes and egress markers on real landscapes. Their work reveals normally invisible risk, quantifies it, and provides citizens with an opportunity to see their everyday surroundings from a risk assessment perspective.
2.5. AR for emergency management
AR interfaces provide a medium for users to enhance real-world environments with virtual information. In many respects, emergency management deals with hypothetical situations that could benefit from AR interfaces (i.e. what an evacuation would look like). The preceding sections of this paper outlined some of the resources used in emergency management to analyse emergency egress and highlighted some of the problems associated with the representation of real-world environments in digital spaces. The subsequent sections present the authors’ workflow for developing situated AR interfaces that enable visual analysis of human movement in real-world spaces. The objective is to provide a workflow that supplements current toolsets and allows evacuation planners to compare dynamic AI-based evacuation movement against real-world features in situ, thus overcoming the problems of subjective spatial representation.
3. Methodology
The workflow behind a set of new AR interface prototypes for the simulation and visual analysis of human movement, set within the context of real-world environments, is presented here. This research builds upon the authors’ previous work, which highlights the impact that scale of representation and the problems associated with modelling dynamic environments, have on evacuation simulation outcomes (Lochhead and Hedley Citation2017). The following subsections describe the hardware and software used in the development of these interfaces.
3.1. Interface design
The visualizations presented in this paper were developed for mobile devices using the Android operating system. The chosen operating system reflects the available technology and the developer permissions associated with it; this workflow could easily be adapted for Apple- or Windows-based devices by switching the target platform and software development kit (SDK) settings within the development software. These prototypes were tested on the authors’ Samsung Galaxy smartphones (S4 and S7) and Samsung Galaxy tablet (Tab S). These mobile devices are compact, yet powerful enough to run the simulations efficiently, and have the built-in cameras required for image recognition. Location-based services are also available on these devices and could be used for location-based AR; however, smartphone GPS accuracy ranging from 1 to 20 m (Wu et al. Citation2015) was determined to be insufficient for visual analyses that rely on highly accurate locational data. Any error in alignment caused by inaccurate or unstable positional data would translate to misalignment of the AR-based geovisualization.
3.2. AR tracking
The developed prototypes are an example of image-based AR. The software has been designed to recognize (track) an image and uses that image to render and align the virtual content according to the position and size of that image relative to the position of the user (camera) in the real world. One of the most common types of images used for AR is a coded black-and-white image similar to a QR code (Cheng and Tsai Citation2013). The high contrast of these images is ideal for image recognition by the software. These prototypes use what is known as natural feature tracking, where the software can be trained to recognize any visual feature. The performance of the augmentation is dependent on the contrast within the image, thus natural features must be chosen carefully to ensure optimal performance. In these examples, the prototype applications have either been developed to recognize the visual features of a building (from a photograph) or of a specific fiducial marker.
3.3. Development
The workflow used to produce these prototypes is just one example of several methods for producing AR-based geovisualizations. Regardless of the workflow, there are some essential components, including 3D objects, specialized modelling and development software, and a display device. The following subsections outline the process behind these prototypes.
3.3.1. 3D modelling
The foundation of these prototypes is a 3D model of the building in which the evacuation simulation will take place (). Each of the 3D models was constructed using SketchUp 3D modelling software. An architectural drawing (.dwg file) of the floor plan for each floor within the building was imported into SketchUp, and the 3D structure of each was then extruded from the 2D drawing per building specifications. The floors were then aligned one on top of the other, ramps were added to represent the stairwells connecting each floor, and the completed models were exported as 3D files (.fbx or .obj).
3.3.2. 3D virtual environment
A software system that was capable of AR integration, supported Android development, and which contained 3D navigation networks, AI-based agents, and 3D physics was required for these prototypes. A 3D game engine (Unity version 5.3.4) and Vuforia’s AR SDK were selected since they contained the necessary functionality.
Unity contains a built-in feature known as a navigation mesh that defines the walkable area within the VE (). A navigation mesh was created for each of the 3D models, the specifications of which are defined by the dimensions of the people (AI agents) within the VE. These prefabricated AI-based agents are used to simulate the movement of people within the VE. The characteristics of those agents are defined by average adult male height and shoulder width, and their walking speed is fixed, based on prior evacuation analyses (Lochhead and Hedley Citation2017), and falls within a range of documented evacuation walking speeds (Rinne, Tillander, and Grönberg Citation2010).
Figure 3. The movement of the AI agents in the simulation is restricted to the navigation mesh (darker grey regions of the floor).
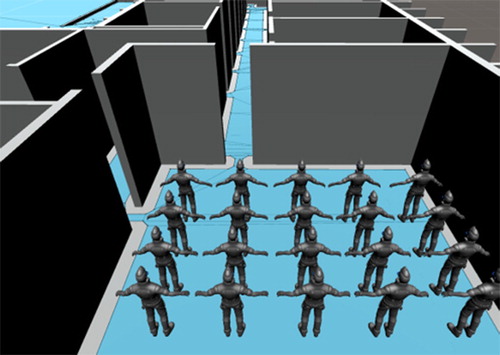
Vuforia is a popular piece of AR software that integrates seamlessly with Unity. The SDK and the accompanying developer portal allow the user to create customized AR applications as well as image targets for natural feature tracking. Each of the VEs was developed around those specialized targets, and any 3D objects attached to the targets are rendered in AR when the target image is detected by the mobile device’s camera.
3.3.3. Android deployment
Unity supports development for a wide range of platforms including mobile devices, computers, and videogame systems. The Android build support used in this research was installed as part of the Unity software package. Additionally, the Android SDK and Java development kit (JDK) were required. Once installed and properly integrated with Unity, the application can be deployed directly to a mobile device via USB connection.
4. Situated AR simulations
This research focused on the production of a workflow and a series of prototypes for evacuation analyses that are situated in the real environments of analysis. The prototypes presented in the following sections demonstrate these assessments at different scales and assess movement from the interior and exterior of a building. The first two examples demonstrate image-based AR developed around images of a building, and the second two using a portable image target that can be positioned for analyses in different locations.
4.1. AR example #1
The first AR-based geovisualization simulates the movement of evacuees as they move from various locations inside a multilevel building to a single destination outside of that building. The simulation contains 3D terrain, derived from a digital elevation model (DEM), that provides the walkable surface allowing agents to navigate the exterior of the building, a 3D model of the building itself, and Unity’s standard asset characters serving as the AI agents (the evacuees). The DEM and building renderers have been turned off so that only the agents and their pathways are augmented onto the landscape. The objective was to enable the user to analyse the simulated movement of people as they evacuate the building and to provide an interface that allows for the comparison of agent movement to the physical features of the built environment. This provides an opportunity for situated visual analysis related to queries such as how many evacuees would be impacted by the bench and statue in front of the main door? These analyses could then be used to guide more complex computer-based evacuation simulations that test the impact of those features on emergency egress.
In this example, a photograph of the building was captured with the same Samsung tablet that runs the application. That photograph was converted into an image target using Vuforia’s online developer tools and was then uploaded to the Unity VE. That image target was positioned in the scene so that the features of the building in the image align with the features of the building in the model. When the user positions themselves in front of the building and directs the device’s camera towards the building the software searches for the features that are present in the image and displays the evacuees on the screen as augmented 3D content on the landscape ().
Figure 4. (Main) The AR application recognizes the features of the building and supplements the real world with dynamic, virtual evacuees, providing an opportunity for situated evacuation analysis. (Inset) A view of the tablet being used for situated AR analysis.
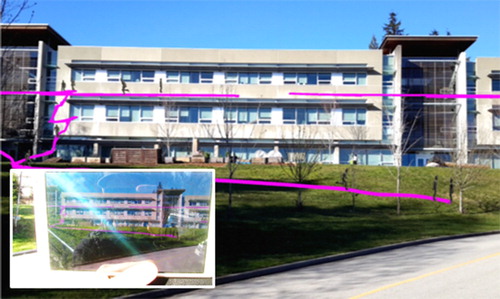
This visualization was inspired by a conversation with an emergency management team, in which they stated that they do not know what it would look like if everyone was forced to evacuate. This prototype demonstrates that AR could be used to visualize the mass evacuation of people from a building and that AR could provide emergency management officials with the experiential knowledge that is gained through a real evacuation event, or a full-scale evacuation exercise, without the associated temporal and financial overhead.
4.2. AR example #2
The second AR prototype was developed using the same principles as the first but focuses on a specific section of that same building. Instead of assessing a mass evacuation, it addresses egress through one of the main entrances. A photograph of that entrance was used to create an AR image for natural feature tracking, therefore when the mobile device’s camera is directed towards the doorway, the display reveals virtual content superimposed on the real world ().
Figure 5. (Left) The AR software has been trained to recognize the features of the building from a photograph. (Middle) When the device’s camera is aimed at the building, the application searches for the features in that photograph. (Right) Once the software has recognized the image, it superimposes the virtual evacuees in the real world.
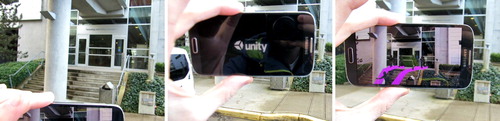
The complex nature of the built environment can sometimes result in structural features in peculiar places. This example highlights one such case, where a support column for an overhead walkway is positioned at the bottom of the staircase that leads to the main entrance. The column (and a garbage can) is positioned such that they could impact egress from the building. This AR interface allows the user to visually assess their potential impact based on the simulated movement of the building’s occupants that would be expected to evacuate through that doorway.
4.3. AR example #3
The third example of situated AR focuses on the movement of people within a building. The built environment is dynamic, changing as people and objects move over time. Yet it is often represented using an obsolete set of static features or simply as an empty 3D structure. These situated AR-based egress analyses help overcome those obstacles by placing the analysis in the real-world spaces being evaluated.
This prototype was developed using image-based AR and requires a specific printed image target. The position and size of that target in the real world must match the position and size of that target in the VE so that the AR simulation aligns properly with the building. In this example, the VE includes a 3D model of the building and several AI agents. The 3D model of the building is not rendered in the AR application but is used to provide the necessary occlusion that prevents the user from seeing agents through walls. When the user places the image target at the designated position and directs their mobile device at it, virtual agents are displayed travelling from their offices to the nearest stairwell ().
Figure 6. AR allows for situated analysis of virtual human movement in real space. These images depict the application in use, displaying simulated movement of evacuees through one of the 6th floor corridors.
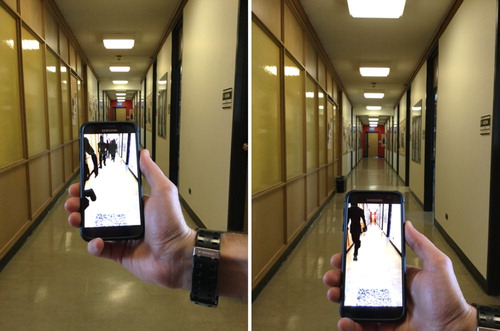
The objective of an AR interface like this one is to identify the features within the built environment that could impede egress, but which are not typically accounted for in computer-based egress analyses (or were not present when that egress model was constructed). Situated analyses could determine that those features would have little impact on egress or could be used to identify features that must be included in the egress model for more in-depth analyses of their impact. Doors are an example of dynamic features that are not accounted for in models, but which have an impact on human movement in real life. While algorithms attempt to account for the impacts of doors, the characteristics of the doors themselves are not constant over space and time. Furthermore, one doorway may only serve a small population, while another may be used by hundreds of evacuees; therefore, any assumptions about impedance are not universal to every doorway. AR interfaces such as this could be used to assess the movement of people through these features ().
4.4. AR example #4
Architectural drawings are the foundation for building construction in most egress modelling programmes, and therefore in any evacuation assessment based on them. Of the 26 egress models evaluated by Kuligowski, Peacock, and Hoskins (Citation2010), 21 were compatible with CAD drawings which are celebrated for their efficiency and accuracy. Identifying errors in these models is difficult, as the builder (or viewer) must be familiar with the structure and (or) cross-reference the features in the drawings with those in the real world. That task is nearly impossible if the modelling and simulation are conducted from a remote location. The final example of situated AR egress analysis illustrates how AR could be used to confirm the accuracy of these models.
This example employs the same model, agents, and image target used in example 3, but assess the movement of people from their offices on the 6th floor to the exterior of the building on the 4th floor (). Here, the image target is positioned at a specific, measured location outside of the building that precisely matches its position within the simulation space. The 3D model of the building was developed from architectural drawings supplied by the institution and posted on their website, so it is assumed that these are accurate representations of the building’s features.
Figure 8. AR image targets can be positioned anywhere, as long as their position in the real world matches their location in the virtual environment. In this instance, the image position on the outside of the building allows for the visual analysis of human movement within it. (Main) A screenshot of virtual evacuees superimposed on the real world. (Inset) The author using the AR application.
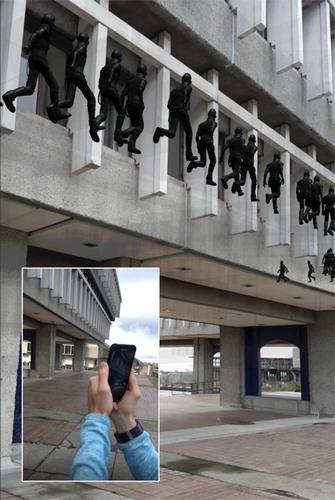
The results of this situated simulation reveal that agents can exit the building through a doorway on the north side of that stairwell that is not present in the real world (). While an exit is present on the south side of that stairwell, it leads to an interior courtyard which could be a hazardous environment after an event such as an earthquake or fire. Therefore, any egress analysis based on that incorrect architectural drawing would be inaccurate and could potentially place people in danger if they are taught to evacuate based on those inaccurate assessments. AR provides the context that is required to identify modelling errors by connecting the simulation space with the real world.
Figure 9. Situated AR can be used to ensure the accuracy of the 3D models used in evacuation analyses. In this instance, the location of a doorway in the architectural drawings does not match the real-world layout of the building; therefore, agents are able to evacuate through a doorway that does not exist. (Left) The author assessing human movement using situated AR. (right) A screenshot of the virtual agents evacuating through a non-existent doorway.
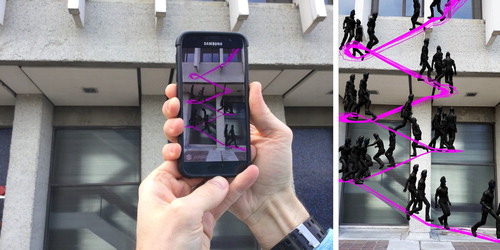
5. Discussion
The work that is presented in this paper serves to exemplify how AR can be used to bridge the gap between simulated evacuation analyses and the real world. This workflow helps to overcome the limitations associated with GIS-based analysis and egress modelling but is not a replacement for them. AR-based analyses are a tool to be used by emergency management personnel for situated evacuation analyses, supplementing and informing the existing workflow in an attempt to improve overall emergency preparedness.
A few of the limitations observed during the development and testing of these prototypes, and some possible areas for future research and development related to situated AR-based movement analyses, are presented in the following subsections. Also presented are some comments on the anticipated application of this technology to current emergency management practices.
5.1. Limitations
AR applications could be a valuable and powerful tool for the visual analysis and communication of emergency egress in the built environment, but it is critical that the virtual world and the real world are seamlessly aligned, and that their connection is sustained throughout the experience. Accurate tracking of the image and registration of objects to that image are fundamental to any successful AR application. Instead of focusing on issues of tracking and possible solutions to that problem, specific limitations associated with occlusion and depth perception in AR are discussed.
Issues of occlusion arise when virtual objects that should be hidden behind real-world objects are superimposed in front of them. For example, if the situated AR simulation is analysing the movement of virtual people down a hallway, any virtual people that are not in that hallway (i.e. are still in an office) should not be visible. If they are visible, it becomes extremely difficult to discern which ones are, and are not, in that hallway. Overcoming occlusion is a major issue for AR, and the solution often lies in the development of controlled environments based on prior knowledge of the real world (Lonergan and Hedley Citation2014). In this case, an invisible 3D model of the building was placed in the VE to match the real-world structure so that any virtual objects behind the invisible walls are not rendered on the AR display. However, what could not be overcome were occlusion issues related to objects not contained within the VE (i.e. garbage cans, benches, and doors). Potential solutions to these occlusion issues lie in experimental technology such as Google’s Tango, where the AR experience does not rely on images or positional information explicitly but uses computer vision for real-time depth-sensing and contextual awareness (Jafri Citation2016). While Tango itself may not have materialized, its depth-sensing approach to positioning runs true in recently launched projects such as Android’s ARCore and Apple’s ARKit.
In the case of the presented AR interfaces that are situated outside of the building, occlusion is not an issue since the objective of the visualization is to reveal the evacuation pathways within that building. Yet through this design, depth perception issues emerge, and it becomes difficult to tell which agent is on which floor, or which agent is on which side of the building. To overcome this problem, agents and their pathways could be colour-coded by floor, or additional functionality could be added to the application that would allow the user to focus on one floor at a time. Another possible solution would be to use location-based AR, freeing the user from the constraints of the image and enabling them to vary their perspective. Current generations of mobile technology allow for precise positioning using GPS and Wi-Fi signals, and researchers have been able to use that functionality to obtain locational accuracy within 4 m (Torres-Sospedra et al. Citation2015). While accurate enough for some spatial applications, a 4 m positional error would have significant alignment implications for these visualizations. Positional accuracy will remain a major challenge for indoor (and outdoor) spatial navigation applications; however, positional accuracy and location-based services should improve with time, eventually allowing the viewer to freely explore virtual information that corresponds exactly with the real-world spaces they occupy.
5.2. Situated AR for egress analysis
The situated AR development presented in this paper represents a step forward by connecting computer-based egress modelling with the real world. The collection of sophisticated algorithms and software currently used for evacuation assessments and building safety classifications do what they were designed to, but the results are without real-world context. The emergency manager that states ‘we do not know what it would look like if everyone was forced to evacuate’ will not find a comprehensive answer in mathematical equations or static visualizations. Recent additions to egress modelling have provided 3D visualization capabilities, but these visualizations remain disconnected from the real world. Situating those simulations in the real world with an AR interface provides a new level of visual representation and analysis, allowing that same emergency manager to begin to understand what that dynamic process would look like in real life.
The purpose of this research was to develop a workflow for situated AR-based analyses of human movement. The context provided by situated AR allows the viewer to compare the movement of people in the VE to the features of the landscape in the real world. Those features are often omitted from evacuation assessments or are accounted for in attribute tables as static features with defined egress impedance values, and this workflow introduces a tool for situated visual assessment of their potential impact on simulated human movement. In the absence of real-life exercises, or actual hazardous events, how is one to know what impact they might have?
5.3. Application of AR to egress analysis
The prototypes presented in this paper illustrate how situated AR could be used for egress analysis, but questions about their applied use and effectiveness remain unanswered. Simply put, just because you can does not mean that you should. The subsequent phases of this research will be focused on pragmatic field testing to assess the application of situated AR to emergency management. That testing will address the ability of these visualizations to improve user cognition and increase risk awareness. Studies on the usefulness of AR for wayfinding have seen mixed results, where the effectiveness is partially determined by personal preference and experience (Dünser et al. Citation2012). The desired outcome is that these tools prove beneficial and supplement the current approach to emergency management, stimulating new methods of visual reasoning in evacuation analyses.
This research, and the development behind this set of AR tools for situated visual analyses of human movement, represents an extension to the authors’ ongoing evacuation simulation research. That research focused on the analysis of human movement using a desktop computer interface and suffers from an inherent dissociation from the real-world spaces of analysis. Research has shown that our reliance on satellite navigation technology has created a spatial disconnection, suggesting that our faith in the output from that technology has left us unaware of our place in space (Speake and Axon Citation2012). These findings could hold true for emergency management, where one has conducted the computer simulations but does not fully comprehend how they relate to real spaces. The goal for situated AR-based analysis is to bridge the gap and improve the user’s ability to comprehend the spatiality of the phenomena by situating the hypothetical in real environments.
6. Conclusion
This paper presented the research and development behind a collection of prototype interfaces for MR-based situated geovisual analyses of virtual human movement in real space and demonstrated select applications of the technology. A selection of tools currently used in emergency management for egress analysis was summarized, and some associated limitations were discussed. Specifically, their limited capacity to capture the complex and dynamic geometries of the built environment that are relevant to emergency egress scenarios, and the generalizations about human movement that result from inaccurate representations of complex built spaces, was highlighted. It was suggested that new, situated 3D geovisual analytical approaches, that dovetail with current GIS workflows, are needed to connect simulated human movement with the complex and dynamic real-world spaces they address.
A series of situated AR-based simulation prototypes were introduced that allow emergency managers to visually assess the simulated movement of building occupants from a building’s exterior, to visualize the potential impact of obstacles on egress, to observe the flow of virtual evacuees down real corridors, and to compare simulated evacuation patterns against the real-world features that influence them. While these prototypes may seem like a novel technique for simulation and analysis, they represent a fundamental change to simulation visualizations by connecting virtual spaces of simulation with the real-world spaces of risk. The capacity to spatially contextualize egress analyses represents the first step towards closing the gap between abstract analyses, real-world cognition, and spatial knowledge transfer of risk. The hope is that the applications presented in this paper serve to influence egress analyses, changing the way simulated human movement is evaluated, communicated, and understood.
Disclosure statement
No potential conflict of interest was reported by the authors.
ORCID
Ian Lochhead http://orcid.org/0000-0002-0832-1154
References
- Bass, William M., and R. Denise Blanchard. 2011. “Examining Geographic Visualization as a Technique for Individual Risk Assessment.” Applied Geography 31 (1): 53–63. doi:10.1016/j.apgeog.2010.03.011.
- Billinghurst, Mark, Adrian Clark, and Gun Lee. 2015. “A Survey of Augmented Reality.” Foundations and Trends® in Human–Computer Interaction, 8:73–272. doi:10.1561/1100000049.
- Cepolina, Elvezia M. 2005. “A Methodology for Defining Building Evacuation Routes.” Civil Engineering and Environmental Systems 22 (1): 29–47. doi:10.1080/10286600500049946.
- Cheng, Kun-Hung, and Chin-Chung Tsai. 2013. “Affordances of Augmented Reality in Science Learning: Suggestions for Future Research.” Journal of Science Education and Technology 22 (4): 449–462. doi:10.1007/s10956-012-9405-9.
- Chiu, Yung Piao, and Yan Chyuan Shiau. 2016. “Study on the Application of Unity Software in Emergency Evacuation Simulation for Elder.” Artificial Life and Robotics 21 (2): 232–238. doi:10.1007/s10015-016-0277-6.
- Cova, Thomas J. 1999. “GIS in Emergency Management.” Geographical Information Systems: Principles, Techniques, Applications, and Management, no. Rejeski 1993: 845–858. http://citeseerx.ist.psu.edu/viewdoc/download?doi=10.1.1.134.9647&rep=rep1&type=pdf.
- Cutter, Susan L. 2003. “GI Science, Disasters, and Emergency Management.” Transactions in GIS 7 (4): 439–445. doi:10.1111/1467-9671.00157.
- Dunleavy, Matt, Chris Dede, and Rebecca Mitchell. 2009. “Affordances and Limitations of Immersive Participatory Augmented Reality Simulations for Teaching and Learning.” Journal of Science Education and Technology 18 (1): 7–22. doi:10.1007/s10956-008-9119-1.
- Dünser, Andreas, Mark Billinghurst, James Wen, Ville Lehtinen, and Antti Nurminen. 2012. “Exploring the Use of Handheld AR for Outdoor Navigation.” Computers & Graphics 36 (8): 1084–1095. doi:10.1016/j.cag.2012.10.001.
- Eaglin, Todd, Kalpathi Subramanian, and Jamie Payton. 2013. “3D Modeling by the Masses: A Mobile App for Modeling Buildings.” 2013 IEEE International Conference on Pervasive Computing and Communications Workshops, PerCom Workshops 2013, 315–317.
- Elsayed, Neven A M, Bruce H Thomas, Kim Marriott, Julia Piantadosi, and Ross T Smith. 2016. “Situated Analytics : Demonstrating Immersive Analytical Tools with Augmented Reality.” Journal of Visual Language and Computing 36: 13–23. doi:10.1016/j.jvlc.2016.07.006.
- Emergency Management British Columbia. 2011. “Emergency Management in BC : Reference Manual.”
- Gröger, Gerhard, and Lutz Plümer. 2012. “CityGML – Interoperable Semantic 3D City Models.” ISPRS Journal of Photogrammetry and Remote Sensing 71: 12–33. doi:10.1016/j.isprsjprs.2012.04.004.
- Gwynne, S. M V. 2012. “Translating Behavioral Theory of Human Response into Modeling Practice.” National Institute of Standards and Technology. Gaithersburg, MD, 69. doi:10.6028/NIST.GCR.972.
- Gwynne, S. M V, L. M. Hulse, and M. J. Kinsey. 2016. “Guidance for the Model Developer on Representing Human Behavior in Egress Models.” Fire Technology 52 (3): 775–800. doi:10.1007/s10694-015-0501-2.
- Hedley, Nick. 2008. “Real-Time Reification: How Mobile Augmented Reality May Change Our Relationship with Geographic Space.” Proceedings of the 2nd International Symposium on Geospatial Mixed Reality, 28–29 August, Laval University, Quebec City, Quebec, Canada. http://regard.crg.ulaval.ca/index.php?id=1.
- Hedley, Nick. 2017. “Augmented Reality.” International Encyclopedia of Geography, edited by Douglas Richardson, Noel Castree, Michael F. Goodchiild, Audrey Kobayashi, Weidong Liu, and Richard A. Marston. Wiley. doi:10.1201/9780849375477.ch213.
- Hedley, Nicholas R., Mark Billinghurst, Lori Postner, Richard May, and Hirokazu Kato. 2002. “Explorations in the Use of Augmented Reality for Geographic Visualization.” Presence: Teleoperators and Virtual Environments 11 (2): 119–133. doi:10.1162/1054746021470577.
- Hedley, N., and C. Chan. 2012. "Using Situated Citizen Sampling, Augmented Geographic Spaces and Geospatial Game Design to Improve Resilience in Real Communities at Risk from Tsunami Hazards." International society for photogrammetry and remote sensing (ISPRS) XXII International Congress 2012, 25 Aug–1 Sept, 2012, Melbourne, Australia.
- Isikdag, Umit. 2015. “BIM and IoT: A Synopsis from GIS Perspective.” ISPRS – International Archives of the Photogrammetry, Remote Sensing and Spatial Information Sciences, XL-2/W4:33–38. doi:10.5194/isprsarchives-XL-2-W4-33-2015.
- Isikdag, Umit, Sisi Zlatanova, and Jason Underwood. 2013. “A BIM-Oriented Model for Supporting Indoor Navigation Requirements.” Computers, Environment and Urban Systems 41. 112–123. doi:10.1016/j.compenvurbsys.2013.05.001.
- Jafri, Rabia. 2016. “A GPU-Accelerated Real-Time Contextual Awareness Application for the Visually Impaired on Google’s Project Tango Device.” Journal of Supercomputing 73 (2). 1–13. doi:10.1007/s11227-016-1891-8.
- Kim, W., N. Kerle, and M. Gerke. 2016. “Mobile Augmented Reality in Support of Building Damage and Safety Assessment.” Natural Hazards and Earth System Sciences 16 (1). 287–298. doi:10.5194/nhess-16-287-2016.
- Koua, E. L., A. Maceachren, and M.-J. Kraak. 2006. “Evaluating the Usability of Visualization Methods in an Exploratory Geovisualization Environment.” International Journal of Geographical Information Science 20 (4): 425–448. doi:10.1080/13658810600607550.
- Kraak, Menno-Jan, and Alan M. MacEachren. 2005. “Geovisualization and GIScience.” Cartography and Geographic Information Science 32 (2): 67–68. doi:10.1559/1523040053722123.
- Kuligowski, Erica D., Richard D. Peacock, and Bryan L. Hoskins. 2010. “A Review of Building Evacuation Models; 2nd Edition.” NIST Technical Note 1680. doi:10.6028/NIST.TN.1471.
- Lin, Hui, Min Chen, Guonian Lu, Hui Lin, Min Chen, and Guonian Lu. 2013. “Virtual Geographic Environment: A Workspace for Computer-Aided Geographic Experiments.” Annals of the Association of American Geographers 103 (3): 465–482. doi:10.1080/00045608.2012.689234.
- Liu, Xin, Xiangyu Wang, Graeme Wright, Jack Cheng, Xiao Li, and Rui Liu. 2017. “A State-of-the-Art Review on the Integration of Building Information Modeling (BIM) and Geographic Information System (GIS).” ISPRS International Journal of Geo-Information 6 (2): 53. doi:10.3390/ijgi6020053.
- Lochhead, Ian M, and Nick Hedley. 2017. “Modeling Evacuation in Institutional Space: Linking Three-Dimensional Data Capture, Simulation, Analysis, and Visualization Workflows for Risk Assessment and Communication.” Information Visualization. doi:10.1177/1473871617720811.
- Lonergan, Chris, and Nick Hedley. 2014. “Flexible Mixed Reality and Situated Simulation as Emerging Forms of Geovisualization.” Cartographica: The International Journal for Geographic Information and Geovisualization 49 (3). 175–187. https://muse-jhu-edu.proxy.lib.sfu.ca/journals/cartographica/v049/49.3.lonergan.html. doi: 10.3138/carto.49.3.2440
- Lonergan, Chris, and Nicholas Hedley. 2015. “Navigating the Future of Tsunami Risk Communication: Using Dimensionality, Interactivity and Situatedness to Interface with Society.” Natural Hazards 78 (1). 179–201. doi:10.1007/s11069-015-1709-7.
- MacEachren, Alan M., and Menno-Jan Kraak. 2001. “Research Challenges in Geovisualization.” Cartography and Geographic Information Science 28 (1): 3–12. doi:10.1559/152304001782173970.
- Meng, Fanxing, and Wei Zhang. 2014. “Way-Finding During a Fire Emergency: An Experimental Study in a Virtual Environment.” Ergonomics 57 (6): 816–827. doi:10.1080/00140139.2014.904006.
- Milgram, P, H Takemura, A Utsumi, and F Kishino. 1994. “Augmented Reality: A Class of Displays on the Reality-Virtuality Continuum.” SPIE 2351 (Telemanipulator and Telepresence Technologies):282–292. doi: 10.1.1.83.6861.
- Ntuen, Celestine A., Eui H. Park, and Kim Gwang-Myung. 2010. “Designing an Information Visualization Tool for Sensemaking.” International Journal of Human-Computer Interaction 26 (2–3): 189–205. doi:10.1080/10447310903498825.
- Nunes, Nuno, Kimberly Roberson, and Alfredo Zamudio. 2014. “The MEND Guide. Comprehensive Guide for Planning Mass Evacuations in Natural Disasters. Pilot Document.”
- Paelke, Volker, and Monika Sester. 2010. “Augmented Paper Maps: Exploring the Design Space of a Mixed Reality System.” ISPRS Journal of Photogrammetry and Remote Sensing 65 (3): 256–265. doi:10.1016/j.isprsjprs.2009.05.006.
- Pike, William a, John Stasko, Remco Chang, and Theresa a O Connell. 2009. “The Science of Interaction.” Information Visualization 8 (4). 263–274. doi:10.1057/ivs.2009.22.
- Public Safety Canada. 2011. “An Emergency Management Framework for Canada.” Ottawa.
- Ribeiro, João, João Emílio Almeida, Rosaldo J. F. Rossetti, António Coelho, and António Leça Coelho. 2012. “Towards a Serious Games Evacuation Simulator.” 26th European conference on modelling and simulation ECMS 2012, Koblenz, Germany, 697–702.
- Rinne, Tuomo, Kati Tillander, and Peter Grönberg. 2010. “Data Collection and Analysis of Evacuation Situations.” VTT Tiedotteita – Valtion Teknillinen Tutkimuskeskus.
- Ronchi, Enrico, and Daniel Nilsson. 2013. “Fire Evacuation in High-Rise Buildings : A Review of Human Behaviour and Modelling Research.” Fire Science Reviews 2 (7): 1–21. doi:10.1186/2193-0414-2-7.
- Schall, Gerhard, Erick Mendez, Ernst Kruijff, Eduardo Veas, Sebastian Junghanns, Bernhard Reitinger, and Dieter Schmalstieg. 2009. “Handheld Augmented Reality for Underground Infrastructure Visualization.” Personal and Ubiquitous Computing 13 (4): 281–291. doi:10.1007/s00779-008-0204-5.
- Shelton, Brett E., and Nicholas R. Hedley. 2004. “Exploring a Cognitive Basis for Learning Spatial Relationships with Augmented Reality.” Technology, Instruction, Cognition and Learning 1: 323–357. http://digitalcommons.usu.edu/itls_facpub/92/.
- Slocum, Terry A., Connie Blok, Bin Jiang, Alexandra Koussoulakou, Daniel R. Montello, Sven Fuhrmann, and Nicholas R. Hedley. 2001. “Cognitive and Usability Issues in Geovisualization.” Cartography and Geographic Information Science 28 (1): 61–75. doi:10.1559/152304001782173998.
- Smith, Shana, and Emily Ericson. 2009. “Using Immersive Game-Based Virtual Reality to Teach Fire-Safety Skills to Children.” Virtual Reality 13 (2): 87–99. doi:10.1007/s10055-009-0113-6.
- Speake, Janet, and Stephen Axon. 2012. “‘I Never Use “Maps” Anymore’: Engaging with Sat Nav Technologies and the Implications for Cartographic Literacy and Spatial Awareness.” The Cartographic Journal 49 (4): 326–336. doi:10.1179/1743277412Y.0000000021.
- Tang, Fangqin, and Aizhu Ren. 2012. “GIS-Based 3D Evacuation Simulation for Indoor Fire.” Building and Environment 49 (March): 193–202. doi:10.1016/j.buildenv.2011.09.021.
- Tang, Chieh-Hsin, Wu-Tai Wu, and Ching-Yuan Lin. 2009. “Using Virtual Reality to Determine How Emergency Signs Facilitate Way-Finding.” Applied Ergonomics 40 (4): 722–730. doi:10.1016/j.apergo.2008.06.009.
- Thill, Jean Claude, Thi Hong Diep Dao, and Yuhong Zhou. 2011. “Traveling in the Three-Dimensional City: Applications in Route Planning, Accessibility Assessment, Location Analysis and Beyond.” Journal of Transport Geography 19 (3): 405–421. doi:10.1016/j.jtrangeo.2010.11.007.
- Torrens, Paul M. 2015. “Slipstreaming Human Geosimulation in Virtual Geographic Environments.” Annals of GIS 21 (March): 325–344. doi:10.1080/19475683.2015.1009489.
- Torres-Sospedra, Joaquín, Joan Avariento, David Rambla, Raúl Montoliu, Sven Casteleyn, Mauri Benedito-Bordonau, Michael Gould, and Joaquín Huerta. 2015. “Enhancing Integrated Indoor/Outdoor Mobility in a Smart Campus.” International Journal of Geographical Information Science 8816 (July): 1–14. doi:10.1080/13658816.2015.1049541.
- Tsai, Ming-Kuan, Yung-Ching Lee, Chung-Hsin Lu, Mei-Hsin Chen, Tien-Yin Chou, and Nie-Jia Yau. 2012. “Integrating Geographical Information and Augmented Reality Techniques for Mobile Escape Guidelines on Nuclear Accident Sites.” Journal of Environmental Radioactivity 109 (July): 36–44. doi:10.1016/j.jenvrad.2011.12.025.
- Tsai, Ming-Kuan, and Nie-Jia Yau. 2013. “Enhancing Usability of Augmented-Reality-Based Mobile Escape Guidelines for Radioactive Accidents.” Journal of Environmental Radioactivity 118 (April): 15–20. doi:10.1016/j.jenvrad.2012.11.001.
- Wilson, Robert D, and Brandon Cales. 2008. “Geographic Information Systems, Evacuation Planning and Execution.” Communications of the IIMA 8 (4): 13–30. doi:10.1108/17506200710779521.
- Woolard, A., V. Lalioti, N. Hedley, J. Julien, M. Hammond, and N. Carrigan. 2003. “Using ARToolKit to Prototype Future Entertainment Scenarios.” ART 2003 – IEEE International Augmented Reality Toolkit Workshop, 69–70. doi:10.1109/ART.2003.1320430.
- Wright, John K. 1942. “Map Makers Are Human: Comments on the Subjective in Maps.” Geographical Review 32 (4): 527–544. doi: 10.2307/209994
- Wu, Chenshu, Zheng Yang, Yu Xu, Yiyang Zhao, and Yunhao Liu. 2015. “Human Mobility Enhances Global Positioning Accuracy for Mobile Phone Localization.” IEEE Transactions on Parallel and Distributed Systems 26 (1): 131–141. doi:10.1109/TPDS.2014.2308225.
- Zhou, Yuhong, Thi Hong Diep Dao, Jean Claude Thill, and Eric Delmelle. 2015. “Enhanced 3D Visualization Techniques in Support of Indoor Location Planning.” Computers, Environment and Urban Systems 50. Elsevier Ltd:15–29. doi:10.1016/j.compenvurbsys.2014.10.003.
- Zlatanova, Sisi, George Sithole, Masafumi Nakagawa, and Qing Zhu. 2013. “Problems in Indoor Mapping and Modelling.” ISPRS – International Archives of the Photogrammetry, Remote Sensing and Spatial Information Sciences, XL-4/W4:63–68. doi:10.5194/isprsarchives-XL-4-W4-63-2013.