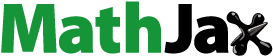
ABSTRACT
Shrub encroachment into arid and semi-arid grasslands has elicited extensive research attention worldwide under the background of climate change and increasing anthropogenic activities. Shrub encroachment may considerably impact local ecosystems and economies, including the conversion of the structure and function of ecosystems, the shift in ambient conditions, and the weakness of local stock farming capacity. This article reviews recent research progresses on the shrub encroachment process and mechanism, shrub identification and dynamic monitoring using remote sensing, and modeling and simulation of the shrub encroachment process and dynamics. These studies can help to evaluate the ecological effect of shrub encroachment, and thus, practically manage and recover the ecological environment of degraded areas. However, the lack of effective measures and data for monitoring shrub encroachment at a large spatial scale severely limits research on the mechanism, modeling, and simulation of shrub encroachment, and the shrub encroachment stages can hardly be quantitatively defined, resulting in insufficient analysis and simulation of shrub encroachment for different spatiotemporal scales and stages shift. Improvement in remote sensing-based shrub encroachment dynamic monitoring might be crucial for analyzing and understanding the process and mechanism of shrub encroachment, and multi-disciplinary and multi-partnerships are required in the shrub encroachment studies.
1. Introduction
Grasslands are among the most important types of global vegetation land cover, occupying over 40% of the world's terrestrial area, excluding Greenland and Antarctica (Suttie, Reynolds, and Batello Citation2005). Over 50% of grasslands worldwide experience different stages of degradation under the background of climate change and increasing anthropogenic activities (White, Murray, and Rohweder Citation2000). Among these degradation stages, shrub encroachment (also called shrub invasion or woody thicketization) in arid and semi-arid grasslands and savannas has elicited extensive attention (Archer, Schimel, and Holland Citation1995; Eldridge et al. Citation2011; Naito and Cairns Citation2011). Shrub encroachment has been occurring worldwide, including in the savannas of southern Africa (Levick and Rogers Citation2011), the arid grasslands of Australia (Robinson, van Klinken, and Metternicht Citation2008), the rangelands of southern Argentina (Cipriotti and Aguiar Citation2012), the dry grasslands of the western United States (Goslee et al. Citation2003; McGlynn and Okin Citation2006), the Mediterranean regions (Maestre et al. Citation2009), the grasslands of Inner Mongolia in China (Zhou Citation1990; Tong et al. Citation2001; Xiong et al. Citation2003; Peng et al. Citation2013; Peng, Li, and Tong Citation2013; Zhang et al. Citation2017), and the alpine grassland of the Qinghai–Tibet Plateau (Zhang et al. Citation2006; Wang et al. Citation2013).
Shrub encroachment into arid and semi-arid grasslands can be observed through the increase in the density, coverage, and biomass of shrubs or woody plants (Van Auken Citation2009), which significantly impacts local ecosystems. Shrub encroachment results in the shift from a herbaceous-dominant ecosystem to a shrub-dominant ecosystem, along with changes in the ecological structure and function of an ecosystem, including vegetation productivity, biological diversity, carbon storage, and flux (Briggs, Blair, and Mccarron Citation2005; Eldridge et al. Citation2011; Peng, Li, and Tong Citation2013). The increase of shrubs in grasslands may alter the conditions of the environment, such as the physical structures and chemical properties of soil (Archer, Schimel, and Holland Citation1995; Zhang, Shi, and Zheng Citation2001; Li and Gao Citation2012) and the re-allocation of water (Peng, Li, and Tong Citation2014). These changes in ambient conditions may facilitate the growth of shrubs, form a positive feedback effect that can make the shrub encroachment process irreversible (Archer, Schimel, and Holland Citation1995; Scheffer and Carpenter Citation2003), and finally lead to the formation of sand dune shrublands (Xiong et al. Citation2003) or desertification (Kéfi et al. Citation2007). Shrub encroachment lowers the biomass of edible pasture, thereby decreasing stock farming capacity, which affects the local economy (Naito and Cairns Citation2011). Therefore, research on shrub encroachment into arid and semi-arid grasslands is important to improve the understanding of the response and adaptation of grassland ecosystems to climate change and anthropogenic activities. Such research can help properly evaluate the ecological effect of shrub encroachment, and thus, practically manage and recover the ecological environment of degraded areas.
Current studies have identified the main factors that induce shrub encroachment into arid and semi-arid grasslands, such as regional and global climate change (Tietjen et al. Citation2010), increasing atmospheric CO2 concentration (Mooney and Cleland Citation2001), decreasing wildfire frequency (Roques, O’Connor, and Watkinson Citation2001; Lohmann et al. Citation2014), and increasing grazing pressure (Van Auken Citation2009). These exogenic factors and feedback mechanisms of an ecosystem (D’Odorico et al. Citation2010) mutually affect the process and status of shrub encroachment. However, debates continue on the scales, ranges, and relations of impact factors (D’Odorico, Okin, and Bestelmeyer Citation2012). The research on the shrub encroachment mechanism requires the long-term and dynamic monitoring of the shrub encroachment process (Roques, O’Connor, and Watkinson Citation2001; Van Auken Citation2009). Long-term monitoring methods include field observation within a limited region (Cipriotti and Aguiar Citation2012) and the dynamic interpretation of historic aerial photos (Goslee et al. Citation2003) or high spatial resolution (HSR) remote sensing images (Laliberte et al. Citation2004). At the regional scale or within a large geological range, moderate or coarse spatial resolution remote sensing data are useful sources for monitoring the shrub encroachment process and dynamics. Existing methods include vegetation indices (Xu et al. Citation2010), spectral mixture analysis (Sohn and McCoy Citation1997), multi-temporal or time series data analysis (Wilfong, Gorchov, and Henry Citation2009; Zhou et al. Citation2013), and multi-angle remote sensing (Chopping et al. Citation2006, Citation2008). However, accurately recognizing shrub and estimating its coverage from grass–shrub–soil mixture pixels are difficult due to the high similarity between the spectra of grass and shrub. The lack of effective measures and data for monitoring shrub encroachment at a large spatial scale severely limits research on the mechanism, modeling, and simulation of shrub encroachment. The improvement of shrub extraction, inversion, and monitoring methods, along with providing long-term and large spatial range shrub dynamic data, can help analyze the effects of various factors at different spatiotemporal scales. They can also help model and simulate the dynamics of the shrub encroachment process.
This article reviews recent progresses in the research on the shrub encroachment process and mechanism (Section 2), shrub identification and dynamic monitoring using remote sensing (Section 3), and modeling and simulation of the shrub encroachment processes and dynamics (Section 4). Several conclusions are drawn in Section 5.
2. Process and mechanisms of shrub encroachment
2.1. Stages of shrub encroachment
Shrub encroachment into grassland indicates a shift from the stable landscape state dominated by grass to another state dominated by shrubs (Anderies, Janssen, and Walker Citation2002; van Langevelde et al. Citation2003; Okin, D’Odorico, and Archer Citation2009). The shrub encroachment process can be observed through several demographic changes (D’Odorico, Okin, and Bestelmeyer Citation2012). Thus, researchers have divided this process into stages or phases. Xiong, Han, and Bao (Citation2005) proposed three states of the shrub encroachment process based on the steady states of an ecosystem. State 1 or pristine grassland is identified as potential sandy desertification. It is dominated by perennial gramineous grasses, and the ecological function of shrub patches is weaker than the grass matrix. The main grass species are native high gramineous grasses inside and outside shrub patches. State 2 or thicketization grassland is identified as light sandy desertification. It is dominated by shrub patches that consist of shrubs and understory grasses. The main grass species are native high gramineous grasses inside shrub patches and small gramineous grasses or weed outside shrub patches. The ecological function of shrub patches is enhanced in the ecosystem during this state. State 3 or dune shrubland is characterized by heavy sandy desertification. Coppice dunes or nebkhas develop only on sandy soil, thereby indicating severe grassland degradation, soil erosion, and desertification. No continuous grass layer exists within shrub patches, and the basic ecological function of the ecosystem tends to disappear. The shift between states relies on ecological thresholds. The crossing of an ecological threshold indicates changes in the original ecosystem structure and the loss of the corresponding ecological functions, which suggests the overall degradation of the ecosystem (Xiong and Han Citation2005). D’Odorico, Okin, and Bestelmeyer (Citation2012) divided the shrub encroachment process into four stages: (I) pure grassland with nearly no shrub, (II) grassland with limited shrub cover, (III) shrubland where most grass cover is absent and shrubs have increased in size and density, and (IV) shrub duneland. Stages II–IV can correspond to Stages 1–3 of Xiong, Han, and Bao (Citation2005). shows the stages of Caragana microphylla Lam. encroachment in a steppe in Inner Mongolia, China based on the four stages proposed by D’Odorico, Okin, and Bestelmeyer (Citation2012).
Figure 1. Four stages of C. microphylla Lam. encroachment into steppe in Inner Mongolia, China: (a) Stage I, (b) Stage II, (c) Stage III, and (d) Stage IV. (Photos taken by X. Cao in 2015–2016)
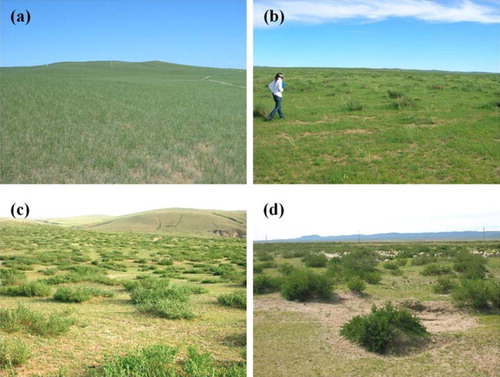
The determination of the states or stages of shrub encroachment is dependent on the experience or knowledge of ecologists rather than on quantitative measures or indicators. However, the relationship between the size and number of shrub patches can show the change in landscape pattern, which may be an ‘indicator’ of ecosystem response to climate change or human activities (Rietkerk et al. Citation2004; Kéfi et al. Citation2007; Scheffer et al. Citation2009). The size and number of vegetation patches were observed as a power law in southern Africa (Scanlon et al. Citation2007) and a truncated power law in Mediterranean arid ecosystems (Kéfi et al. Citation2007). Increasing grazing pressure may lead to a deviation from the power law (Kéfi et al. Citation2007). In a typical steppe region in the Xilin River Basin, Inner Mongolia, grassland degradation caused by overgrazing finally resulted in C. microphylla patches that dominated the shrub-thicketizated grassland (Xiong and Han Citation2006). The landscape pattern of shrub patches in grasslands can help to reflect the stages or phases of shrub encroachment by power law.
2.2. Mechanisms of shrub encroachment
Understanding the mechanism of shrub encroachment is of considerable importance for managing and protecting arid grassland ecosystems (Briggs, Blair, and Mccarron Citation2005). Belayneh and Tessema (Citation2017) summarized six theories for the mechanisms of shrub encroachment in semi-arid African ecosystems, including two-layer model, one-layer model, state-and-transition, equilibrium, disequilibrium, and non-equilibrium rangeland theories. The two-layer model (Walter Citation1939) uses moisture in top and lower layers of soil to describe the shift in competitive advantage from grass species to trees or shrubs, because heavy grazing pressure in dryland decreases grass cover and soil moisture becomes available to the trees or shrubs. The one-lay model (Adams Citation1967) states that grass fires can result in a constant nitrogen loss and limit grass growth, while benefit Acacia bushes due to its capacity of nitrogen-fixing. The state-and-transition model describes the rangelands in dryland environments as ecosystems with stable states, and transitions between stable states can be initiated by influence of biotic and abiotic factors (Westoby, Walker, and Noy-Meir Citation1989; Briske, Fuhlendorf, and Smeins Citation2003; van Langevelde et al. Citation2003). The equilibrium, disequilibrium, and non-equilibrium rangeland theories describes three types of ecosystems which are characterized by one stable state, two stable states and a fluctuation in tree-grass balance, respectively (Briske, Fuhlendorf, and Smeins Citation2003; Sankaran, Ratnam, and Hanan Citation2004; Sankaran et al. Citation2005), while the causes of shrub encroachment in these ecosystems may be different.
D’Odorico, Okin, and Bestelmeyer (Citation2012) categorized the mechanisms that drive shrub encroachment into exogenic or endogenic factors. Exogenic mechanisms determine the shift in competitive advantage from grassland to shrubland (Archer, Schimel, and Holland Citation1995). The factors associated with exogenic mechanisms involve regional and global warming, increasing atmospheric CO2 concentration, large-scale fire control, and increasing grazing pressure. A low temperature may damage shrub seedling, whereas an increasing temperature induced by global warming can decrease the mortality of shrub shoots and foster shrub growth (Tietjen et al. Citation2010). The water use efficiency of C4 plants (mainly herbaceous plants) is higher than that of C3 plants (shrubs), whereas increasing CO2 concentration can enhance the water use efficiency of C3 plants and reduce the competitive advantage of C4 plants (Mooney and Cleland Citation2001). A wildfire can eliminate shrub shoots and reduce shrub regeneration. However, long-term and large-scale fire suppression in rangeland and grazing-induced fuel reduction will decrease fire frequency and enhance shrub survival rate (Roques, O’Connor, and Watkinson Citation2001; Xiong et al. Citation2003; Lohmann et al. Citation2014). Increasing grazing activities or overgrazing will accrete grazing by livestock, cut down grass cover (van de Koppel et al. Citation2002; Xiong et al. Citation2003), and promote the diffusion of shrub seeds (Archer Citation1989), which will also result in shrub encroachment (Van Auken Citation2009). Shrub encroachment may be the consequence of a combination of factors that are difficult to separate (Van Auken Citation2009).
Endogenic mechanisms include ecosystem feedback that may create bistability (D’Odorico, Okin, and Bestelmeyer Citation2012). A state shift in bistable ecosystem dynamics can be explained by four positive feedback processes, namely, vegetation–microclimate feedback, fire–vegetation feedback, soil erosion–vegetation feedback, and grazing of small mammals–vegetation cover feedback (). At the landscape scale to meso-scale, shrub encroachment enhances the proportion of bare soil, thereby increasing diurnal soil heat fluxes and nocturnal upward long-wave radiation. These changes will modify microclimate conditions with an increase in near-surface air temperature and benefit shrub survival (D’Odorico et al. Citation2010). At the landscape scale, fire is sustained by contiguous fuels (dry grass); periodic fires kill shrub seedlings and prevent shrubs from expanding (Roques, O’Connor, and Watkinson Citation2001). Fire management will decrease fire frequency and disrupt the fire–grass feedback, thereby leading to shrub expansion. At the patch scale, erosion–vegetation feedback operates in an environment where grasses exhibit competitive advantage, whereas shrubs are more likely to obtain soil and water resources. High grass cover prevents resource redistribution by wind and water erosion, improves water infiltration, and maintains grass production. Surface soil resources are depleted and redistributed to shrubs when grass coverage is lost (Schlesinger et al. Citation1990; Zhang, Shi, and Zheng Citation2001; Xiong and Han Citation2005; Han et al. Citation2014). The small mammals–vegetation cover feedback tends to increase the ratio of shrub to grass cover because shrub patches provide nests and shelters for small mammals and help them nibble on grass roots (Kerley and Whitford Citation2009).
Figure 2. Positive feedback for shrub–grass bistable state shift at different scales (adapted from (D’Odorico et al. Citation2010)).
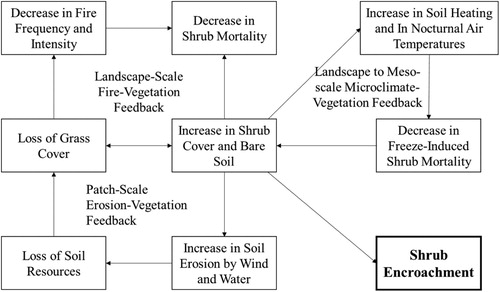
Certain problems remain in the mechanism analysis of shrub encroachment. Factors that lead to shrub encroachment are controversial in terms of scale, size, and interactions (D’Odorico, Okin, and Bestelmeyer Citation2012). Studies on the mechanism of shrub encroachment are mostly aimed at a specific scale rather than at multiple scales (Goslee et al. Citation2003; Laliberte et al. Citation2004; Briggs, Blair, and Mccarron Citation2005; Robinson, van Klinken, and Metternicht Citation2008; Levick and Rogers Citation2011). However, shrub encroachment is likely due to multiple factors and their interactions at multiple scales (Archer, Schimel, and Holland Citation1995; Peters et al. Citation2006; Belayneh and Tessema Citation2017). During different stages of shrub encroachment, influencing factors may vary (D’Odorico, Okin, and Bestelmeyer Citation2012), and no relevant research has yet been conducted on the stage classification and mechanism analysis of shrub encroachment at different stages. Only a few studies have addressed the quantitative analysis of the influencing factors of shrub encroachment due to the lack of a wide range of dynamic monitoring data. For example, Roques, O’Connor, and Watkinson (Citation2001) utilized aerial photographs taken from 1947 to 1990 and survey data from 1997 to study shrub encroachment in the lowland meadows of Swaziland using the multivariate regression analysis method. The influencing factors included fire frequency, grazing pressure, precipitation, soil type, and initial coverage of shrubs. Therefore, the long-term, large-scale, and multi-scale observations of shrubs and encroaching processes are the bases for studying the shrub encroachment mechanism.
3. Shrub identification and encroachment dynamic monitoring with remote sensing data
The spatiotemporal monitoring of shrub encroachment is the primary requirement for shrub encroachment research. Long-term field observations or in situ experiments are the traditional measures for studying shrub invasion or grassland degradation. However, these methods are constrained by intensive labor, high cost, limited spatial and temporal ranges, and difficulty in explaining spatial heterogeneity at a large spatial scale (Naito and Cairns Citation2011). In recent years, aerial photos and remote sensing data have been widely used to monitor shrub encroachment in grasslands, and various methods have been developed to identify shrubs in grass–shrub–soil co-existing landscapes. We summarized remote sensing-based techniques according to the spatial resolutions of data. The methods developed for identifying shrub and estimating its coverage were also introduced.
3.1. HSR data
Very high spatial resolution (VHSR) remote sensing images are preferred for detecting shrubs in grasslands and monitoring their dynamics. HSR data include satellite images, such as those taken by IKONOS, QuickBird and WorldView; aerial and unmanned aerial vehicle (UAV) photographs; light detection and ranging (LiDAR) data; and airborne hyperspectral data. shows the shapes of shrubs (C. microphylla Lam. in a steppe in Inner Mongolia, China) on the ground and in HSR images. In the VHSR remote sensing images (< 4 m), shrubs typically present circular or elliptical shapes with different sizes, which are clearly distinguished from background herbaceous plants and soil. Methods for identifying shrubs include human interpretation (Briggs, Blair, and Mccarron Citation2005; Xu and Li Citation2009), unsupervised classification (Frank and Tweddale Citation2006; Robinson, van Klinken, and Metternicht Citation2008), and object-oriented classification (Laliberte et al. Citation2004; Levick and Rogers Citation2011).
Figure 3. Shapes of C. microphylla Lam. patches at different observation scales: (a) field observation on the ground, (b) UAV image with a resolution of 0.08 m, (c) Google Earth image with a resolution of approximately 0.3 m; and (d) WorldView-2 multispectral fusion image with a resolution of 0.5 m.
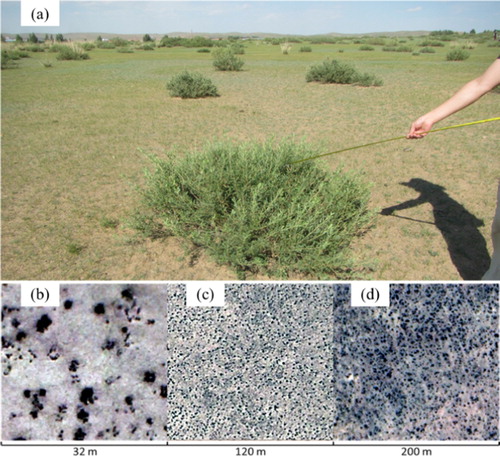
Earlier photos are mostly panchromatic; hence, the typical method for extracting shrub individuals or patches is human interpretation or threshold selection to extract the darkest areas (Hutchinson, Unruh, and Bahre Citation2000; Lahav-Ginott, Kadmon, and Gersani Citation2001). To avoid errors that might arise from the single thresholding of a panchromatic HSR image, Goslee et al. (Citation2003) manually marked the center points of all shrub patches and then adopted an iterative algorithm to expand outward from the marked point until an edge difference value was reached. This parameter was calculated based on the contrast between dark shrub canopies and lighter background areas in each image. Through this method, Goslee et al. (Citation2003) extracted the population dynamics and spatial pattern of all shrubs (Prosopis glandulosa) with a diameter greater than 2 m in the Chihuahuan Desert, southern New Mexico by using 10 high-resolution aerial and satellite images taken from 1936 to 1996. For multispectral or colored HSR images, object-oriented classification can improve the accuracy of shrub extraction. Laliberte et al. (Citation2004) used image segmentation and object-based classification to monitor shrub changes from 1937 to 1996 in the Jornada Basin of southern New Mexico based on aerial photos taken during this period and QuickBird images from 2003. Both of these studies found an increase in the shrub coverage of the desert grasslands in southern New Mexico. Robinson, van Klinken, and Metternicht (Citation2008) collected aerial photos from 1943 to 2004 in the semi-arid grassland of western Australia and used an iterative self-organizing clustering procedure (ISODATA) to extract patches and monitor dynamics of invasive mesquite trees and shrubs. Garrity et al. (Citation2008) used spatial wavelet analysis to automatically detect the location and crown diameter of shrub individuals ranging from 0.25 to 1 m in HSR multispectral images. Shrubs are frequently higher than background soil and herbaceous species, and thus, LiDAR data can provide information that is useful for identifying shrubs and estimating their height and structure (Levick and Rogers Citation2011). Hellesen and Matikainen (Citation2013) combined LiDAR data with a point density of 13 points/m2 and color infrared orthoimages with a spatial resolution of 0.5 m; they then used an object-oriented classification and regression tree classifier to extract shrubs and trees. Their results indicated that using LiDAR data could considerably increase the accuracy of shrub and tree extraction in complex environments in Denmark.
HSR images can be used directly to identify shrubs from background grassland and to monitor shrub population and coverage at the individual and patch scales. However, HSR data are characterized by high costs for covering large regions and historical data are lacking in many regions. Therefore, HSR data are difficult to use in large-area shrub mapping and dynamic shrub monitoring. HSR images may miss shrubs that are smaller than the spatial discriminability of the data (Frank and Tweddale Citation2006). In many studies, HSR images are only used as references or validation data for large-area shrub monitoring and mapping (Chopping et al. Citation2006; Olthof and Fraser Citation2007; Selkowitz Citation2010).
3.2. Moderate to coarse spatial resolution data
In shrub encroachment research or dynamic monitoring at the regional scale, moderate to coarse spatial resolution (30 m–1 km) data are frequently used to identify shrubs and estimate their coverage. These data include images taken by the Systeme Probatoire d’Observation de la Terre (SPOT) (Duncan et al. Citation1993), Landsat Thematic Mapper (TM) (Olthof and Fraser Citation2007; Selkowitz Citation2010), moderate-resolution imaging spectroradiometer (MODIS) (Selkowitz Citation2010), and multi-angle imaging spectroradiometer (MISR) (Chopping et al. Citation2008). Synthetic aperture radar (SAR) data, such as Advanced Land Observing Satellite (ALOS) Phased Array Type L-Band SAR (PALSAR) (Mitchard et al. Citation2009, Citation2011; Skowno et al. Citation2017), are also used to monitor woodland expansion in grasslands in central and southern Africa. These technologies include spectral mixture analysis (SMA) for multi-spectral data, phenology or sensitivity analysis for multi-temporal data, and geometric-optical (GO) modeling for multi-angle data.
3.2.1. SMA for multi-spectral data
SMA or spectral unmixing can provide land cover information at subpixel levels for practical applications. The concept of SMA is to resolve a linear or nonlinear combination of mixed spectrum through its constituent spectral components or endmembers and to obtain their subpixel fractional cover (Somers et al. Citation2011). Linear SMA (LSMA) assumes that a landscape is composed by several materials on the ground, i.e. endmembers, and that each incident photon interacts with only a single pixel component, thereby disregarding multiple scattering among endmembers (Camacho-De Coca et al. Citation2004; Song Citation2005). LSMA can linearly model the mixed spectral information and estimate the fraction of each endmember. LSMA has been extensively used in estimating vegetation coverage (Chen et al. Citation2001; Small Citation2001; Wu and Murray Citation2003; Niu, Liu, and Wang Citation2005; Pan and Li Citation2010). Sohn and McCoy (Citation1997) simulated endmember spectra in a desert grassland environment at the TM pixel scale by using measured spectra and applied LSMA and the constrained least squares method to obtain shrub coverage.
The SMA model exhibits several advantages over conventional empirical models for estimating vegetation coverage. This model can build multiple representative endmembers to simulate various backgrounds, such as soil and dry grass, in a landscape with low vegetation coverage, thereby enhancing the accuracy of shrub coverage estimation. Empirical models that suffer from background noise can barely provide an accurate estimation (Henry and Hope Citation1998; Rogan and Franklin Citation2001). The SMA model can be independent of field measurement if pure pixels are found in a remote sensing image and used as endmembers (Vila and Barbosa Citation2010; Veraverbeke et al. Citation2012). However, the number of endmembers are constrained by the number of spectral bands in remote sensing data, e.g. a maximum of four endmembers are generally recommended for Landsat TM/ETM+ data (Theseira, Thomas, and Sannier Citation2002). In a shrub and grass mixed landscape, SMA may encounter a collinearity problem because of the spectral similarity between shrub and grass endmembers (Chen et al. Citation2011); such problem becomes more serious when a virtual endmember-based nonlinear SMA (NLSMA) model is used. Appropriate seasonal remote sensing data have been suggested to reduce the influence of grass (Selkowitz Citation2010). Another important issue is the nonlinear effect of multiple scattering from the 3D structure of shrubs. Wang et al. (Citation2015) simulated the relationship between the multiple scattering effect and shrub parameters (e.g. density and height) by using a Monte Carlo ray tracing model, namely, Forest LIGHT (FLIGHT). Their results indicated that the contribution of multiple scattering to landscape spectra increases linearly with the fractional cover of shrub and crown height. Multiple scattering exhibits a stronger effect in the near-infrared range than in the visible bands, thereby implying that selecting LSMA or NLSMA significantly affects shrub coverage estimation. In arid and semi-arid grasslands, low vegetation coverage makes selecting endmembers from remote sensing images difficult. Possible means to obtain endmember spectra include simulation from field-measured spectra (Sohn and McCoy Citation1997) and purification from high vegetation coverage pixels (Ma et al. Citation2015). Through the assumption that the reflectance of pure vegetation is identical among all impure vegetated pixels within a target image, the concept of spectral purification involves simultaneously resolving shrub endmember spectra and all endmember fractions using multiple NLSMA equations (Ma et al. Citation2015). The results showed that the purified shrub endmember spectrum was close to the measured spectrum in a semi-arid steppe area in Inner Mongolia. The endmember purification method does not rely on field-measured spectra. Thus, this method is practical for large-area shrub mapping.
3.2.2. Multi-temporal remote sensing data
Multi-temporal remote sensing data can indicate the discrepancy of phenology and sensitivity to climate variance among different vegetation types. Target species can be detected using multi-temporal remote sensing data based on the phenological differences of various species. Wilfong, Gorchov, and Henry (Citation2009) used three Landsat TM/ETM+ images taken in January, June, and November. They found that the difference in the normalized difference vegetation index (NDVI) between January and November could best distinguish invasive shrubs (Amur honeysuckle) from native woody species based on the phenological difference among invasive and native species. Selkowitz (Citation2010) compared shrub canopy mapping accuracies in Arctic Alaska by using multi-temporal Landsat ETM+, multi-temporal MODIS, and multi-spectral and multi-angle MISR data and applying the regression tree method, and found that the Landsat image from July exhibited the highest mapping accuracy. Combined with meteorological data, multi-temporal remote sensing data can help determine sensitivity difference to climate variance among shrub and grass species, and then effectively identify shrubs. Zhou et al. (Citation2013) assumed that during a dry year with slight precipitation, the growth of leguminous shrub (C. microphylla) will be influenced less than that of grass species, and the difference in the growth status of C. microphylla between a dry and a wet year will be smaller than that of grass species. Two indicators were designed based on MODIS NDVI/enhanced vegetation index (EVI) according to the preceding assumption that considered the difference between maximal or accumulative NDVI/EVI values of dry and wet years, as follows:(1)
(1)
(2)
(2) where DM and DA respectively denote the difference in maximum NDVI (or EVI) and the difference in annual accumulated NDVI (or EVI), Maxwet and Accwet respectively denote the maximum and annual accumulated NDVI (or EVI) in a wet year, and Maxdry and Accdry indicate the same definition in a dry year. The two indicators were then used to identify C. microphylla in a steppe in Inner Mongolia (Zhou et al. Citation2013).
3.2.3. GO model and multi-angle remote sensing data
Shrubs exhibit a distinct 3D structure that differs from background herbaceous species in arid and semi-arid grasslands. Therefore, using a GO model and multi-angle remote sensing data to identify shrubs in grassland and estimate their coverage is feasible. Franklin et al. (Citation1994) built a spatial discrete GO radiative transfer model for shrubs to simulate reflectance at a large spatial scale using herbaceous vegetation and soil in a savanna as background and disregarding shaded canopies and shadows on the soil. Chopping et al. (Citation2006) used multi-angle Compact High-resolution Imaging Spectrometer/Project for On-board Autonomy (CHRIS/PROBA) data and a GO model to inverse shrub coverage in the experiment range in Jornada, New Mexico. CHRIS/PROBA data use 62 bands within the 415–1050 nm spectral range and 5 observation angles, with a spatial resolution of 17 m. The measured shrub density and average radius were used to parameterize the Walthall bidirectional reflectance distribution function (BRDF) model, and an isotropic-LiSparse-RossThin kernels-driven BRDF model was used to predict the Walthall background model coefficients. In the same experimental region, Chopping et al. (Citation2008) used MISR data with nine observation angles and a spatial resolution of 275 m with a hybrid GO model for inverse shrub coverage inversion. The background models included volume scattering kernel weight from a Li–Ross BRDF model, diffuse brightness from a modified Rahman–Pinty–Verstraete BRDF model, other Li–Ross kernel weights (e.g. isotropic, geometric), and MISR near-nadir bidirectional reflectance factors (BRFs) in the blue, green, and near infrared bands. The forward modeling BRFs are in agreement with the MISR data in terms of shape and magnitude. However, the model inversion results of shrub coverage exhibit a relatively low agreement with HSR IKONOS data. Multi-angle, multi-temporal, and multi-spectral data can be used in shrub mapping; for example, Selkowitz (Citation2010) used multi-temporal MISR data, IKONOS data, and the regression tree model to estimate shrub coverage in an Arctic area.
The advantages of moderate to coarse spatial resolution remote sensing data include low cost, large spatial coverage, and over 30 years of available dynamic data. However, their disadvantages are also evident. Shrub is difficult to precisely recognize from a shrub–grass–soil mix because of the spectral similarity between shrub and grass. Thus, vegetation indices, SMA, and other typical methods can be applied only to estimate shrub coverage under a scenario without grass (Xu et al. Citation2010; Vanselow and Samimi Citation2014). When multiple temporal remote sensing data are used, the 16-day revisiting interval of Landsat may miss the key phenological date. Meanwhile, remote sensing data with high revisiting frequency, such as MODIS, are characterized by low spatial resolution, which limits shrub identification or coverage estimation accuracy (Zhou et al. Citation2013). The major difficulty in applying GO models and multi-angle remote sensing data to desert grasslands is the spatial variation in combined soil and understory background reflectance; such variation cannot be modeled as a Lambertian scatterer or by using a fixed BRDF model (Chopping et al. Citation2008).
4. Dynamic modeling and simulation of shrub encroachment
4.1. Modeling of population structure
The growth pattern of shrubs, including regeneration and mortality, and the determination of shrub life span are of considerable value to the understanding of the shrub encroachment process and the prediction of possible changes in land cover and biodiversity (Wiegand et al. Citation2000). A precise population age structure model is an effective surrogate for understanding the growth pattern and reflecting the regeneration and mortality of a population. Crisp and Lange (Citation1976) analyzed the influence of grazing on shrub population growth based on the shrub age structures of multiple regions under different grazing pressures. Their research predicted that shrubs in certain regions would be extinct after 100 years if grazing pressure would remain unchanged. Seligman and Henkin (Citation2000) obtained shrub age structures after a fire by sampling several grasslands in the eastern Mediterranean area; they used the age structures to analyze shrub recovery mechanism after a fire. Kuuseoks, Dong, and Reed (Citation2001) quantified the relationship between shrub regeneration/mortality rate and environmental factors, such as illumination and upper vegetation canopy density, to improve the understanding of the growth mechanism of understory shrubs. Myers-Smith et al. (Citation2011) designed an age evaluation model for a red willow and then estimated the life span of the willow. The relationship between the shrub encroachment process and these disturbance events is analyzed by investigating historical natural disturbance events.
The shrub population structure is typically constructed by collecting field samples and then measuring the ages of the samples (Kuuseoks, Dong, and Reed Citation2001; Myers-Smith et al. Citation2011). A precise model of shrub population structure requires abundant shrub age samples to accurately estimate regeneration and mortality rates. However, one of the main problems in the construction of shrub population structure is the difficulty in collecting a sufficient number of field samples (Crisp and Lange Citation1976; Wiegand et al. Citation2000). The destructive measurement method for shrub age is another factor that limits the number of field samples. Cao et al. (Citation2018) recently proposed a shrub age structure estimation model that depended on the relationship among shrub age, canopy size, and precipitation. Canopy size can be obtained from HSR remote sensing images. This technique can help build a precise shrub age structure model using a small number of field samples and a less destructive method.
4.2. Modeling of shrub growth
The simulation of shrub encroachment mainly relies on plant growth and spatial expansion models. Plant growth models describe the dynamic process of plant growth and hydrology with a series of functions. For example, the logistic function can be used to describe the dynamic process of shrubs and herbaceous plants, and it can involve influencing factors such as grazing pressure, climatic factors, and fire (D’Odorico, Okin, and Bestelmeyer Citation2012). The EcoHydrology in Drylands (EcoHyD) model (Tietjen et al. Citation2010) comprises a vegetation process model and a hydrological process model by calculating the annual growth process of shrubs, perennial herbs, and herbaceous plants in 2 weeks and the daily soil moisture contents of the two soil layers. EcoHyD can be used to simulate the influence of fire management measures on shrub encroachment (Lohmann et al. Citation2014). Chiou et al. (Citation2013) used boosted regression trees to identify and quantify the potential factors influencing the likelihood of shrub invasion in Taiwan. The influencing factors include climatic conditions (temperature and precipitation), landscape features (altitude; slope ratio and aspect; percentages of natural or secondary forest, agriculture land, developed area, and water bodies; and distances to the nearest forest edge and river), and anthropogenic factors (length of forest edge, and distances to the nearest road and agriculture land), and result indicated the most influential variables are average annual temperature, altitude, precipitation, and slope.
Spatial expansion models typically simplify complex growth process models as a series of sub-models and use methods based on discrimination rules. Cellular automaton (CA) model was popularly used to simulate the spatial grass-shrub dynamics. Jeltsch et al. (Citation1996, Citation1997) simulated dynamic changes in shrub and grass by considering precipitation, soil moisture, grazing, and fire within 20,000 5 × 5 m grids based on the CA model. Zhou, Istanbulluoglu, and Vivoni (Citation2013) built a Cellular Automata Tree-Grass-Shrub Simulator (CATGraSS) to simulate a tree-grass-shrub coexistence environment in a semiarid climate. In CATGraSS model, topography determines spatial distribution of solar radiation and future the spatial variations in evapotranspiration, soil moisture, and plant water stress. Each cell has a single type, i.e. tree, shrub, tree seedlings, shrub seedlings, grass, or soil. Plant growth is driven by evapotranspiration, and plant establishment and mortality are linked to water stress. Caracciolo, Noto, and Istanbulluoglu (Citation2014) and Caracciolo et al. (Citation2016) used CATGraSS to simulate shrub encroachment in the northern Chihuahuan desert with new algorithms concerning fire, grazing, and seed dispersal caused by animals and wind effect. Cipriotti et al. (Citation2012) designed a spatial simulation model–DINVEG to describe the long-term dynamics of dominant grass and shrub species in the semiarid steppe of Argentina. The vegetation dynamic is linked with water balance model, and a 50 × 50 m steppe plot with a spatial resolution of 0.2 × 0.2 m was modeled. Komac et al. (Citation2013) built a state transition probability model of shrubs, grass, and barren land by considering influencing factors such as fire and mechanized shrub removal management measures; they simulated the shrub encroachment process at the patch scale (1 m grid). However, current simulations of shrub encroachment are mostly conducted at the patch and landscape scales, and shrub encroachment simulation remains lacking at the regional scale and during stage transformation.
5. Conclusion and discussion
Shrub encroachment into arid semi-arid grasslands has elicited research attention for decades. However, challenges remain in the quantitative analysis of shrub encroachment mechanisms, simulation and prediction of shrub encroachment, and dynamic monitoring at the landscape and regional scales. First, present studies on the shrub encroachment process and mechanism have involved integrated factors, such as water use, temperature, soil, and biological and anthropogenic activities. However, these results are mainly dependent on the observation and analysis of the natural shrub encroachment process over small areas. They lack support from large-scale observations or long-term field control experimental evidence. Second, no consensus has been reached on whether or under which conditions shrub encroachment can be restored due to the incomplete understanding of its process and mechanism in grasslands. Meanwhile, the shrub encroachment stages can hardly be quantitatively defined, and no indicator or method is available for the classification of shrub encroachment stages based on vegetation information from remote sensing inversion. A possible means to describe the status or stages of shrub encroachment is by combining vegetation coverage information with patch characteristics at the landscape scale. Third, multi-scale remote sensing presents challenges to the dynamic monitoring of shrub encroachment in arid/semi-arid grasslands. HSR remote sensing data cannot directly identify small shrubs. Furthermore, several disadvantages exist, such as the high cost of large range coverage and the lack of historical data. Thus, monitoring the spatial distribution of shrubs at the regional scale and the dynamics of shrubs for a long period is difficult. The use of moderate and coarse spatial resolution multi-spectral remote sensing data to identify shrubs and accurately estimate their coverage is difficult due to spectral mixture, lack of spatiotemporal continuous data, and limited spatial resolution. Further studies are required for methods that consider phenological differences or sensitivity to precipitation differences between shrubs and grasses. Finally, the quantitative analysis and simulation of shrub encroachment at different spatiotemporal scales and during the stage shift process remain insufficient due to the lack of understanding of the shrub encroachment mechanism and process. In summary, shrub encroachment dynamic monitoring based on remote sensing at the landscape or regional scale can play a crucial role in analyzing and understanding the process and mechanism of shrub encroachment into arid/semi-arid grasslands. The research on shrub encroachment should be multi-disciplinary and should involve multi-partnerships.
Disclosure statement
No potential conflict of interest was reported by the authors.
Additional information
Funding
References
- Adams, M. E. 1967. “A Study of the Ecology of Acacia mellifera, A. seyal and Balanites aegyptiaca in Relation to Land-clearing.” Journal of Applied Ecology 4 (1): 221–237.
- Anderies, J. M., M. A. Janssen, and B. H. Walker. 2002. “Grazing Management, Resilience, and the Dynamics of a Fire-driven Rangeland System.” Ecosystems 5 (1): 23–44. doi:10.1007/s10021-001-0053-9.
- Archer, S. 1989. “Have Southern Texas Savannas Been Converted to Woodlands in Recent History?” The American Naturalist 134 (4): 545–561. doi:10.1086/284996.
- Archer, S., D. S. Schimel, and E. A. Holland. 1995. “Mechanisms of Shrubland Expansion: Land Use, Climate or CO2?” Climatic Change 29 (1): 91–99. doi:10.1007/BF01091640.
- Belayneh, A., and Z. K. Tessema. 2017. “Mechanisms of Bush Encroachment and Its Inter-connection with Rangeland Degradation in Semi-arid African Ecosystems: A Review.” Journal of Arid Land 9 (2): 299–312. doi:10.1007/s40333-016-0023-x.
- Briggs, J. M., J. M. Blair, and J. Mccarron. 2005. “An Ecosystem in Transition: Cause and Consequences of the Conversion of Mesic Grassland to Shrubland.” BioScience 55 (7): 561–572. doi:10.1641/0006-3568(2005)055.
- Briske, D. D., S. D. Fuhlendorf, and F. E. Smeins. 2003. “Vegetation Dynamics on Rangelands: A Critique of the Current Paradigms.” Journal of Applied Ecology 40 (4): 601–614.
- Camacho-De Coca, F., F. J. García-Haro, M. A. Gilabert, and J. Meliá. 2004. “Vegetation Cover Seasonal Changes Assessment from TM Imagery in a Semi-arid Landscape.” International Journal of Remote Sensing 25 (17): 3451–3476. doi:10.1080/01431160310001618761.
- Cao, X., Y. Liu, Q. Liu, X. Cui, X. Chen, and J. Chen. 2018. “Estimating Population Structure of Encroaching Shrubs in Arid/Semiarid Grassland Using High-spatial-Resolution Remotely Sensed Imagery.” Remote Sensing of Environment, Under Review.
- Caracciolo, D., E. Istanbulluoglu, L. V. Noto, and S. L. Collins. 2016. “Mechanisms of Shrub Encroachment Into Northern Chihuahuan Desert Grasslands and Impacts of Climate Change Investigated Using a Cellular Automata Model.” Advances in Water Resources 91: 46–62.
- Caracciolo, D., L. V. Noto, and E. Istanbulluoglu. 2014. “Modelling the Shrub Encroachment in a Grassland with a Cellular Automata Model.” Proceedings of the International Association of Hydrological Sciences 364: 20–25.
- Chen, J., Y. Chen, C. He, and P. Shi. 2001. “Sub-pixel Model for Vegetation Fraction Estimation Based on Land Cover Classification.” Journal of Remote Sensing 5: 416–423.
- Chen, X., J. Chen, X. Jia, B. Somers, J. Wu, and P. Coppin. 2011. “A Quantitative Analysis of Virtual Endmembers’ Increased Impact on the Collinearity Effect in Spectral Unmixing.” IEEE Transactions on Geoscience and Remote Sensing 49 (8): 2945–2956. doi:10.1109/TGRS.2011.2121073.
- Chiou, C. R., H. H. Wang, Y. J. Chen, W. E. Grant, and M. L. Lu. 2013. “Modeling Potential Range Expansion of the Invasive Shrub Leucaena Leucocephala in the Hengchun Peninsula, Taiwan.” Invasive Plant Science and Management 6 (4): 492–501.
- Chopping, M., L. Su, A. Laliberte, A. Rango, D. P. C. Peters, and N. Kollikkathara. 2006. “Mapping Shrub Abundance in Desert Grasslands Using Geometric-optical Modeling and Multi-angle Remote Sensing with CHRIS/Proba.” Remote Sensing of Environment 104 (1): 62–73. doi:10.1016/j.rse.2006.04.022.
- Chopping, M., L. Su, A. Rango, J. V. Martonchik, D. P. C. Peters, and A. Laliberte. 2008. “Remote Sensing of Woody Shrub Cover in Desert Grasslands Using MISR with a Geometric-optical Canopy Reflectance Model.” Remote Sensing of Environment 112 (1): 19–34. doi:10.1016/j.rse.2006.04.023.
- Cipriotti, P. A., and M. R. Aguiar. 2012. “Direct and Indirect Effects of Grazing Constrain Shrub Encroachment in Semi-arid Patagonian Steppes.” Applied Vegetation Science 15 (1): 35–47. doi:10.1111/j.1654-109X.2011.01138.x.
- Cipriotti, P. A., M. R. Aguiar, T. Wiegand, and J. M. Paruelo. 2012. “Understanding the Long-term Spatial Dynamics of a Semiarid Grass-shrub Steppe through Inverse Parameterization for Simulation Models.” Oikos 121: 848–861. doi:10.1111/j.1600-0706.2012.20317.x.
- Crisp, M. D., and R. T. Lange. 1976. “Age Structure, Distribution and Survival Under Grazing of the Arid-zone Shrub Acacia Burkittii.” OIKOS 27 (1): 86–92.
- D’Odorico, P., J. D. Fuentes, W. T. Pockman, S. L. Collins, Y. He, J. S. Medeiros, S. Dewekker, and M. E. Litvak. 2010. “Positive Feedback between Microclimate and Shrub Encroachment in the Northern Chihuahuan Desert.” Ecosphere 1 (6). doi:10.1890/ES10-00073.1.
- D’Odorico, P., G. S. Okin, and B. T. Bestelmeyer. 2012. “A Synthetic Review of Feedbacks and Drivers of Shrub Encroachment in Arid Grasslands.” Ecohydrology 5 (5): 520–530. doi:10.1002/eco.259.
- Duncan, J., D. Stow, J. Franklin, and A. Hope. 1993. “Assessing the Relationship between Spectral Vegetation Indices and Shrub Cover in the Jornada Basin, New Mexico.” International Journal of Remote Sensing 14: 3395–3416.
- Eldridge, D. J., M. A. Bowker, F. T. Maestre, E. Roger, J. F. Reynolds, and W. G. Whitford. 2011. “Impacts of Shrub Encroachment on Ecosystem Structure and Functioning: Towards a Global Synthesis.” Ecology Letters 14 (7): 709–722. doi:10.1111/j.1461-0248.2011.01630.x.
- Frank, T. D., and S. A. Tweddale. 2006. “The Effect of Spatial Resolution on Measurement of Vegetation Cover in Three Mojave Desert Shrub Communities.” Journal of Arid Environments 67: 88–99. doi:10.1016/j.jaridenv.2006.09.020.
- Franklin, J., J. Duncan, A. R. Huete, W. J. D. van Leeuwen, X. Li, and A. Bégué. 1994. “Radiative Transfer in Shrub Savanna Sites in Niger: Preliminary Results from HAPEX-Sahel. 1. Modelling Surface Reflectance Using a Geometric-optical Approach.” Agricultural and Forest Meteorology 69 (3–4): 223–245. doi:10.1016/0168-1923(94)90027-2.
- Garrity, S. R., L. A. Vierling, A. M. S. Smith, M. J. Falkowski, and D. B. Hann. 2008. “Automatic Detection of Shrub Location, Crown Area, and Cover Using Spatial Wavelet Analysis and Aerial Photography.” Canadian Journal of Remote Sensing 34: S376–S384. doi:10.5589/m08-056.
- Goslee, S. C., K. M. Havstad, D. P. C. Peters, A. Rango, and W. H. Schlesinger. 2003. “High-resolution Images Reveal Rate and Pattern of Shrub Encroachment Over Six Decades in New Mexico, U.S.A.” Journal of Arid Environments 54 (4): 755–767. doi:10.1006/jare.2002.1103.
- Han, Y., J. Niu, Q. Zhang, J. Dong, X. Zhang, and S. Kang. 2014. “The Changing of Vegetation Pattern and Its Driven Forces of Grassland in Xinlin River Basin in Thirty Years.” Chinese Journal of Grassland 36: 70–77.
- Hellesen, T., and L. Matikainen. 2013. “An Object-based Approach for Mapping Shrub and Tree Cover on Grassland Habitats by Use of LiDAR and CIR Orthoimages.” Remote Sensing 5 (2): 558–583. doi:10.3390/rs5020558.
- Henry, M. C., and A. S. Hope. 1998. “Monitoring Post-burn Recovery of Chaparral Vegetation in Southern California Using Multi-temporal Satellite Data.” International Journal of Remote Sensing 19 (16): 3097–3107. doi:10.1080/014311698214208.
- Hutchinson, C. F., J. D. Unruh, and C. J. Bahre. 2000. “Land Use vs. Climate as Causes of Vegetation Change: A Study in SE Arizona.” Global Environmental Change 10 (1): 47–55. doi:10.1016/S0959-3780(00)00009-1.
- Jeltsch, F., S. J. Milton, W. R. J. Dean, and N. van Rooyen. 1996. “Tree Spacing and Coexistence in Semiarid Savannas.” The Journal of Ecology 84 (4): 583–595. doi:10.2307/2261480.
- Jeltsch, F., S. J. Milton, W. R. J. Dean, and N. van Rooyen. 1997. “Analysing Shrub Encroachment in the Southern Kalahari: A Grid-based Modelling Approach.” The Journal of Applied Ecology 34 (6): 1497. doi:10.2307/2405265.
- Kéfi, S., M. Rietkerk, C. L. Alados, Y. Pueyo, V. P. Papanastasis, A. ElAich, and P. C. de Ruiter. 2007. “Spatial Vegetation Patterns and Imminent Desertification in Mediterranean Arid Ecosystems.” Nature 449 (7159): 213–217. doi:10.1038/nature06111.
- Kerley, G. I. H., and W. G. Whitford. 2009. “Can Kangaroo Rat Graminivory Contribute to the Persistence of Desertified Shrublands?” Journal of Arid Environments 73 (6–7): 651–657. doi:10.1016/j.jaridenv.2009.01.001.
- Komac, B., S. Kéfi, J. Escós, and C. L. Alados. 2013. “Modeling Shrub Encroachment in Subalpine Grasslands Under Different Environmental and Management Scenarios.” Journal of Environmental Management 121: 160–169.
- Kuuseoks, E., J. Dong, and D. Reed. 2001. “Shrub Age Structure in Northern Minnesota Aspen Stands.” Forest Ecology and Management 149 (1–3): 265–274. doi:10.1016/S0378-1127(00)00559-4.
- Lahav-Ginott, S., R. Kadmon, and M. Gersani. 2001. “Evaluating the Viability of Acacia Populations in the Negev Desert: A Remote Sensing Approach.” Biological Conservation 98 (2): 127–137. doi:10.1016/S0006-3207(00)00147-6.
- Laliberte, A. S., A. Rango, K. M. Havstad, J. F. Paris, R. F. Beck, R. McNeely, and A. L. Gonzalez. 2004. “Object-oriented Image Analysis for Mapping Shrub Encroachment from 1937 to 2003 in Southern New Mexico.” Remote Sensing of Environment 93 (1–2): 198–210. doi:10.1016/j.rse.2004.07.011.
- Levick, S. R., and K. H. Rogers. 2011. “Context-dependent Vegetation Dynamics in an African Savanna.” Landscape Ecology 26 (4): 515–528. doi:10.1007/s10980-011-9578-2.
- Li, X., and Y. Gao. 2012. “Effects of Shrub Encroachment in Desert Grassland on Runoff and the Induced Nitrogen Loss in Southeast Fringe of Tengger Desert.” Acta Ecologica Sinica 32: 7828–7835.
- Lohmann, D., B. Tietjen, N. Blaum, D. F. Joubert, and F. Jeltsch. 2014. “Prescribed Fire as a Tool for Managing Shrub Encroachment in Semi-Arid Savanna Rangelands.” Journal of Arid Environments 107: 49–56. doi:10.1016/j.jaridenv.2014.04.003.
- Ma, L., Y. Zhou, J. Chen, X. Cao, and X. Chen. 2015. “Estimation of Fractional Vegetation Cover in Semiarid Areas by Integrating Endmember Reflectance Purification Into Nonlinear Spectral Mixture Analysis.” IEEE Geoscience and Remote Sensing Letters 12 (6): 1175–1179.
- Maestre, F. T., M. A. Bowker, M. D. Puche, M. Belén Hinojosa, I. Martínez, P. García-Palacios, A. P. Castillo, et al. 2009. “Shrub Encroachment Can Reverse Desertification in Semi-arid Mediterranean Grasslands.” Ecology Letters 12 (9): 930–941. doi:10.1111/j.1461-0248.2009.01352.x.
- McGlynn, I. O., and G. S. Okin. 2006. “Characterization of Shrub Distribution Using High Spatial Resolution Remote Sensing: Ecosystem Implications for a Former Chihuahuan Desert Grassland.” Remote Sensing of Environment 101: 554–566.
- Mitchard, E. T. A., S. S. Saatchi, S. L. Lewis, T. R. Feldpausch, I. H. Woodhouse, B. Sonké, C. Rowland, and P. Meir. 2011. “Measuring Biomass Changes due to Woody Encroachment and Deforestation/Degradation in a Forest-Savanna Boundary Region of Central Africa Using Multi-temporal L-Band Radar Backscatter.” Remote Sensing of Environment 115 (11): 2861–2873. doi:10.1016/j.rse.2010.02.022.
- Mitchard, E. T. A., S. S. Saatchi, I. H. Woodhouse, G. Nangendo, N. S. Ribeiro, M. Williams, C. M. Ryan, S. L. Lewis, T. R. Feldpausch, and P. Meir. 2009. “Using Satellite Radar Backscatter to Predict above-Ground Woody Biomass: A Consistent Relationship across Four Different African Landscapes.” Geophysical Research Letters 36 (23). doi:10.1029/2009GL040692.
- Mooney, H. A., and E. E. Cleland. 2001. “The Evolutionary Impact of Invasive Species.” Proceedings of the National Academy of Sciences of the United States of America 98 (10): 5446–5451. doi:10.1073/pnas.091093398.
- Myers-Smith, I. H., D. S. Hik, C. Kennedy, D. Cooley, J. F. Johnstone, A. J. Kenney, and C. J. Krebs. 2011. “Expansion of Canopy-forming Willows Over the Twentieth Century on Herschel Island, Yukon Territory, Canada.” Ambio 40 (6): 610–623. doi:10.1007/s13280-011-0168-y.
- Naito, A. T., and D. M. Cairns. 2011. “Patterns and Processes of Global Shrub Expansion.” Progress in Physical Geography 35 (4): 423–442. doi:10.1177/0309133311403538.
- Niu, B., J. Liu, and Z. Wang. 2005. “Remote Sensing Information Extraction Based Vegetation Fraction in Semiarid Area.” Geo-Infomation Science 7: 84–97.
- Okin, G. S., P. D’Odorico, and S. R. Archer. 2009. “Impacts of Feedbacks on Chihuahuan Desert Grasslands: Transience and Metastability Driven by Grass Recruitment.” Journal of Geophysical Research 114 (G01004). doi:10.1029/2008JG000833.
- Olthof, I., and R. H. Fraser. 2007. “Mapping Northern Land Cover Fractions Using Landsat ETM.” Remote Sensing of Environment 107 (3): 496–509. doi:10.1016/j.rse.2006.10.009.
- Pan, J., and T. Li. 2010. “Extracting Desertification from Landsat Imagery Based on Spectral Mixture Analysis and Abdedo Vegetation Feature Space.” Journal of Natural Resources 25: 1960–1969.
- Peng, H., X. Li, G. Li, Z. Zhang, S. Zhang, L. Li, G. Zhao, Z. Jiang, and Y. Ma. 2013. “Shrub Encroachment with Increasing Anthropogenic Disturbance in the Semiarid Inner Mongolian Grasslands of China.” Catena 109: 39–48. doi:10.1016/j.catena.2013.05.008.
- Peng, H., X. Li, and S. Tong. 2013. “Effects of Shrub Encroachment on Biomass and Biodiversity in the Typical Steppe of Inner Mongolia.” Acta Ecologica Sinica 33: 7221–7229.
- Peng, H., X. Li, and S. Tong. 2014. “Effects of Shrub (Caragana Microphalla Lam.) Encroachment on Water Redistribution and Utilization in the Typical Steppe of Inner Mongolia.” Acta Ecologica Sinica 34 (9): 2256–2265.
- Peters, D. P. C., B. T. Bestelmeyer, J. E. Herrick, E. L. Fredrickson, H. C. Monger, and K. M. Havstad. 2006. “Disentangling Complex Landscapes: New Insights Into Arid and Semiarid System Dynamics.” BioScience 56 (6): 491. doi:10.1641/0006-3568(2006)56[491:DCLNII]2.0.CO;2.
- Rietkerk, M., S. C. Dekker, P. C. De Ruiter, and J. van de Koppel. 2004. “Self-organized Patchiness and Catastrophic Shifts in Ecosystems.” Science 305 (5692): 1926–1929. doi:10.1126/science.1101867.
- Robinson, T. P., R. D. van Klinken, and G. Metternicht. 2008. “Spatial and Temporal Rates and Patterns of Mesquite (Prosopis Species) Invasion in Western Australia.” Journal of Arid Environments 72 (3): 175–188. doi:10.1016/j.jaridenv.2007.05.011.
- Rogan, J., and J. Franklin. 2001. “Mapping Wildfire Burn Severity in Southern California Forests and Shrublands Using Enhanced Thematic Mapper Imagery.” Geocarto International 16 (4): 91–106. doi:10.1080/10106040108542218.
- Roques, K. G., T. G. O’Connor, and A. R. Watkinson. 2001. “Dynamics of Shrub Encroachment in an African Savanna: Relative Influences of Fire, Herbivory, Rainfall and Density Dependence.” Journal of Applied Ecology 38 (2): 268–280. doi:10.1046/j.1365-2664.2001.00567.x.
- Sankaran, M., N. P. Hanan, R. J. Scholes, J. Ratnam, D. J. Augustine, B. S. Cade, J. Gignoux, et al. 2005. “Determinants of Woody Cover in African Savannas.” Nature 438: 846–849.
- Sankaran, M., J. Ratnam, and N. P. Hanan. 2004. “Tree-grass Coexistence in Savannas Revisited-Insights from an Examination of Assumptions and Mechanisms Invoked in Existing Models.” Ecology Letters 7 (6): 480–490.
- Scanlon, T. M., K. K. Caylor, S. A. Levin, and I. Rodriguez-Iturbe. 2007. “Positive Feedbacks Promote Power-law Clustering of Kalahari Vegetation.” Nature 449 (7159): 209–212. doi:10.1038/nature06060.
- Scheffer, M., J. Bascompte, W. A. Brock, V. Brovkin, S. R. Carpenter, V. Dakos, H. Held, E. H. van Nes, M. Rietkerk, and G. Sugihara. 2009. “Early-warning Signals for Critical Transitions.” Nature 461 (7260): 53–59. doi:10.1038/nature08227.
- Scheffer, M., and S. R. Carpenter. 2003. “Catastrophic Regime Shifts in Ecosystems: Linking Theory to Observation.” Trends in Ecology and Evolution 18 (12): 648–656. doi:10.1016/j.tree.2003.09.002.
- Schlesinger, W. H., J. F. Reynolds, G. L. Cunningham, L. F. Huenneke, W. M. Jarrell, R. A. Virginia, and W. G. Whitford. 1990. “Biological Feedbacks in Global Desertification.” Science 247 (4946): 1043–1048. doi:10.1126/science.247.4946.1043.
- Seligman, N. G., and Z. Henkin. 2000. “Regeneration of a Dominant Mediterranean Dwarf-Shrub After Fire.” Journal of Vegetation Science 11 (6): 893–902. doi:10.2307/3236559.
- Selkowitz, D. J. 2010. “A Comparison of Multi-spectral, Multi-angular, and Multi-temporal Remote Sensing Datasets for Fractional Shrub Canopy Mapping in Arctic Alaska.” Remote Sensing of Environment 114 (7): 1338–1352. doi:10.1016/j.rse.2010.01.012.
- Skowno, A. L., M. W. Thompson, J. Hiestermann, B. Ripley, A. G. West, and W. J. Bond. 2017. “Woodland Expansion in South African Grassy Biomes Based on Satellite Observations (1990–2013): General Patterns and Potential Drivers.” Global Change Biology 23 (6): 2358–2369. doi:10.1111/gcb.13529.
- Small, C. 2001. “Estimation of Urban Vegetation Abundance by Spectral Mixture Analysis.” International Journal of Remote Sensing 22: 1305–1334.
- Sohn, Y., and R. M. McCoy. 1997. “Mapping Desert Shrub Rangeland Using Spectral Unmixing and Modeling Spectral Mixtures with TM Data.” Photogrammetric Engineering & Remote Sensing 63 (6): 707–716.
- Somers, B., G. P. Asner, L. Tits, and P. Coppin. 2011. “Endmember Variability in Spectral Mixture Analysis: A Review.” Remote Sensing of Environment 115 (7): 1603–1616. doi:10.1016/j.rse.2011.03.003.
- Song, C. 2005. “Spectral Mixture Analysis for Subpixel Vegetation Fractions in the Urban Environment: How to Incorporate Endmember Variability?” Remote Sensing of Environment 95 (2): 248–263. doi:10.1016/j.rse.2005.01.002.
- Suttie, J. M., S. G. Reynolds, and C. Batello. 2005. Grasslands of the World. Grasslands of the World. Roma: Food and Agriculture Organization of the United Nations.
- Theseira, M. A., G. Thomas, and C. A. D. Sannier. 2002. “An Evaluation of Spectral Mixture Modelling Applied to a Semi-Arid Environment.” International Journal of Remote Sensing 23 (4): 687–700. doi:10.1080/01431160010019652.
- Tietjen, B., F. Jeltsch, E. Zehe, N. Classen, A. Groengroeft, K. Schiffers, and J. Oldeland. 2010. “Effects of Climate Change on the Coupled Dynamics of Water and Vegetation in Drylands.” Ecohydrology 3 (2): 226–237. doi:10.1002/eco.
- Tong, C., W. Yong, Y. Wu, L. Zhao, C. Jiang, and S. Yong. 2001. “Change in the Spatia Structure of Grassland Vegetation in the Xilin River Basin.” Acta Scientiarum Naturalium Universitatis NeiMonggol 32: 562–566.
- Van Auken, O. W. 2009. “Causes and Consequences of Woody Plant Encroachment Into Western North American Grasslands.” Journal of Environmental Management 90 (10): 2931–2942. doi:10.1016/j.jenvman.2009.04.023.
- van de Koppel, J., M. Rietkerk, F. van Langevelde, L. Kumar, C. A. Klausmeier, J. M. Fryxell, J. W. Hearne, et al. 2002. “Spatial Heterogeneity and Irreversible Vegetation Change in Semiarid Grazing Systems.” The American Naturalist 159 (2): 209–218. doi:10.1086/324791.
- van Langevelde, F., C. A. D. M. van de Vijver, L. Kumar, J. van de Koppel, N. de Ridder, J. van Andel, A. K. Skidmore, et al. 2003. “Effects of Fire and Herbivory on the Stability of Savanna Ecosystems.” Ecology 84 (2): 337–350. doi:10.1890/0012-9658(2003)084[0337:EOFAHO]2.0.CO;2.
- Vanselow, K. A., and C. Samimi. 2014. “Predictive Mapping of Dwarf Shrub Vegetation in an Arid High Mountain Ecosystem Using Remote Sensing and Random Forests.” Remote Sensing 6 (7): 6709–6726. doi:10.3390/rs6076709.
- Veraverbeke, S., B. Somers, I. Gitas, T. Katagis, A. Polychronaki, and R. Goossens. 2012. “Spectral Mixture Analysis to Assess Post-Fire Vegetation Regeneration Using Landsat Thematic Mapper Imagery: Accounting for Soil Brightness Variation.” International Journal of Applied Earth Observation and Geoinformation 14 (1): 1–11. doi:10.1016/j.jag.2011.08.004.
- Vila, J. P. S., and P. Barbosa. 2010. “Post Fire Vegetation Regrwoth Detection in the Deiva Marina Region Using LANDSAT Tm and ETM Data.” Ecological Modelling 221: 75–84. doi:10.1016/j.ecolmodel.2009.03.011.
- Walter, H. 1939. “Grassland, Savanna and Bush in Arid Parts of Africa and Their Ecological Dependence.” Jahrbücher für Wissenschaftlike Botanik 87: 750–860. (in German)
- Wang, J., Y. Cai, Z. An, and J. Liu. 2013. “The Distribution Changes of Alpine Shrub and Its Climatic Driving Forces in Junhe Sub-Watershed Based on Remote Sensing.” Resources Science 35 (6): 1300–1309.
- Wang, J., X. Cao, J. Chen, and X. Cui. 2015. “A Simulation Study for Assessment of Multiple Scattering Effects on Mixture of Shrub and Soil.” IEEE Transactions on Geoscience and Remote Sensing 53 (9): 4910–4921.
- Westoby, M., B. Walker, and I. Noy-Meir. 1989. “Opportunistic Management for Rangelands Not at Equilibrium.” Journal of Range Management 42 (4): 266–274.
- White, R. N., S. Murray, and M. Rohweder. 2000. Pilot Analysis of Global Ecosystems: Grassland Ecosystems. World Resources Institute. Washington, DC. http://www.wri.org/wr2000.
- Wiegand, T., S. J. Milton, K. J. Esler, and G. F. Midgley. 2000. “Live Fast, Die Young: Estimating Size-age Relations and Mortality Pattern of Shrubs Species in the Semi-arid Karoo, South Africa.” Plant Ecology 150 (1–2): 115–131. doi:10.1023/A:1026526419886.
- Wilfong, B. N., D. L. Gorchov, and M. C. Henry. 2009. “Detecting an Invasive Shrub in Deciduous Forest Understories Using Remote Sensing.” Weed Science 57 (5): 512–520. doi:10.1614/WS-09-012.1.
- Wu, C. S., and A. T. Murray. 2003. “Estimating Impervious Surface Distribution by Spectral Mixture Analysis.” Remote Sensing of Environment 84 (4): 493–505. doi:10.1016/S0034-4257(02)00136-0.
- Xiong, X., and X. Han. 2005. “Spatial Heterogeneity in Soil Carbon and Nitrogen Resources, Caused by Caragana Microphylla, in the Thicketization of Semiarid Grassland, Inner Mongolia.” Acta Ecologica Sinica 25 (7): 1678–1683.
- Xiong, X., and X. Han. 2006. “Application of State and Transition Models to Discussing the Thicketization of Steppe in Xilin River Basin, Inner Mongolia.” Acta Prataculturae Sinica 15: 15–19.
- Xiong, X., X. Han, Y. Bai, and Q. Pan. 2003. “Increased Distribution of Caragana Microphylla in Rangelands and Its Causes and Consequences in Xilin River Basin.” Acta Prataculturae Sinica 12: 57–62.
- Xiong, X., X. Han, and Y. Bao. 2005. “Discussion on the Research Into Sandy Desertification, Accompanying by Thicketization of Semiarid Grasslands in Inner Mongolia, China.” Acta Prataculturae Sinica 14: 1–5.
- Xu, M., C. Cao, Q. Tong, Z. Li, H. Zhang, Q. He, M. Gao, et al. 2010. “Remote Sensing Based Shrub Above-Ground Biomass and Carbon Storage Mapping in Mu Us Desert, China.” Science China Technological Sciences 53 (s1): 176–183. doi:10.1007/s11431-010-3231-1.
- Xu, L., and F. Li. 2009. “Digital Measurement for Typical Desert Shrubs Based on Aerial Images.” Journal of Northeast Forestry University 37 (9): 86–90.
- Zhang, P., H. Qing, L. Zhang, Y. Xu, L. Mu, R. Ye, X. Qiu, H. Chang, H. Shen, and J. Yang. 2017. “Population Structure and Spatial Pattern of Caragana Tibetica Communities in Nei Mongol Shrub-Encroached Grassland.” Chinese Journal of Plant Ecology 41 (2): 165–174. doi:10.17521/cjpe.2015.0448.
- Zhang, H., P. Shi, and Q. Zheng. 2001. “Research Progress in Relationship between Shrub Invasion and Soil Heterogeneity Natural Semi-Arid Grassland.” Acta Phytoecologica Sinica 25 (3): 366–370.
- Zhang, W., Y. Zhang, Z. Wang, M. Ding, X. Yang, X. Lin, and Y. Yan. 2006. “Analysis of Vegetation Change in Mt. Qomolangma Natural Reserver.” Progress in Geography 25: 12–21.
- Zhou, D. 1990. “Encroached-grassland of Caragana Microphylla Lam. in Inner Mongolia.” Grassland and Prataculture 3: 17–19.
- Zhou, Y., J. Chen, X. Chen, X. Cao, and X. Zhu. 2013. “Two Important Indicators with Potential to Identify Caragana Microphylla in Xilin Gol Grassland from Temporal MODIS Data.” Ecological Indicators 34: 520–527. doi:10.1016/j.ecolind.2013.06.014.
- Zhou, X., E. Istanbulluoglu, and E. R. Vivoni. 2013. “Modeling the Ecohydrological Role of Aspect-controlled Radiation on Tree-grass-Shrub Coexistence in a Semiarid Climate.” Water Resources Research 49 (5): 2872–2895. doi:10.1002/wrcr.20259.