ABSTRACT
In 2015, it was adopted the 2030 Agenda for Sustainable Development to end poverty, protect the planet and ensure that all people enjoy peace and prosperity. The year after, 17 Sustainable Development Goals (SDGs) officially came into force. In 2015, GEO (Group on Earth Observation) declared to support the implementation of SDGs. The GEO Global Earth Observation System of Systems (GEOSS) required a change of paradigm, moving from a data-centric approach to a more knowledge-driven one. To this end, the GEO System-of-Systems (SoS) framework may refer to the well-known Data-Information-Knowledge-Wisdom (DIKW) paradigm. In the context of an Earth Observation (EO) SoS, a set of main elements are recognized as connecting links for generating knowledge from EO and non-EO data – e.g. social and economic datasets. These elements are: Essential Variables (EVs), Indicators and Indexes, Goals and Targets. Their generation and use requires the development of a SoS KB whose management process has evolved the GEOSS Software Ecosystem into a GEOSS Social Ecosystem. This includes: collect, formalize, publish, access, use, and update knowledge. ConnectinGEO project analysed the knowledge necessary to recognize, formalize, access, and use EVs. The analysis recognized GEOSS gaps providing recommendations on supporting global decision-making within and across different domains.
1. Introduction
Sustainable development concerns three main dimensionalities: economic, social, and environmental extents; in our time, humanity is facing important challenges in all of them. Global environmental changes are mostly induced by human activities and have reached a scale where increasing pressures may lead to cross Earth system thresholds (e.g. Planetary Boundaries) with potential irreversible consequences (Rockström, Bai, and deVries Citation2018). In the scientific Community, it is now recognized that humanity has entered a new geological epoch commonly named Anthropocene: the period during which human activity has having a global significant impact on the Earth system (Biermann et al. Citation2016; Verburg et al. Citation2016).
Therefore, there is an urgent need for sustainable development and exploring the complex, interlinked, and mutually interacting chains of causes and effects at the global scale between human needs and natural capitals (Díaz et al. Citation2018). The need for action, to address global challenges, is now reflected in the United Nations 2030 Agenda for Sustainable Development. Set by the United Nations General Assembly in 2015 (United Nations Citation2015), the agenda defines 17 Sustainable Development Goals (SDGs). They encompass the three pillars of sustainable development, including: poverty, hunger, health, education, climate change, gender equality, water, sanitation, energy, urbanization, environment, and social justice.
In the context of this paper, we will mainly focus on the sustainability challenges that have an environmental dimension and that can be monitored using Earth Observations (EO).
1.1. From data to knowledge for sustainable development
A sustainable development needs to minimize negative externalities, while simultaneously seeking to maximize the positive ones. To achieve that, information and simulations on types of consumption and production processes are vital. To reach the objective of a stable functioning of the Earth‘s life, it is important to provide sound knowledge to policy-makers working with quantifiable targets, at multiple geographical scales and across disciplinary sectors (Rockstrom et al. Citation2009; Steffen et al. Citation2015). Informed governance can lead to policies, planning, and codes supporting best practices for sustainable development (Griggs et al. Citation2013, Citation2014).
Therefore, in the necessary transformation for a development that is fully sustainable, Information Technology (which is already fuelling the Digital Transformation of our Society) plays a critical role (UN DESA Citation2013). In particular, it is of paramount importance to leverage the sophisticated analytics and projection technologies (e.g. physical, statistical, and Machine Learning models) and generate the knowledge required by the decision-makers. These models are becoming more and more efficient and reliable, due to the availability of a staggering amount of EO datasets, which are acquired daily. Big data generation is another important change carried out by the Digital Transformation of our Society. Significant international programmes and initiatives, dealing with Global Change and contributing to the SDG agenda implementation, has investigated the systematic generation of knowledge from data. The manuscript will introduce and discuss valuable developments carried out by members of the Group on Earth Observation (GEO), in this area.
1.1.1. Earth observation data and information
To address global sustainability challenges, timely and reliable access to environmental data and information is necessary. Data provide the foundation for reliable and accountable scientific understanding and knowledge to support informed decisions and evidence-based policy advice (Giuliani et al. Citation2017b). Consequently, achieving the objective of a sustainable development requires the integration of various data sets describing physical, chemical, biological and socio-economic conditions (Lehmann et al. Citation2017). All together, these different data allow characterizing a given location on Earth. When combined, environmental data allow monitoring and assessing the status of the environment at various scales (e.g. national, regional, global), understanding interactions between different systems (e.g. atmosphere, hydrosphere, biosphere), and model future changes (Costanza et al. Citation2016).
In this context, EO data refers to measurements of variables related to the various components of the system Earth (e.g. oceans, land surface, solid Earth, biosphere, cryosphere, atmosphere and ionosphere) and their interactions. These measurements are obtained by individual or combined, fixed or mobile sensing elements, being instruments or human observers, either in situ or through remote sensing (European Commission Citation2014). In particular, the GEO distinguishes two different broad categories of EO data: ‘In situ observations’ that are understood as observations captured locally, i.e. within a few kilometres of the object or phenomenon being observed – these include measurements taken for instance at ground stations, by aircraft and probes, ships and buoys. By contrast ‘remote sensing’ data encompasses observations made at a larger distance – this refers typically to space-borne Earth observations. EO data is a valid and globally consistent source of information for monitoring the state of the planet and increasing our understanding of Earth processes (Giuliani et al. Citation2017a).
1.1.2. The global earth observation system of systems (GEOSS)
GEO has been established in 2005 in response to the need for coordinated, comprehensive, and sustained observations related to the state of the Earth (Anderson et al. Citation2017). GEO is coordinating the implementation of GEOSS, a global and flexible network of content providers connecting together existing observing systems around the world and allowing decision makers to access a wide range of information (GEO secretariat Citation2015). GEOSS increases our understanding of Earth processes and enhances predictive capabilities that underpin sound decision-making: it provides access to data, information and knowledge to a wide variety of users (Nativi et al. Citation2015). GEO works across eight Societal Benefit Areas (SBA) (e.g. Biodiversity and Ecosystems, Food and Agriculture, Water) to translate Earth Observations into support for decision making. GEO global engagement priorities include supporting the UN 2030 Agenda for Sustainable Development, the Paris Agreement on Climate, and the Sendai Framework for Disaster Risk Reduction (GEO secretariat Citation2015; Giuliani et al. Citation2017b; CEOS Citation2018). These three global policies are a universal call to action to end poverty, protect the planet and ensure that all people enjoy peace and prosperity. GEO is instrumental in integrating EO data for measuring and tracking progresses towards these various indicators frameworks in support of effective policy responses for adaptation, mitigation, resilience (GEO Citation2015).
1.1.3. Knowledge bodies generation and management
In 2015, the GEO Ministerial declaration ‘affirms that GEO and its Earth observations and information will support the implementation of, inter alia, the 2030 Global Goals for Sustainable Development’ (GEO Ministerial Declaration Citation2015). For GEOSS, supporting such vision and strategic objectives requires a change of paradigm, moving from a data-centric approach to a more information and knowledge-centric approach. For addressing this evolution, the System-of-Systems (SoS) framework (developed by GEOSS) may refer to the well-known Data-Information-Knowledge-Wisdom (DIKW) paradigm (Ackoff Citation1989; Rowley Citation2007). In DIKW, information is an added-value product resulting from the comprehension of available data and their relations with physical and/or social phenomena. In turn, knowledge is generated by understanding information and elaborating valuable patterns.
In the context of an EO SoS, a set of main elements (along with the methodologies for their generation) were recognized as connecting links for generating knowledge from EO and non-EO data – e.g. social and economic datasets. These elements are: (a) Phenomenon Essential Variables (EVs), (b) Environmental Priority Indicators; (c) Environmental and socio-economic indices; (d) Goals and Targets (Nativi, Mazzetti, and Santoro Citation2016; Reyers et al. Citation2017). Their generation and use requires for the development of a SoS Knowledge Base (KB) and its related management processes. These new challenges were considered as part of the new GEO Foundational Task (called GCI Operation), introduced in 2016 (GEO Citation2015). The GEOSS KB management process is evolving the GEOSS Software Ecosystem into a GEOSS Social Ecosystem (Nativi et al. Citation2017; GEO Citation2018). The KB management process includes the following main phases (Liebowitz Citation1999; Evans, Dalkir, and Bidian Citation2014): (a) collect knowledge; (b) formalize knowledge; (c) publish knowledge; (d) access knowledge; (e) use knowledge; (f) update knowledge. shows the knowledge body lifecycle outlying the high-level services for knowledge management and the related main challenges, for the scope of this manuscript.
1.2. DIKW pattern applied to policy for sustainable development
1.2.1. From EO data to EVs and to indicators
In the recent years, the outstanding advancement in information science and technology made terms like Data, Information, and Knowledge ubiquitous. They are used to name emerging sub-disciplines and methodologies like data engineering and data science, information management, knowledge management, etc. or to identify computer science related processes like data mining, information processing, knowledge generation which are now paradigmatically applied to disciplinary sciences (Bates Citation2000) – for example, in modelling biological systems as information processing systems. Unfortunately, even due to their use in different domains for different objectives, such key terms do not have a shared definition. Also inside the information science community, where they originated, they are perceived as elusive concepts, and difficult to characterize (Floridi Citation2017). Therefore, they have different definitions in different communities (e.g. information sciences, disciplinary sciences, philosophical sciences) and in many cases, they are simply used without any formal definition.
In the scope of this manuscript, to provide a definition of Data, Information, Knowledge and Wisdom terms, it useful to note that, although they are often used interchangely or with overlapping meaning, there is a general consensus about two key facts:
These terms refer to different concepts;
These terms can be ordered from the lowest semantic level of Data to the highest one of Wisdom.
This brings to the concepts of a DIKW hierarchy (a static representation of Data, Information, Knowledge and Wisdom ordering) and a DIKW pattern – representing the dynamic process of semantic content extraction when moving from Data, to Information, Knowledge and finally, Wisdom (Rowley Citation2007). In DIKW, Information may be defined an added-value product resulting from the comprehension of available Data and their relations with physical and/or social phenomena. In turn, Knowledge may be defined as artefact generated by understanding Information and elaborating valuable patterns. In some cases, the DIKW hierarchy is represented as a pyramid to highlight the intuitive idea that the generation of a small quantity of Wisdom, requires an increasing quantity of Knowledge, Information and Data.
Although the DIKW representation (as a hierarchy, pattern, or pyramid) suffers from many points of weakness (e.g. lack of a clear definition of terms; unclear ordering relation; unclear definitions of quantitative aspects), it can still be useful as a framework for communicating general concepts and their relations. In particular, the DIKW pattern fits well in the representation of the process for supporting policy and decision-making, starting from the collection and analysis of raw data.
As already discussed, in the recent years, several policy goals have been set: they include the 17 UN SDGs, the objectives of the Sendai Framework on Disasters, and the objectives of the Conference of Parties 2015 on Climate (COP21). The achievement of these policy goals can be measured in respect of specific policy targets. The assessment of targets, and in general the definition of possible actions towards their fulfilment, require informed decision-making. Therefore, policy-makers are asking the scientific community to provide the necessary knowledge for evidence-based decision-making. This results in the necessity of extracting Knowledge from the big amount of collected Data (in particular EO one) and from the available socio-economic Information.
This transition from Data to Knowledge requires filling the wide gap between acquired data, and policy targets and goals. A procedure, proposed and elaborated in some recent research and innovation projects dealing with GEOSS, consists in adopting a systematic approach, regardless the goal nature and domain. This is depicted in . First, acquired (or observed) Data are processed to generate Information in form of relevant physical parameters named EVs. Then, EVs can be processed to summarize the Knowledge in one or more Indicators or Indices, whose value is related to a specific Target. Finally, by comparing the Indicators/Indices value from a real or simulated situation against a given Target, it is possible to assess and evaluate the progress toward the fulfilment of the specific policy goal represented by the Target. In this case, the Indicators/Indices represent the Knowledge necessary to policy-makers for wise decisions, which is elicited from the Information conveyed by the EVs, which, in turn, are generated from the observed Data.
In this process, the procedures for generating EVs first and Indicators/Indices later play a fundamental role. Open Science (i.e. knowledge bodies sharing and re-usability) would require the formalization (i.e. full documentation) of these procedures to justify the Information and Knowledge generation – i.e. EVs and Indicators/Indices values. These procedures can be formalized as workflows involving data transformations and scientific models.
2. GEOSS KB management
Each EV can be seen as a valuable piece of knowledge (i.e. a knowledge body), which must be managed through its own lifecycle, as a constituent part of the GEOSS KB. In the following, we will write ‘knowledge’ to refer to any kind of knowledge in a general sense, and ‘Knowledge’ to refer to the proper use of the term in the sense specified above in the DIKW pattern. Referring to , EVs lifecycle consists of five different phases. Each phase is discussed in the next sections.
2.1. Phase I: knowledge collection
2.1.1. EVs scope and definition
2.1.1.1. Scope
Recognizing the need to adequately describe the different components of the Earth system (e.g. atmosphere, geosphere, hydrosphere, and biosphere) and to ensure that observational data can deliver actionable information to the largest number of users, the concept of EVs is increasingly used in different communities (Reyers et al. Citation2017). The EVs definition process allows identifying those variables that have a high impact and should have priority in designing, deploying and maintaining observation systems and making data and products available. The concept of EVs assumes that there exists a (minimal) number of variables that are essential to characterize the state (and trends) of a given system, without losing significant information. This set of variables needs to be observed, if past changes in the system must be documented as well as if predictability of future changes must be developed. Identifying this set of EVs allows for a commitment of inherently scarce resources to the essential observation needs. It also supports and eases the management of data and observations all along the chain from the measurement of raw data, through the processing and to the delivery of products, information and services needed by end users.
In a multi-organizational and multi-disciplinary SoS, interoperability is certainly a major challenge (Domenico et al. Citation2006; Nativi et al. Citation2015; Santoro, Nativi, and Mazzetti Citation2016). There exist different types of interoperability levels (e.g. composability, semantic interoperability, structural & syntactic interoperability, integratability) (Tolk, Diallo, and Turnitsa Citation2007) to be considered as required by the diverse kinds of integrative science to be pursued – e.g. multi-disciplinary, inter-disciplinary, participatory, etc. (Vaccari et al. Citation2012). Semantic interoperability is extremely important for achieving multi- and inter-disciplinary systems. The definition of EVs helps semantic interoperability development, in a significant way by contributing to introduce a common lexicon and an unambiguous formalization of the features and behaviour of these variables, for a given scope.
2.1.1.2. Definition and outlook
The development of a specific set of EVs is a community process leading to an agreement on an essential set of parameters to meet the objectives of a given community and to support national to global monitoring, reporting, research, and forecasting (Reyers et al. Citation2017). EVs are crucial for the creation of practice-relevant knowledge covering two aspects: (1) technical and (2) social/policy relevance.
The EVs concept was first defined by the climate community, by the Global Climate Observing System (GCOS), which agreed on a set of 50 Essential Climate Variables (ECVs). This was done to respond to a general call for more coordinated and consistent approach to climate observations (Ostensen, O’Brien, and Cooper Citation2008; Hollmann et al. Citation2013; Bojinski et al. Citation2014; Zeng et al. Citation2015) – examples of ECVs are: precipitation, cloud properties, ozone, water vapour at the surface, and wind speed and direction in the upper-air. These variables are required to support the work on the United Nations Framework Convention on Climate Change (UNFCC) and the Intergovernmental Panel on Climate Change (IPCC) (Bojinski et al. Citation2014). ECVs are selected based on their relevance for characterizing Earth’s climate system as well as their technical and economic feasibility for systematic observations (Giuliani et al. Citation2017b). ECVs are now widely used in both science and policy domains; they are regularly reviewed to adapt to the need posed by new priorities, novel knowledge and recent innovations.
Since then, other scientific communities have followed a similar approach. The more mature EVs are in Climate, Ocean, and Biodiversity domains (Lindstrom et al. Citation2012; Pereira et al. Citation2013). Other communities are currently working on defining a common set of EVs, such as Water, Agriculture, and Ecosystems (GEO Citation2014; ConnectinGEO Citation2016). In particular, a long work on the Essential Biodiversity Variables (EBVs) has further clarified the role of EVs lying between primary observations and indicators (Geijzendorffer et al. Citation2015). Such a definition allows accommodating both the diversity of data providers and the changing demand for indicators across regions and different policy needs (Reyers et al. Citation2017).
It is noteworthy how the identification of EVs has depended on use-cases. In principle, system state is a concept that is independent of the addressed user scenarios; however, it is generally impossible to have a complete system representation that is valid and accurate for any potential scenario. On the contrary, in scientific practice, it is usual to design physical and computational models tailored to specific objectives. Therefore, although we can talk about domain-related EVs, in practice, we should consider scenario-related EVs. For example, if we consider the assessment of UN SDG, we will be able to identify UN SDG EVs (Reyers et al. Citation2017). Indeed, the UN SDGs allow identifying the required Indicators (and Indices) which, in turn, allow defining the required knowledge models to generate them from a set of physical parameters: the EVs.
The H2020 ConnectinGEO projectFootnote1 reviewed a set of EVs developed in the framework of several GEO communities. This work revealed that there exist: (a) different definitions of EVs, (b) diverse levels of maturity, and (c) a considerable overlap between EVs identified by different communities (ConnectinGEO Citation2016). The total number of EVs reviewed by ConnectinGEO was 147 (ConnectinGEO Citation2016). Some of the EVs are actually not just a single variable, but a cluster of several ones. The community that has defined the highest number of EVs is currently the Climate one, GCOS,Footnote2 covering (with its ECVs) one-third of the total number. Most of the ECVs are also relevant to the other GEO SBAs or themes. Others communities already working on a mature set of EVs are the Weather and the Ocean ones – respectively, led by: WMO and its Global Atmosphere Watch: GAW,Footnote3 and the Global Ocean Observing System (GOOS).Footnote4 The EVs discussion and related work is growing fast in the Biodiversity (Scholes et al. Citation2012; Pereira et al. Citation2013; Geijzendorffer et al. Citation2015), Water (Duffy and Leonard Citation2013) and Energy communities; while, in other areas like Agriculture, Disasters, Ecosystems, Health, and Urban Development, the work on specific EVs is still in the initial stage. Fortunately, they can rely on several EVs already identified in areas that are also relevant to them.
In general, a great overlap between communities exists in terms of identified EVs. This suggests that each community, working on identifying EVs for their specific purposes, should first review the currently available EVs. Such an approach would allow taking stock of the work already done and recognizing those variables that are cross-cutting. In this way, it will be easier to advocate for further improvements (in terms of methodologies, accuracy, spatial and temporal sampling, etc.) on a small number of key variables. outlines the main interrelations among EVs, as suggested by the communities themselves and recognizing the EV definitions overlapping – from the ConnectinGEO survey. To accommodate the different views recognized by its survey, ConnectinGEO proposed a broad definition of EVs: ‘a minimal set of variables that determine the system’s state and developments, are crucial for predicting system developments, and allow us to define metrics that measure the trajectory of the system’ (ConnectinGEO Citation2016).
Table 1. Main interrelations among community essential variables, according to the ConnectinGEO survey.
2.1.2. Indicator/indices scope and definition
Monitoring and assessment of policy goals are carried out through the comparison of the current (or simulated) state of the universe of discourse (e.g. a system or a process) with the desired state represented by a policy target. The state is commonly identified using significant figures called Indicators and Indices. Generally, the Organization for Economic Co-operation and Development (OECD) and the European Commission Joint Research Centre (JRC) define an Indicator as:
a quantitative or a qualitative measure derived from a series of observed facts that can reveal relative positions (e.g. of a country) in a given area. When evaluated at regular intervals, an indicator can point out the direction of change across different units and through time. In the context of policy analysis […], indicators are useful in identifying trends and drawing attention to particular issues. (OECD Citation2008)
the representation of statistical data for a specified time, place or any other relevant characteristic, corrected for at least one dimension (usually size) so as to allow for meaningful comparisons. It is a summary measure related to a key issue or phenomenon and derived from a series of observed facts. Indicators can be used to reveal relative positions or show positive or negative change. (Eurostat Citation2014)
a parameter, or a value derived from parameters, that points to, provides information about and/or describes the state of the environment, and has a significance extending beyond that directly associated with any given parametric value. The term may encompass indicators of environmental pressures, conditions and responses. (UNSD Citation1997)
formed when individual indicators are compiled into a single index on the basis of an underlying model. The composite indicator should ideally measure multidimensional concepts which cannot be captured by a single indicator, e.g. competitiveness, industrialization, sustainability, single market integration, knowledge-based society, etc. (OECD Citation2008)
2.1.3. WFs scope and definition
The generation of EVs from Data and, in turn, of Indicators/Indices from EVs, generally requires the execution of workflows implementing a business process consisting in multiple steps of datasets/variables collection, transformation and processing. This is commonly achieved by applying scientific models and knowledge management procedures.
According to the Workflow Management Coalition (WfMC)Footnote5 a business process is ‘a set of one or more linked procedures or activities which collectively realize a business objective or policy goal’ (Workflow Management Coalition Citation1996). Thus, a business process may include both manual and automated activities. While, a workflow is defined as ‘the automation of a business process, in whole or part, during which documents, information, or tasks are passed from one participant to another for action according to a set of procedural rules’ (Workflow Management Coalition Citation1996). Business process management technologies can be used to implement integrated modelling and support environmental management and Global Change policy development (Santoro, Nativi, and Mazzetti Citation2016).
Scientific workflows have emerged to tackle the problem of excessive complexity in scientific experiments and applications. They provide a formal description of a process for accomplishing a scientific objective, typically expressed in terms of tasks and data (i.e. formal encoding of measurement, observation, simulation or processing outcome) dependencies among them. They allow users to easily express multi-step computational tasks – for example, retrieve data from an instrument or a database, reformat the data, and run an analysis. For a scientific workflow, one of the major property is the data flow management. Workflows can vary from simple to complex ones: tasks in a scientific workflow can be very different, from short serial tasks to very large parallel tasks, surrounded by a large number of small and serial tasks used for pre- and post-processing. Commonly, workflow are recursive – i.e. a task can be implemented by a sub-workflows used as a subroutine in a larger workflow. Finally, in a distributed service-oriented environment, tasks can be implemented by either a local or a remote service.
2.1.4. KB to support the DIKW pattern
To support the DIKW pattern for policy-making (i.e. to derive the final Knowledge to be provided to decision and policy makers), several knowledge bodies are necessary; they can be:
structured bodies (like data ) that formalize the different aspects of the DIKW pattern to enable machine-to-machine interaction and, hence, automatic processing;
unstructured bodies (like publications) that are intended for human-to-machine interaction and complement the knowledge automatically generated by the system.
Structured knowledge bodies comprise general and domain ontologies (to represent relevant concepts and their inner relationships) and vocabularies listing the individual realizations of each concept. For example, the concepts of Data, EVs, Indicators/Indices, and Workflows, along with their relationship, should be part of a general ontology for policy-making. Moreover, for each different domain, vocabularies defining instances of these concepts (i.e. Data, EVs, Indicators/Indices, and Workflows) should be made available. Properly formalized, such knowledge bodies would allow:
To make inferences – for example, by answering to questions like: which indicators can be generated from a specific data source? Which EVs are required to generate a specific Indicator?
To automate the knowledge generation process – for example, by selecting, as input of a given scientific model, only those data sources that are semantically correct.
Unstructured knowledge bodies include every knowledge artefact for human users, such as peer-reviewed publications, manuals, web pages, videos, etc. All these kinds of knowledge bodies should be collected and connected in a dedicated KB. One of the KB core functions is to facilitate the linkage between policy goals and targets to EVs. The targets are connected to indicators that act as ‘report cards’ for the progress towards the targets. EVs need to be monitored in order to allow indicators quantification.
Commonly, KB is implemented as a searchable database of content that forms a body of knowledge about a particular domain. For supporting sustainable development policy, a KB aims to go beyond that and implement a business ecosystem platform to facilitate knowledge sharing, content management and communication. In particular, the ecosystem should facilitate the implementation of those artefacts that are essential to assess different policy frameworks. To achieve that, the KB has to collect, formalize, share and facilitate the use of a set of knowledge bodies, including: data, processing algorithms/codes, business process models (implemented as workflows), publications, computing infrastructures references, etc. In GEOSS, for instance, the following definition was proposed for the GEOSS KB: ‘A dynamic and evolvable information framework, organized as a repository of existing and distributed knowledge bodies, to facilitate Information and Knowledge generation and sharing from Earth Observations’ (Nativi, GEO Plenary Citation2016).
2.2. Phase II: knowledge formalization
2.2.1. EVs formalization for discovery and use
2.2.1.1. Description elements
Multi-source data integration is fundamental for multi-organizational and interdisciplinary frameworks – and in particular for environmental monitoring (Domenico et al. Citation2006; Nativi et al. Citation2015). Metadata (i.e. resource description) is recognized as a primary instrument to enable efficient and effective discovery and use of (environmental) data (Litwin and Rossa Citation2011; Giuliani et al. Citation2016). Describing EVs through standardized metadata is an essential condition for effective discovery and use. To enable an effective data (re-)use, data description (i.e. metadata) should provide precise information on data quality and uncertainty. These elements are essential to support meaningful decision-making processes (Cruz, Monteiro, and Santos Citation2012; Hong, Zhang, and Meeker Citation2018; Bustin et al. Citation2013). To turn data into usable information and knowledge, there is the need to utilize lexical and semantic tools (Nativi et al. Citation2015; Santoro, Nativi, and Mazzetti Citation2016). These tools help facing metadata heterogeneity (i.e. the diversity of metadata structures and content) and providing harmonized access to and sharing of the vast volume of data characterizing complex domain. In particular, ontologies can be used to describe the spatial and temporal relationships charactering a domain concepts (Domenico et al. Citation2006; Nativi, Mazzetti, and Geller Citation2012); this allows to link different data sources and models to the recognized EVs.
2.2.1.2. Expert elicitation
As depicted in , knowledge about data comes from different stakeholders (i.e. experts) involved in the process of its generation, evaluation, and use. Some of them create the original data description (generating the first metadata), while others may augment such a description by either enriching original metadata, or by using tags and annotation instruments to generate new metadata elements; in particular:
Data producers know what is the intrinsic content and quality of a data source (e.g. the acquisition process, the sensitivity of the instrument, etc.); they commonly create the first metadata corpus;
Data providers know the processing and transformation executed by exposed access services (e.g. interpolation, re-projection, the exposed encoding formats, etc.); they can augment the metadata corpus provided to users;
Data users may provide additional information associated to a given dataset, including: feedbacks, about the overall fitness for purpose of the dataset for a specific application, and associations with other useful resources or tools – e.g. visualization and processing tools.
The new elements generated by Users are usually managed as a separate metadata corpus. While, direct metadata enrichment (see ) contributes new knowledge to the existing metadata description, by either adding new metadata fields or updating the existing ones – e.g. new keywords can be added from a controlled vocabulary; dataset temporal resolution can be inferred from the abstract description. In many cases, when it is not possible to modify existing metadata at the origin, metadata enrichment is implemented in a middle-layer where metadata, provided by from heterogeneous data sources, are aggregated and harmonized (Nativi et al. Citation2015).
Tagging and annotation tasks can be implemented according to the Linked Data approach (W3C Citation2018). In this case, a third-party builds a KB storing the relationships between datasets and ontologies. For example using the RDF triples, an entry in the KB can report that the dataset identified by a specific URL is of the type identified by a second URL. This approach is extremely flexible allowing to formalize knowledge not only about datasets (e.g. EVs), but also on other resource types (e.g. WFs) provided the necessary ontologies and vocabularies. It also allows generating inferences using existing RDF reasoners – although the performances can decrease with the growth of the KB.
2.2.1.3. Encoding instruments
In the EO domain, there exist several initiatives and programmes aiming at recognizing and formalizing well-known (scientific) variables – for example, the Climate and Forecast (CF) convention standard names,Footnote6 the Community Surface Dynamics Modeling System (CSDMS) standard names,Footnote7 EEA GEMET,Footnote8 and NASA SWEET.Footnote9 Also for formal encoding languages and schemas, there exist some collaborative community-based activities to create, maintain, and promote schemas for structured data on the Internet. A valuable example in point is Schema.org vocabularyFootnote10 covering diverse domains and supporting different encodings, including RDFa, Microdata and JSON-LD (W3C Citation2018). Indeed, the use of URIs in RDF facilitates a marketplace of terms and vocabularies.
Regarding data quality formalization, it is important to distinguish between data providers view (e.g. quality check and completeness) and user view (e.g. information on dataset fit-for-purpose, usability, and user feedbacks). Interoperable representations of data uncertainties and quality can be achieved by using well-defined conceptual models and their related formal languages (e.g. Uncertainty Markup Language: UncertML and Quality ML), which support the specification and quantification of data quality and uncertainties (Cruz, Monteiro, and Santos Citation2012; Bastin et al. Citation2013; Scanlon et al. Citation2015). There are several online resources that reference ontologies by simply matching a theme to a URI – see, as a good example, the Protege ontology library.Footnote11 However, as for data resources, ontology quality assessment must be carefully considered (Gurk, Abela, and Debattista Citationn.d).
In a global and multi-disciplinary system like GEOSS, for example, the KB should build on previously introduced instruments and initiatives, in keeping with its system-of-systems philosophy. Due to the large number of data (i.e. the metadata records describing datasets) managed by GEOSS, GEOSS KB is expected to benefit from a diversified approach: mixing metadata enrichment and a linked data representation of knowledge bodies capturing their relations with other existing resources. For example, GEOSS KB would run inference processes, against the metadata base built by the GEOSS Platform, and link models/WFs artefacts to relevant datasets and processing platforms.
2.2.2. WFs and models formalization for discovery and use
The creation of scientific workflows requires the capability to discover and use all the components involved in a multitask procedure: not only data but also scientific models and algorithms. Therefore, the KB must provide sufficient knowledge about data and models/algorithms to support system composability for integrated modelling and interaction with relevant data sources. Referring to the conceptual model of interoperability levels (Tolk, Diallo, and Turnitsa Citation2007), proposed by VMASC (Virginia Modeling, Analysis and Simulation Center), WFs are required to implement the semantic composability level, where the data meaning is shared and the content of information exchange requests are unambiguously defined. This interoperability level sit just on the syntactic interoperability level, where the information exchange format is unambiguously defined – this level is commonly achieved using the existing interoperability technologies, and in particular, the standardization and brokering services.
Therefore, to achieve systems composability, in a given domain, it is fundamental to formalize and use the ontologies expressing the relevant components and their relations, as well as the semantics of the information to be exchanged among the components. Though, it is recognized that expressing semantics and ontologies for enabling Integrated Environmental Modelling (IEM) frameworks is a complex task, due to the requirement of transdisciplinarity, the need of linking models not designed for integration (Laniak et al. Citation2013), the significant variety and quantity of existing models, and the complexity of their description (Cavalcanti et al. Citation2002).
The GEO Model Web initiative (Nativi, Mazzetti, and Geller Citation2012) contributes addressing this challenge by providing a general conceptual framework for model composition. The proposed framework introduces relevant concepts like Model Representation, Model Run and their relationships with Data. It also suggests that a Model Description must base on present community activities dealing with the definition of domain ontologies, vocabularies and other knowledge artefacts. Finally, it distinguishes between the role of Scientists (expert in the scientific process) and IT Professionals (expert in process encoding and run) (Santoro, Nativi, and Mazzetti Citation2016).
The elicitation of uncertainty sources and propagation effects in models become an essential step for the description of WFs in the GEOSS KB. The representation of such uncertainty would allow evaluating models and composing them in WFs, which are supposed to provide information on the quality and uncertainty of generated outputs – i.e. datasets and indicators. Quality and uncertainty have an important role in IEM frameworks: to characterize the quality and uncertainty of data throughout the entire processing chain, it is necessary to trace and describe the quality and uncertainty impact of and propagation through environmental models. On one hand, a model can increase the existing uncertainty of input data due to non-linear effects. On the other hand, it can introduce new uncertainty due at least to: (a) structural uncertainty – intrinsic approximation to reality; (b) representation uncertainty – discretization and projection approximation for space and time dimensions; (c) parameters uncertainty – parametrization and (d) numerical uncertainty – numerical approximation of mathematical models (Bastin et al. Citation2013).
2.2.2.1. Formalization languages
Workflows can be formalized using standard representations like the Business Process Model and Notation (BPMN).Footnote12 This notation allows expressing abstract processes ranging from the full Workflow to the single scientific model. BPMN, introduced by the Business Process Management Initiative (BPMI), is a formal (and graphical) process notation. The objective of BPMN is to support business process management, for both technical users and business users, by providing a notation that is intuitive to business users, yet able to represent complex process semantics. The BPMN specification also provides a mapping between the graphics of the notation and the underlying constructs of execution languages, particularly Business Process Execution Language (BPEL) by OASIS.Footnote13 Presently, the specification is standardized by the OMG (Object Management Group),Footnote14 endorsed by WfMC, and broadly adopted across the industry. BPMN 2.0Footnote15 (released in Jan 2011) introduced the ability to serialize process models and diagrams. The XPDL standard from WfMC covers how to store and interchange process definitions. For example, XPDLFootnote16 provides a file format that supports every aspect of the BPMN process definition notation including graphical descriptions of the diagram, as well as executable properties used at run time.
Another popular WF encoding language is SCUFL2Footnote17 introduced for specifying Taverna workflows. SCUFL2 defines a model, a workflow bundle file format, and a Java API for working with workflow structures. SCUFL2 is the workflow language for Taverna 3 (by Apache),Footnote18 and replaces the old t2flow format.
Still, WF notations do not express the full knowledge on WF (or BP model) that a KB should and could provide. The main goal of these notations is to represent processes (sometimes complex) in terms of components and the relations existing among them – i.e. formalizing how WF elements are linked together. What is missing is the description (including the meaning) of the process as a whole, of its components, and ‘why’ they are connected in a given way. For instance, let us consider a WF linking the output of model A as an input of model B: generally, WF notations provide the necessary means to specify that model B execution must wait for model A successful execution, and model A output must be ingested into model B. On the other hand, a KB should also provide information about why it is scientifically sound to link models A and B in such a way and for a given purpose. Beside the formal representation of WF, a KB should provide semantic information about the WF itself, and about how this links to input and output dataset or dataset categories (e.g. EVs, Indicators).
2.2.2.2. The ECOPOTENTIAL proof-of-concept
Recognizing that terrestrial and marine ecosystems provide essential services to human societies, the European Commission ECOPOTENTIALFootnote19 project aims, as a general objective, at maximizing the benefits from the investments made in EO data and information when developing terrestrial and marine ecosystem models and sustainable ecosystem services. To this end, ECOPOTENTIAL created a unified framework for ecosystem studies on protected areas. In particular, for better exploiting the potential of EO data, ECOPOTENTIAL designed and implemented a Virtual Laboratory (VLAB)Footnote20 enabling the execution of formalized WFs (Nativi, Mazzetti, and Santoro Citation2016). VLAB gives access to a wide range of datasets by brokering open EO data services provided by heterogeneous systems – including, GEOSS, OBIS,Footnote21 DEIMS,Footnote22 GBIF,Footnote23 and relevant Copernicus Integrated Ground Segment components.Footnote24 Moreover, through VLAB, ecosystem scientists and modellers can share their models published either as a Web service or as a source code available on an open repository (e.g. GitHubFootnote25). They can also publish workflows (represented in BPMN 2.0) based on available datasets and models for generating knowledge. End users (e.g. decision-makers such as Protected Area managers or their technical staff) can run the published WFs, on selected data inputs, for generating EVs or indicators on a selected area (e.g. one of the ECOPOTENTIAL Protected Areas). VLAB orchestrates the actions needed to implement the required workflow: (a) it accesses and harmonizes the required datasets; (b) it compiles source codes, if needed, and publishes them as Web services on public clouds; (c) it invokes the required Web services; (d) it retrieves output datasets. The VLAB itself runs on a cloud infrastructure to exploit elastic storage and computing capabilities.
Although, the ECOPOTENTIAL VLAB does not provide full representation of knowledge, it is a valuable example of how knowledge bodies can be: (a) shared – in form of scientific models; (b) exposed as an online service – i.e. Web service, either existing or created on-the-fly from source code; (c) generated – by running WFs. Through VLAB, a user can discover scientific models and WFs, visualize them in a formal way, and run them. The models are classified using a set of concepts well described in a domain vocabulary: Ecosystem, Protected Area, and Storyline. For instance, shows the BPMN visual notation by which the ECOPOTENTIAL Community represents and uses the ‘Earth Observation Data for Ecosystem Monitoring (EOSDM) model’ (Lucas and Mitchell Citation2017) on VLAB.
2.2.3. Formalization of other knowledge bodies for discovery and use
In addition to data, models, and WFs description, other knowledge bodies can be incorporated in a KB dealing with science-policy interface in order to fill the gap between scientific information and decision-making. Probably, one of the most interesting knowledge body is on scientific publication: this is asked to formalize the knowledge contained in scientific publications linking them to the other relevant artefacts managed by the KB – i.e. data, algorithms, and WF. For instance, let us consider the original article presenting the Normalized Difference Vegetation Index (NDVI) (Rouse et al. Citation1974): it is possible to recognize the algorithm and understand which data types are required to compute the index. Once more, ontologies and vocabularies are useful to effectively formalize the extracted knowledge and implement sound links to existing artefacts – i.e. relevant models/WFs and datasets. Finally, the manuscript can be also linked to the relevant scientific literature on this subject, contributing to strengthen its scientific credibility – as well as that of its implementing WFs.
Recently, several scientific journals publishers (e.g. Nature, Elsevier, and Taylor & Francis) decided to strongly encourage the deposition of the data and software that contributed to reach the scientific results presented in a published manuscript. In the spirit of the Open Science movement, this is to allow the full assessment (and where possible the reproducibility) of the presented scientific results. This is building and formalizing a significant set of KBs by linking publications, data, and software resources. Besides, most of the editorial platforms expose specialized Application Programming Interfaces (APIs) allowing clients and users to query such KBs and access the matching results – see for example AGU open APIsFootnote26 and EGU online platforms.Footnote27 KB to support policy for a sustainable development should build interoperability with such knowledge frameworks, making use of these APIs.
2.3. Phase III: knowledge sharing
Sharing formalized knowledge entails to make possible its use, for example, by enabling third-parties to discover, access, and use the described artefacts – e.g. datasets, EVs, algorithms, and workflows. Knowledge sharing may also include the support for the execution of discovered WFs and the generation of new information and knowledge – such as indicators and indexes. Beyond that, a well-managed KB should also enable inferences generation to answer to high-level questions, such as: which models are available to generate a specific indicator? Which indicators can be generated from a specific dataset? This advanced capability might also be exposed to underpin semantic search functionalities.
In the present Web era, resources sharing is commonly supported by exposing selected functionalities through dedicated interfaces (Richardson and Ruby Citation2007). The design and development of these interfaces is a crucial task because has a strong impact on the content type to be shared and on the use performances of the KB. Two general approaches are commonly adopted, nowadays: (application) Service Interfaces and APIs.
Service Interfaces are designed to expose macro-functionalities (or coarse functionalities) that are of direct interest for the user in a given application archetype (e.g. Discovery, Evaluate, and Download) well considered by the interface publisher. The semantics of the exposed interface functionalities is commonly at the level of the application end-users. Through those interfaces, an application can ask the implementing system to perform a (macro) service, which is a task on behalf of the end-user. Since these interfaces expose high-level functionalities, they, generally, require the specification of several parameters to better tailor the request to the user needs. Therefore, Service Interfaces can result complex, although, on the other hand, they can execute sophisticated tasks with a single request. Service Interfaces fits well to scenarios where typical applications can be built with an orchestration of a well-identified set of high-level activities – i.e. those that are exposed through the service interface. Service Interfaces are well-suited to standardization, since it is expected that the identified set of high-level functionalities (characterizing a service) is stable, making the, usually, long specification process convenient.
As the name implies, Application Programming Interfaces (APIs) are designed to expose fine-grained functionalities (or micro-functionalities) that are useful to software developers for creating advanced applications (Woods and Mattern Citation2006). Therefore, APIs typically work at a semantic level that is lower than the one characterizing the application end-user. For example, while a Service Interface could provide a resource representation as a map with a single request, an API could provide a way to interact with the map single tiles, or even to allow changing the way the utilized server generates maps and tiles. APIs fits well to very dynamic scenarios, where applications are not predictable, and it is necessary to expose all the relevant resources and capabilities, leaving developers free to integrate them in full (and diverse) applications. APIs are commonly introduced to support dynamic scenarios and, hence, they need frequent revision and update. Besides, they are generally tailored to work on a specific implementing platform. For these reasons, a fully standardization process may be problematic. However, since applications are often built as mash-ups using APIs of different vendors, the standardization of APIs documentation becomes fundamental to facilitate developers work (The Importance of Standardized API Design Citation2017) – see for example the SwaggerFootnote28 open source software framework backed by a large ecosystem of tools that helps developers design, build, document, and consume RESTful APIs.
For applications development, the components granularity level has recently gained importance. In software engineering, this is reflected by the introduction of the concept of microservice (Clark Citation2016). A micro-service oriented architecture style is a variant of the Service Oriented Architecture (SOA) style, with the additional constraint of fine-grained services and lightweight protocols. In this view, Service Interfaces can be considered as the way to interact with a system according to the SOA style, while APIs fit in a micro-service oriented architecture, with respective advantages and drawbacks. While Service Interface design can be community-driven to identify the smallest set of essential application services, API design is typically provider-driven, since community needs are not predictable, and, hence, the approach consists in exposing the implementing platform capabilities to application developers.
In order to support the diverse (and often still unknown) possible applications, a KB supporting sustainable development policy should expose a set of APIs that fit in a micro-service oriented architecture.
2.3.1. GEOSS Platform APIs and service interfaces
To support the development of user-driven applications (including mobile Apps), a set of APIs and Service Interfaces were developed by the GEOSS Platform (GEOSS Platform Citation2018) providing client systems, intermediate users (i.e. developers), and end-users with a set of micro- and application services to discover, evaluate, and access the millions of linked resources – which are shared by the GEO Members. These interfaces were designed at a technology-neutral level, and then consistently implemented utilizing different technologies to address diverse user categories. In fact, not necessarily all the developers and users have the same knowledge or expertise either in the use of geospatial resources, or in the utilization of a particular technology. The different implementations are described in the following paragraphs highlighting the main targeted users.
2.3.1.1. Standard geospatial web service
GEOSS Platform exposes a set of Service Interfaces that implement standardized protocols for the discovery and access of the millions of geospatial resources managed by the platform. Such interfaces are compliant with the most popular international standards for geospatial data interoperability, including: OGC CSW, WCS, WMS, and WFS; OAI-PMH; ISO 19115; Dublin Core Metadata Initiative; ebRIM; DCAT, etc. These interfaces are conceived to mainly target advanced users, who are experienced in the utilization of geospatial data (and related international standards) as well as interoperability technologies. By using one of this interface, a GEOSS user (or application developer) can interact with the GEOSS Platform as it were a single virtual geospatial data system, exposing a well-adopted geospatial standard interface.
2.3.1.2. Server-side APIs
GEOSS Platform also exposes a Web-based server-side APIs (aka Web APIs) to provide application developers with the building blocks necessary to create a Web application. Such building blocks are accessible via a set of public endpoints associated with well-defined request/response messages. Utilizing these APIs, developers can interact with the GEOSS Platform through any programming environment that allows communication via the HTTP/HTTPS protocol and JSON (or XML) message encoding/parsing. Targeted users are expert in the development of applications that communicate with remote servers over HTTP/HTTPS. These developers are required to implement the connection module(s) to interact with the different endpoints, by creating first valid HTTP requests, and then parsing the response messages.
Besides the common functionalities for the discovery and access, these APIs provide a set of advanced functionalities:
filtering the query result-set (i.e. the returned resources satisfying a query constraints) by using a set of well-defined fields that characterize any managed resource – e.g. SBA/Thematic-area, resource provider, access technology, resource encoding, etc.
extending the regular queries with semantically related keywords by using one or more lexicons whose choice may be selected by the developer.
Presently, these APIs were implemented using a couple of distinct technologies: an extended OpenSearch protocolFootnote29 and a RESTfulFootnote30 protocol. As of May 2018, the GEOSS Platform team started a process with the Open Geospatial Consortium (OGC) to standardize the REST implementation of the Server-side APIs.
2.3.1.3. Client-side APIs
In addition to the Server-side APIs, GEOSS Platform publishes a set of Client-side APIs, too. They are provided as software library that developers can use to build an application. These APIs are a high-level client-side library; utilizing that, developers are alleviated from the need of implementing low-level interaction with the constitutive components of a SoS. They allow developers to simply work with objects, representing the system resources, and avoid dealing with the system itself.
Client-side APIs are currently available in Javascript languageFootnote31; it is scheduled to support more programming/scripting languages in the next future – e.g. Python. This type of APIs mainly targets Web developers with good knowledge of W3C technologiesFootnote32 (including: HTML5, Javascript, and CSS) and facilitates the development of Web and mobile applications.
2.3.2. VLAB APIs for knowledge sharing
To share additional resources beyond datasets, APIs must provide the necessary functionalities to browse and navigate the conceptual network representing a given domain (Santoro et al. Citation2012) – for example, concepts like EVs, Indicators, Policy Goals, etc. In addition, these APIs should allow to link the domain concepts with the actual datasets and workflows, which can be used for their instantiation.
The ECOPotential VLAB exposes this type of APIs (as a RESTful implementation) to support the ECOPotential use-cases. In particular, the VLAB APIsFootnote33 provide a simple way to navigate a set of resources representing instances of the following concepts:
Domain Ontology elements – for example, ECOPOTENTIAL defined a simple Ecosystems ontology consisting of the concepts: Ecosystem; Protected Area; Storyline (narrative description of ecosystem services-related issues in one of the Protected Areas along with a scientific approach to deal with them);
Models/Workflows – in the ECOPOTENTIAL project, the models were connected to one or more Storylines.
The APIs allow to:
discover the domain concepts, their implementing resources, and the related relations – e.g. which workflows address a given storyline;
publish a new abstract Model/Workflow;
publish a new implementation of a Model/Workflow;
trigger the execution of a Model/Workflow implementation, by ingesting user-defined inputs, reading the execution status, and retrieving outputs or error messages when the execution is completed.
To discover GEOSS datasets and ingested them into a Model/Workflow, an extension of the GEOSS APIs was prototyped, too. This extension consists in the introduction of a couple of new query parameters for the server- and client-side APIs: (i) workflow identifier, and (ii) input identifier. A proof-of-concept was presented at the GEO Plenary 2018, held in Washington DC. As depicted , a user can navigate the Ecosystem domain ontology selecting first a Protected Area, then a relevant storyline for that area, and finally getting the WF associated to the storyline – the user can run the WF and generate the scenario outcome, on-the-fly. This also required an innovative GUI (developed by the European Space Agency) characterizing the GEOSS Platform.
Figure 5. The extended GEOSS Web Portal GUI, developed to demonstrate the use of VLAB with the GEOSS Platform (concept browsing on left, workflow visualization and execution on the right).
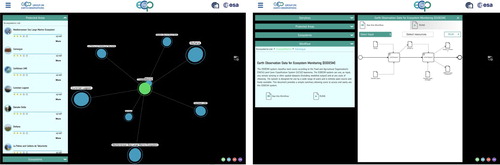
Starting from this prototype, another project funded by the European Commission: GEO-EssentialFootnote34 is developing a generalization of the VLAB APIs. The aim is to enhance the present APIs and provide a set of high-level functionalities to discover the concepts characterizing the SDGs framework (as well as other policy frameworks) and link them to the useful Models/Workflows. These APIs would play an important role for using a KB supporting sustainable development policy.
2.4. Phase IV: knowledge use
A KB providing full knowledge about existing models/algorithms and their relations with accessible datasets would allow automated composition and running of those workflows that contains such artefacts. By using the content of KB, a platform could generate valid workflows connecting models based on matching data types, or could start discovery of the datasets that are valid inputs for a specific model. The platform could then expose not only the representation of the workflow, but also the (micro-)services (via APIs) for its assisted composition, running, and results publishing.
The collection, formalization and sharing phases are the necessary preparatory tasks to make knowledge usable and enable the provision of actual services to support policy-makers. While the knowledge collection phase is essentially a preliminary step for building a KB, and the knowledge sharing phase makes possible multichannel and multimodal delivery of knowledge, it is the knowledge formalization phase that plays the central role for the actual use of knowledge. Indeed, the formalization step makes knowledge suitable for automated machine-processing, increasing the capability of generating inferences and insights from collected knowledge. In particular, the formalization of a WF in an executable language enables automatic generation of EVs and Indicators on user request. At the same time the formalization of WF relationships with datasets, EVs, and Indicators, enable advanced functionalities like automated or semi-automated discovery of suitable input datasets – including the possibility to validate WF chaining for integrated modelling.
However, the automated machine processing of WFs is not always a straightforward process. The necessary level of detail for executing a WF is definitely higher than that for its representation and visualization. This is a typical challenge encountered when WFs are formalized using a high-level representation notation – e.g. BPMN. These notations aim to document a Business Process that is not necessarily machine-executable; therefore, their translation (aka compilation) into a low-level executable representation is not always possible. On the other hand, a WF execution language (such as BPEL) can be directly executed but it requires a proper software environment – i.e. a specific workflow engine. A potential solution to keep the system flexible is to use a notation language (like BPMN) as the abstract description of an executable process – like BPEL. That means to include, as a convention, all the information required for the execution of the modelled process. In this way, the business process notation document can be converted (i.e. compiled) in any supported executable language, enabling the use of multiple workflow engines and software environments (Santoro, Nativi, and Mazzetti Citation2016).
The H2020 ECOPOTENTIAL VLAB addressed this specific challenge by adopting a set of widespread technologies. In particular, for the extended WF representations, it utilizes a BPMN convention that adds constraints to provide the necessary information for WF execution – e.g. the link to potential data sources. In addition, the single BPMN tasks are further formalized as invocation of external Web services or as software source code, stored in public archives. Thanks to this extra information, the VLAB orchestrator component is able to retrieve and compile all the necessary resources and execute the WF. Finally, the well-adopted Docker container technologyFootnote35 provides the homogeneous software environment for running the resulting executable code (Nativi, Mazzetti, and Santoro Citation2016).
In the framework of ERA-PLANET (European Research Area Network for Observing our changing Earth)Footnote36 the GEO-Essential project aims at utilizing the VLAB capabilities by formalizing the relationships among datasets, EVs, Indicators, and WFs to support policy-making applications. Moreover, an inference engine will allow reasoning either for providing knowledge to users, or to validate inputs and outputs of WFs (Mazzetti, Santoro, and Nativi Citation2018).
2.5. Phase V: knowledge update
This last phase of the knowledge management lifecycle makes use of the meta-knowledge, generated by the previous phases, to update and refine the managed knowledge body. Meta-knowledge includes some results generated by the previous phase, and in particular the recognized shortcomings and gaps. For example, to implement access to the existing EVs managed by the GEOSS Platform (i.e. the sharing knowledge phase), ConnectinGEO carried out a gap analysis and recognized several shortcomings that seem to stem from the first two phases of the EVs lifecycle, in the GEOSS framework.
2.5.1. Analysis of EVs sharing through the GEOSS Platform
2.5.1.1. The process and the developed platform
ConnectinGEO carried out a careful analysis of the EVs, which are currently managed by the GEOSS Platform, compared to a set of variables indicated by sustainable development experts (Santoro, Masó, and Nativi Citation2017). The European Commission project implemented a process to match the recognized variables (i.e. cross-cutting EVs) against the content managed by GEOSS through its platform. To carry out a constant monitoring of existing gaps, a specific platform was developed – as showed in . This analytical platform is called ConnectinGEO Analyzing Platform – empowered by the GEO DABFootnote37 technology. It provides a set of inventory and analytical services, implemented by the following components: (a) OI (Observation Inventory) Generator; (b) OI Analyser; (c) Metadata Enricher. The gap analysis process consists of the following sequential steps – that are repeated because the process is recursive:
Generation of the GEOSS Observation Inventory DB: by analysing the entries of the DB that manages the harmonized metadata, which describes the resources available on the GEOSS Platform.
Generation of the Possible Gaps DB: by analysing the list of user needs against the records of the GEOSS Observation Inventory DB.
Generation of the Enriched GEOSS Observations Inventory DB: it is generated by enriching the metadata characterizing the GEOSS Observation Inventory DB to address some of the recognized gaps.
Figure 6. The ConnectinGEO process and dedicated platform to analyse the EVs accessibility on GEOSS via the GEOSS Platform.
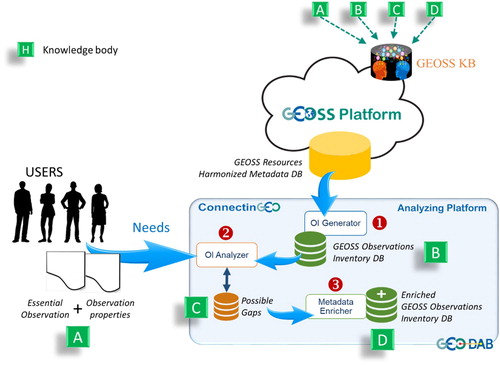
Along with the formalized user needs, all the generated DBs are knowledge bodies to be managed by the GEOSS Platform KB – labelled A, B, C, and D in . In addition, the Possible Gaps DB contributes to the meta-knowledge that enables the update phase of the GEOSS KB. Once the OI DB is enriched (i.e. the Enriched GEOSS Observations Inventory DB is worked out), this can be used to re-run the gap analysis and cycle; the following new steps may be added to the process representing a clear example of knowledge update – these phases are not showed in :
Update the Possible Gaps DB by re-running phase (2) but using, this time, the Enriched GEOSS Observations Inventory DB.
Update the Enriched GEOSS Observations Inventory DB by re-running phase (3) but using, this time, the updates of the Possible Gaps DB.
The Metadata Enricher is a very flexible component that can apply a set of different enriching modules – according to the recognized type of resource, the enricher component can set the most effective enriching modules. For the scope of the carried out analysis, two modules were utilized: (a) the Spatial Resolution enrichment, normalizing the unit of measurement in km; and (b) the Temporal Resolution enrichment, normalizing the unit of measurement in day. Both modules infer the missing information by analysing unstructured descriptions charactering a dataset such as: title, abstract, keywords, etc.
2.5.1.2. The user needs
The user needs utilized for updating the GEOSS KB are elicited and formalized by experts engaged with the ConnectinGEO project; they recognized 16 cross-cutting EVs (to be searched), along with their features to make them (re-)usable. The EVs list is reported in .
Table 2. Cross-cutting EVs, as specified by the ConnectinGEO experts.
2.5.1.3. Analysis and recognized gaps
At the end of 2016, the analysis recognized that about 10% of the GEOSS content seemed to match the list of EVs recognized by experts. All the 16 EVs are discoverable via GEOSS Platform; however, only 14 of them are accessible. Finally, only 5 of them match the desired spatial and temporal resolutions. shows the matching statistics.
Figure 7. Discoverable and accessible EVs via the GEOSS Platform (in 2016): by matching the variable standard name (A) and by matching the standard name and the desired spatial and temporal resolutions (B).
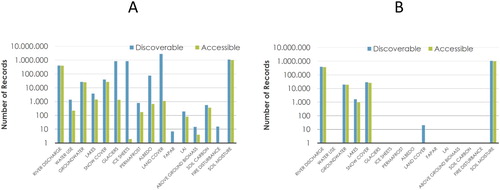
A set of possible gaps were recognized dealing with the datasets processing level, discoverability, accessibility, and granularity level. The can be summarized as:
GEOSS contains many ‘raw’ data that are instrumental to generate the searched EVs – however, users must know how to process them for that.
Discovery and access metadata are often incomplete, imprecise (or unstructured), and the granularity level is unclear – spatial and temporal extent is a clear example, and it was addressed by using the metadata enrichment approach, where possible.
Often there is no ‘direct’ access to EVs: the access is implemented via ‘landing page(s)’ and/or requires a (personal) account.
This experience confirmed that the knowledge formalization phase is extremely important to make a KB usable and, hence, support policy implementation and assessment.
3. Conclusions and discussion
Creating and monitoring integrated environmental policies requires digital frameworks to transform observations and simulations (i.e. datasets) into valuable information and extract the necessary knowledge. For these frameworks, KBs play an important role. KB dealing with global change and sustainable development policies must be seen as a business ecosystem platform to facilitate knowledge sharing, content management and communication. It is commonly implemented around a database managing diverse kinds of knowledge bodies, including: datasets, EVs, WFs, lexicons, papers, computing infrastructures, etc. Knowledge bodies must be collected in a formal way and shared on the Web to support the implementation of WFs that generate the required information and knowledge to support the environmental policies
When defining SDGs or other integrated indicators, it is of paramount importance to re-use the existing initiatives and platforms avoiding to duplicate efforts (Giuliani et al. Citation2011). In this context, EO and the connected initiatives and platforms can play an important role for enabling the creation of integrated environmental indicators. Interoperability and standardization are critical for distributed resources discovery, access, and use. Interoperability, in particular, must be implemented up to the semantic and composability levels. Integrated policy initiatives and programmes should include the necessary work to link (conceptually, operationally and institutionally) the ongoing efforts for resources sharing – i.e. the existing data, information, and knowledge management frameworks.
As for the KB management lifecycle, knowledge collection phase is the preliminary step for building a KB; while, knowledge sharing phase makes possible multichannel and multimodal delivery of knowledge. However, to enable knowledge use, the knowledge formalization phase plays a central role – as also described in the following good practices.
Indeed, besides, efficiently and effectively accessing and using EO data, the next challenge for any scientific communities is to generate and manage knowledge. To harness the power of EO data, it will be a necessity to facilitate the linkage between societal objectives and information generated from data. Therefore, having a dynamic and evolvable information framework, organized as a repository of existing and distributed knowledge bodies, certainly contributes to further close the gap between data providers and users.
3.1. Good practices for knowledge management
Surveying the present EVs lifecycle in the GEOSS framework, this project recognized a set of good practices for managing EVs, and, more generally, knowledge bodies:
3.1.1. Knowledge collection
Engage the relevant community of practices in the knowledge body collection and definition process, by establishing a permanent forum. The following stakeholders must be considered: knowledge producers, providers, users, as well as metadata enrichers.
Focus the forum discussion on recognizing those artefacts that are essential for understanding the state and developments of a specific system – all artefacts are useful, but a set of them constitutes a reference space to understand the state of a given system.
Organize workshop on the set of artefacts that describes a specific system, periodically.
3.1.2. Knowledge formalization
Formalize knowledge bodies and their related descriptions using semi-structured languages. They must be formal and flexible enough to enable machine-to-machine interoperability allowing knowledge bodies advancement and refining. Quality must be carefully considered – applying a satisficing approach.
Promote the (re-)use of existing lexicons. The use of URIs in RDF is recommended to facilitate a marketplace of terms and vocabularies. The use of linked data is also recommended to stablish relationships among knowledge bodies.
Augment and enrich the knowledge bodies description (i.e. metadata), where necessary and possible.
Support knowledge bodies composability for integrated modelling, by formalizing the ontologies expressing relevant components and their relations, as well as the semantics of the information to be exchanged among the components. The elicitation of knowledge bodies uncertainty must be carefully considered.
Formalize also the processes that are related with a knowledge body, either they are WFs to generate the knowledge body, or known Business Processes that make use of the knowledge body in a sound way. Use standard notations for that.
Build interoperability with other synergetic knowledge formalization frameworks, making use of the APIs exposed by them.
3.1.3. Knowledge sharing
Develop and expose open APIs to allow knowledge bodies discoverability, access, and use. These APIs must be able to support the development of many diverse applications, in keeping with a micro-service oriented architecture. In particular:
o Support discoverability and access of the processes (i.e. WFs and Business Processes) associated to the knowledge bodies.
o Provide functionalities to discover the concepts characterizing the SDGs framework (or other policy frameworks) and link them to the useful knowledge bodies – e.g. Models and Workflows.
Adopt standard notations to document the exposed APIs – e.g. the Swagger open source software framework.
3.1.4. Knowledge use
Facilitate the execution of the WFs and Business Processes associated with a knowledge body in order to generate new knowledge.
Enable inferences and insights generation from collected knowledge, by allowing automated machine processing – in particular enable automatic generation of knowledge bodies (like EVs and Indicators) on user request.
Enable advanced functionalities like automated or semi-automated discovery of knowledge bodies suitable to ingest complex WF and Business Models.
Keep the system flexible enough, to be technology neutral, but effective generating new knowledge. By example, if the knowledge body is a model, use a notation language (like BPMN) as the abstract description of the executable implementation – like BPEL.
3.1.5. Knowledge update
Collect the meta-knowledge generated by the previous management phases and use it to recognize existing gaps and update the managed knowledge bodies.
Address the recognized gaps.
3.2. Operational implementations and prototypes
Recently, the European Commission has funded some projects to design and develop services and prototypes to implement some of the good practices for knowledge management.
3.2.1. ConnectinGEO and the EVs knowledge body
ConnectinGEO recognized the importance of EVs as a key knowledge body towards the creation of information necessary to support the SDGs agenda – more than 140 EVs were reviewed, interacting with all the GEOSS SBA Communities. The project also outlined how the engagement of experts is necessary for the definition of EVs and the formalization of their relationships with observables and indicators/indices. Since there exist different definitions of EVs, diverse levels of maturity, and a considerable overlap between EVs characterizing different domains, ConnectingGEO proposed a broad definition of EVs. Finally, the project developed a dedicated digital platform to monitor how effectively GEOSS addresses user needs for EVs.
3.2.2. ECOPOTENTIAL and the transition from data to knowledge
The experience of the ECOPOTENTIAL VLAB showed how the recent IT advancements supports the transition from Data to Knowledge to interface Science to Policy. In particular, it demonstrated that brokering and virtualization/containerization technologies help to address the heterogeneity of several knowledge bodies (e.g. data and programming frameworks), dealing with scientific models, providing a harmonized environment for the generation of information from data. In addition, the project used semantic technologies to enable the formalization, publication and use of knowledge. ECOPOTENTIAL also confirmed that to support the full lifecycle of knowledge, the direct involvement of domain experts is necessary for the knowledge elicitation and collection phases.
ECOPOTENTIAL VLAB demonstrated a way to address the challenge of formalizing scientific models in an effective but, at the same time, flexible and portable way. To achieve that the virtual framework used a set of widespread technologies. ECOPOTENTIAL VLAB represents a valuable example of how knowledge bodies (in particular scientific models) can be shared, made accessible via online APIs, and also generated by running community-defined WFs.
3.2.3. GEO-Essential, ERA-Planet and VLAB extension for SDGs
In the framework of ERA-PLANET, the GEO-Essential project aims at extending the ECOPOTENTIAL VLAB prototype to formalize the relationships among datasets, EVs, Indicators, and WFs to support policy-making applications. Moreover, GEO-Essential is developing a generalization of VLAB APIs to provide a set of high-level functionalities to discover the concepts characterizing the SDGs framework – as well as other policy frameworks. Finally, the project will develop an inference engine to allow reasoning for providing knowledge to users.
Disclosure statement
No potential conflict of interest was reported by the authors.
ORCID
Stefano Nativi http://orcid.org/0000-0003-3185-8539
Paolo Mazzetti http://orcid.org/0000-0002-8291-1128
Additional information
Funding
Notes
References
- Ackoff, R. L. 1989. “From Data to Wisdom.” Journal of Applied Systems Analysis 15: 3–9.
- Anderson, K., B. Ryan, W. Sonntag, A. Kavvada, and L. Friedl. 2017. “Earth Observation in Service of the 2030 Agenda for Sustainable Development.” Geo-spatial Information Science 20 (2): 77–96. doi: 10.1080/10095020.2017.1333230
- Bastin, L., D. Cornford, R. Jones, G. Heuvelink, E. Pebesma, C. Stasch, S. Nativi, P. Mazzetti, and M. Williams. 2013. “Managing Uncertainty in Integrated Environmental Modelling: The UncertWeb Framework.” Environmental Modelling & Software 39: 116–134. doi: 10.1016/j.envsoft.2012.02.008
- Bates, M. J. 2000. “The Invisible Substrate of Information Science.” Journal of the American Society for Information Science 50 (12): 1043–1050. doi: 10.1002/(SICI)1097-4571(1999)50:12<1043::AID-ASI1>3.0.CO;2-X
- Biermann, F., X. M. Bai, N. Bondre, W. Broadgate, C. T. A. Chen, O. P. Dube, J. W. Erisman, et al. 2016. “Down to Earth: Contextualizing the Anthropocene.” Global Environmental Change-Human and Policy Dimensions 39: 341–350. doi: 10.1016/j.gloenvcha.2015.11.004
- Bojinski, S., M. Verstraete, T. C. Peterson, C. Richter, A. Simmons, and M. Zemp. 2014. “The Concept of Essential Climate Variables in Support of Climate Research, Applications, and Policy.” Bulletin of the American Meteorological Society 95 (9): 1431–1443. doi: 10.1175/BAMS-D-13-00047.1
- Bombelli, A., I. Serral, P. Blonda, J. Masó, H.-P. Plag, S. Jules-Plag, I. McCallum, and ConnectinGEO. 2016. EVs Current Status in Different Communities and Way to Move Forward. https://ddd.uab.cat/record/146882.
- Cavalcanti, M. C., M. Mattoso, M. L. Campos, F. Llirbat, and E. Simon. 2002. “Sharing Scientific Models in Environmental Applications.” Proceedings of the 2002 ACM Symposium on Applied Computing. New York, NY, USA: ACM, 453–457.
- Clark, K. J. 2016. “Microservices, SOA, and APIs: Friends or Enemies?” [Online]. IBM developerWorks. Accessed July 26, 2018. http://www.ibm.com/developerworks/websphere/library/techarticles/1601_clark-trs/1601_clark.html.
- Costanza, R., L. Daly, L. Fioramonti, E. Giovannini, I. Kubiszewski, L. F. Mortensen, K. E. Pickett, K. V. Ragnarsdottir, R. De Vogli, and R. Wilkinson. 2016. “Modelling and Measuring Sustainable Wellbeing in Connection with the UN Sustainable Development Goals.” Ecological Economics 130: 350–355. doi: 10.1016/j.ecolecon.2016.07.009
- Cruz, S. A. B., A. M. V. Monteiro, and R. Santos. 2012. “Automated Geospatial Web Services Composition Based on Geodata Quality Requirements.” Computers & Geosciences 47: 60–74. doi: 10.1016/j.cageo.2011.11.020
- Díaz, S., U. Pascual, M. Stenseke, B. Martín-López, R. T. Watson, Z. Molnár, R. Hill, et al. 2018. “Assessing Nature’s Contributions to People.” Science 359 (6373): 270–272. doi: 10.1126/science.aap8826
- Domenico, B., J. Caron, E. Davis, S. Nativi, and L. Bigagli. 2006. “GALEON: Standards-based Web Services for Interoperability among Earth Sciences Data Systems.” 2006 IEEE International Symposium on Geoscience and Remote Sensing. Presented at the 2006 IEEE International Symposium on Geoscience and Remote Sensing, 313–316.
- Duffy, C., and L. N. Leonard. 2013. “A Call for a Community Strategy to the 'Essential Terrestrial Variables' Necessary for Catchment Modeling Anywhere in the US (Invited).” Abstract U42A-03 presented at 2013 Fall Meeting, AGU, San Francisco, CA, December 9–13.
- European Commission. 2014. “GEOSS: Achievements to Date and Challenges to 2025.” [Online]. Accessed November 18, 2018. https://ec.europa.eu/transparency/regdoc/rep/10102/2014/EN/10102-2014-292-EN-F1-1.Pdf.
- Eurostat. 2014. Glossary: Statistical indicator. Eurostat website, “Statistics Explained”. https://ec.europa.eu/eurostat/statistics-explained/index.php/Glossary:Statistical_indicator.
- Evans, Max, Kimiz Dalkir, and Catalin Bidian. 2014. “A Holistic View of the Knowledge Life Cycle: The Knowledge Management Cycle (KMC) Model.” Electronic Journal of Knowledge Management 12 (2): 148–160.
- Floridi, L. 2017. “Semantic Conceptions of Information.” In The Stanford Encyclopedia of Philosophy, edited by E. N. Zalta. Stanford: Metaphysics Research Lab, Stanford University. Online document: https://plato.stanford.edu/archives/spr2017/entries/information-semantic/.
- Geijzendorffer, I. R., E. C. Regan, H. M. Pereira, L. Brotons, N. Brummitt, Y. Gavish, P. Haase, et al. 2015. “Bridging the Gap Between Biodiversity Data and Policy Reporting Needs: An Essential Biodiversity Variables Perspective.” Journal of Applied Ecology 35 (5): 1341–1350. doi: 10.1111/1365-2664.12417
- GEO. 2014. The GEOSS Water Strategy: From Obervations to Decisions. Online document: ftp://ftp.earthobservations.org/TEMP/Water/GEOSS_WSR_Full_Report.pdf.
- GEO. 2015. GEO Strategic Plan 2016–2025: Implementing GEOSS. Online document: https://www.earthobservations.org/documents/GEO_Strategic_Plan_2016_2025_Implementing_GEOSS.pdf.
- GEO. 2015. The Group on Earth Observations (GEO) Mexico City Declaration. Online document: http://www.earthobservations.org/documents/ministerial/mexico_city/MS3_Mexico_City_Declaration.pdf.
- GEO. 2015. The Contributions of Earth Observations in Achieving the Post-2015 Development Agenda1 a summary outcome of the GEO – UN Roundtable, Geneva - February 18, 2014. Online document: https://unstats.un.org/unsd/statcom/statcom_2015/seminars/earth_observ/GEO-UN%20SDG%20Summary%20Outcome.pdf.
- GEOSS Platform. 2018. [Online]. Accessed November 17, 2018. http://www.earthobservations.org/gci.php.
- GEOSS Platform Operation Team. 2018. Executive Summary Report of the 3rd Data Provider Workshop. Online document: https://www.earthobservations.org/documents/me_201805_dpw/me_201805_dpw_summary_report.pdf.
- Giuliani, G., H. Dao, A. De Bono, B. Chatenoux, K. Allenbach, P. De Laborie, D. Rodila, N. Alexandris, and P. Peduzzi. 2017a. “Live Monitoring of Earth Surface (LiMES): A Framework for Monitoring Environmental Changes from Earth Observations.” Remote Sensing of Environment 202: 222–233. doi: 10.1016/j.rse.2017.05.040
- Giuliani, G., Y. Guigoz, P. Lacroix, N. Ray, and A. Lehmann. 2016. “Facilitating the Production of ISO-compliant Metadata of Geospatial Datasets.” International Journal of Applied Earth Observation and Geoinformation 44: 239–243. doi: 10.1016/j.jag.2015.08.010
- Giuliani, G., S. Nativi, A. Obregon, M. Beniston, and A. Lehmann. 2017b. “Spatially Enabling the Global Framework for Climate Services: Reviewing Geospatial Solutions to Efficiently Share and Integrate Climate Data & Information.” Climate Services 8: 44–58. doi: 10.1016/j.cliser.2017.08.003
- Giuliani, G., N. Ray, S. Schwarzer, A. De Bono, P. Peduzzi, H. Dao, J. Van Woerden, R. Witt, M. Beniston, and A. Lehmann. 2011. “Sharing Environmental Data Through GEOSS.” International Journal of Applied Geospatial Research 2 (1): 1–17. doi: 10.4018/jagr.2011010101
- Griggs, D., M. S. Smith, J. Rockstrom, M. C. Ohman, O. Gaffney, G. Glaser, N. Kanie, I. Noble, W. Steffen, and P. Shyamsundar. 2014. “An Integrated Framework for Sustainable Development Goals.” Ecology and Society 19 (4): NA–NA. Online paper: https://www.ecologyandsociety.org/vol19/iss4/art49/. doi: 10.5751/ES-07082-190449
- Griggs, D., M. Stafford-Smith, O. Gaffney, J. Rockstrom, M. C. Ohman, P. Shyamsundar, W. Steffen, G. Glaser, N. Kanie, and I. Noble. 2013. “Sustainable Development Goals for People and Planet.” Nature 495 (7441): 305–307. doi: 10.1038/495305a
- Gurk, S. M., C. Abela, and J. Debattista. n.d. Towards Ontology Quality Assessment. Online document: http://ceur-ws.org/Vol-1824/ldq_paper_2.pdf.
- Hinkel, J. 2009. “The PIAM Approach to Modular Integrated Assessment Modelling - ScienceDirect.” Environmental Modelling & Software 24 (6): 739–748. doi: 10.1016/j.envsoft.2008.11.005
- Hollmann, R., C. J. Merchant, R. Saunders, C. Downy, M. Buchwitz, A. Cazenave, E. Chuvieco, et al. 2013. “The Esa Climate Change Initiative Satellite Data Records for Essential Climate Variables.” Bulletin of the American Meteorological Society 94 (10): 1541–1552. doi: 10.1175/BAMS-D-11-00254.1
- Hong, Y., M. Zhang, and W. Q. Meeker. 2018. “Big Data and Reliability Applications: The Complexity Dimension.” Journal of Quality Technology 50 (2): 135–149. doi: 10.1080/00224065.2018.1438007
- The Importance of Standardized API Design. 2017. Swagger.io. [Online]. Accessed July 26, 2018. https://swagger.io/blog/api-design/the-importance-of-standardized-api-design/.
- Laniak, G. F., G. Olchin, J. Goodall, A. Voinov, M. Hill, P. Glynn, G. Whelan, et al. 2013. “Integrated Environmental Modeling: A Vision and Roadmap for the Future.” Environmental Modelling & Software 39: 3–23. doi: 10.1016/j.envsoft.2012.09.006
- Lehmann, A., R. Chaplin-Kramer, M. Lacayo, G. Giuliani, D. Thau, K. Koy, G. Goldberg Jr, et al. 2017. “Lifting the Information Barriers to Address Sustainability Challenges with Data from Physical Geography and Earth Observation.” Sustainability 9 (5): 858. doi: 10.3390/su9050858
- Liebowitz, J. 1999. “Key Ingredients to the Success of an Organization’s Knowledge Management Strategy.” Knowledge and Process Management 6 (1): 37–40. doi: 10.1002/(SICI)1099-1441(199903)6:1<37::AID-KPM40>3.0.CO;2-M
- Lindstrom, E., J. Gunn, A. Fischer, A. McCurdy, L. Glover, K. Alverson, B. Berx, P. Burkill, F. Chavez, and D. Checkley. 2012. A Framework for Ocean Observing. By the Task Team for an Integrated Framework for Sustained Ocean Observing, UNESCO 2012, IOC/INF-1284, doi: 10.5270/OceanObs09-FOO. Online document: http://www.oceanobs09.net/foo/FOO_Report.pdf.
- Litwin, L., and M. Rossa. 2011. Geoinformation Metadata in INSPIRE and SDI: Understanding. Editing. Publishing. Berlin, Heidelberg: Springer-Verlag.
- Lucas, R., and A. Mitchell. 2017. “Integrated Land Cover and Change Classifications.” In The Roles of Remote Sensing in Nature Conservation, 295–308. Cham: Springer International Publishing.
- Mazzetti, P., M. Santoro, and S. Nativi. 2018. “Knowledge Services Architecture”. Deliverable D1.1 of H2020 project GEOEssential. Available at: http://www.geoessential.eu/wp-content/uploads/2019/01/GEOEssential-D_1.1-v1.1-final.pdf.
- Nativi, S. 2016. “GEOSS Content Gaps and the Role of GEOSS Knowledge Base.” Presentation at the side event: 'From Data to Knowledge and GEOSS Knowledge Base' of the GEO XIII Plenary 2016, St. Petersburg (Russia). https://www.researchgate.net/publication/309857618_GEOSS_content_gaps_and_the_role_of_GEOSS_Knowledge_Base.
- Nativi, S., P. Mazzetti, P. De Salvo, S. Ramage, and GEOSS Platform Operation Team. 2017. Report on the 2nd GEO Data Providers Workshop. Online document: https://www.researchgate.net/publication/319036749_Report_on_the_2nd_GEO_Data_Providers_Workshop.
- Nativi, S., P. Mazzetti, and G. N. Geller. 2012. “Environmental Model Access and Interoperability: The GEO Model Web Initiative.” Environmental Modelling & Software 39: 214–228. doi: 10.1016/j.envsoft.2012.03.007
- Nativi, S., P. Mazzetti, and M. Santoro. 2016. Design of the ECOPOTENTIAL Virtual Laboratory. Online document: https://www.researchgate.net/publication/306240336_Design_of_the_ECOPOTENTIAL_Virtual_Laboratory.
- Nativi, S., P. Mazzetti, M. Santoro, F. Papeschi, M. Craglia, and O. Ochiai. 2015. “Big Data Challenges in Building the Global Earth Observation System of Systems.” Environmental Modelling & Software 68: 1–26. doi: 10.1016/j.envsoft.2015.01.017
- OECD and EC DG JRC. 2008. Handbook on Constructing Composite Indicators. Paris: OECD.
- Ostensen, O., D. O’Brien, and A. Cooper. 2008. “Measurements to Know and Understand Our World.” ISO Focus, February, 35–37.
- Paganini, M., I. Petiteville, S. Ward, G. Dyke, M. Steventon, J. Harry, F. Kerblat, and CEOS. 2018. Satellite Earth Observations in Support of the Sustainable Developement Goals. Rome: European Space Agency.
- Pereira, H. M., S. Ferrier, M. Walters, G. N. Geller, R. H. G. Jongman, R. J. Scholes, M. W. Bruford, et al. 2013. “Essential Biodiversity Variables.” Science 339 (6117): 277–278. doi: 10.1126/science.1229931
- Reyers, B., M. Stafford-Smith, K.-H. Erb, R. J. Scholes, and O. Selomane. 2017. “Essential Variables Help to Focus Sustainable Development Goals Monitoring.” Current Opinion in Environmental Sustainability 26–27: 97–105. doi: 10.1016/j.cosust.2017.05.003
- Richardson, L., and S. Ruby. 2007. RESTful Web Services. 1 edizione. Farnham: O’Reilly Media.
- Rockström, J., X. Bai, and B. deVries. 2018. “Global Sustainability: The Challenge Ahead.” Global Sustainability 1: E6. doi:10.1017/sus.2018.8.
- Rockstrom, J., W. Steffen, K. Noone, A. Persson, F. S. Chapin, E. F. Lambin, T. M. Lenton, et al. 2009. “A Safe Operating Space for Humanity.” Nature 461 (7263): 472–475. doi: 10.1038/461472a
- Rouse Jr, J. W., R. H. Haas, J. A. Schell, and D. W. Deering. 1974. “Monitoring Vegetation Systems in the Great Plains with Erts.” NASA Special Publication 351: 309.
- Rowley, J. 2007. “The Wisdom Hierarchy: Representations of the DIKW Hierarchy.” Journal of Information Science 33 (2): 163–180. doi: 10.1177/0165551506070706
- Santoro, M., P. Mazzetti, S. Nativi, C. Fugazza, C. Granell, and L. Díaz. 2012. “Methodologies for Augmented Discovery of Geospatial Resources.” Discovery of Geospatial Resources: Methodologies, Technologies, and Emergent Applications 172: 203.
- Santoro, M., S Nativi, J. Maso, and S. Jirka. 2017. “Observation Inventory Description and Results Report". Deliverable D4.2 of H2020 project ConnectinGEO. Online document: https://ddd.uab.cat/record/146891.
- Santoro, M., S. Nativi, and P. Mazzetti. 2016. “Contributing to the GEO Model Web Implementation: A Brokering Service for Business Processes.” Environmental Modelling & Software 84: 18–34. doi: 10.1016/j.envsoft.2016.06.010
- Scanlon, T., J. Nightingale, J.-P. Muller, F. Boersma, A. De Rudder, and J. Lambert. 2015. “QA4ECV: A Robust Quality Assurance Service for Terrestrial and Atmopsheric ECVs and ECV Precursors.” Presented at the RSPSoc, NCEO and CEOI-ST Joint Annual Conference.
- Scholes, R. J., M. Walters, E. Turak, H. Saarenmaa, C. Heip, É. Tuama, D. P. Faith, et al. 2012. “Building a Global Observing System for Biodiversity.” Current Opinion in Environmental Sustainability 4 (1): 139–146. doi: 10.1016/j.cosust.2011.12.005
- Steffen, W., K. Richardson, J. Rockström, S. E. Cornell, I. Fetzer, E. M. Bennett, R. Biggs, et al. 2015. “Planetary Boundaries: Guiding Human Development on a Changing Planet.” Science 347: 736. doi: 10.1126/science.1259855
- Tolk, A., S. Diallo, and C. Turnitsa. 2007. “Applying the Levels of Conceptual Interoperability Model in Support of Integratability, Interoperability, and Composability for System-of-systems Engineering.” Journal on Systemics, Cybernetics and Informatics 5 (5): 65–74.
- UN DESA, ed., 2013. Sustainable Development Challenges. New York: United Nations.
- United Nations. 2015. Transforming our World: The 2030 Agenda for Sustainable Development. New York: United Nations.
- UNSD (United Nations Statistics Division). 1997. Glossary of Environment Statistics. Studies in Methods. Series F, No. 67. New York 1997. Available online: https://unstats.un.org/unsd/publication/SeriesF/SeriesF_67E.pdf.
- Vaccari, L., M. Craglia, C. Fugazza, S. Nativi, and M. Santoro. 2012. “Integrative Research: The EuroGEOSS Experience.” IEEE Journal of Selected Topics in Applied Earth Observations and Remote Sensing 5 (6): 1603–1611. doi: 10.1109/JSTARS.2012.2190382
- Verburg, P. H., J. A. Dearing, J. G. Dyke, S. van der Leeuw, S. Seitzinger, W. Steffen, and J. Syvitski. 2016. “Methods and Approaches to Modelling the Anthropocene.” Global Environmental Change-Human and Policy Dimensions 39: 328–340. doi: 10.1016/j.gloenvcha.2015.08.007
- W3C. 2018. Linked Data. [Online]. Accessed November 16, 2018. https://www.w3.org/standards/semanticweb/data.
- Woods, D., and T. Mattern. 2006. Enterprise SOA: Designing IT for Business Innovation. Boston: O’Reilly Media.
- Workflow Management Coalition. 1996. "Terminology & Glossary." Document Number WFMC-TC-1011. Issue 2.0. Online document: http://www.aiai.ed.ac.uk/project/wfmc/ARCHIVE/DOCS/glossary/glossary.html.
- Zeng, Y., Z. Su, J.-C. Calvet, T. Manninen, E. Swinnen, J. Schulz, R. Roebeling, et al. 2015. “Analysis of Current Validation Practices in Europe for Space-Based Climate Data Records of Essential Climate Variables.” International Journal of Applied Earth Observation and Geoinformation 42: 150–161. doi: 10.1016/j.jag.2015.06.006
Appendix
Definition used in the scope of this manuscript.