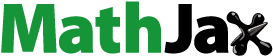
ABSTRACT
The ability to correct for the influence of forest cover is crucial for retrieval of surface geophysical parameters such as snow cover and soil properties from microwave remote sensing. Existing correction approaches to brightness temperatures for northern boreal forest regions consider forest transmissivity constant during wintertime. However, due to biophysical protection mechanisms, below freezing air temperatures freeze the water content of northern tree species only gradually. As a consequence, the permittivity of many northern tree species decreases with the decrease of air temperature under sub-zero temperature conditions. This results in a monotonic increase of the tree vegetation transmissivity, as the permittivity contrast to the surrounding air decreases. The influence of this tree temperature-transmissivity relationship on the performance of the frequency difference passive microwave snow retrieval algorithms has not been considered. Using ground-based observations and an analytical model simulation based on Mätzler’s approach (1994), the influence of the temperature-transmissivity relationship on the snow retrieval algorithms, based on the spectral difference of two microwave channels, is characterized. A simple approximation approach is then developed to successfully characterize this influence (the RMSE between the analytical model simulation and the approximation approach estimation is below 0.3 K). The approximation is applied to spaceborne observations, and demonstrates the capacity to reduce the influence of the forest temperature-transmissivity relationship on passive microwave frequency difference brightness temperature.
KEYWORDS:
1. Introduction
Spaceborne passive microwave (PM) snow retrieval methods are an important approach to estimate terrestrial seasonal snow depth (SD) and snow water equivalent (SWE) (see Kelly et al. Citation2003; Derksen Citation2008; Kelly Citation2009; Takala et al. Citation2011; Tedesco and Jeyaratnam Citation2016; Pan et al. Citation2016; Saberi et al. Citation2017). Tb differences between a lower frequency channel and a higher frequency channel (e.g. 18.70 and 36.5 GHz), abbreviated to ΔTb, of snow-covered ground is sensitive to SD and SWE, have stimulated the development of frequency difference algorithms to estimate global SWE and SD (see Kelly et al. Citation2003).
Forest transmissivity is an important parameter in the attenuation of PM observations from snow covered terrain. Previous studies have established relations between transmissivity, vegetation biomass and stem volume (see Roy et al. Citation2012; Cohen et al. Citation2015; Kruopis Citation1999; Langlois et al. Citation2011), assuming transmissivity to remain constant in frozen conditions. However, Li et al. (Citation2019) observed a temperature-transmissivity relationship under sub-zero temperature conditions in which the transmissivity increased with a decreasing temperature. This phenomenon is caused by the vegetation water content freezing and vegetation permittivity change under sub-zero conditions (Kou et al. Citation2015). The upwelling emission above the tree canopy consists mainly of the combined upwelling tree thermal emission and the ground emission which penetrates through the tree canopy. In sub-zero temperature conditions the tree transmissivity increases with the decreasing temperature as water near the surface of the tree vegetation material (woody or leaf biomass) freezes. Therefore, the tree thermal emission decreases, and the proportion of ground emission which penetrates through the tree increases. For this reason, the upwelling emission above the tree canopy is influenced more and more by the ground emission instead of the tree thermal emission. As discussed by Li et al. (Citation2020a), the temperature-transmissivity relationship influences spaceborne – or airborne-observations. In forested regions, ΔTbs decrease with increasing air temperatures that are below freezing. Typical global PM snow retrieval algorithms (see Kelly et al. Citation2003; Kelly Citation2009; Takala et al. Citation2011; Pulliainen and Grandeil Citation1999; Luojus et al. Citation2013; Xue and Forman Citation2015; Li and Kelly Citation2017; Kelly, Li, and Saberi Citation2019) ignore the influence of this temperature-transmissivity relationship on ΔTb. Therefore, ΔTb variations responding to the temperature-transmissivity relationship add uncertainty to SD and SWE retrievals.
Theoretically, a fully parameterized forest radiative transfer (RT) model can be applied to solve this issue. However, the parameters for the model (e.g. transmissivity) are difficult to obtain at the satellite PM observation scale. The influence of temperature on tree transmissivity under sub-zero temperature conditions further complicates this effort. Therefore, a feasible solution is needed to reduce the influence of the temperature-transmissivity relationship of the forest canopy on ΔTbs in snow retrievals.
In this study, we develop a semi-empirical approximation to describe how the ΔTb of the ground emission is moderated by the forest through the temperature-transmissivity relationship. As a result, the influence of the forest on the performance of the frequency difference PM snow retrieval algorithms can be mitigated.
2. Data and methods
2.1. The study site
The selected study site is close to the Finnish Meteorological Institute’s (FMI) Arctic Research Center in Sodankylä, Finland (67.36 N, 26.63 E), located in the boreal forest belt of the northern Scandinavian Peninsula. In this region, the forest consists of pine (about 75%), spruce (about 14%), and birch (about 9%). This region has a very typical landscape of the boreal forest belt with a medium density forest coverage. According to the data provided by the Finnish Forest Research Institute, the forest coverage () at this site is about 55% (Tomppo et al. Citation2011).
2.2. Data collection
2.2.1. In-situ ground radiometer observations and satellite passive microwave observations
The SodRad (Sodankylä Radiometer) radiometer system was used for the in-situ ground based downward and upward-looking observations of the ground surface and forest vegetation at the intensive observation area (IOA) and forest. This radiometer operates at 10.65, 18.7, 21 and 36.5 GHz channels in both horizontal (H) and vertical (V) polarizations (pol) (see Lemmetyinen et al. Citation2016; Lemmetyinen et al. Citation2018). A mature Scots pine tree (Pinus sylvestris L.) about 15 m tall was selected as a specimen (target tree) representing forest conditions. The upwelling microwave Tb of the ground (Tbground), the downwelling Tb of the specimen tree (↓Tbtree), and the downwelling Tb of the sky (Tbsky) at 45° elevation were measured twice per day (one during daytime and one at night) from 5 Sep 2016 to 17 Apr 2017. Full experimental details can be found in Li et al. (Citation2019) .
Figure 1. The configuration of the radiometer observation experiment (Li et al. Citation2019).
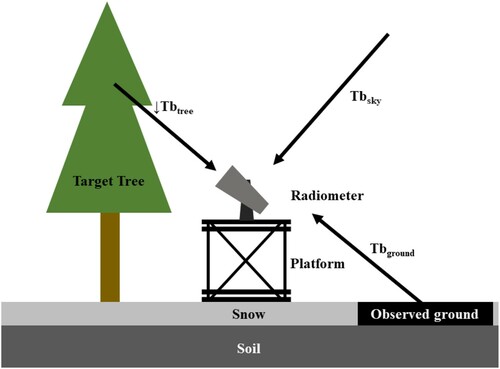
Satellite-observed Tbs (TbAMSR) at 10.65, 18.7, 21 and 36.5 GHz in both H and V pol were obtained by the Advanced Microwave Scanning Radiometer 2 (AMSR2) (Okuyama and Imaoka Citation2015) from 1 Sept 2016 to 30 Apr. 2017. The descending passes were chosen since this is when the snow was likely to be below freezing. This study used the Level 1 resampled product (L1R) (Maeda, Taniguchi, and Imaoka Citation2016) with the footprints of the TbAMSR in all the frequencies resampled to 24 × 42 km ellipse (along scan × along track).
2.2.2. Ancillary data
Two automatic weather stations (AWS) were installed at the study site, one under the forest canopy and another is in the forest opening. The air temperature, the soil temperature, and the snow depth were measured every 10 min by the AWS. These AWS data were synchronized with the ground radiometer observations and spaceborne observation data. The AWS-measured snow depth in the forest (SDforest) and forest opening (SDopen) was from a SR50 device. The forest and the forest opening sites are the two major landscape components in the AMSR2 footprint, the weighted average of SDforest and SDopen was used to represent the snow depth of the AMSR2 footprint (SDAMSR). SDAMSR was calculated as . The average value of these two AWS measured air temperatures at 2 meters above ground level were used to represent the overall air temperature of the study site (Tair). The soil temperature (Tground) was measured at −5 cm depth in the forest opening.
To compare the tree skin temperature with the air temperature, two thermometers were installed at the bark-cambium interface of the target tree’s trunk, facing north at a height of 2.2 and 4.5 m. The temperature of the bark-cambium interface was measured in the interval of 10 min, and the averaged value of the two thermometers’ measurement is used to represent the tree skin temperature (Ttree).
shows the snow depth and temperature information in the IOA. The first snowfall occurred on 28 Oct. 2016. The snow depth in the forest was about 70% of the snow depth in the forest opening. During the period that the ground was snow-free, Tair, Tground and Ttree were similar with variable fluctuations, while during the period that the ground was covered by snow, Tground was less variable, while Tair and Ttree fluctuated. The behavior of Tair and Ttree are very similar. The root mean square difference between Tair and Ttree is 2.34 K.
2.2.3. Forest emission modeling
The full details of this in-situ forest emission simulation can be found in the work of Li et al. (Citation2019). Only a brief description is included in this section. This paper used many abbreviations. For the readability, these abbreviations are listed in the appendix.
Following the approach of Mätzler (Citation1994), the upwelling emission observed above the forest cover () can be expressed by the radiative transfer (RT) equations below:
(1)
(1) where
and
are the transmissivity and the reflectivity of the forest vegetation layer respectively,
is the reflectivity of the ground,
is the temperature,
is the downwelling sky emission, and
is the upwelling ground emission. Since
is relatively small, vegetation is treated as an attenuating layer as a commonly simplified approximation (see Jackson and Schmugge Citation1991; Njoku and Entekhabi Citation1996; Pulliainen and Grandeil Citation1999; Langlois et al. Citation2011) and
is considered equal to 0. In thermal equilibrium,
can be estimated by:
(2)
(2) where
is the ground soil temperature. The model developed by Li et al. (Citation2020a) is applied to estimate tree transmissivity under sub-zero temperature conditions:
(3)
(3) where
is an empirical parameter, and
is the mean transmissivity of the forest layer measured when the air temperature is above 0°C. The parameter
and
have been determined by Li et al. (Citation2020a) are presented in for this site. As explained by Li et al. (Citation2020a), the air temperature is easier to obtain during the operational snow retrieval than the tree skin temperature, and according to , the value of Tair and Ttree are very similar. Therefore,
is the air temperature instead of tree skin temperature, and
is estimated based on the value of Tair in this study.
Table 1. Estimated parameters, R2, and the RMSE of transmissivity model.
The RT model (1) is applied to the forest emission simulation. The in-situ measured values Tbground, Tbsky, Tair, and Tground are used as ,
,
, and
in (1) and (2).
is estimated by (3), and
is estimated by (2). With these inputs, the
calculated by (1) is named as ↑TbRTforest. Details of this in-situ forest emission simulation can be found in the work of Li et al. (Citation2020a).
2.2.4 An approximation of the influence of forest emission on spaceborne ΔTbs developed based on the in-situ forest emission simulation
In forested areas, the ΔTb of the ground emission beneath the forest () is mainly used for PM SWE or SD retrievals. However, the ΔTb of the upwelling emission observed above the canopy (
) is that which is actually obtained by spaceborne or airborne instruments, as shown in . Therefore, to reduce the influence of forest cover on the frequency difference PM snow retrieval algorithms
should be estimated from
. As discussed, while forest RT models can be implemented for this purpose, to obtain the parameters required by the RT model is a challenge at the scale of satellite observations. Therefore, a simplified semi-empirical approximation is developed based on the result of in-situ forest emission simulations.
Figure 3. Schematic illustration of the influence of forest biomass on the observation of ground ΔTb.
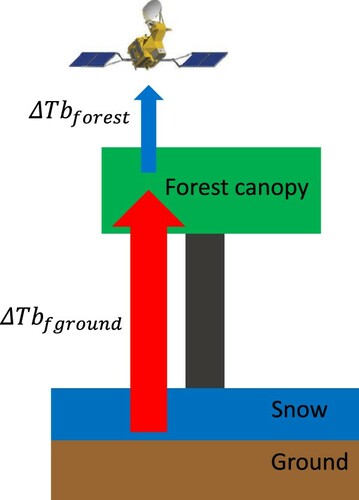
In this approach, under sub-zero temperature conditions, the influence of the temperature-transmissivity relationship on is approximated by a linear equation
, where
is the air temperature, and
and
are the empirical parameters to represent the influence of
on
through the temperature-transmissivity relationship. The approximation approach developed to describe the relationship between
,
, and
is thus presented as:
(4)
(4) where
and
are the empirical parameters of this in-situ based forest emission simulation study.
For calibrating (4), the ΔTb of ↑TbRTforest (ΔTbRTforest) is calculated by (1). By using the ΔTbRTforest, Tair, and the radiometer observed ΔTb of Tbground to represent ,
, and
in (4),
and
are estimated by the least squares fitting method. Combinations of the Tb difference of the channels 10.65 - 36.5 GHz V pol (10-37 V) and 18.7 - 36.5 GHz V pol (18-37 V) were studied. The results are presented in in the Results section.
With the estimated and
, the
approximation in (4) is now denoted as ΔTbAPPforest. In this study, based on the AWS measured Tair, ΔTbAPPforest was simulated with
values of 10, 20, 30, 40, and 50 K by (4). In , ΔTbAPPforest is compared with ΔTbRTforest.
To demonstrate the potential of this approximation in the spaceborne application, a simple test was made using AMSR2 observations to demonstrate how this approach could be applied to reduce the influence of temperature-transmissivity relationship on spaceborne observed ΔTb. The scene of a snow covered AMSR2 footprint was simplified into two main elements: forest and forest opening. Accordingly, the ΔTb of the spaceborne observation () is expressed below:
(5)
(5) where
is the ΔTb of the upwelling emission above the canopy in the forest region,
is the ΔTb of the ground emission in the forest openings, and
is the percentage of the forest fraction. As explained,
= 0.55.
For convenience, we followed the approach of Langlois et al. (Citation2011), assuming the ground conditions in the forest region and in the forest opening to be similar. The variable represents the overall ΔTb of sub-canopy ground emission from the spaceborne AMSR2 observation footprint as shown in . Accordingly,
. Equation (5) can therefore be re-written as:
(6)
(6) where
and
are the empirical parameters for the spaceborne observation. By re-arranging (6),
can be estimated through
. As explained,
is the value theoretically independent of the forest cover. Therefore, using
instead of
in the spaceborne snow retrieval procedure, we can reduce the influence of forest emission on passive microwave snow retrieval in forested terrain.
For estimating the empirical parameter and
in this study, the values of
,
and T are needed to calibrate (6). The values of
and T were obtained from AMSR2 Tb data and the AWS air temperature data respectively. For
, because the radiometer only observed the ground emission in the forest opening, this observation cannot be used directly. Therefore, a polynomial regression model between the ΔTb of the ground emission (
) and the snow depth (
) is established based on the radiometer observations Tb and the snow depth:
(7)
(7) where the
and
are the regression coefficients. Equation (7) was calibrated by the least squares fitting method based on SDopen and the ΔTb of the radiometer observed Tbground in the forest opening. Results of the calibrated Equation (7) are shown in with Equation (7) as a solid lines and radiometer observations of ΔTb of Tbground as blue–green-red circle markers. The blue–green-red color bar represents coincident air temperature. shows the fitting parameters
and
, R2 and the RMSE.
Figure 5. Radiometer-observed ΔTb of Tbground (blue-green-red circle markers) with the (7) simulated results (black solid lines), the blue-green-red bar represents Tair.
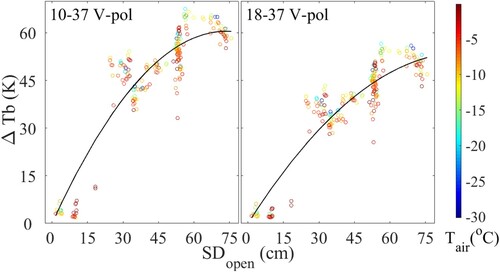
Table 2. Estimated parameters, R2, and RMSE of Equation (7).
This very localized ground ΔTb estimation approach (Equation (7)) can be applied in a very limited region, but it can be deployed by a more generally applicable modeling approach [see HUT (Pulliainen and Grandeil Citation1999), MEMLS (Wiesmann and Mätzler Citation1999), and DMRT (Tsang et al. Citation2006; Picard et al. Citation2013)] for future applications. It is also independent of snow on tree canopy, which according to the work of Li et al. (Citation2019), has negligible influence on tree emission in this study although, under different climate conditions, the tree canopy snow may have a stronger influence.
With (7) calibrated, can be estimated with SDAMSR. In (6), the ΔTb of TbAMSR (ΔTbAMSR) is used as
, and Tair is used as
, with
and
estimated by a least squares fit. The results are presented in .
Since (6) has been calibrated, the simulated by this approximation approach is named as ΔTbAPPsb. ΔTbAPPsb was simulated by Equations (6) and (7) at the
equal to 10, 25, 40, 55, and 70 cm based on the value of Tair and
. In , ΔTbAPPsb is compared with ΔTbAMSR.
In this paper, as a case study, in Sodankylä is calculated based on the ΔTbAMSR and Tair by the re-arranged Equation (6). The
calculated by this approximation approach (6) is named as ΔTbAPPsbground. To demonstrate how Equation (6) could reduce the influence of the forest on the ground ΔTbs, ΔTbAPPsbground and ΔTbAMSR are compared with the radiometer observed ΔTb of Tbground in .
For validating this approximation approach developed in Sodankylä, this approach was also applied in Enontekiö Näkkälä (Lat/Lon: 68.6/23.58, forest fraction: 24.6%) and Salla Naruska (Lat/lon: 67.16/29.18, forest fraction: 67.0%) in the Lapland region. The AMSR2 observed Tb from 1 Sep 2016 to 31 May 2017 is used. The snow depth and air temperature is obtained from the Global Surface Summary of the Day (GSOD) dataset (WMO station number: 2726 and 2745).
3. Results
In , ΔTb model and approximation values for 10-36 V and 18-36 V are shown. Colored circles denote the RT model estimates from Equation (1) that simulate ΔTbRTforest, and the colored lines show the simplified approximation approach from Equation (4) that simulate ΔTbAPPforest. ΔTbAPPforest was simulated with values of = 10, 20, 30, 40, and 50 K in (4). Because ΔTbRTforest is calculated based on the radiometer observed ground Tb in the forest opening, the blue–green-red bar represents SDopen. According to , the ΔTbRTforest and ΔTbAPPforest is influenced by both the air temperature and the snow depth. ΔTbRTforest with a deeper snow depth has a higher sensitivity to the air temperature. This is because as the snow depth increases, the ΔTb of the ground emission also tends to increase (as shows). presents the estimated parameters and the goodness of fit of Equationequation (4)
(4)
(4) during the model calibration between ΔTbRTforest, Tair, and the radiometer observed ΔTb of Tbground.
Figure 6. Comparison between ΔTbRTforest (blue-green-red circle markers) and ΔTbAPPforest which is simulated at equal to 10, 20, 30, 40, and 50 K by (4) (lines), the blue-green-red bar represents SDopen.
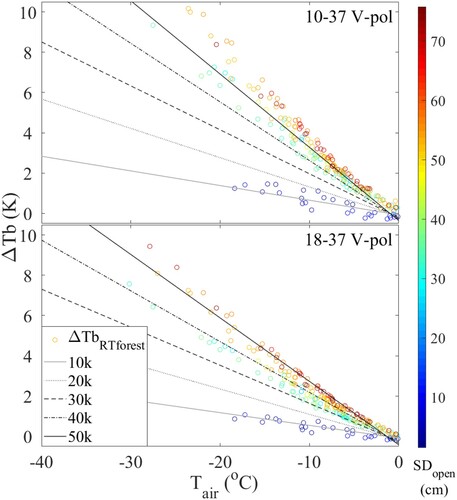
Table 3. Estimated parameters bis and cis, and corresponding R2, and RMSE of (4) against RT model (1) for the model calibration.
According to and , the approximation approach (ΔTbAPPforest) has a good agreement with the RT model simulations (ΔTbRTforest). This result indicates that the approximation [Equation (4)] developed is applicable as a simplified method to parameterize the influence of forests on ground ΔTbs.
In , the approximation approach from Equation (6) is applied to the spaceborne AMSR2 data. ΔTbAMSR (the AMSR2 observed ΔTb) is represented by the colored circles, and ΔTbAPPsb (the simulated ΔTb in AMSR2 footprint) is represented by the lines. ΔTbAPPsb was simulated by (6) and (7) for set to 10, 25, 40, 55, and 70 cm. In , the y-axis represents the ΔTb value of ΔTbAMSR and ΔTbAPPsb, the x-axis represents Tair. Because ΔTbAMSR and ΔTbAPPsb are the ΔTb in AMSR footprint, the blue–green-red bar in represents SDAMSR. shows that the ΔTbAMSR and ΔTbAPPsb are sensitive to both air temperature and the snow depth, which is comparable to the ΔTbRTforest and ΔTbAPPforest in . For non-forested areas within AMSR2 footprints, only snow depth influences the ground ΔTb. Therefore, compared with ΔTbRTforest and ΔTbAPPforest which are simulated in a full forest covered condition, ΔTbAMSR and ΔTbAPPsb have a higher sensitivity to the snow depth, and a lower sensitivity to the air temperature. shows the estimated parameters and the goodness of fit of Equation (6) during the model calibration between ΔTbAMSR, Tair, and SDAMSR.
Figure 7. Comparison between ΔTbAMSR (blue-green-red circle markers) and ΔTbAPPsb which is simulated at snow depths equal to 10, 25, 40, 55, and 70 cm (lines), the blue-green-red bar represents SDAMSR.
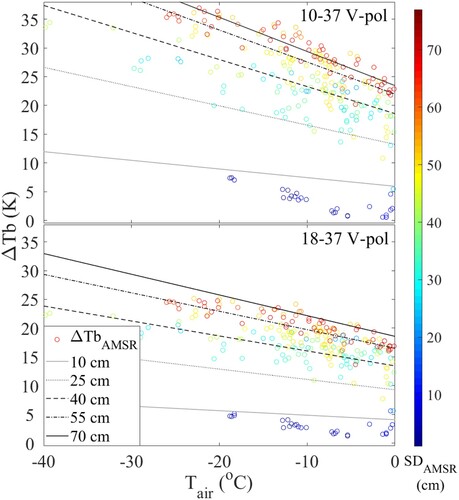
Table 4. The estimated parameters bsb and csb, and the corresponding R2, and RMSE of (6) against ΔTbAMSR during the model calibration with training dataset.
According to and , the ΔTbAPPsb has a good agreement with ΔTbAMSR, indicating that the approximation approach developed in this study can be used to simulate how the forest cover influences the ΔTb of the ground emission observed by spaceborne Tbs. Therefore, the re-arranged approximation approach (6) can be applied to reduce the influence of forest emission on spaceborne-observed ΔTb.
presents ΔTbAPPsbground calculated from the re-arranged approximation approach (6) based on ΔTbAMSR, Tair, and SDAMSR. As discussed, ΔTbAPPsbground can be considered as an estimate of the ground ΔTb without the influence of the forest. As shows, ΔTbAPPsbground is not influenced by Tair, unlike ΔTbAMSR in . In , the y-axis represents the value of ΔTbAPPsbground, the x-axis represents Tair, and the blue–green-red bar represents SDAMSR.
Figure 8. ΔTbAPPsbground (blue-green-red circle markers) against Tair, the blue-green-red bar represents SDAMSR.
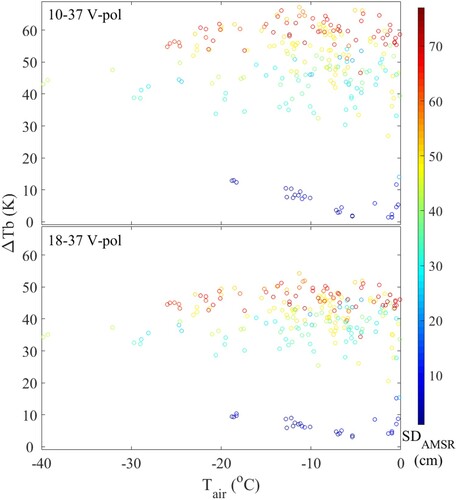
Finally, in , we show ΔTbAPPsbground (blue–green-red circles in panels (a) and (b)) and ΔTbAMSR (blue–green-red circles in panels (c) and (d)) as a function of snow depth and air temperature. The radiometer observed ΔTb of Tbground are shown as black crosses. For the radiometer observed ΔTb of Tbground, because the radiometer ground observation made in the forest opening, the Tbs only be influenced by SDopen. Therefore, the x-axis represents SDopen for this data set in . While for ΔTbAPPsbground and ΔTbAMSR, they are the simulation and observation in AMSR2 footprint. The Tbs are influence by the snow depth in the whole AMSR observation footprint instead of the snow depth in forest open. Therefore, x-axis represents SDAMSR for these data set.
Figure 9. Compared ΔTbAPPsbground (blue-green-red circle markers in (a) and (b)) and ΔTbAMSR (blue-green-red circle markers in (c) and (d)) with the radiometer observed ΔTb of Tbground (black cross markers), the blue-green-red bar represents Tair.
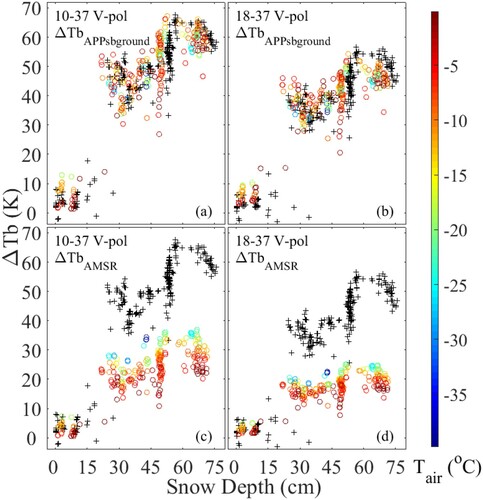
Comparing ΔTbAPPsbground with ΔTbAMSR, ΔTbAPPsbground is not influenced by the air temperature, and has a greater sensitivity to the snow depth. Comparing ΔTbAPPsbground and ΔTbAMSR with the radiometer-observed ΔTb of Tbground, ΔTbAPPsbground has a higher similarity with the ΔTb of Tbground. According to , the ΔTb of Tbground is not sensitive to air temperature. As discussed, the temperature-transmissivity relationship causes the ΔTbAMSR to be sensitive to air temperature under sub-zero temperatures. The results of this comparison indicate that the influence the temperature-transmissivity relationship of the forest on the AMSR2 observed ΔTb is reduced in ΔTbAPPsbground by the approximation approach developed in this paper.
4. Validation
The RMSE between the AMSR2 observed ΔTb and the Equation (6) simulated ΔTb in Enontekiö Näkkälä and Salla Naruska are 8.82 and 4.57 K respectively, which indicates that the approximation approach has a good performance at these two locations. In , the simulated ΔTb in AMSR2 footprint (ΔTbAPPsb) is compared with the AMSR2 observed data (ΔTbAMSR). The ΔTbAPPsb is simulated at the snow depth of 10, 25, 40, 55, and 70 cm by the Equation (6) with the parameters estimated in Sodankylä (). The ΔTbAMSR is represented by blue–green-red circle markers. The blue–green-red bar represents the snow depth in GSOD dataset.
Figure 10. Comparison between ΔTbAMSR (blue-green-red circle markers) and ΔTbAPPsb which is simulated at snow depths equal to 10, 25, 40, 55, and 70 cm (lines), the blue-green-red bar represents SDAMSR.
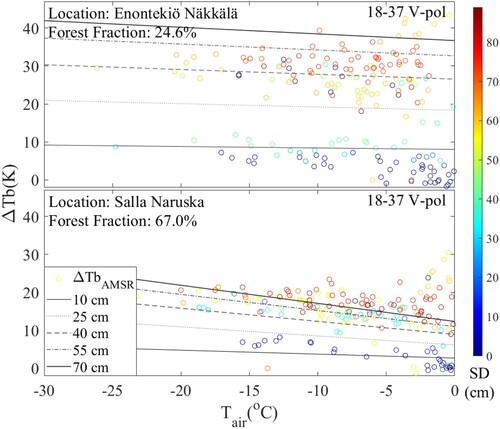
Salla Naruska has a higher forest fraction than Enontekiö Näkkälä. Therefore, the temperature-transmissivity relationship should have a higher influence on the ΔTb in Salla Naruska. According to , the ΔTb in Salla Naruska has a higher sensitivity to the temperature, which indicated that the forest has a higher influence on ΔTb in Salla Naruska. Both the observed and the simulated results have shown this tendency.
In , ΔTbAMSR in (c) and (d) are the AMSR2 observed ΔTb in Enontekiö Näkkälä and Salla Naruska. While, ΔTbAPPsbground in (a) and (b) are the ΔTbAMSR corrected by the re-arranged approximation approach (6). Both ΔTbAMSR and ΔTbAPPsbground are compared with the SodRod radiometer in-situ observed brightness temperature in Sodankylä (ΔTbground). The blue–green-red bar represents the air temperature in GSOD dataset.
Figure 11. Compared ΔTbAPPsbground (blue-green-red circle markers in (a) and (b)) and ΔTbAMSR (blue-green-red circle markers in (c) and (d)) with the radiometer observed ΔTb of Tbground (black cross markers), the blue-green-red bar represents Tair.
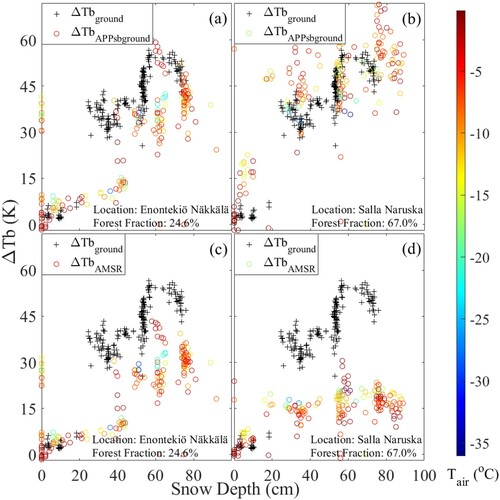
The results in is very similar as the result in . Comparing ΔTbAPPsbground with ΔTbAMSR, ΔTbAPPsbground has a higher similarity with the in-situ radiometer observed ΔTb, and has a greater sensitivity to the snow depth. The results indicate that the influence of the temperature-transmissivity relationship on the AMSR2 observed ΔTb in Enontekiö Näkkälä and Salla Naruska is reduced by the approximation approach.
5. Discussion
The temperature-transmissivity relationship, first reported by Li et al. (Citation2019), indicated that the sensitivity of ΔTb to the ground SD and SWE increases in a forest environment as air temperatures decrease below sub-zero temperature conditions (Li et al. Citation2020a). When observed by aircraft or satellite radiometers the ΔTb above the forest canopy is influenced by both air temperature and the ΔTb of the ground emission underneath the forest under sub-zero temperature conditions (see and ). The strong influence of the air temperature on the ΔTb explains why a constant adjustment coefficient approach (see Chang, Foster, and Hall Citation1996; Foster et al. Citation2005) is insufficient to correct the influence of forest attenuation on PM snow retrievals. This may also have contributed to differing parameterizations of forest microwave transmissivity obtained in previous studies (Cohen et al. Citation2015; Kruopis Citation1999; Langlois et al. Citation2011).
The soil condition is another factor could influence the ΔTb of the ground. The soil frozen causes the soil dielectric constant decrease, thereby the ΔTb of the ground decreases (Zhao et al. Citation2011). However, in this study, the temperature and the dielectric constant of the soil are relatively stable after the ground is covered by snow, the AWS measured results are presented in Li (Citation2020b). Since this study focused on the snow covered ground, the soil condition has a limited influence on the ground ΔTb during winter time in this study.
According to and , ΔTbAPPsbground is not influenced by air temperature, unlike ΔTbAMSR. Furthermore, ΔTbAPPsbground has a stronger sensitivity to the snow depth than ΔTbAMSR. Compared with ΔTbAMSR, the behavior of ΔTbAPPsbground with respect to the air temperature and snow depth has a higher similarity with the behavior of the ground-based radiometer observed ΔTb of Tbground. This result indicates that the approximation approach (Equation (6)) developed in this paper can effectively reduce the influence from both the forest attenuation effect (Foster et al. Citation2005) and the temperature-transmissivity relationship (Li et al. Citation2019; Li et al. Citation2020a) on the spaceborne or airborne observed ΔTb during snow retrievals.
Comparing with , and despite the different data sources in calibrating (4) and (6), the coefficients bis and cis are remarkably similar in magnitude to bsb and csb, given the many orders of magnitude difference in the spatial scales to which these apply. The differences between the in-situ and spaceborne b and c coefficients may be due to the different observation scale (the local single tree observation vs. the satellite scale observation), the biomass density difference between a single tree and a forest, and other land uses that are within the AMSR2 footprint that are not accounted for in this simple analysis. In addition, differences in the viewing geometries of the radiometers and the actual snow depth in the AMSR2 footprint from SDAMSR also likely contribute to the differences in the coefficients. The negative values of the c coefficients imply a change of sign of the brightness difference from below the canopy to above the canopy as the air temperature approaches freezing, which is not expected from the physics of the problem, nor from the measurements we have. However, the differential canopy transmissivity is negative only for a few degrees Celsius, at most, below freezing. If we consider these to be fitting parameters for a more complex differential canopy transmissivity for which (4) and (6) are the first order Taylor series, we can see that this may simply be a result of fitting errors for this particular forest due to the approximations inherent in the form of (4) and (6).
In addition to the vegetation permittivity, the structure of the forest medium (e.g. density, stem volume, and height) also has a strong influence on the ΔTb. The pattern of the forest structure variation relates to the spatial scale of the observation (Smith and Urban Citation1988; Larue et al. Citation2017; Löfman and Kouki Citation2003; Shugart, Saatchi, and Hall Citation2010). At a small spatial scale of observation (at the scales which forest gaps can be observed), the period of the forest succession, disturbance and recovery can be observed. Therefore, the structure of a mature forest ecosystem is a heterogeneous mixture of patches at this scale. However, at a larger observation scale, like the AMSR2 observation, only the aggregated pattern can be observed, the heterogeneous nature of the forest spatial variability at the local scale has a limited influence on large scale observations. The structure of the forest stand is largely controlled by the climatic factors such as temperature and precipitation (Smith and Urban Citation1988; López-Serrano et al. Citation2005, Wang et al. Citation2006, Shugart, Saatchi, and Hall Citation2010; Pan et al. Citation2013) at this observation scale. Therefore, the structure of the forest stands is relatively consistent within the same biome (Holdridge Citation1967; Sitch et al. Citation2008; Wang et al. Citation2006).
In Equation (6), although the forest fraction has been considered, the variation of the forest structure has not been discussed yet. The forest structure should have a strong influence on the equation parameter and
. In this paper, the study sites are located inside the same biome. Therefore, the parameters
and
should be relatively consistent within the same biome. The validation in Enontekiö Näkkälä and Salla Naruska also support this argument. However, if this approach is needed to be applied over a different biome with a different forest structure, the influence of the forest structure on parameters
and
should be further evaluated. Maybe a lookup table could be developed to determine the parameters for different types of forest.
6. Conclusion
The permittivity variation caused by the cold temperature has a significant influence on the tree microwave radiative transfer (Li et al. Citation2019). Therefore, this phenomenon is an important factor in passive microwave passive microwave snow retrieval (Li et al. Citation2020a). However, this phenomenon has not been considered by current snow retrieval algorithms. A feasible correction approach is required.
For correcting the influence of this phenomenon on snow retrieval, simplified approximation was developed in this study to describe how the temperature-transmissivity relationship influences ΔTb in a forested region. This approximation approach has been evaluated over the study sites in Sodankylä, Enontekiö Näkkälä, and Salla Naruska. The results of the evaluation have demonstrated that this approximation approach has a strong potential to reduce the influence of the forest on the performance of the PM frequency difference snow retrieval algorithms. Therefore, it is recommended that this approximation should be evaluated more widely and adopted for and global scale PM snow retrievals in the future.
Acknowledgments
The ground measured radiometer data and ancillary data are provided by the FMI Arctic Research Centre, the GCOM-W1 AMSR2 level 1R Tb product is provided by JAXA, and MODIS MOD44b Version 6 Vegetation Continuous Field product data is provided by NASA EOSDIS Land Processes DAAC.
Disclosure statement
No potential conflict of interest was reported by the author(s).
Additional information
Funding
References
- Chang, A. T. C., J. L. Foster, and D. K. Hall. 1996. “Effects of Forest on the Snow Parameters Derived from Microwave Measurements During the BOREAS Winter Field Campaign.” Hydrological Processes 10 (12): 1565–1574.
- Cohen, Juval, Juha Lemmetyinen, Jouni Pulliainen, Kirsikka Heinila, Francesco Montomoli, Jaakko Seppanen, and Martti T. Hallikainen. 2015. “The Effect of Boreal Forest Canopy in Satellite Snow Mapping-A Multisensor Analysis.” IEEE Transactions on Geoscience and Remote Sensing 53 (12): 6593–6607.
- Derksen, Chris. 2008. “The Contribution of AMSR-E 18.7 and 10.7 GHz Measurements to Improved Boreal Forest Snow Water Equivalent Retrievals.” Remote Sensing of Environment 112 (5): 2701–2710.
- Foster, James L., Chaojiao Sun, Jeffrey P. Walker, Richard Kelly, Alfred Chang, Jiarui Dong, and Hugh Powell. 2005. “Quantifying the Uncertainty in Passive Microwave Snow Water Equivalent Observations.” Remote Sensing of Environment 94 (2): 187–203.
- Holdridge, L. R. 1967. Life Zone Ecology. San José. Costa Rica: Tropical Science Center.
- Jackson, T. J., and T. J. Schmugge. 1991. “Vegetation Effects on the Microwave Emission of Soils.” Remote Sensing of Environment 36 (3): 203–212.
- Kelly, Richard E. 2009. “The AMSR-E Snow Depth Algorithm: Description and Initial Results.” Journal of the Remote Sensing Society of Japan 29 (1): 307–317.
- Kelly, Richard E., Alfred T. Chang, Leung Tsang, and James L. Foster. 2003. “A Prototype AMSR-E Global Snow Area and Snow Depth Algorithm.” IEEE Transactions on Geoscience and Remote Sensing 41 (2): 230–242.
- Kelly, Richard E., Qinghuan Li, and Nastaran Saberi. 2019. “The AMSR2 Satellite-Based Microwave Snow Algorithm (SMSA): A New Algorithm for Estimating Global Snow Accumulation.” In International Geoscience and Remote Sensing Symposium (IGARSS). https://doi.org/https://doi.org/10.1109/IGARSS.2019.8898525.
- Kou, Xiaokang, Linna Chai, Lingmei Jiang, Shaojie Zhao, and Shuang Yan. 2015. “Modeling of the Permittivity of Holly Leaves in Frozen Environments.” IEEE Transactions on Geoscience and Remote Sensing. doi:https://doi.org/10.1109/TGRS.2015.2431495.
- Kruopis, Nerijus. 1999. “Passive Microwave Measurements of Snow-Covered Forest Areas in Em Ac’95.” IEEE Transactions on Geoscience and Remote Sensing. doi:https://doi.org/10.1109/36.803417.
- Langlois, Alexandre, Alain Royer, Florent Dupont, Alexandre Roy, Kalifa Goita, and G. Picard. 2011. “Improved Corrections of Forest Effects on Passive Microwave Satellite Remote Sensing of Snow Over Boreal and Subarctic Regions.” IEEE Transactions on Geoscience and Remote Sensing. doi:https://doi.org/10.1109/TGRS.2011.2138145.
- Larue, Fanny, Alain Royer, Danielle De Sève, Alexandre Langlois, Alexandre Roy, and Ludovic Brucker. 2017. “Validation of GlobSnow-2 Snow Water Equivalent Over Eastern Canada.” Remote Sensing of Environment. doi:https://doi.org/10.1016/j.rse.2017.03.027.
- Lemmetyinen, J., A. Kontu, L. Leppänen, J. Vehviläinen, R. Vehmas, Qinghuan Li, K. Rautiainen, and J. Pulliainen. 2018.. “Season -Length Observations of Active and Passive Microwave Signatures of Snow Cover in a Boreal Forest Environment.” In International Geoscience and Remote Sensing Symposium (IGARSS). Vol. 2018-July. https://doi.org/https://doi.org/10.1109/IGARSS.2018.8517328.
- Lemmetyinen, Juha, Anna Kontu, Jouni Pulliainen, Juho Vehviläinen, Kimmo Rautiainen, Andreas Wiesmann, Christian Mätzler, et al. 2016. “Nordic Snow Radar Experiment.” Geoscientific Instrumentation, Methods and Data Systems. doi:https://doi.org/10.5194/gi-5-403-2016.
- Li, Qinghuan. 2020b. “The Influence of Winter Time Boreal Forest Tree Transmissivity on Tree Emission and Passive Microwave Snow Observations.” Doctoral Dissertation, Waterloo, ON: University of Waterloo.
- Li, Qinghuan, and R. E. J. Kelly. 2017. “Correcting Satellite Passive Microwave Brightness Temperatures in Forested Landscapes Using Satellite Visible Reflectance Estimates of Forest Transmissivity.” IEEE Journal of Selected Topics in Applied Earth Observations and Remote Sensing 10 (9). doi:https://doi.org/10.1109/JSTARS.2017.2707545.
- Li, Qinghuan, R. E. J. Kelly, J. Lemmetyinen, and J. Pan. 2020a. “Simulating the Influence of Temperature on Microwave Transmissivity of Trees During Winter Observed by Spaceborne Microwave Radiometery.” IEEE Journal of Selected Topics in Applied Earth Observations and Remote Sensing. doi:https://doi.org/10.1109/JSTARS.2020.3017618.
- Li, Qinghuan, R. E. J. Kelly, L. Leppanen, J. Vehvilainen, A. Kontu, J. Lemmetyinen, and J. Pulliainen. 2019. “The Influence of Thermal Properties and Canopy-Intercepted Snow on Passive Microwave Transmissivity of a Scots Pine.” IEEE Transactions on Geoscience and Remote Sensing 57 (8). doi:https://doi.org/10.1109/TGRS.2019.2899345.
- Löfman, Satu, and Jari Kouki. 2003. “Scale and Dynamics of a Transforming Forest Landscape.” Forest Ecology and Management. doi:https://doi.org/10.1016/S0378-1127(02)00133-0.
- López-Serrano, F. R., A. García-Morote, M. Andrés-Abellán, A. Tendero, and A. Del Cerro. 2005. “Site and Weather Effects in Allometries: A Simple Approach to Climate Change Effect on Pines.” Forest Ecology and Management. doi:https://doi.org/10.1016/j.foreco.2005.05.014.
- Luojus, K., J. Pulliainen, M. Takala, et al. 2013. GlobSnow-2 Product User Guide Version 1.0. Paris: European space agency.
- Maeda, Takashi, Yuji Taniguchi, and Keiji Imaoka. 2016. “GCOM-W1 AMSR2 Level 1R Product: Dataset of Brightness Temperature Modified Using the Antenna Pattern Matching Technique.” IEEE Transactions on Geoscience and Remote Sensing. doi:https://doi.org/10.1109/TGRS.2015.2465170.
- Mätzler, C. 1994. “Microwave Transmissivity of a Forest Canopy: Experiments made with a Beech.” Remote Sensing of Environment 48 (2): 172–180.
- Njoku, Eni G., and Dara Entekhabi. 1996. “Passive Microwave Remote Sensing of Soil Moisture.” Journal of Hydrology. doi:https://doi.org/10.1016/0022-1694(95)02970-2.
- Okuyama, Arata, and Keiji Imaoka. 2015. “Intercalibration of Advanced Microwave Scanning Radiometer-2 (AMSR2) Brightness Temperature.” IEEE Transactions on Geoscience and Remote Sensing. doi:https://doi.org/10.1109/TGRS.2015.2402204.
- Pan, Yude, Richard A. Birdsey, Oliver L. Phillips, and Robert B. Jackson. 2013. “The Structure, Distribution, and Biomass of the World’s Forests.” Annual Review of Ecology, Evolution, and Systematics. doi:https://doi.org/10.1146/annurev-ecolsys-110512-135914.
- Pan, Jinmei, Michael Durand, Melody Sandells, Juha Lemmetyinen, Edward J. Kim, Jouni Pulliainen, Anna Kontu, and Chris Derksen. 2016. “Differences Between the HUT Snow Emission Model and MEMLS and Their Effects on Brightness Temperature Simulation.” IEEE Transactions on Geoscience and Remote Sensing. doi:https://doi.org/10.1109/TGRS.2015.2493505.
- Picard, G., L. Brucker, A. Roy, F. Dupont, M. Fily, A. Royer, and C. Harlow. 2013. “Simulation of the Microwave Emission of Multi-Layered Snowpacks Using the Dense Media Radiative Transfer Theory: The DMRT-ML Model.” Geoscientific Model Development. doi:https://doi.org/10.5194/gmd-6-1061-2013.
- Pulliainen, Jouni T., and Jochen Grandeil. 1999. “HUT Snow Emission Model and Its Applicability to Snow Water Equivalent Retrieval.” IEEE Transactions on Geoscience and Remote Sensing. doi:https://doi.org/10.1109/36.763302.
- Roy, Alexandre, Alain Royer, Jean Pierre Wigneron, Alexandre Langlois, Jean Bergeron, and Patrick Cliche. 2012. “A Simple Parameterization for a Boreal Forest Radiative Transfer Model at Microwave Frequencies.” Remote Sensing of Environment. doi:https://doi.org/10.1016/j.rse.2012.05.020.
- Saberi, Nastaran, Richard Kelly, Peter Toose, Alexandre Roy, and Chris Derksen. 2017. “Modeling the Observed Microwave Emission from Shallow Multi-Layer Tundra Snow Using DMRT-ML.” Remote Sensing. doi:https://doi.org/10.3390/rs9121327.
- Shugart, H. H., S. Saatchi, and F. G. Hall. 2010. “Importance of Structure and Its Measurement in Quantifying Function of Forest Ecosystems.” Journal of Geophysical Research: Biogeosciences. doi:https://doi.org/10.1029/2009JG000993.
- Sitch, Stephan, C. Huntingford, N. Gedney, P. E. Levy, M. Lomas, S. L. Piao, R. Betts, et al. 2008. “Evaluation of the Terrestrial Carbon Cycle, Future Plant Geography and Climate-Carbon Cycle Feedbacks Using Five Dynamic Global Vegetation Models (DGVMs).” Global Change Biology. doi:https://doi.org/10.1111/j.1365-2486.2008.01626.x.
- Smith, Thomas M., and Dean L. Urban. 1988. “Scale and Resolution of Forest Structural Pattern.” Vegetatio. doi:https://doi.org/10.1007/BF00044739.
- Takala, Matias, Kari Luojus, Jouni Pulliainen, Chris Derksen, Juha Lemmetyinen, Juha Petri Kärnä, Jarkko Koskinen, and Bojan Bojkov. 2011. “Estimating Northern Hemisphere Snow Water Equivalent for Climate Research Through Assimilation of Space-Borne Radiometer Data and Ground-Based Measurements.” Remote Sensing of Environment. doi:https://doi.org/10.1016/j.rse.2011.08.014.
- Tedesco, Marco, and Jeyavinoth Jeyaratnam. 2016. “A New Operational Snow Retrieval Algorithm Applied to Historical AMSR-E Brightness Temperatures.” Remote Sensing. doi:https://doi.org/10.3390/rs8121037.
- Tomppo, E., M. Katila, K. Mäkisara, and J. Peräsaari. 2011. The Multi-Source National Forest Inventory of Finland — Methods and Results 2011. Vantaa: Finnish Forest Res. Inst.
- Tsang, L., Jin Pan, Ding Liang, Zhongxin Li, and Don Cline. 2006. “Modeling Active Microwave Remote Sensing of Snow Using Dense Media Radiative Transfer (DMRT) Theory with Multiple Scattering Effects.” In International Geoscience and Remote Sensing Symposium (IGARSS). https://doi.org/https://doi.org/10.1109/IGARSS.2006.127.
- Wang, Xiangping, Jingyun Fang, Zhiyao Tang, and Biao Zhu. 2006. “Climatic Control of Primary Forest Structure and DBH–Height Allometry in Northeast China.” Forest Ecology and Management 234 (1-3): 264–274.
- Wiesmann, Andreas, and Christian Mätzler. 1999. “Microwave Emission Model of Layered Snowpacks.” Remote Sensing of Environment. doi:https://doi.org/10.1016/S0034-4257(99)00046-2.
- Xue, Yuan, and Barton A. Forman. 2015. “Comparison of Passive Microwave Brightness Temperature Prediction Sensitivities Over Snow-Covered Land in North America Using Machine Learning Algorithms and the Advanced Microwave Scanning Radiometer.” Remote Sensing of Environment. doi:https://doi.org/10.1016/j.rse.2015.09.009.
- Zhao, Tianjie, Lixin Zhang, Lingmei Jiang, Shaojie Zhao, Linna Chai, and Rui Jin. 2011. “A new Soil Freeze/Thaw Discriminant Algorithm Using AMSR-E Passive Microwave Imagery.” Hydrological Processes 25 (11): 1704–1716.
Appendix
The abbreviations used in this paper.
Table