ABSTRACT
Data Trusts are an important emerging approach to enabling the much wider sharing of data from many different sources and for many different purposes, backed by the confidence of clear and unambiguous data governance. Data Trusts combine the technical infrastructure for sharing data with the governance framework of a legal trust. The concept of a data Trust applied specifically to spatial data offers significant opportunities for new and future applications, addressing some longstanding barriers to data sharing, such as location privacy and data sovereignty. This paper introduces and explores the concept of a ‘spatial data Trust’ by identifying and explaining the key functions and characteristics required to underpin a data Trust for spatial data. The work identifies five key features of spatial data Trusts that demand specific attention and connects these features to a history of relevant work in the field, including spatial data infrastructures (SDIs), location privacy, and spatial data quality. The conclusions identify several key strands of research for the future development of this rapidly emerging framework for spatial data sharing.
1. Introduction
Spatial data is one of the main drivers of technological and economic development across many sectors of government, industry, and society. Spatial data is central to ongoing advancements in critical domains including health, finance, transportation, navigation, environmental management, and many others. The pervasive nature of spatial referencing in data is already widely acknowledged (Hahmann and Burghardt Citation2013). A survey conducted by CartoFootnote1 showed that the majority (over 90%) of large business companies and organizations capture and store location data (Carto Citation2018). Spatial data holds immense value for a range of stakeholders including government institutions, private companies, public groups, First Nations, non-profit organizations, and individuals (Danish Enterprise and Construction Authority Citation2010; Cabinet Office Citation2018; Australian Government, The Department of Industry, Innovation and Resources Citation2019; Australian Government, The Department of Prime Minister and Cabinet Citation2022).
Nevertheless, using and sharing spatial data comes with challenges. For example, one longstanding challenge is associated with tracking the quality of spatial data and providing confidence in its fitness for use (Goodchild Citation2007; Ahonen-Rainio and Kraak Citation2005; Basiri et al. Citation2019; Senaratne et al. Citation2017; Woodcock Citation2002). In addition to the technical and conceptual challenges posed by spatial data quality, the assessment of fitness for use can be highly context-dependent. For example, management and maintenance of physical road transport infrastructure typically require spatial data with much higher precision and positional accuracy than is required for, say, routing or navigation. Such issues present challenges even within an organization and are only magnified when attempting to share spatial data.
Another key barrier to sharing spatial data can be location privacy. Location privacy concerns a person’s right to control the information about their location as personal and sensitive data (Beresford and Stajano Citation2003; Duckham and Kulik Citation2006; European Parliament and Council of the European Union Citation2016; Scassa Citation2010). According to European Union’s General Data Protection Regulation (GDPR), location data is included in the personal data category as information relating to an identifiable individual or data subject (European Parliament and Council of the European Union Citation2016). Hence, a lack of assurances that personal location data will be safely and securely managed, adequately anonymized, and protected from reidentification can present impediments to sharing, especially when considering how or whether to share data sets containing potentially sensitive data about individuals’ locations.
Considerations such as quality and privacy, as well as issues such as transparency, equity, interoperability, can present impediments to sharing of spatial data (Durrant et al. Citation2021; Stalla-Bourdillon et al. Citation2020). According to Waterman et al. (Citation2021), some organizations simply avoid releasing and sharing data because of the risks associated with, and time required to anonymize personal data. In addition, many government and private organizations restrict data sharing or release data only under a specific data licensing agreement (Janssen et al. Citation2020; Harvey and Tulloch Citation2006). Hence, in the absence of ready mechanisms for supporting safe and effective sharing, data custodians are faced with a stark choice: to share and risk the possibility of inappropriate handling of data or simply to restrict access to potentially useful and otherwise valuable data. The former approach carries increased reputational and legal risks, for example, related to quality issues and privacy breaches. The latter approach runs counter to the spirit of decades of open data initiatives and spatial data infrastructure (SDI) principles.
To illustrate, consider the example scenario depicted in . In , a private courier company routinely generates potentially valuable data about employee locations and movements in the course of their deliveries. This valuable data may already be held on behalf of the company by a remote cloud data storage service. A location-based service (LBS) provider, in turn, may have developed new routing tools or business intelligence analytics that can use the insights from such movement data to add value to their own customers. However, the potential for mutually beneficial data sharing—where the LBS provider is able to increase value to their end users through higher quality data offerings; the courier company is able to maximize the untapped value of its business data; and the courier employees can be assured their location privacy is protected—will typically be foregone because of the effort, costs, and risks involved in overcoming the significant barriers to sharing, such as those discussed above.
Many of these issues have technological aspects to their solutions. For example, there are numerous different mechanisms proposed in the literature for protecting a person’s location privacy (such as obfuscation, geomasking, differential privacy, etc. Krumm Citation2007; Duckham and Kulik Citation2006; Solanas and Martínez-Ballesté Citation2008; Seidl, Jankowski, and Clarke Citation2018). However, in all cases, technology alone cannot overcome all the barriers to sharing. Assurances about usage, quality, licensing, privacy, control, sovereignty, and many other non-technical issues are necessary prerequisites to sharing. Hence, effective mechanisms for data governance also play a crucial role in managing, handling, and protecting spatial data to promote sharing. Such governance becomes even more critical in an era of artificial intelligence and machine learning, where big data algorithms may inadvertently present new risks by reinforcing biases, lacking transparency, and issues with accountability (Janssen et al. Citation2020).
The core motivation for this work is connected with the importance of spatial data governance in supporting the increased sharing of spatial data. More specifically, conventional spatial data governance models can limit the potential for using and sharing the rising tide of big spatial data from diverse sources. Conventional data governance approaches often fail to unlock unrealized value in spatial data sets, for example, as a result of challenges associated with data quality, data privacy, and data sovereignty. While there is significant literature on the governance of spatial data (e.g. the literature on spatial data infrastructures, surveyed in Section 2.5), this paper focuses primarily on spatial data Trusts as an emerging approach to data sharing of critical importance in the spatial domain. Data TrustsFootnote2 are important in enabling the much wider sharing of data from many different sources and for many different purposes, backed by the confidence of clear and unambiguous data governance. Data Trusts combine the technical infrastructure for sharing data with the governance framework of a legal trust. Legal trusts are often used to protect and maintain assets, such as land trusts. The concept of a data Trust applied specifically to spatial data offers significant opportunities for new and future applications.
Data Trusts are a relatively new type of data governance model. This approach is already in use across health, finance, and civic industry sectors. However, the data Trust governance approach is still relatively unexplored for managing, protecting, and sharing spatial data. Hence, our main aim in this work is to identify and explore the foundations of spatial data Trusts, in the context of the data Trusts already emerging in other domains. Our work proposes a set of five distinctive characteristics of spatial data Trusts necessary for increasing access, protection, sharing, and value of spatial data. The set of five special characteristics is intended to assist in the development of new spatial data governance approaches that enable sustainable and effective access and sharing of spatial data. Further, the work aims to lay the foundations for maximizing the value of spatial data, whilst mitigating the risks of spatial data sharing, such as breaches of privacy, data misuse, and financial losses. In exploring these underpinning properties of spatial data Trusts, this paper aims to tackle the following fundamental question: What special functions and unique characteristics are required for a spatial data Trust to meet current challenges in spatial data governance?
The paper is organized as follows. In Section 2, the paper introduces and explores the essential features of data Trusts in the context of wider spatial data governance initiatives. Section 3 grounds this introduction to data Trusts by presenting an extensive exploration and classification of current and emerging examples of data Trust initiatives across different domains. Building on this context, Section 4 the explores the key characteristics of spatial data Trusts, leading to the definition of a model of spatial data Trusts with five key elements: domain specificity, data quality, data privacy, data sovereignty, and data value. Section 5 critically reviews this model, and sets out the key avenues for future work.
2. Data trusts and data governance
The Open Data Institute (ODI) defines data Trusts as a data governance model with a legal structure that enables independent stewardship of data (Open Data Institute Citation2018b). A data Trust has also been defined as a bottom-up data governance framework that enables individuals and organizations to provide, share, and protect data backed by legal or quasi-legal assurances, and facilitated by the board of trustees (Delacroix and Lawrence Citation2019). Data Trusts must therefore provide clear, appropriate, fair, and transparent guidelines for decision-making about data and managing and protecting the rights associated with data. In short, data Trusts are about providing assured and independent stewardship of data with the aim of respecting and satisfying multiple (usually competing) interests.
2.1. Structure of a data trust
The structure of any data Trust involves three main parties: (i) data trustees, (ii) data ‘trustors’ or ‘settlors,’ and (iii) data beneficiaries (Delacroix and Lawrence Citation2019; Manohar, Ramesh, and Kapoor Citation2020). Data trustees are independent individuals or organizations that coordinate and manage the Trust and its data as a reliable and trusted intermediary. Data trustors (or settlors) are individuals or organizations who provide their data to a data Trust on the understanding that their rights and obligations will be honored by the data Trust on their behalf. Data beneficiaries are individuals or organizations who are able to gain access to data through the data Trust. A simple schematic view of a data Trust illustrating these three parties is shown in .
The role of the data trustees is clearly crucial to a successful and effective data Trust. The main roles of trustees are to enable the collection, communication, and sharing of data with data beneficiaries. Sharing through the data Trust aims ultimately to increase the value of data for all parties, supporting applications that serve a greater public interest.
When compared to other emerging data governance models (including data commons, data cooperatives, data collaborative, data market places, account aggregators, and personal stores Micheli et al. Citation2020; Manohar, Ramesh, and Kapoor Citation2020; Lea et al. Citation2016; Bruhn Citation2014), what makes data Trust distinct is its defined legal framework. An independent board of trustees, including legal professionals, may oversee the operations and services of a data Trust (Place Citation2022b). This model of data governance is founded on the traditional concept of Trust. A data Trust treats data as property—managed, mediated, and shared by a board of trustees in order to satisfy the interests of key stakeholders, such as data trustors. This approach applies existing legal policies and frameworks to hold the data Trust accountable, enforcing the Trust’s fiduciary obligations to data trustors. The legal framework for data Trusts may be tailored to different needs and data characteristics, including the data type, organizational purpose, profile of data trustors, and the particular level of data protection and shareability required by a trustee.
2.2. Functions of a data trust
In this section, we summarize some of the most important functions of a data Trust across different sectors. In particular, it is possible to summarize the operations of a data Trust as providing four key functions.
2.2.1. Data sharing
Ultimately, the most important function of any data Trust is to enable sharing of data. Underpinning this function, a data Trust will develop and deploy a set of policy and legal agreements to support data sharing between trustors and data beneficiaries (Rinik Citation2020; Delacroix and Lawrence Citation2019; Austin Citation2019; Stalla-Bourdillon et al. Citation2020). These agreements may include a defined data standards and specifications; lists of accepted data formats; guidelines about providing and requesting data; and other types of policies associated with data sharing. This function must also support the removal of data and/or sharing restrictions from the data Trust. The agreements and regulations encapsulated by the data Trust may depend on the type of data being shared and for what purpose. In addition, data Trust promotes the openness of data through its sharing policy. In many cases, this sharing and governance model may have a social function, such as democratizing access to data for vulnerable or marginalized groups in society, for example, First Nations and public movements.
2.2.2. Data protection
Ensuring protection of the rights and obligations associated with shared data, then, is essential to the functioning of a data Trust (Delacroix and Lawrence Citation2019; Stalla-Bourdillon et al. Citation2020). Data provided by trustors through a data Trust must be protected from security and privacy risks, and safe for beneficiaries to use. The data Trust must be able to exert authority to protect shared data from unfair or unlawful use. To achieve this, the data Trust may enforce cybersecurity measures to secure important information from corruption, compromise, or loss, for example. A data Trust may also provide protection to personal data, such as through aggregation or anonymization of data.
2.2.3. Accountability
A data Trust must provide assurances as to its abilities to discharge its responsibilities to mitigate and minimize risks (Delacroix and Lawrence Citation2019; Mukhametov Citation2021; Stalla-Bourdillon et al. Citation2020). For example, a data Trust must assess and monitor potential risks to trustors of releasing data and proactively scrutinize its own methods for sharing. Other potential risks to beneficiaries may include uncertainty and poor quality of data, leading to being unfit for the required purpose. Data quality in particular may strongly affect costs and the opportunities for the re-use of data. Failures in any of these areas may have knock-on reputational risks to a data Trust. However, ultimately, the trustees must provide sufficient mechanisms to be held directly accountable for those failures. Trustees may discharge those responsibilities with reference to the FAIR (findable, accessible, interoperable, and reusable) principles for data reuse (Wilkinson et al. Citation2016; Boeckhout, Zielhuis, and Bredenoord Citation2018), becoming increasingly widely adopted Mons et al. (Citation2017).
More specifically, trustees can impose requirements in accordance with FAIR principles. For example, a board of trustees may make data findable and reusable by man-dating deposited data include accurate provenance information, a clear agreement for data use, detailed metadata, and unique digital object identifiers (DOIs). Similarly, trustees can increase interoperability and accessibility by requiring standardized data formats and access protocols, backed by secure and authenticated data storage.
2.2.4. Transparency
Finally, a data Trust must provide transparency in communication between key stakeholders (Stalla-Bourdillon et al. Citation2020). Transparency is essential for building a stronger relationship between trustors and beneficiaries. For example, a data Trust is responsible to inform trustors and beneficiaries about any agreements, permissions, or restrictions on data. Transparency is in accordance with the FAIR principles already highlighted as important to data Trusts, above.
2.3. Development of data trusts
Research on the development and implementation of data Trusts is still in its relatively early stages. Nevertheless, Paprica et al. (Citation2020) identified five main requirements for establishing a successful data Trust: (i) to satisfy a legal framework for facilitating, protecting, and sharing data; (ii) to be an accountable and transparent data governing body; (iii) to provide well defined and organized guidelines and policies for data management; (iv) to enforce data beneficiaries to comply with data Trust agreements and policies; and (v) to enable an early, effective, and direct relationship with public and relevant stakeholders. In addition, according to Open Data Institute (Citation2018a), the fulfillment of five main requirements mentioned above can be achieved with six different phases (see ) in the development of data Trusts:
Scoping aims to identify the challenges associated with the governance of the data. Scoping typically involves a suitability analysis of the potential and appropriateness of the use of a data Trust. The scoping phase involves exploring the benefits for stakeholders (individuals, government and non-government organizations, businesses, public and community groups, and others) and building relationships with potential trustors and data beneficiaries of the proposed data Trust.
Co-design defines the purpose of the data Trust, its business model, and its organizational structure. For example, the purposes of a data Trust might include: improving urban livability; mitigating environmental risks, such as bushfires and flooding; or supporting geospatial analytics for improved environmental decision- making. Co-design also explores the available sources of funding and develops the organizational and legal structure of a data Trust. This includes providing a set of agreements on how data is managed, protected, and shared, including data standards and technical requirements. For example, the new board of trustees may decide on who and how many people should be involved in Trust’s daily operations. Also, the co-design stage can define potential benefits to all data trustors and beneficiaries.
Launching starts the organization in compliance with its defined purpose and co-designed agreements. A data Trust is required to conduct its operations in an accountable and transparent manner. This involves carefully executing and managing data agreements with data trustors and beneficiaries.
Operation requires that a data Trust maintain key functions, including data deposit, privacy protection, executing (approving or rejecting) data requests, advisory support, and technical services related to data management. In addition, the data Trust may need to secure funding for its operations and conduct marketing to promote its services. The data Trust may carry out audits and regular checks to asses if all stakeholders (trustors and data beneficiaries) conform to the Trust’s values and guidelines. For example, audits and regular checks may detect a potential breach of data privacy and reduce other risks associated with data mishandling and mismanagement of data.
Evaluation involves the assessment of the overall impact of the data Trust on the process of facilitating improved data access, better data protection, and increased data sharing. For example, data trustors and beneficiaries may be asked to provide feedback and advice about potential improvements to the data Trust. Operations and services conducted by the Trust may be evaluated to determine their correctness and the appropriateness of Trust decision-making processes. The evaluation may be conducted by external third-parties to avoid conflicts of interest within the Trust. In addition, the business model of a data Trust may also require evaluation to determine if the Trust itself should be redesigned or retired.
Retiring is the final stage of the data Trust life-cycle. In some cases, the evaluation of a data Trust may demonstrate a need for the Trust to be retired. If so, an appropriate timeline for closing down the Trust will be set and communicated to relevant stakeholders. The operations and services will be shut down and relevant agreements with data trustors and data beneficiaries must be terminated in an orderly manner before the closure of a data Trust.
Figure 3. A schematic view of data Trust life-cycle (Open Data Institute Citation2018a).
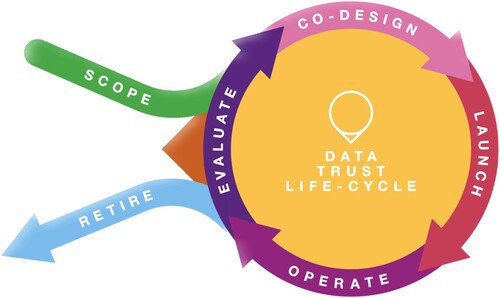
2.4. Challenges for data trusts
The development of an effective and sustainable data Trust comes also with some challenges. For example, challenges to achieve financially sustainable data Trusts are often associated with the scope and co-design stages of the data Trust life-cycle. According to Open Data Institute (Citation2020), most data Trusts have limited funding resources before becoming fully operational. Hence, data Trusts may face particular financial challenges at the beginning of their operation. At the beginning of operation, a data Trust may need to rely only on grants, volunteers, and other funding resources.
One of the most challenging aspects of data Trust operation is access funding for the design and implementation of new data management, protection, and sharing services, in response to evolving risks, such as privacy breaches. Most data Trusts have limited operating funding to invest in organizational changes. In turn, this can impact a data Trust’s business model and decision-making processes. Other risks can include the breakdown in the relationship with the key stakeholders, including local community groups, individuals, and private and government organizations (Open Data Institute Citation2020). For instance, a failure of the Sidewalks Labs pilot project, Urban Data Trust, was attributed to a stakeholder perception of insufficient transparency, lack of consultation, lack of accountability, and privacy risks associated with using public space for Sidewalks Labs’ economic interests (Scassa Citation2020; Mann et al. Citation2020). Arguably, the proposed Trust provided insufficient clarity about processes and goals related to facilitating, protecting, and sharing collected data.
2.5. Alternative approaches to spatial data governance
Definitions of governance generally concern strategies for ‘achieving direction, control, and coordination of wholly or partially autonomous individuals or organizations on behalf of interests to which they jointly contribute’ (Lynn, Heinrich, and Hill Citation2000, p. 235). Acts of governing are typically a combination of three common modes of coordination—hierarchies, markets, or networks (Thompson et al. Citation1991). Crompvoets and Ho (Citation2019) used these modalities to distinguish between structural versus managerial instruments. However, while a multi-modal approach is common in governance strategies, at times, these modes can be in competition with one another (Meuleman Citation2008).
The governing of spatial data has been and continues to be an ongoing challenge for governments and industries alike. In part, this is due to the heterogeneous nature of spatial data production, resulting in differing types and quality of spatial data. Historically, National Mapping and Cadaster Agencies (NMCAs) have been one of the primary spatial data governance approaches globally. One of the main roles of an NMCA is collecting, managing, and sharing authoritative spatial data for greater socioeconomic benefits, including urban planning, construction, environmental management, and other public purposes (Bennett et al. Citation2012; Kent and Hopfstock Citation2018; Seifert and Salzmann Citation2022). The spatial data governance model of an NMCA can be seen as a top-down approach, managed by spatial professionals and driven by structural instruments with full control over spatial data (i.e. collection, quality assessment, protection, and distribution). More recent times have also witnessed the rapid expansion of corporate spatial data governance, such as by GoogleFootnote3, MetaFootnote4, AmazonFootnote5, and 1spatialFootnote6, with increasing commercial revenue the overriding priority.
In governments and NMCAs, spatial data infrastructures (SDIs) have been the common approach to facilitate data discovery, access, and use of multi-source spatial data (Groot Citation1997; Rajabifard and Williamson Citation2001; Granell et al. Citation2009). In turn, the governance of SDIs has become a topic of importance often in the context of a legislative framework. For example, the EU INSPIRE Directive is one of the most notable instances of SDI governance, which itself acts as a framework for the meta-governance of spatial data Crompvoets et al. (Citation2018).
As the quantity and quality of spatial data have grown significantly, so discourse around spatial data and SDI governance has evolved in response. For example, Sjoukema, Bregt, and Crompvoets (Citation2017) have analyzed the longstanding SDIs of Belgium and Netherlands and showed how the governance of these SDIs has adapted over time in response to changing goals and needs. They conclude this evolution is moving towards an approach that adopts a more diverse mix of policy instruments to enhance governance, albeit also seeming to preference hierarchical instruments. Another increasingly common variant of SDIs that emphasize the more technical aspects of spatial data sharing is digital infrastructures like Digital Earth (DE), a concept popularized by then US Vice-President, Al Gore (Gore Citation1992). A DE similarly functions as a platform or ecosystem for sharing, analyzing, and visualizing spatial data for improved insight and decision-making with a clear mandate to provide a public benefit (Nativi, Mazzetti, and Craglia Citation2021). However, in addition to large volumes of dynamic spatial data, DE platforms often combine repositories of big data with capabilities for machine learning, artificial intelligence, and spatial analysis. This presents new challenges in governing spatial data as most of the data and techniques populating DEs remain proprietary and existing governance approaches tend to exclude citizens (Micheli et al. Citation2020, Citation2022).
While not explicitly spatial, Janssen et al. (Citation2020) propose an approach to data governance approach for big, open, and linked data (BOLD) and related big data algorithmic systems (BDAS). The authors note that since BDAS relies on myriad input data sources—some with known provenance, others without—effective data governance is essential to trustworthy BDAS. The authors propose an AI-based BDAS and associated framework of data governance that includes a system-level governance model for BDAS, data stewardship and base registries, and a trusted data-sharing framework.
Moving away from larger scale models that address the needs of large data-producing organizations, Micheli et al. (Citation2020) explored four emerging models of data governance that tend to be practiced by smaller data actors as alternatives: data sharing pools, data cooperatives, public data trusts, and personal data sovereignty. The authors argue that it is important to consider the social aspects and power relations in data production, use, and benefit and that inclusive, ‘bottom-up’ approaches led by civic society and public bodies are key to more democratic data governance approaches.
2.6. Challenges for spatial data governance alternatives
Despite these efforts to establish clear spatial data governance mechanisms, there are still many areas where spatial data governance remains under-realized and underresearched. NMCAs as large and complex government institutions often have legal and organizational difficulties in providing timely decisions and responding to rapid socioeconomic and technological changes (Hämäläinen and Krigsholm Citation2022; Nedovićöć-Budić and Pinto Citation2000). Although NMCAs share some of the common key functions (data protection and accountability) with data Trusts, the organizational and structural data governance approach of NMCAs is typically incompatible with an open, decentralized, and bottom-up governance approach (Robinson et al. Citation2010). For example, on the longstanding critical issue of self-determination and governance of Indigenous spatial data, there remains limited confidence in NMCAs (Gupta, Blair, and Nicholas Citation2020; Rainie et al. Citation2019; Terri Janke and Company Citation2017; Sletto Citation2009). In an era of advocacy around open data, making Indigenous data findable, accessible, interoperable, and reusable (i.e. the FAIR principles introduced above, (Wilkinson et al. Citation2016)) can actively present concerns related to undermining self-determination and the protection of sensitive Indigenous knowledge. In response, the CARE principles (collective benefit, authority, responsibility, and ethics) were developed by the Global Indigenous Data Alliance (Citation2019) to ground data governance in Indigenous worldviews and to ensure data is used for the benefit of Indigenous communities. Another instance of this is the Canadian First Nations governance framework, OCAPTM (ownership, control, access, and possession), which ensures individual and community ownership of information.
On the other hand, commercial companies (e.g. Google, Amazon, and Meta) must pursue their own independent and commercially-driven data governance solutions. This can often lead to siloing, inaccessibility, higher costs, fragmentation, and duplication of spatial data. Specifically, the commercially-driven spatial data governance approach is in contrast to the FAIR principles and OCAPTM governance framework mentioned above. Hence, commercial spatial data governance models tend to exacerbate spatial data sharing issues such as lack of accessibility, affordability, and availability of spatial data.
There is also growing awareness of the wider challenges of ethical use of spatial data, especially sensitive personal location data. For example, the release of personal data about trips on Melbourne’s public transport system by Public Transport Victoria (PTV) to a 2018 Melbourne Datathon event raised serious concerns over location data privacy (Mary Citation2019). A group of researchers from the University of Melbourne were able to re-identify themselves by using released transportation data captured in 2018 (Culnane, Rubinstein, and Teague Citation2019). Using social network data (Twitter), researchers were able to re-identify co-travelers and even strangers’ identities. In March 2014, the New York City Taxi & Limousine Commission released 173 million individual taxi trip logs recorded by taxis’ GNSS, under a Freedom of Information (FOI) request. Using basic spatial analysis skills, the pseudonym identifiers for individual taxi drivers enable the reidentification of drivers’ income, demographics, work patterns, and even home addresses (Douriez et al. Citation2016). Further studies showed the potential to reidentify passenger behaviors and identities with only a small amount of auxiliary knowledge, such as celebrity gossip blogs used to identify a celebrity’s movements (Tockar Citation2014). Tracking and maintaining data subjects’ consent is frequently a further weakness in location privacy protection. British company Huq (https://huq.io/) recently reported in a privacy breach that users’ location information was collected without the appropriate user’s consent (Wakefield Citation2019).
Recent research has demonstrated how location data can be used to reveal sensitive personal attributes, such as race, income, and education Pandey and Caliskan (Citation2021). Spatial data is widely used in decision and policy-making applications, and as a consequence, uncontrolled access and analysis of such data can open the possibility of targeting disadvantaged communities, leading to social discrimination and unfair treatment, for example, in access to shared resources, financial equity, or hiring decisions. Though there are mechanisms Hajian, Bonchi, and Castillo (Citation2016) to protect data from biased algorithms and analyses in general, such mechanisms are not well suited to the structure and continuous nature of geographic space Shaham, Ghinita, and Shahabi (Citation2022); He et al. (Citation2022). The application of general purpose techniques for the protection of sensitive data from algorithmic bias may render spatial data unfit for use Riederer and Chaintreau (Citation2017).
According to Huijboom and van den Broek (Citation2011) and Johnson et al. (Citation2017), the protection of sensitive data and individual privacy may also increase costs and create additional barriers to the accessibility and shareability of spatial data. In addition, poorly developed access mechanisms and insufficient data provenance information may be another critical barrier for increasing accessibility and sharing of data. For example, according to Sieber and Johnson (Citation2015) and Robinson and Mather (Citation2017), storing data in a freely accessible and open data repository may in isolation not increase the chances of use and sharing of spatial data.
In addition, some challenges related to sharing of spatial data generated by government organizations are related to the costs of open data provision (Johnson et al. Citation2017; Janssen, Charalabidis, and Zuiderwijk Citation2012). This includes the costs of data collection, publication, and sharing together with the implementation and maintenance of online data platforms. The data quality may also vary between different spatial data governance organizations, which provides further barriers to assessing and managing fitness for use of spatial data. Other costs and limitations associated with open and shareable spatial data include inappropriate data preparation and structure, and unequal and inconsistent provision of open data by different government and non-government organizations. These challenges have a negative impact on the success of spatial data sharing and SDI implementation (Nedovíc-Budíc and Pinto Citation2000; Harvey and Tulloch Citation2006; Janssen, Charalabidis, and Zuiderwijk Citation2012).
Ultimately, the true value of spatial data—both social and economic—can only be realized if the production, use, and management of spatial data are governed effectively. Such governance becomes even more critical in an era of artificial intelligence and machine learning, where big data algorithms can inadvertently present risks (e.g, by reinforcing biases, lack transparency, etc., Janssen and Kuk Citation2016). Hence, this paper focuses on spatial data Trusts as an emerging and alternative approach to spatial data governance that complements and extends the significant literature on the governance of spatial data introduced above. The following section reviews some of the successfully developed data Trusts today, including some pilot data Trusts.
3. Data trust initiatives
In recent years, new data Trust initiatives have begun to emerge across different domains and industry sectors. This section reviews some of the key established and successfully developed data Trusts, together with emerging initiatives and pilot projects, as the basis for our analysis of data Trusts for the spatial domain.
3.1. Health and human services
Some of the most successful early adoptions of data Trusts are focused on protecting, managing, and sharing medical data for purpose of research in the health domain. MIDATAFootnote7, for example, is a not-for-profit data Trust that aims to improve the use and increase the value of health data, while allowing individuals to retain control over their personal information. The organizational structure of the MIDATA Trust includes a development team, a board, and a council of data ethics. Its operational services are funded by paid research trials and membership fees. Members of MIDATA are able to contribute data to medical research by providing permission to access their personal information or actively participating in data stewardship (). The MIDATA Trust is accountable for risks related to securing and sharing the medical data of members.
Figure 4. Overview of MIDATA framework (MIDATA Citation2022).
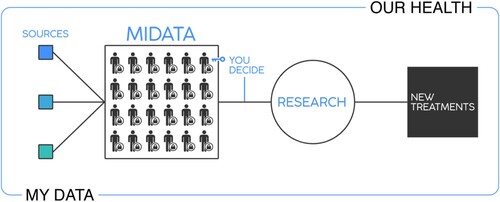
Open HumansFootnote8 is another not-for-profit, community-based data Trust that enables medical and other individual data collections across different data streams for research, education, and health projects (Greshake Tzovaras et al. Citation2019). This data Trust is managed by a nine-member board of directors and funded through grants. Open Humans allows access to personal data, but also allows members to restrict and control sharing of their own data. Similar to MIDATA, the Open Humans Data Trust enables granular and dynamic control over medical data, supporting bottom-up decision-making. Each individual can either accept or reject the use of their own data for a particular project. Available data include personal genetic and medical data, but also other data types—including location data—collected from mobile devices (such as GNSS location, FitBit data, Google location history data, RunKeeper data, and so on).
Other successful data Trusts in the sector include the Johns Hopkins Data TrustFootnote9, which aims to secure and protect Johns Hopkins Institute patients’ data, while enabling controlled sharing for beneficial data analytics and research. The Trust is funded by federal and state agencies and other industry partners. The Silicon Valley Regional Data TrustFootnote10 (SVRDT) is another data-sharing platform that provides collection, privacy, and protected sharing of administrative data from health and human services, as well as education and juvenile probation in the three counties in Silicon Valley. The Silicon Valley Regional Data Trust aims to enable data-driven decision-making and analytics for improved educational service and information sharing. It also serves as a research tool for analyzing student learning skills and to help improve school and life experiences for young people in Silicon Valley. The data Trust is supported financially by state and local government agencies as well as donations.
3.2. Finance and infrastructure
TRUATAFootnote11 is a private data Trust founded by IBM and Mastercard. The Trust provides a set of standards for hosting, anonymization, and protection of data according to European General Data Protection Regulation (GDPR). It also includes data analytics services and tools which can support insights, algorithms, and reports to unlock value in data for beneficiaries (i.e. clients, see ). Specifically, illustrates TRUATA services through thirteen steps of collecting, de-identifying, testing, aggregating, analyzing, and utilizing customer data while reducing the risks associated with privacy breaches.
Figure 5. Overview of TRUATA data Trust workflow (Lee and Behan Citation2019).
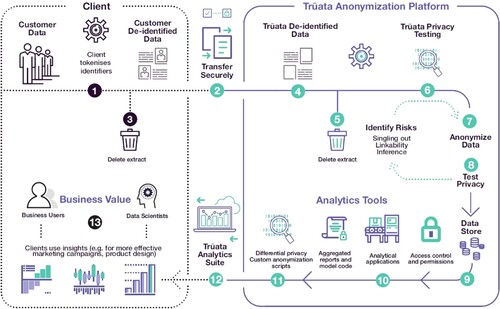
BenevaFootnote12 is another private data Trust funded by the Beneva insurance company. The data Trust enables data protection and privacy for customers through a high level of anonymization of personal data. Data sharing is executed through a common customer portal which records clients’ actions and aims to maintain data quality. From an wider industry perspective, the Construction Data TrustFootnote13 is a not-for-profit data Trust that enables the construction industries to share their data in a secured and access controlled manner. The shared data aims help industries to improve performance, productivity, and safety issues within construction.
3.3. Civic and social
In the public sector, The Commonwealth Data TrustFootnote14 is a public data governance body implemented and funded by the Office of Data Governance and Analytics in Virginia, US.Footnote15 This data Trust provides a safe and compliant data sharing framework that sets requirements for its members through a data user agreement (Office of Data Governments and Analytics Virginia Citation2022). One of the main roles of this data Trust is also to enable data discovery and analysis for government and non-profit organizations in the Commonwealth of Virginia, including the Department of Aviation, Library of Virginia, Virginia Department of Health, among others. Shared data may include personal information (date of birth, race, employment, and occupation), location, metadata.
In the UK, the Greater London Authority (GLA) and Royal Borough of Greenwich (RBG) Data Trust Pilot (Open Data Institute Citation2018b) are investigating the feasibility of the set up of a data Trust to support two use cases of Sharing Cities program.Footnote16 The first use case aims to make the less-polluting transport options popular by using parking data, and the other use case aims to improve the energy efficiency of social housing. The study applies different methodologies to understand the objectives and issues with the data collection and sharing strategies of Sharing Cities program, and the needs of the stakeholders. Although the study did not identify a clear supporting argument for creating a data Trust for Sharing Cities program, it does identify a number of recommendations for the establishment of a data Trust to improve public services in London.
The Food Data Trust (Brewer et al. Citation2021) is an initiative from the UK Food Standards Agency (FSA) and the University of Lincoln to enable easy and secure exchange of information across different sectors of the food system. The shared data can create new opportunities for business and supply chain system that might otherwise not be shared in a competitive commercial environment. Food Data Trust framework defines rules and regulations for interactions, technology, and standards for interoperability between heterogeneous systems, and business models to deliver the benefits and incentives for the real world.
Other data Trusts in the social and political domains include the Worker Info Exchange (WIF)Footnote17, a non-profit data governance organization which enables data access and facilitates data protection and rights for workers and trade unions. Enabling data access to workers and trade unions, the organization aims to increase the transparency of members working conditions, job payments, and performance assessments and assists employees to protect their rights and negotiate improved working conditions. WIF is funded by not-for-profit and private organizations such as Open Society FoundationsFootnote18, Digital Freedom FundFootnote19, and Mozilla.Footnote20
Finally, DATA TRUSTFootnote21 is a data Trust for protecting, analyzing, and sharing voter and electoral data for the US Republican party, social welfare, and other for-profit and non-profit organizations. This Trust provides security and privacy protection for personal information collected from public records, consumer data vendors, and other data suppliers (DATA TRUST Citation2022). Through this Trust, data beneficiaries have access to a modeling library, historical election information, daily absentee, and early voting data. DATA TRUST’s operational costs are funded by client fees. The Trust’s clients include political parties, and consulting, marketing, and data analytics companies.
3.4. First nations
As indicated above, an emerging application area for data Trusts of particular significance is the protection of Indigenous data sovereignty. Indigenous data sovereignty (IDS) is concerned with safeguarding the rights of Indigenous nations to control the collection, ownership, and application of data generated by or about First Peoples (Rainie et al. Citation2019; Duckham and Ho Citation2020). The Maranguka Community HubFootnote22 is a successful Indigenous data sovereignty initiative guided and managed by the Bourke Tribal Council in Australia. This initiative is founded on a data Trust governance framework and supported by Seer Data & Analytics.Footnote23 The community hub holds, manages, and provides data access and sharing from several different data providers. Data providers include some of federal and local government organizations including NSW Health, NSW Education, NSW Communities & Justice, NSW Police, Department of Social Services, and non-profit organizations in Bourke Tribal Council. The trust data framework is established on principles of responsible, safe, sovereign data governance including data ownership rights, sharing, access, and protection rules (Seer Data & Analytics Citation2021).
Another suggested pilot project proposed by Global Partnership on Artificial Intelligence (GPAI) concerns stewardship and use of Indigenous people’s data in Peru (Global Partnership on Artificial Intelligence Citation2022). The main aim of the proposed data Trust is to protect data on the migration of Indigenous people affected by major climate and environmental issues. summarizes the proposed framework for collecting, managing, and protecting Indigenous people’s data together with Trust’s relationship and engagement with other key stakeholders.
Figure 6. Proposed pilot climate data Trust project for Indigenous people’s migration in Peru (Global Partnership on Artificial Intelligence Citation2022).
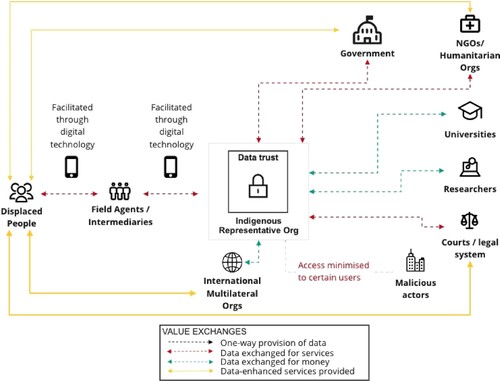
3.5. Spatial sector
Although few if any initiatives are today applying the data Trust model explicitly to spatial data, early examples are beginning to emerge. PLACEFootnote24 is an emerging spatial data Trust for high-resolution optical imagery derived from drones and commercial street camera systems (Place Citation2022b). PLACE was formed by the Omidyar NetworkFootnote25 and is supported financially by multiple foundations and industry partners.Footnote26 The trust enables the aggregation of data from a wide variety of local organizations and sources, such as local government. PLACE offers commercial licenses to beneficiaries as well as free public-good licensing. The PLACE trust is responsible for managing memberships, data access, data specifications, licensing and fees, as well as other activities such as an open learning hub. Established in 2020, the PLACE framework, illustrated in , is still in flux, but represents a glimpse of a model for spatial data Trusts that could have a significant wider impact.
Figure 7. Overview of PLACE data Trust (Place Citation2022a).
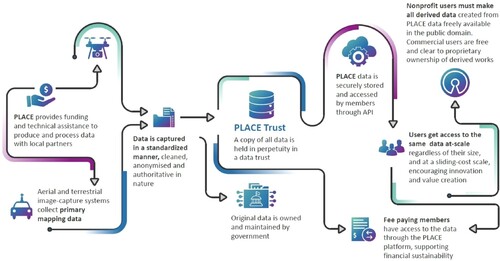
Another planned data Trust centered around spatial data is associated with the establishment of improved cycling infrastructure in London (Global Partnership on Artificial Intelligence Citation2022). The future aim of the London Cycling Data Trust is to encourage cyclists and the cycling community to contribute their movement data for the purposes of improving the cycling network in London. Aggregated movement data contributed by individual cyclists to the trust would be shared to a local government and transport authority for uses with positive environmental, social, and economic impact on urban cycling infrastructure. Such data Trust initiatives resonate with established work on user-generated geographic content (UGGC), volunteered geographic information (VGI), and citizen science (e.g. Hologa and Riach Citation2020; Knura et al. Citation2021). However, the focus of a data Trust sits squarely on the governance of data, and the barriers to sharing that can arise from a lack of clarity around the rights of trustors, rather than on techniques for capturing data from humans-as-sensors. In fact, the term ‘VGI’ has from its inception been the subject of controversy as a result of the ambiguity as to the rights of ‘volunteers’ and indeed whether data is truly ‘volunteered’ at all (Tulloch Citation2008).
3.6. Summary
While not exhaustive, the projects identified above and summarized in below provide a representative and sizable sample of the major data Trust initiatives in place or in planning at the end of 2022. The ability of the reviewed data Trust initiatives to successfully and effectively operate is attributable to:
accountable and effective data governance;
balanced assessment between benefits and risks of sharing data;
operation by independent development teams and boards of trustees;
distribution of data value to different stakeholders;
responsible data governance and active stakeholder engagement;
enforcement and compliance; and
access to sufficient financial resources to run data Trust operations.
Table 1. Summary of data Trust initiatives.
One of the most important properties of every successful data Trust is striking an appropriate balance between accountability and effectiveness of data governance (O’hara Citation2019; Open Data Institute Citation2019). In particular, a successful data Trust should avoid the implementation of too many rules to allow efficient execution of key functions (data sharing, access, openness, and transparency). However, inadequate protections may lead to unauthorized and unregulated data sharing, causing a breakdown in trust or creating conflicts of interest between trustors and data beneficiaries. Some of the data Trusts reviewed above, such as MIDATA, John Hopkins Data Trust, TRUATA, and PLACE, place particular emphasis on clear data governance rules to allow for a balanced approach between accountability and effectiveness.
The success of data Trust also relates to a balanced assessment of the benefits and risks associated with data sharing (Open Data Institute Citation2019). In general, a board of trustees should integrate this property into the underlying data Trust framework. For example, the Commonwealth Data Trust includes a data set readiness reviewFootnote27 and a group of standardized data Trust user agreements, to minimize the risks with data security and privacy while increasing the shareability of data.
Successful data Trusts are usually developed by independent teams and run by an independent board of trustees. For example, PLACE and Open Humans include in their organizational structure an independent board of trustees for overseas data Trust operations and to prevent conflict of interests between key stakeholders.
A data Trust also regulates the distribution of data value between a range of stakeholders citepDelacroix2019, ODI2018-three-pilots. In particular, many data Trusts provide appropriate policies to facilitate the use of data for commercial purposes. For example, some data Trusts—such as TRUATA, Beneva, and DATA TRUST—are predominantly focused on providing commercial data sharing services to their beneficiaries (the Trust’s clients). Other examples, including PLACE and MIDATA, allow data stewardship and data sharing operations for both commercial and non-commercial uses. Open Humans allow only non-commercial uses of data.
A successful data Trust relies on responsible data governance and active engagement with all stakeholders (Delacroix and Lawrence Citation2019; Scassa Citation2020; Seer Data & Analytics Citation2021). Active engagement with data subjects (trustors) allows the identification of appropriate operations associated with data management, protection, and sharing. For example, PLACE, Open Humans, MIDATA, SVRDT, and The Maranguka Community Hub actively work with a range of government and non-government organizations, local communities, industry partners, individuals, and other data providers to enable effective data use and sharing for addressing important social, economic, and environmental issues.
An effective data Trust also enforces rules to manage, protect, and share data (Open Data Institute Citation2018a). For instance, John Hopkins Data Trust and Commonwealth Data Trust provide clear, step-wise guidelines for protecting trustors’ data privacy. The data Trust is responsible for monitoring and preventing potential liabilities to data trustors (Pinsent Masons and Queen Mary University of London and BPE Solicitors Citation2019). For example, WIF actively carries out investigations and monitors potential liabilities often caused by unfair, unlawful, and automated dismissals and management decisions towards workers in the gig industry sector.
Finally, access to sufficient financial resources is crucial for the successful operation of data Trusts according to Open Data Institute (Citation2020). Some of the reviewed data Trust initiatives, including MIDATA, Open Humans, John Hopkins, TRUATA, WIF, and PLACE, have diversified financial resources provided by multiple industry partners, government and non-government organizations, funding institutions, and grants.
This section has provided a summary of the most important properties for a successful data Trust, based on our review of data Trust initiatives in section 3. Although the data Trust approach is gathering momentum and is set to grow rapidly in the next few years, the selection of reviewed data Trust initiatives does provide a basis for consideration of the central question in this paper, and the subject of the next section: What special functions and unique characteristics are required for a spatial data Trust to meet current challenges in spatial data governance?
4. Spatial data trusts
In the context of the preceding discussion on the general characteristics and specific examples of data Trusts, this section identifies the specific characteristics of spatial data Trusts associated with the unique nature of spatial data. Our analysis identifies five special characteristics, namely: (i) domain specificity, (ii) data quality, (iii) data privacy, (iv) data sovereignty, and (v) data value ().
4.1. Domain specificity
A fundamental implication of the review of data Trusts in Section 3 is that no single data Trust can hope to satisfy the requirements of all data trustors and beneficiaries. Delacroix and Lawrence (Citation2019) echo this observation and strongly support the development of different data Trusts across various domains. In their work, Delacroix and Lawrence (Citation2019) are explicit that multiple, different data Trusts will be required to address the specific challenges associated with various data types, privacy risks, data quality constraints, and other specialized requirements of trustors beneficiaries in different domains.
In particular, data trustees from one domain are unlikely to possess the expertise required to provide the required level of certainty and assurance to trustors and beneficiaries in another domain. Medical data held and managed by MIDATA and John Hopkins data Trusts (Section 3.1), for example, will include personal information about patients, medical assessment, medical history, and other medical information (MIDATA Citation2022) that is likely to raise issues not encountered by trustees with expertise in the financial sectors (Section 3.2), such as TRUATA, and vice versa.
The same expectations of domain specificity apply to spatial data and spatial expertise. Managing, protecting, and sharing spatial data will require trustees’ with knowledge and expertise in the spatial domain. Understanding the particular privacy, data quality, and licensing requirements of the high-resolution optical imaginary collected and managed by the PLACE data Trust (Section 3.5, Place Citation2022b), for example, will certainly require an understanding of aerial mapping systems, sensors and UAVs, and information about point cloud quality and positional accuracy that is only common among spatial domain experts.
The following sections expand on our analysis, going into detail about each specific characteristic of a spatial data Trust.
Hence, we propose as our first special characteristic of spatial data Trusts that trustees will include significant domain expertise in spatial data and spatial analysis in order to adequately address particular characteristics associated with spatial data, including issues such as spatial data quality and location privacy.
4.2. Data quality
Uncertainty is an endemic feature of geographic information. As a consequence, spatial data quality is among the most longstanding research topics in GI science (cf. Chrisman Citation1984; Fisher Citation1989; Goodchild Citation1995). Much early work on spatial data quality has its roots in the NCDCDS’ (US National Committee on Standards in Digital Cartographic Data) five elements of spatial data quality: lineage or provenance; positional accuracy; attribute accuracy; logical consistency; and completeness or exhaustiveness (National Committee for Digital Cartographic Data Standards Citation1988). While many other elements of spatial data quality have been proposed (including semantic and temporal accuracy, detail, source, and usage as distinct from lineage, map information level, reliability, and cartographic identifiability Morrison Citation1995; Goodchild and Proctor Citation1997; Aalders Citation1996; Moellering Citation1997), these five elements have influenced academic research on the topic (Guptill and Morrison Citation1995; Qiu and Hunter Citation2002; Chrisman Citation1997) and a range of data quality standards (Moellering Citation1997) including the latest ISO standard on spatial data quality (ISO Citation2013).
In practice, this classical account of spatial data quality is decreasingly evident in use today. One reason for this decline is that such a descriptive approach is often not especially helpful in practically assessing whether a particular spatial data set is in fact appropriate for a desired usage (Frank, Grum, and Vasseur Citation2004), usually termed ‘fitness-for-use’ (Chrisman Citation1984). Alternative approaches to data quality from outside of GI science have also offered different and more principled accounts of data quality. Building on the theory of semiotics, for example, (Price and Shanks Citation2005) define three key classes of data quality elements: syntactic (conformance to database or data format rules, such as logical consistency); semantic (correspondence to external phenomena, such as completeness, accuracy, precision); and pragmatic (concerning the importance or worth of data for a particular use, such as accessibility, relevance, value, or fitness for use). An approach to capturing and reasoning about data quality that is growing in practical application is via knowledge graphs and ontologies. In particular, PROV-OFootnote28 is an upper-level ontology for describing information provenance across a wide range of applications and domains (Lebo et al. Citation2013). The application of PROV-O to spatial data quality and provenance has been explored in Cox and Car (Citation2015) and Cox (Citation2017), and more recently as part of an integrated ontology of spatial sensor data, its semantics, provenance, and quality (Haller et al. Citation2019; Janowicz et al. Citation2019).
Understanding and managing the quality of data is an essential feature of data Trusts and spatial data governance. As the volume and variety of spatial data sources grows, including crowdsourced, volunteered, and user-generated spatial data (e.g. Mahabir et al. Citation2017; Rocha, Montoya, and Ortiz Citation2021, and cf. Sections 2.5 and 3.5), so the requirements to explicitly and expertly manage data quality and assess fitness for use grow.
Hence, we propose as our second special characteristic of spatial data Trusts the need to support trustors in capturing and documenting the quality of entrusted spatial data, and beneficiaries in assessing fitness-for-use of that data in their specific applications. Both these activities rely of spatial domain expertise, as discussed above.
4.3. Data privacy
Protecting privacy is a critical responsibility of the custodians of personal data, in most cases enshrined in law with serious consequences for privacy breaches. Article 12 of the UN Universal Declaration of Human Rights recognizes privacy in general as a fundamental human right (General Assembly of the United Nations Citation1948). Information privacy is a special case of privacy that concerns the right of a person to control when, how, and to what extent information about them is communicated to others Westin (Citation1967). Information privacy has become an especially urgent issue in recent years, underlined for example by the mishandling of personal data by Cambridge Analytica and Facebook, for example (Davies Citation2015; Tuttle Citation2018). Location privacy in turn is a special type of information privacy that concerns control over personal location information (Duckham and Kulik Citation2006).
Spatial data is especially vulnerable to location privacy breaches. As already highlighted, with the proliferation of location sensing technologies, personal location information today is routinely collected, communicated, and processed automatically with a high degree of precision and accuracy, feeding an abundance of location-based services. There exist a variety of mechanisms for protecting location privacy, usually classified into four categories: regulatory, privacy policies, anonymity, and obfuscation (Duckham and Kulik Citation2006).
Regulatory approaches to privacy involve establishing rules for governing the fair use of personal information, usually based on the principles of fair information practices (FIPs, U.S. Department of Justice, Office of Privacy and Civil Liberties Citation2004; U.S. Deptartment of Health, Education and Welfare, Secretary’s Advisory Committee on Automated Personal Data Systems Citation1973). FIPs can be summarized as notice and transparency, consent and use limitation, access and participation, integrity and security, enforcement, and accountability (Worboys and Duckham Citation2004).
Privacy policies are trust-based mechanisms for digitally prescribing certain uses of location information (G¨orlach, Heinemann, and Terpstra Citation2005; Myles, Friday, and Davies Citation2003). Privacy policies are not generally legally enforceable, though, and are therefore susceptible to inadvertent or malicious disclosures.
Anonymization involves disassociating personal identifiers from data. The concept of k-anonymity, for example, aims to provide minimum guarantees of the size of a set k of individuals that will always remain indistiguishable (Bamba et al. Citation2008; Duckham and Kulik Citation2005a). However, true anonymity often hinders authentication and personalization, which are essential for many applications of spatial. In another form of anonymity, called pseudonymity, an individual remains anonymous while maintaining a persistent system identity (Pfitzmann and Köhntopp Citation2001). However, pseudonyms are especially vulnerable to reidentification over time—a danger for all anonymized spatial data, as we shall see below.
Finally, obfuscation or geomasking refers to the practice of deliberately reducing the quality of location information in order to protect location privacy. Since early work in the field in Duckham and Kulik (Citation2005a, Citation2005b), many other approaches have been explored and are today widely used, including as an option available in most smartphones today to limit the level of location precision available to third parties.
The issues surrounding location privacy protection are well known to be complex, especially as a result of the potential for reidentification of even anonymized location data (such as those examples already discussed in Section 2.6). More than a decade ago, Krumm (Citation2007) tested the effectiveness of privacy protection techniques on movement data by using four different algorithms to re-identify person’s home address from anonymized movement data. Despite removing sensitive information associated with movement, the success rate of re-identifying person’s home address was from 1% to 5%. While low in absolute terms, such rates still present significant risks to individuals and raise concerns about the level of location privacy of pseudonymized spatial data. Another study by de Montjoye et al. (Citation2013) demonstrated that four locations specified hourly and with the spatial resolution provided by the mobile operator are sufficient for the identification of 95% of individuals. Of particular concern is the growing ability to combine big data from multiple sources to invade personal location privacy. In 2021, a threat analysis by the US Census Bureau found that it was possible to re-identify more than 50 million individuals by combining 2010 US census with an array of other big data sets available today. In response, the 2020 US Census saw the introduction of a new privacy protection technique called differential privacy, based on pioneering theoretical work by computer scientist Cynthia Dwork (Citation2006).
It follows that spatial data Trusts must encompass the expertise necessary to provide
assurance to trustors and beneficiaries about location privacy compliance and protection. This responsibility requires acknowledgment of the wide variety of types of location references (not only coordinates, but other spatial references such as street addresses, geocodes, and names of landmarks and points-of-interest); obfuscation and geomasking techniques for location data; as well as the wider issues of consent, privacy legislation, and fair information practices.
As a recent example, the Sidewalk Labs pilot project in Toronto, Canada was derailed in part as a result of location privacy concerns (Scassa Citation2020; Artyushina Citation2020; Mann et al. Citation2020). People entering the area covered by the project were potentially tracked, with assumed consent for those entering those public spaces. However, locals were sometimes unaware of the nature of the arrangements and there was no defined protocol for handling personal location data (e.g. if requested, how will their data be decommissioned). Concerns around these issues, and their inadequate handling through the proposed Sidewalk Labs Urban Data Trust, contributed to the untimely abandonment of this iconic smart cities project. An effective spatial data Trust will need the sophistication to be able to manage the privacy implications of personal location data to the satisfaction of trustors, beneficiaries, data subjects, legislators, and the wider public in cases like Sidewalk Labs and other examples encountered above, such as the PTV data privacy breach. illustrates an example of proposed spatial data Trust and its effective role in managing, protecting, and sharing personal location data.
Figure 9. Graphical overview of proposed spatial data Trust and its effective role in collecting, managing, protecting and sharing personal location data.
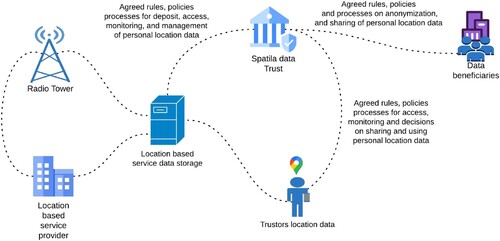
Consequently, we propose as our third special characteristic of spatial data Trusts the provision of agreed rules, policies, and processes for deposit, access, monitoring, management, anonymization, and auditing of personal location data.
4.4. Data sovereignty
Where as data privacy is connected with the right to control personal data by individuals, data sovereignty concerns the control of data by nations. The data sovereignty of nation states is today regarded as critical to national security interests, with spatial data a key component of that interest. Spatial data is acknowledged by the Australian Government, for example, as essential to national security in connection with managing risks from pandemics, wildfires, floods, and extreme weather (Australian Government, The Department of Prime Minister and Cabinet Citation2022).
As well as protecting national interests, effective mechanisms for achieving trust between nations can be essential to building the confidence for mutually beneficial sharing of spatial data. A failure to adequately manage data sovereignty of spatial data can inhibit data sharing with both domestic and international partners (Waterman et al. Citation2021). At a national level, a spatial data Trust can help to mediate data sharing between multiple countries, especially in the case of managing transboundary data sharing. For example, despite the agreement on establishing a common integrated water resources management body between Laos, Cambodia, Thailand, and Vietnam, the transboundary water and environmental issues in the Lower Mekong River basin remain unresolved in the region. According to Thu and Wehn (Citation2016), a key barrier is a willingness to share sovereign spatial data due to national security concerns. A data Trust able to enable active participation and build confidence in multi-nation data sharing holds the potential to unlock the integrated planning and analysis unachievable today, but vital to improve the resilience of local communities to floods, water pollution, and food security in the Lower Mekong River basin.
The issue of data sovereignty is of critical importance to First Nations, just as to nation states. Indigenous data sovereignty concerns the rights of First Nations and Indigenous people to control and govern data produced by and about Indigenous people, Traditional Knowledge, and the lands of which they are the Traditional Owners (Rainie et al. Citation2019; Snipp Citation2016). In times of increased digitization and data sharing, the careless transformation of Traditional Knowledge into a digital form of information often leads to the loss of ownership and control by Indigenous people and an Indigenous ‘data gap’ (Terri Janke and Company Citation2017).
In recent times, government agencies have acted as stewards by collecting, managing, and aggregating Indigenous data with the aim of preserving culture and heritage of traditional land owners. Nevertheless, this data governance framework further reinforces the historical power asymmetry between government institutions and Indigenous communities (Sletto Citation2009). Government custodianship prevents Indigenous communities from controlling their data, excluding them from decision-making, often to the direct disadvantage of those very communities. In a number of documented cases, misuse of sovereign Indigenous data and exclusion of Indigenous communities from decisions based on that data has contributed to building and mining on the land of Traditional Owners (Gooch Citation2021; Williamson, Provost, and Price Citation2022).
Another concrete example of the conflicts that can arise with government custodianship of Indigenous data is explored in the work by McCue (Citation2014). Acquiring a property in the Chilliwack region of British Columbia, Canada, the new legal owners had planned to redevelop the site. However, the presence of a known Indigenous burial mound on the site that precludes development was not listed land title documents. Indeed, tens of thousands of documented archaeological sites in British Columbia do not appear on land titles (Gupta, Blair, and Nicholas Citation2020) because unaddressed Indigenous data sovereignty issues prevent sharing of spatial data between the government’s own Land Title and Survey and Archaeology agencies. Although caveat emptor, property purchasers therefore bear the responsibility to check potential locations of Indigenous cultural and heritage sites before purchase, this lack of sharing tends to lead to inefficiency and complex litigation (Devillers and Goodchild Citation2009), that might be avoided if the mechanisms existed to share data between agencies whilst still maintaining the Indigenous sovereignty over the archaeological data.
It should therefore be no surprise that Indigenous data sovereignty is one of the early adoption areas for data Trusts (see Section 3.4). Further, Indigenous data sovereignty is acknowledged to present particular challenges in the case of spatial data (Duckham and Ho Citation2020; Gupta, Blair, and Nicholas Citation2020; McMahon, Smith, and Whiteduck Citation2017), as illustrated in the examples above. Hence, we propose as our fourth special characteristic of spatial data Trusts the ability to establish and protect data sovereignty, including Indigenous data sovereignty.
4.5. Data value
Spatial data is today being produced at an incredible rate. This production is both centralized and diffuse. At the planetary scale, space programs such as the EU’s Copernicus program and the US government’s Landsat program are producing freely available earth observation data that is commonly used to build insight on environmental and human settlement issues at various scales. At the other extreme, smartphones are now the dominant mobile device, especially in advanced economies (Taylor and Silver Citation2019). Each smartphone can routinely generate highly detailed location data about its user, whether or not they are aware of this collection. A study in 2015 found that mobile applications (especially Android ones) were profligate in tracking their users: an average of 6,200 times per participant over the two-week study period, or once every three minutes.Footnote29 With the rapid growth in sensor-based technologies, such as the Internet of Things, big data sets—and spatial big data in particular—are becoming the norm.
As more organizations turn to data-driven solutions to improve their products and services, the understanding of data value has gained ever-increasing importance (Schmarzo Citation2013). Spatial data is of immense value to society, as argued in Section
The significant value of spatial data today is only set to increase. In recent research commissioned by the Australian Government, the current value of earth and marine observation (EMO) data to Asia-Pacific Economic Cooperation (APEC) Forum economies (which accounts for nearly 40% of global population) was estimated to be US$372 billion projected to grow to US$1.35 trillion by 2030 (Australian Government, The Department of Industry, Innovation and Resources Citation2019). The value of the analysis of such data to the spatial analytics industry is predicted to be worth USD$256 billion by 2028 (Meticulous Research Citation2021).
As data valuation frameworks are usually application-specific, a general-purpose solution can be challenging to build. A big data value chain framework for end-to-end data monetization is presented in Faroukhi et al. (Citation2020). Deloitte presented a data valuation framework (Deloitte Citation2020) with four key components: (i) identifying current data assets, (ii) identifying attributes from current data assets, (iii) identifying use cases and corresponding data value exploration, and (iv) exploring alternative or future use cases if the current use cases and valuation are not satisfactory ().
Figure 10. Data valuation framework (Deloitte Citation2020).
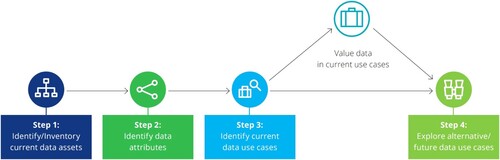
Despite the importance and undoubted value of spatial data, there is often a gap in understanding the actual value of spatial data (Shuliang, Gangyi, and Ming Citation2013). Thus it remains a challenge for individuals and organizations to define, understand, and quantify the value of their spatial data (Pantelis and Aija Citation2013). Hence, our fifth and final special characteristic of spatial data Trusts is the ability to leverage spatial domain knowledge and create a data governance model that helps unlock otherwise unrealized value in spatial data sets.
5. Discussion and conclusions
This paper has identified and explored five foundational characteristics of spatial data Trusts. These characteristics are grounded on the specific nature and attributes of spatial data, as well as lessons gleaned from emerging data Trust initiatives evident in other sectors. In all cases, spatial data brings particular issues and challenges require specific expertise and special handling.
5.1. Key challenges
Through current examples of data Trust initiatives and the identification of special characteristics of spatial data Trust, this paper has attempted to demonstrate the importance of Trusts to improve managing and sharing of spatial data. While a relatively new data governance model today, we anticipate that spatial data Trusts will play a central role in protecting, mediating, and enabling spatial data sharing between a range of key stakeholders in the future. At its core, five special characteristics identified and explored in the previous section are fundamentally concerned with improving protection and promoting sharing of spatial data.
We have argued based on the literature and exemplar data Trusts from other domains that domain specificity is a prerequisite of creating the confidence and establishing the relationships required for effective sharing. One of the key challenges in spatial data sharing is the complex structure and unique provenance information of location data. In particular, provenance metadata for location data differs from other data types used in health, finance, and civic sectors. For example, provenance information of spatial data often includes a detailed description about data format, accuracy, precision, technical requirements for observing location data, surveying methods, equipment (sensors, GPS systems, UAVs, total stations), and other data specifications. Hence, our professional spatial expertise is essential in building, managing, and operating the key functions of spatial Trust. Research by Global Partnership on Artificial Intelligence (Citation2022) showed that the requirement to engage domain experts in data stewardship as data trustees may be difficult to put into practice. Nevertheless, the presence of domain experts and spatial professionals in the role of trustees is fundamental to spatial data governance, and spatial data Trusts are no exception.
We have also emphasized that the management spatial data quality is an essential feature of spatial data Trust. A key challenge is how to manage uncertainty in spatial data, resulting from imperfect observations and equipment, operating under different environmental and physical conditions, and collected from various data sources (including crowdsourced, volunteered, and user-generated). Thus, we highlight the importance of spatial data Trusts that support the management of spatial data quality, using provenance information associated with how, where, and when data is collected, and supporting fitness-for-use assessments. Specifically, the improved management of spatial data quality under a spatial Trust should involve a board of trustees with domain expertise in spatial data quality and assessment of fitness for use.
Location privacy protection is another identified challenge to spatial data governance. Individual location may reveal personal and sensitive information (occupation, health conditions, education, food or store preference, age, and etc.). Thus, effective spatial data Trusts handling personal location data must enforce strict privacy protection measures, rules, policies, and processes to secure location data and guarantee safe sharing of personal and sensitive location data provided by trustors (individuals, organizations, and communities). Our selection of data Trust initiatives across multiple sectors (see section 3) has demonstrated the growing need for addressing the wider challenges of privacy protection and ethical use of personal data (including location data).
A further identified challenge is associated with Indigenous data sovereignty, and enabling Indigenous management, protection, and sharing of spatial data about First Nations people and lands. The historical power imbalance between (colonial) NMCAs and First Nations means NMCAs are typically ill-equipped for safeguarding Indigenous data sovereignty (Gupta, Blair, and Nicholas Citation2020; Rainie et al. Citation2019; Terri Janke and Company Citation2017; Sletto Citation2009). In contrast, spatial data Trusts such as PLACE enable protection and inclusion of vulnerable or marginalized groups, including as Indigenous communities.
Other challenges related to data sovereignty more broadly include spatial data important for disaster resilience (pandemics, wildfires, floods, droughts, etc.) and national security (transboundary conflicts, military operations, and international relations). In general, spatial data Trusts design needs to lessen asymmetries and power imbalances between trustees and beneficiaries by implementing bottom-up data governance approach (Delacroix and Lawrence Citation2019). Trusts should apply careful management, protection, and control of sharing First Nations data in particular. We also recommend that Trusts impose stronger measures for controlling the dissemination of spatial data sets of national importance while enabling potential multi-national spatial data exchange.
Data Trusts have an important role in unlocking otherwise unshared data sets by strengthening location privacy protection and enabling data sovereignty for both First Nations and nation states. Further, our work also highlights the importance of spatial data Trusts in developing the capabilities and opportunities of unlocking otherwise unrealized value in spatial data through assured and controlled data sharing. The increasing number of spatial data sources, analytical tools, and spatial applications presents another challenge in understanding the actual value of spatial data. The current rate of production of spatial data is fast, diffuse, and unsystematic, which creates a serious issues to establishing a reliable data valuation framework.
Hence, future research in support of spatial data Trusts should address defining and quantifying spatial data value. A spatial data Trust might then include a spatial data value assessment framework similar to that proposed in (Deloitte Citation2020) (see ). The assessment should provide relatively detailed information about spatial data value and potential use. The assessment of the actual value should account for openness, availability, and sharing properties of the spatial data unlocked with respect to the other four special characteristics of a spatial data Trust (domain specificity, quality, privacy, and sovereignty). For example, data beneficiaries engaging directly with a spatial data Trust may avoid complex, multi-party data-sharing agreements with many different fiduciaries and trustors. Such coordination at scale may significantly reduce the costs associated with spatial data sharing through the value chain.
5.2. Summary and general recommendations
summarizes the key challenges and general recommendations associated with our five special characteristics of spatial data Trusts. In summary, we recommend that spatial experts and professionals should be included and involved in building, managing, and facilitating the organizational structure and functions of a spatial data Trust. Spatial data Trust should report the quality of spatial data based and support assessment of spatial data fitness-for-use. Reliable and effective location privacy protection is also required for any spatial data Trust handling personal location data (see section 4.3).
Table 2. Five special characteristics, challenges, and general recommendations associated to spatial data Trusts.
Our work also indicates that successful and effective spatial data Trusts should enable control of spatial data by First Nations and Indigenous communities. Trust design should minimize asymmetry in power over data management and ensure fair, account-
able, and transparent decision processes. Spatial data Trusts should also benefit from processes to assess the value of spatial data through a valuation framework and add value through improved reliability, accessibility, transparency, and accountability.
Finally, our review indicates that a spatial data Trust should be independent, not directly involved in creating spatial data. A spatial data Trust will not normally be responsible for the technical storage and management of spatial data, however—such functions are best managed by services that specialize in this area. A spatial data Trust may require multiple funding streams to support its operations and services. Nevertheless, this work does not go as far as recommending any particular financial or organizational model to run a spatial data Trust.
5.3. Limitations and future work
The scope of our work identified and proposed five special characteristics of spatial data Trusts as an emerging spatial data governance approach. Our work does not explore the characteristics and potential use of some other prominent data governance models (data commons, data cooperatives, data collaborative, data market place, and account aggregators) for sharing spatial data. In addition, this paper does not provide a review of business and revenue models of data Trusts. Another limitation is that unlike other data Trust initiatives in health, civic, and finance sectors, spatial data Trusts are relatively new and in the early stage of their development. Hence, our work is limited to a relatively small number of currently implemented or proposed spatial data Trust initiatives in section 3. A more detailed and in-depth analysis of the future role of spatial data Trusts in a spatial data governance must await future developments in the approach.
This paper also points the direction towards further avenues for research in connection with spatial data trusts. Fairness and detection of bias in spatial algorithms is an active research topic Shaham, Ghinita, and Shahabi (Citation2022); He et al. (Citation2022); Riederer and Chaintreau (Citation2017), which connects directly with the broader aims of fairness in spatial data governance, and in spatial data Trusts specifically. A spatial data Trust might take on the responsibility for preventive measures to protect against bias in spatial analyses on spatial data sets, much as anonymization and obfuscation are applied today in the domain of location privacy.
Future research may also include exploring other alternative and complementary data governance (data commons, data cooperatives, data collaborative, data marketplace, account aggregators, and personal data stores) to spatial data Trusts. Exploration of the full range of possible sharing frameworks will be important to developing and refining effective data governance approaches in the future. Additionally, our work primarily assumes spatial data Trust supported by the legal framework of Trust law, for example, commonly applied in the UK and to land Trusts internationally. However, it may also be possible in some cases to provide comparable levels of confidence in data Trusts without explicit legal backing, especially in cases where consortia or partnerships of mutual trustors and beneficiaries emerge. In every scenario, however, the growth in volume and value of spatial data generation seems set to make spatial data Trusts and increasingly frequent component of the spatial data governance arsenal in the coming decade.
Acknowledgments
The authors are grateful for insightful early discussions with Prof Lisa Given and Prof Anne-Laure Mention.
Disclosure statement
No potential conflict of interest was reported by the authors.
Notes
2 Throughout this paper and consistent with Delacroix and Lawrence (Citation2019) we capitalize the phrase ‘Trust’ to avoid potential ambiguity when the discussion also at various points touches ‘trust’ more generally.
References
- Aalders, H. J. G. L. 1996. “Quality Metrics for GIS.” In Advances in GIS Research II; Proceedings Seventh International Symposium on Spatial Data Handling, edited by M. J. Kraak, and M. Molenaar, 1, 5B1–5B10. Delft: Delft University of Technology, and International Geographical Union.
- Ahonen-Rainio, P., and M.-J. Kraak. 2005. “Deciding on Fitness for Use: Evaluating the Utility of Sample Maps as an Element of Geospatial Metadata.” Cartography and Geographic Information Science 32 (2): 101–112. doi:10.1559/1523040053722114.
- Artyushina, A. 2020. “Is Civic Data Governance the key to Democratic Smart Cities? The Role of the Urban Data Trust in Sidewalk Toronto.” Telematics and Informatics 55: 1–13. 1016/j.tele.2020.101456.
- Austin, L. M., and D. Lie. 2019. “Safe Sharing Sites Symposium.” New York University Law Review 94 (4): 581–623.
- Australian Government, The Department of Industry, Innovation and Resources. 2019. “Current and future value of earth and marine observing to the Asia-Pacific re-gion.” Last accessed on November 25, 2022, www.industry.gov.au/publications/current-and-future-value-earth-and-marine-observing-asia-pacific-region.
- Australian Government, The Department of Prime Minister and Cabinet. 2022. “Australian Data Strategy: The Australian Government’s whole-of-economy vision for data.” Last accessed on November 22, 2022, www.ausdatastrategy.pmc.gov.au/.
- Bamba, B., L. Liu, P. Pesti, and T. Wang. 2008. “Supporting Anonymous Location Queries in Mobile Environments with Privacygrid.” In Proceedings of the 17th International Conference on World Wide Web, edited by Jinpeng Huai, Robin Chen, Hsiao-Wuen Hon, Yunhao Liu, Wei-Ying Ma, Andrew Tomkins, and Xiaodong Zhang, 237–246. New York: Association for Computing Machinery. doi:10.1145/1367497.1367531.
- Basiri, A., M. Haklay, G. Foody, and P. Mooney. 2019. “Crowdsourced Geospatial Data Quality: Challenges and Future Directions.” International Journal of Geographical Information Science 33 (8): 1588–1593. doi:10.1080/13658816.2019.1593422.
- Bennett, R., A. Rajabifard, I. Williamson, and J. Wallace. 2012. “On the Need for National Land Administration Infrastructures.” Land Use Policy 29 (1): 208–219. https://www.sciencedirect.com/science/article/pii/S0264837711000627. doi:10.1016/j.landusepol.2011.06.008
- Beresford, A. R., and F. Stajano. 2003. “Location Privacy in Pervasive Computing.” IEEE Pervasive Computing 2 (1): 46–55. doi:10.1109/MPRV.2003.1186725.
- Boeckhout, M., G. A. Zielhuis, and A. L. Bredenoord. 2018. “The FAIR Guiding Principles for Data Stewardship: Fair Enough?” European Journal of Human Genetics 26 (7): 931–936. doi:10.1038/s41431-018-0160-0.
- Brewer, S., S. Pearson, P. Godsiff, and R. Maull. 2021. Food Data Trust: A framework for information sharing.
- Bruhn, J. 2014. “Identifying Useful Approaches to the Governance of Indigenous Data.” International Indigenous Policy Journal 5 (2): 1–32. doi:10.18584/iipj.2014.5.2.5.
- Cabinet Office. 2018. An Initial Analysis of the Potential Geospatial Economic Opportunity.” Last accessed on 15 November 2022, https://assets.publishing.service.gov. uk/government/uploads/system/uploads/attachment_data/file/733864/Initial_ Analysis_of_the_Potential_Geospatial_Economic_Opportunity.pdf.
- Carto. 2018. “State of Location Intelligence 2018.” Last accessed on 15 November 2022, www.carto.com/state-of-location-intelligence-2018/.
- Chrisman, N. 1984. “Part 2: Issues and Problems Relating to Cartographic Data Use, Exchange and Transfer: The Role Of Quality Information In The Long-Term Functioning Of A Geographic Information System.” Cartographica: The International Journal for Geographic Information and Geovisualization 21 (2–3): 79–88. doi:10.3138/7146-4332-6J78-0671.
- Chrisman, N. R. 1997. Exploring Geographic Information Systems. New York: Wiley.
- Cox, S. J. D. 2017. “Ontology for Observations and Sampling Features, with Alignments to Existing Models.” Semantic Web 8 (3): 453–470. doi:10.3233/SW-160214
- Cox, S. J. D., and N. J. Car. 2015. “PROV and Real Things.” In Proc. 21st International Congress on Modelling and Simulation, edited by Robert Anderssen, Tony Weber, and Malcolm McPhee, 620–626. Canberra: The Modelling and Simulation Society of Australia and New Zealand Inc.
- Crompvoets, J., and S. Ho. 2019. “Developing a Framework for National Institutional Arrangements in Geospatial Information Management.” In Sustainable Development Goals Connectivity Dilemma, edited by A. Rajabifard, Chap. 9, 141–161. Boca Raton: CRC Press.
- Crompvoets, J., G. Vancauwenberghe, S. Ho, I. Masser, and W. D. Vries. 2018. “Governance of National Spatial Data Infrastructures in Europe.” International Journal of Spatial Data Infrastructures Research 13: 253–285. doi:10.2902/1725-0463.2018.13.art16.
- Culnane, C., A. Rubinstein, I. P. Benjamin and A. Teague. 2019. “Stop the Open Data Bus, We Want to Get Off.”
- Danish Enterprise and Construction Authority. 2010. The value of Danish address data.” Last accessed on 15 November, 2022, www.opendatatoolkit.worldbank.org/docs/value-assessment-danish-address-data-uk-2010-07-07b.pdf.
- DATA TRUST. 2022. “Data Trust Privacy Notice.” Last accessed on 15 October 2022, www. thedatatrust.com/privacy-policy/#section-2.
- Davies, H. 2015. “Ted Cruz Using Firm That Harvested Data on millions of Unwitting Facebook Users.” The Guardian Last accessed on 11 October 2022, https://www.theguardian.com/us-news/2015/dec/11/senator-ted-cruz-president-campaign-facebook-user-data.
- Delacroix, S., and N. D. Lawrence. 2019. “Bottom-up Data Trusts: Disturbing the ‘one Size Fits All’ Approach to Data Governance.” International Data Privacy Law 9 (4): 236–252. doi:10.1093/idpl/ipz014.
- Deloitte. 2020. “Data valuation: Understanding the value of your data assets.” Last accessed on 15 December 2022, https://www.deloitte.com/global/en/services/financial-advisory/perspectives/datavalue.html.
- de Montjoye, Yves-Alexandre, César A Hidalgo, Michel Verleysen, and Vincent D. Blondel. 2013. “Unique in the Crowd: The privacy bounds of human mobility.” Scientific Reports 3 (1). doi:10.1038/srep01376.
- Devillers, R., and H. Goodchild. 2009. Spatial Data Quality: From Process to Decisions. Boca Raton: CRC Press. doi:10.1201/9780367806903.
- Douriez, M., H. Doraiswamy, J. Freire, and C. T. Silva. 2016. “Anonymizing NYC Taxi Data: Does it Matter?” In 2016 IEEE International Conference on Data Science and Advanced Analytics (DSAA), edited by Osmar Zaiane and Stan Matwin, 140–148. Montreal: IEEE.
- Duckham, M., and S. Ho. 2020. “Indigeneity and Spatial Information Science.” Journal of Spatial Information Science (21): 71–82. doi:10.5311/JOSIS.2020.21.725.
- Duckham, M., and L. Kulik. 2005a. “A Formal Model of Obfuscation and Negotiation for Location Privacy.” In International Conference on Pervasive Computing, edited by Hans Gellersen, Roy Want, and Albrecht Schmidt, 152–170. Heidelberg: Springer. doi:10.1007/11428572_10.
- Duckham, M., and L. Kulik. 2005b. “Simulation of Obfuscation and Negotiation for Location Privacy.” In International Conference on Spatial Information Theory, edited by Anthony Cohn and David Mark, 31–48. Heidelberg: Springer. doi:10.1007/11556114_3.
- Duckham, M., and L. Kulik. 2006. “Location Privacy and Location-Aware Computing.” In Dynamic & Mobile GIS: Investigating Change in Space and Time, edited by R. Billen, E. Joao, and D. Forrest, Chap. 3, 33–52. Boca Raton, FL, USA: CRC Press.
- Durrant, A., M. Markovic, D. Matthews, D. May, G. Leontidis, and J. Enright. 2021. “How Might Technology Rise to the Challenge of Data Sharing in Agri-Food?” Global Food Security 28: 1–8. doi:10.1016/j.gfs.2021.100493.
- Dwork, C. 2006. “Differential Privacy.” In Automata, Languages and Programming, edited by M. Bugliesi, B. Preneel, V. Sassone, and I. Wegener, 1–12. Heidelberg: Springer.
- European Parliament and Council of the European Union. 2016. “General Data Protection Regulation.” Last accessed on 1 February 2023, https://gdpr-info.eu.
- Faroukhi, A. Z., I. El Alaoui, Y. Gahi, and A. Amine. 2020. “An Adaptable Big Data Value Chain Framework for End-to-End Big Data Monetization.” Big Data and Cognitive Computing 4 (4): 1–27. doi:10.3390/bdcc4040034.
- Fisher, P. 1989. “Knowledge-based Approaches to Determining and Correcting Areas of Unreliability in Geographic Databases.” In Accuracy of Spatial Databases, edited by M. F. Goodchild, and S. Gopal, 45–54. London: Taylor and Francis.
- Frank, A. U., E. Grum, and B. Vasseur. 2004. “Procedure to Select the Best Dataset for a Task.” In Geographic Information Science, edited by M. J. Egenhofer, C. Freksa, and H. J. Miller, 81–93. Berlin, Heidelberg: Springer.
- General Assembly of the United Nations. 1948. “Universal declaration of human rights. United Nations Resolution 217 A (III).” December.
- Global Indigenous Data Alliance. 2019. “CARE Principles for Indigenous Data Governance.” Last accessed on 25 November 2022, https://www.gida-global.org/care.
- Global Partnership on Artificial Intelligence. 2022. Enabling Data Sharing for Social Benefit through Data Trusts: Data Trusts in Climate.” Last accessed on December 5, 2022, https://gpai.ai/projects/data-governance/data-trusts/.
- Gooch, D. 2021. “Aboriginal Heritage ‘Totally Disrespected’ as Flinders Ranges Renewable Energy, Mining Projects Forged Ahead.” The ABC News Last accessed on December 9, 2022, https://www.abc.net.au/news/2021-12-06/aboriginal-heritage-flinders-ranges-mining-battery-yadlamalka/100676738.
- Goodchild, M. F. 1995. “Sharing Imperfect Data.” In Sharing Geographic Information, edited by H. Onsrud, and G. Rushton, 413–425. New Jersey: Rutgers.
- Goodchild, M. 2007. “Citizens as Sensors: The World of Volunteered Geography.” Geo Journal 69: 211–221. doi:10.1007/s10708-007-9111-y.
- Goodchild, M. F., and J. Proctor. 1997. “Scale in a Digital Geographic World.” Geographical and Environmental Modelling 1 (1): 5–23.
- Gore Jr, A. 1992. Earth in the Balance. Boston: Houghton Mifflin.
- Görlach, A., A. Heinemann, and W. W. Terpstra. 2005. “Survey on Location Privacy in Pervasive Computing.” In Privacy, Security and Trust Within the Context of Pervasive Computing, edited by Philip Robinson, Harald Vogt, and Waleed Wagealla, 23–34. New York: Springer.
- Granell, C., M. Gould, M. A. Manso, and M. A. Bernabe. 2009. “Spatial Data Infrastructures.” In Handbook of Research on Geoinformatics, edited by Yvan Bédard, Matt Duckham, Michael Gould, Stephen Hirtle, May Yuan, and Alexander Zipf, 36–41. Hershey: IGI Global.
- Greshake Tzovaras, B., M. Angrist, K. Arvai, M. Dulaney, V. Estrada-Galiñanes, B. Gunderson, T. Head, et al. 2019. “Open Humans: A Platform for Participant-Centered Research and Personal Data Exploration.” GigaScience 8 (6): 1–13. doi:10.1093/gigascience/giz076.
- Groot, R. 1997. “Spatial Data Infrastructure (SDI) for Sustainable Land Management.” ITC Journal 3 (4): 287–294.
- Gupta, N., S. Blair, and R. Nicholas. 2020. “What We See, What We Don’t See: Data Governance, Archaeological Spatial Databases and the Rights of Indigenous Peoples in an Age of Big Data.” Journal of Field Archaeology 45 (sup1): S39–S50. doi:10.1080/00934690.2020.1713969.
- Guptill, S. C., and J. L. Morrison. 1995. Elements of Spatial Data Quality. Oxford: Elsevier Science.
- Hahmann, S., and D. Burghardt. 2013. “How Much Information is Geospatially Refer-Enced? Networks and Cognition.” International Journal of Geographical Information Science 27 (6): 1171–1189. doi:10.1080/13658816.2012.743664.
- Hajian, S., F. Bonchi, and C. Castillo. 2016. “Algorithmic Bias: From Discrimination Discovery to Fairness-Aware Data Mining.” In Proceedings of the 22nd ACM SIGKDD International Conference on Knowledge Discovery and Data Mining, edited by Balaji Krishnapuram, Mohak Shah, Alex Smola, Charu Aggarwal, Dou Shen, and Rajeev Rastogi, 2125–2126. New York: Association for Computing Machinery.
- Haller, A., K. Janowicz, S. J. D. Cox, M. Lefrançois, K. Taylor, D. Le Phuoc, J. Lieberman, R. García-Castro, R. Atkinson, and C. Stadler. 2019. “The Modular SSN Ontology: A Joint W3C and OGC Standard Specifying the Semantics of Sensors, Observations, Sampling, and Actuation.” Semantic Web 10 (1): 9–32. doi:10.3233/SW-180320.
- Hämäläinen, E., and P. Krigsholm. 2022. “Exploring the Strategy Goals and Strategy Drivers of National Mapping, Cadastral, and Land Registry Authorities.” ISPRS International Journal of Geo-Information 11 (3): 1–16. doi:10.3390/ijgi11030164.
- Harvey, F., and D. Tulloch. 2006. “Local-Government Data Sharing: Evaluating the Foundations of Spatial Data Infrastructures.” International Journal of Geographical Information Science 20 (7): 743–768. doi:10.1080/13658810600661607.
- He, E., W. Chen, Y. Xie, H. Bao, X. Zhou, X. Jia, Z. Jiang, R. Ghosh, and P. Ravirathinam. 2022. “Sailing in the Location-Based Fairness-Bias Sphere.” In Proceedings of the 30th International Conference on Advances in Geographic Information Systems, SIGSPATIAL, edited by Matthias Renz and Mohamed Sarwat, 1–10. New York: Association for Computing Machinery.
- Hologa, R., and N. Riach. 2020. “Approaching Bike Hazards via Crowdsourcing of Volunteered Geographic Information.” Sustainability 12 (17): 1–14. doi:10.3390/su12177015.
- Huijboom, N., and T. A. van den Broek. 2011. “Open Data: An International Comparison of Strategies.” European Journal of EPractice 12 (1): 1–13.
- ISO. 2013. Geographic Information — Data Quality. Geneva: International Standards Organization.
- Janowicz, K., A. Haller, S. J. D. Cox, D. Le Phuoc, and M. Lefrançois. 2019. “SOSA: A Lightweight Ontology for Sensors, Observations, Samples, and Actuators.” Journal of Web Semantics 56: 1–10. doi:10.1016/j.websem.2018.06.003.
- Janssen, M., P. Brous, E. Estevez, L. S. Barbosa, and T. Janowski. 2020. “Data Governance: Organizing Data for Trustworthy Artificial Intelligence.” Government Information Quarterly 37 (3): 1–8. doi:10.1016/j.giq.2020.101493.
- Janssen, M., Y. Charalabidis, and A. Zuiderwijk. 2012. “Benefits, Adoption Barriers and Myths of Open Data and Open Government.” Information Systems Management 29 (4): 258–268. doi:10.1080/10580530.2012.716740.
- Janssen, M., and G. Kuk. 2016. “The Challenges and Limits of big Data Algorithms in Technocratic Governance.” Government Information Quarterly 33 (3): 371–377. doi:10.1016/j.giq.2016.08.011.
- Johnson, P. A., R. Sieber, T. Scassa, M. Stephens, and P. Robinson. 2017. “The Cost(s) of Geospatial Open Data.” Transactions in GIS 21 (3): 434–445. doi:10.1111/tgis.12283.
- Kent, A. J., and A. Hopfstock. 2018. “Topographic Mapping: Past, Present and Future.” The Cartographic Journal 55 (4): 305–308. doi:10.1080/00087041.2018.1576973.
- Knura, M., F. Kluger, M. Zahtila, J. Schiewe, B. Rosenhahn, and D. Burghardt. 2021. “Using Object Detection on Social Media Images for Urban Bicycle Infrastructure Planning: A Case Study of Dresden.” ISPRS International Journal of GeoInformation 10 (11): 1–25. doi:10.3390/ijgi10110733.
- Krumm, J. 2007. “Inference Attacks on Location Tracks.” In Pervasive Computing, edited by A. LaMarca, M. Langheinrich, and K. N. Truong, 127–143. Berlin, Heidelberg: Springer.
- Lea, N., J. Nicholls, C. Dobbs, N. Sethi, J. Cunningham, J. Ainsworth, M. Heaven, et al. 2016. “Data Safe Havens and Trust: Toward a Common Understanding of Trusted Research Platforms for Governing Secure and Ethical Health Research.” JMIR Medical Informatics 4 (2): 1–17. doi:10.2196/medinform.5571.
- Lebo, T., S. Sahoo, D. McGuinness, K. Belhajjame, J. Cheney, D. Corsar, D. Garijo, S. Soiland-Reyes, S. Zednik, and J. Zhao. 2013. PROV-O: The PROV Ontology. W3C Recommendation. United States: World Wide Web Consortium. Last accessed on 15 November 2022, www.w3.org/TR/2013/REC-prov-o-20130430/.
- Lee, J., and A. Behan. 2019. “Unlock business growth and protect consumer privacy with Trūata.” Last accessed on November 28, 2022, www.ibm.com/products/truata-anonymization-solution.
- Lynn, L. E., C. J. Heinrich, and C. J. Hill. 2000. “Studying Governance and Public Management: Challenges and Prospects.” Journal of Public Administration Research and Theory 10 (2): 233–262. Accessed November 30, 2022. www.jstor.org/stable/ doi:10.1093/oxfordjournals.jpart.a024269
- Mahabir, R., A. Stefanidis, A. Croitoru, A. T. Crooks, and P. Agouris. 2017. “Authoritative and Volunteered Geographical Information in a Developing Country: A Comparative Case Study of Road Datasets in Nairobi, Kenya.” ISPRS International Journal of Geo-Information 6 (1): 1–25. doi:10.3390/ijgi6010024.
- Mann, M., P. Mitchell, M. Foth, and I. Anastasiu. 2020. “#BlockSidewalkto Barcelona: Technological Sovereignty and the Social License to Operate Smart Cities.” Journal of the Association for Information Science and Technology 71 (9): 1103–1115. doi:10.1002/asi.24387.
- Manohar, S., A. Ramesh, and A. Kapoor. 2020. “Understanding data stewardship: taxonomy and use cases.” Last accessed on October 15, 2022, www.aapti.in/blog/data-stewardship-a-taxonomy/.
- Mary, G. 2019. “‘Shocking’ Myki Privacy Breach for Millions of Users in Data Release.” Australian Broadcasting Corporation Last accessed on November 14, 2022, https://www.abc.net.au/news/2019-08-15/myki-data-spill-breaches-privacy-for-millions-of-users/11416616.
- McCue, D. 2014. “Discovery of Ancient Burial Mounds Traps Landowners in Bureaucratic ‘Bottomless pit of Hell’.” The CBS News Last accessed on November 10, 2022, www.cbc.ca/news/canada/british-columbia/national-burial-mounds-property-dispute-chilliwack-bc-1.4979118.
- McMahon, R., T. J. Smith, and T. Whiteduck. 2017. “Reclaiming Geospatial Data and GIS Design for Indigenous-led Telecommunications Policy Advocacy: A Process Discussion of Mapping Broadband Availability in Remote and Northern Regions of Canada.” Journal of Information Policy 7: 423–449. doi:10.5325/jinfopoli.7.2017.0423.
- Meticulous Research. 2021. “Geospatial Analytics Market by Size, Share, Forecasts, & Trends Analysis.” Last accessed on November 27, 2022, www.meticulousresearch.com/product/geospatial-analytics-market-5161.
- Meuleman, L. 2008. Public Management and the Metagovernance of Hierarchies, Networks and Markets: The Feasibility of Designing and Managing Governance Style Combinations. Heidelberg: Physica.
- Micheli, M., C. M. Gevaert, M. Carman, M. Craglia, E. Daemen, R. E. Ibrahim, A. Kotsev, et al. 2022. “AI Ethics and Data Governance in the Geospatial Domain of Digital Earth.” Big Data & Society 9 (2): 1–5. doi:10.1177/20539517221138767
- Micheli, M., M. Ponti, M. Craglia, and A. B. Suman. 2020. “Emerging Models of Data Governance in the age of Datafication.” Big Data & Society 7 (2): 1–15. doi:10.1177/2053951720948087
- MIDATA. 2022. “My Data Our Health.” Last accessed on October 15, 2022, www.midata.coop/en/home/.
- Moellering, H. 1997. Spatial Database Transfer Standards 2: Characteristics for Assessing Standards and Full Descriptions of the National and International Standards in the World. Oxford: Elsevier Science.
- Mons, B., C. Neylon, J. Velterop, M. Dumontier, L. O. B. da, S. Santos, and M. D. Wilkinson. 2017. “Cloudy, Increasingly FAIR; Revisiting the FAIR Data Guiding Principles for the European Open Science Cloud.” Information Services & Use 37 (1): 49–56. doi:10.3233/ISU-170824.
- Morrison, J. C. 1995. “Spatial Data Quality.” In Elements of Spatial Data Quality, edited by S. C. Guptill, and J. L. Morrison, 1–12. Oxford: Elsevier Science.
- Mukhametov, D. R. 2021. “Collective Data Governance for Development of Digital Government. 2021 International Conference on Engineering Management of Communication and Technology (EMCTECH).Vienna, Austria.20-22 October 2021, 1–5. doi:10.1109/EMCTECH53459.2021.9619164
- Myles, G., A. Friday, and N. Davies. 2003. “Preserving Privacy in Environments with Location-Based Applications.” IEEE Pervasive Computing 2 (1): 56–64. doi:10.1109/MPRV.2003.1186726.
- National Committee for Digital Cartographic Data Standards. 1988. “Introduction and Executive Summary.” The American Cartographer 15 (1): 11–20. doi:10.1559/152304088783887161.
- Nativi, S., P. Mazzetti, and M. Craglia. 2021. “Digital Ecosystems for Developing Digital Twins of the Earth: The Destination Earth Case.” Remote Sensing 13 (11): 1–25. doi:10.3390/rs13112119.
- Nedović-Budić, Z., and J. K. Pinto. 2000. “Information Sharing in an Interorganizational GIS Environment.” Environment and Planning B: Planning and Design 27 (3): 455–474. doi:10.1068/b2652.
- Office of Data Governments and Analytics Virginia. 2022. “Commonwealth Data Trust.” Last accessed on 20 October 2022, www.odga.virginia.gov/resources/commonwealth-data-trust/.
- O’hara, K. 2019. Data Trusts: Ethics, Architecture and Governance for Trustworthy Data Stewardship. Southampton: Web Science Institute. doi:10.5258/SOTON/WSI-WP001. https://eprints.soton.ac.uk/428276/.
- Open Data Institute. 2018a. “Data trusts: lesson form three pilots.” Last accessed on 10 February 2023, https://www.theodi.org/article/odi-data-trusts-report/.
- Open Data Institute. 2018b. “Defining a ‘data trust’.” Last accessed on 10 October 2022, www.theodi.org/article/defining-a-data-trust.
- Open Data Institute. 2019. “Greater London Authority and Royal Borough of Greenwich Data Trust Pilot.” Accessed 2022-10-10. https://theodi.org/article/data-trusts-gla/.
- Open Data Institute. 2020. “Designing sustainable data institutions.” Last accessed on February 05, 2023, https://www.theodi.org/article/designing-sustainable-data-institutions-paper/.
- Pandey, A., and A. Caliskan. 2021. “Disparate Impact of Artificial Intelligence Bias in Ridehailing Economy’s Price Discrimination Algorithms.” In Proceedings of the 2021 AAAI/ACM Conference on AI, Ethics, and Society, AIES ‘21, edited by Marion Fourcade, Benjamin Kuipers, Seth Lazar, and Deirdre Mulligan, 822–833. New York: Association for Computing Machinery.
- Pantelis, K., and L. Aija. 2013. “Understanding the Value of (big) Data.” In 2013 IEEE International Conference on Big Data, edited by Xiaohua Hu, Tsau-Young Lin, Vijay Raghavan, Benjamin Wah, Ricardo Baeza-Yates, Geoffrey Fox, Cyrus Shahabi, et al., 38–42. Santa Clara: IEEE. doi:10.1109/BigData.2013.6691691.
- Paprica, P. A., E. Sutherland, A. Smith, M. Brudno, R. G. Cartagena, M. Crichlow, B. K. Courtney, et al. 2020. “Essential Requirements for Establishing and Operating Data Trusts.” International Journal of Population Data Science 5 (1): 1–10. doi:10.23889/ijpds.v5i1.1353.
- Pfitzmann, A., and M. Köhntopp. 2001. “Anonymity, Unobservability, and Pseudonymity—a Proposal for Terminology.” In Designing Privacy Enhancing Technologies, edited by Hannes Federrath, 1–9. Heidelberg: Springer.
- Pinsent Masons and Queen Mary University of London and BPE Solicitors. 2019. “Data trusts: legal and governance considerations.” https://www.theodi.org/wp-content/uploads/2019/04/General-legal-report-on-data-trust.pdf.
- Place. 2022a. “Infographic.” Last accessed on 22 November 2022,
- Place. 2022b. “PLACE Overview.” Last accessed on 22 November 2022, www.thisisplace.org
- Price, R., and G. Shanks. 2005. “A Semiotic Information Quality Framework: Development and Comparative Analysis.” Journal of Information Technology 20 (2): 88–102. doi:10.1057/palgrave.jit.2000038.
- Qiu, J., and G. J. Hunter. 2002. “A GIS with the Capacity for Managing Data quality information.” In Spatial Data Quality, edited by W. Shi, M. F. Goodchild, and P. F. Fisher, 243–264. London and New York: Taylor & Francis.
- Rainie, S. C., T. Kukutai, M. Walter, L. Figueroa-Rodríguez, J. Walker, and P. Axelsson. 2019. “Indigenous Data Sovereignty.” In The State of Open Data: Histories and Horizons, edited by T. Davies, B. Walker, M. Rubinstein, and F. Perini, 300–219. Cape Town, Ottawa: African Minds and International Development Research Centre.
- Rajabifard, A., and I. P. Williamson. 2001. "Spatial Data Infrastructures: Concept, SDI Hierarchy and Future Directions".In Proc. GEOMATICS’80 Conference, 10.National Cartographic Center, Tehran.pages 28 - 37.
- Riederer, C. J., and A. Chaintreau. 2017. “The Price of Fairness in Location Based Advertising.” In Fairness, Accountability and Transparency in Recommender Systems, edited by Paolo Cremonesi, Francesco Ricci, Shlomo Berkovsky, and Alexander Tuzhilin, 1–5. New York: Association for Computing Machinery. doi:10.18122/B2MD8C.
- Rinik, C. 2020. “Data Trusts: More Data Than Trust? The Perspective of the Data Subject in the Face of a Growing Problem.” International Review of Law, Computers & Technology 34 (3): 342–363. doi:10.1080/13600869.2019.1594621.
- Robinson, P., and L. W. Mather. 2017. “Open Data Community Maturity: Libraries as Civic Infomediaries.” Journal of the Urban & Regional Information Systems Association 28 (1): 31–38.
- Robinson, D., H. Yu, W. P. Zeller, and E. W. Felten. 2010. “Government Data and the Invisible Hand.” In Access to Public Sector Information, edited by B. Fitzgerald, Chap. 10, 1, 194–205. Sydney: Sydney University Press. https://open.sydneyuniversitypress.com.au/9781920899394/apsi1-government-data-and-the-invisible-hand.html#Chapter10.
- Rocha, L. A., J. Montoya, and A. Ortiz. 2021. “Quality Assurance for Spatial Data Collected in Fit-for-Purpose Land Administration Approaches in Colombia.” Land 10 (5): 1–16. doi:10.3390/land10050496.
- Scassa, T. 2010. “Geographical Information as ‘Personal Information’.” Oxford University Commonwealth Law Journal 10 (2): 185–214. doi:10.5235/147293410794895322.
- Scassa, T. 2020. “Designing Data Governance for Data Sharing: Lessons from Sidewalk Toronto.” Technology and Regulation 2020: 44–56.
- Schmarzo, B. 2013. Big Data: Understanding how Data Powers big Business. San Francisco: John Wiley & Sons.
- Seer Data & Analytics. 2021. “Community data access and sharing is at the heart of a new Bourke narrative.” Last accessed on 1 November 2022, www.seerdata.ai/maranguka-case-study/.
- Seidl, D. E., P. Jankowski, and K. C. Clarke. 2018. “Privacy and False Identification Risk in Geomasking Techniques.” Geographical Analysis 50 (3): 280–297. doi:10.1111/gean.12144.
- Seifert, M., and M. Salzmann. 2022. “Cadastre.” In Springer Handbook of Geographic Information, edited by W. Kresse, and D. Danko, 581–611. Heidelberg: Springer International Publishing.
- Senaratne, H., A. Mobasheri, A. L. Ali, C. Capineri, and M. Haklay. 2017. “A Review of Volunteered Geographic Information Quality Assessment Methods.” International Journal of Geographical Information Science 31 (1): 139–167. doi:10.1080/13658816.2016.1189556.
- Shaham, S., G. Ghinita, and C. Shahabi. 2022. “Models and Mechanisms for Spatial Data Fairness.” Proceedings of the VLDB Endowment 16 (2): 167–179. doi:10.14778/3565816.3565820.
- Shuliang, W., D. Gangyi, and Z. Ming. 2013. “Big Spatial Data Mining.” In 2013 IEEE International Conference on Big Data, edited by Xiaohua Hu, Tsau-Young Lin, Vijay Raghavan, Benjamin Wah, Ricardo Baeza-Yates, Geoffrey Fox, Cyrus Shahabi, et al., 13–21. Santa Clara: IEEE.
- Sieber, R. E., and P. A. Johnson. 2015. “Civic Open Data at a Crossroads: Dominant Models and Current Challenges.” Government Information Quarterly 32 (3): 308–315. doi:10.1016/j.giq.2015.05.003.
- Sjoukema, J.-W., A. Bregt, and J. Crompvoets. 2017. “Evolving Spatial Data Infrastructures and the Role of Adaptive Governance.” ISPRS International Journal of Geo-Information 6 (8): 1–21. doi:10.3390/ijgi6080254.
- Sletto, B. 2009. “`Indigenous People Don't Have Boundaries': Reborderings, Fire Management, and Productions of Authenticities in Indigenous Landscapes.” Cultural Geographies 16 (2): 253–277. Last accessed on December 15, 2022, www.jstor.org/stable/44251529. doi:10.1177/1474474008101519
- Snipp, M. 2016. “What Does Data Sovereignty Imply: What Does it Look Like?” In Indigenous Data Sovereignty, edited by T. Kukutai, and J. Taylor, 39–55. Canberra: ANU Press and Centre for Aboriginal Economic Policy Research (CAEPR).
- Solanas, A., and A. Martínez-Ballesté. 2008. “A TTP-Free Protocol for Location Privacy in Location-Based Services.” Computer Communications 31 (6): 1181–1191. Advanced Location-Based Services. doi:10.1016/j.comcom.2008.01.007
- Stalla-Bourdillon, S., G. Thuermer, J. Walker, L. Carmichael, and E. Simperl. 2020. “Data Protection by Design: Building the Foundations of Trustworthy Data Sharing.” Data & Policy 2: 1–10. doi:10.1017/dap.2020.1.
- Taylor, K., and L. Silver. 2019. “Smartphone Ownership Is Growing Rapidly Around the World, but Not Always Equally.” Last accessed on November 27, 2022, www.apo.org.au/node/219266.
- Terri Janke and Company. 2017. “Indigenous Knowledge: Issues for Protection and Management.” Last accessed on 16 November 2022, www.apo.org.au/node/182206.
- Thompson, G., J. Frances, R. Levacic, and J. Mitchell. 1991. Markets, Hierarchies and Networks: The Coordination of Social Life. London: Sage.
- Thu, H. N., and U. Wehn. 2016. “Data Sharing in International Transboundary Contexts: The Vietnamese Perspective on Data Sharing in the Lower Mekong Basin.” Journal of Hydrology 536: 351–364. doi:10.1016/j.jhydrol.2016.02.035.
- Tockar, A. 2014. “Riding with the Stars: Passenger Privacy in the NYC Taxicab Dataset.” Neustar Research 15: 1–6.
- Tulloch, D. L. 2008. “Is VGI Participation? from Vernal Pools to Video Games.” GeoJournal 72 (3): 161–171. doi:10.1007/s10708-008-9185-1.
- Tuttle, H. 2018. “Facebook Scandal Raises Data Privacy Concerns.” Risk Management 65 (5): 6–9.
- U.S. Department of Justice, Office of Privacy and Civil Liberties. 2004. “Overview of the Privacy Act of 1974.” May. Last accessed on November 18, 2022, www.justice.gov/opcl/privacy-act-1974.
- U.S. Deptartment of Health, Education and Welfare, Secretary’s Advisory Committee on Automated Personal Data Systems. 1973. “Records, Computers, and the Rights of Citizens.” Last accessed on 22 November 2022, www.justice.gov/opcl/docs/rec-com-rights.pdf.
- Wakefield, Jane. 2019. “Location data collection firm admits privacy breach.” Last accessed on October 9, 2022, www.bbc.com/news/technology-59063766.
- Waterman, L., M. Rivas Casado, E. Bergin, and G. McInally. 2021. “A Mixed Methods Investigation Into Barriers for Sharing Geospatial and Resilience Flood Data in the UK.” Water 13 (9): 1–16. doi:10.3390/w13091235.
- Westin, A. F. 1967. Privacy and Freedom. New York: Atheneum.
- Wilkinson, M., M. Dumontier, I. J. Aalbersberg, G. Appleton, M. Axton, A. Baak, N. Blomberg, et al. 2016. “The FAIR Guiding Principles for Scientific Data Management and Stewardship.” Scientific Data 3 (1): 1–9. doi:10.1038/sdata.2016.18
- Williamson, B., S. Provost, and C. Price. 2022. “Operationalising Indigenous Data Sovereignty in Environmental Research and Governance.” Environment and Planning F 0 (0): 1–24. doi:10.1177/26349825221125496.
- Woodcock, C. 2002. “Uncertainty in Remote Sensing.” In Uncertainty in Remote Sensing and GIS, edited by G. Foody, and P. Atkinson, Chap. 2. 19–24. Chichester: John Wiley & Sons, Ltd.
- Worboys, M. F., and M. Duckham. 2004. GIS: A Computing Perspective. CRC press.