ABSTRACT
This paper develops a social media-disaster resilience analysis framework by categorizing types of social media use and their challenges to better understand and assess its role in disaster resilience research and management. The framework is derived primarily from several case studies of Twitter use in three hurricane events in the United States – Hurricanes Isaac, Sandy, and Harvey. The paper first outlines four major contributions of social media data for disaster resilience research and management, which include serving as an effective communication platform, providing ground truth information for emergency response and rescue operations, providing information on people’s sentiments, and allowing predictive modeling. However, there are four key challenges to its uses, which include easy spreading of false information, social and geographical disparities of Twitter use, technical issues on processing and analyzing big and noisy data, especially on improving the locational accuracy of the tweets, and algorithm bias in AI and other types of modeling. Then, the paper proposes twenty strategies that the four sectors of the social media community – organizations, individuals, social media companies, and researchers – could take to improve social media use to increase disaster resilience.
1. Introduction
The New York Times referred to social media as a ‘lifeline’ in disasters following the devastating 2017 hurricane season in the United States (Fortin Citation2017). Social media hosted millions of requests for information, communication between family, friends, and strangers, calls for donations, spaces to share collective grief and compassion, and rescue requests and pleas for immediate help. Social network applications like Facebook and Twitter and communication apps like Zello, the walkie-talkie type app, highlighted social media’s adaptability to extreme events.
Lindsay’s Citation2011 description of two broad applications for social media in disasters foreshadowed these recent events (Lindsay Citation2011). The first application, which researchers and practitioners have traditionally focused on, sees social media as a passive, information-sharing mechanism to communicate warnings and other time-sensitive content similar to traditional media. The second application Lindsay described did not exist at the time – that social media could be a tool for emergency management and broader communities to gather, organize, and respond to real-time information such as victim requests for assistance, pictures of damages for situational awareness and assessment of needs, among many others. Michael Beckerman, then president and CEO of Internet Association, went as far to claim in his 2013 Congressional testimony that, ‘The convergence of social networks and mobile has thrown the old [disaster] response playbook out the window’ (Maron Citation2013).
While Beckerman may have been early in that statement, social media is changing disaster response. Social media is ubiquitous and represents shifts in how people interact across a variety of social contexts. With the current interest in social media in politics and social life, the question remains as to how we as scientists can utilize this new source of data to benefit disaster response and management (i.e. what insights may we be able to gain now through analyses of social media use?) Social media has changed the information ecosystem and influenced human behavior in many ways that we are literally riding in a ‘social media storm’ (Enserink Citation2022). The recent crises of social media use (such as privacy issues, biased demographic representation, malicious messages, and false news) generate serious challenges for social media use as a tool in disaster management (Ilieva and McPhearson Citation2018). We need teams of scholars building theory across disciplinary and methodological boundaries to address these challenges and ensure that research using social media data is valid and reliable for disaster management (Brainard Citation2022; Kankanamge et al. Citation2019).
This paper develops a framework by categorizing types of social media use and challenges to better understand and assess its role in disaster resilience research and management. We define disaster resilience broadly as the ability of an individual or community to ‘bounce back’ from disaster impacts, and resilience to disasters is achieved through resilience building activities from the four phases of emergency management – mitigation, preparedness, response, and recovery (FEMA Citation2006; Lam et al. Citation2016). Our goal of the framework is to help identify major knowledge gaps and methodological challenges so that we can suggest ways to improve social media use to enhance disaster resilience. We focus on Twitter use. The framework is derived primarily from several case studies of Twitter use in three hurricane events in the U. S. – Hurricanes Isaac, Sandy, and Harvey (e.g. Kirby Citation2019; Kirby, Reams, and Lam Citation2023; Mihunov et al. Citation2020; Wang et al. Citation2019; Wang, Lam, and Mihunov Citation2023; Wang et al. Citation2021; Zou et al. Citation2018, Citation2019a, Citation2019b; Zou et al. Citation2023). Hurricane Isaac hit Louisiana and Mississippi in August 2012, followed by Hurricane Sandy which made landfall in the northeastern U. S. in October the same year. Five years later, Hurricane Harvey struck the Texas coast in August (2017), causing massive flooding in Houston and its surrounding region. Analyses of Twitter use during these three hurricanes should provide useful insights into how Twitter uses changed through time and across space.
Prior to the popularity of social media use in emergency management, there has been substantial literature on active crowdsourcing using specially-designed internet platforms for communication during disasters (Harrison and Johnson Citation2016; Kankanamge et al. Citation2019; Citation2020; Liu Citation2014; Sagun, Bouchlaghem, and Anumba Citation2009), this paper aims to bring an added perspective, which is to explicitly link social media use with disaster resilience by examining the scientific issues and potential in resilience analysis and predictive modeling. We address three overarching questions: (1) What are the major contributions of Twitter use in disaster resilience research and management? (2) What are the challenges and obstacles? (3) What are the practical strategies to improve Twitter use to enhance disaster resilience? Addressing these important questions at this critical juncture of social media growth and misuses will help lay the groundwork for improved social media use that will ultimately benefit society.
2. Methodology
This paper will (1) first highlight the uses and challenges of Twitter use for disaster resilience research and management through synthesizing several case studies of three hurricane events in the U. S. – Hurricanes Isaac, Sandy, and Harvey (e.g. Kirby Citation2019; Kirby, Reams, and Lam Citation2023; Mihunov et al. Citation2020; Shams, Goswami, and Lee Citation2019; Shang, Sun, and Lam Citation2020; Singh et al. Citation2020; Wang, Lam, and Mihunov Citation2023; Wang et al. Citation2019; Wang et al. Citation2022; Wang et al. Citation2021; Yang and Ju Citation2021; Zou et al. Citation2018, 2019a, 2019b; Zou et al. Citation2023). These case studies were conducted by a multidisciplinary team and include technical resilience analyses as well as online surveys of individuals and organizations. (2) Then, the paper proposes a set of strategies that the four sectors of the social media community – including governmental and non-governmental organizations, individuals, social media companies, and researchers – could take to increase resilience capacity and reduce the impacts of disasters. In addition, the paper will include other pertinent literature in the discussion to provide historical context and help further illustrate some of the points. is an overview of the methodology used in this study.
The framework includes three sections. First, Twitter applications in disaster resilience research and management are grouped into four categories, including communication, ground truthing and emergency rescue, sentiment and content analysis, and predictive modeling. Then, four major challenges are identified, including the spread of false information, social and geographical disparities of Twitter use, technical issues such as locational accuracy, and algorithm bias. Finally, a set of strategies that could be taken by the four sectors of the social media community are proposed to help improve the uses and reduce the impacts of the four challenges. Some of the proposed strategies are straightforward and should be actionable, while others may be difficult to follow. By highlighting the uses, the challenges, and the proposed strategies, we can further reveal the gaps and needs for future research and best-practice management.
3. Social media use in disaster resilience research and management
3.1. Communication
Volunteer crowdsourcing as a form of citizen engagement has been used as a tool for disaster risk reduction (Kankanamge, Yigitcanlar, and Goonetilleke Citation2020; Kankanamge et al. Citation2019). Crisis crowdsourcing platforms developed by governments, non-governmental organizations, and concerned public are considered active crowdsourcing, such as the Scipionus platform used during Hurricane Katrina in 2005 and the Ushahidi platform for Haiti earthquake in 2010 (Harrison and Johnson Citation2016). On the other hand, expressions through social media such as Twitter are considered passive crowdsourcing. There is a wealth of literature on the best-practice methods and frameworks for designing effective crowdsourcing platforms, as well as on issues related to the trustworthiness of the crowdsourced data (Kantarci, Carr, and Pearsall Citation2016; Liu 2016).
With the popularity of social media continues to grow in the U. S. and around the world, social media has evolved into an effective communication platform. Social media has been compared to other disaster warning channels, such as television or radio, in terms of access, preference, and warning message content. Disaster scholars tested and built upon existing theoretical models about human behavior and risk communication, such as assessing the impact of warning message content and message style on individual protective action behavior (Fitzpatrick and Mileti Citation1991; Lindell and Perry Citation2012; Mileti and Fitzpatrick Citation1992; Sorensen Citation2000; Sorensen and Sorensen Citation2007). Compared with other warning message channels, social media’s unique ability to promote retransmission or serial transmission of information raised new questions for scholars about what affects the amplification or spread of potentially useful warning information through online social networks (Sutton et al. Citation2015a). Particularly, scholars identified certain content and style of the short, terse warning messages common to social media and other mobile messaging applications that affect not only comprehension but also retransmission (Sutton et al. Citation2013). Directive or instructional sentence style, identifiable hashtags (the # that appears before words to allow searching on various social media platforms), impact information, and protective action information increase the likelihood of retransmission of messages (Sutton et al. Citation2013; Sutton et al. Citation2015a; Sutton et al. Citation2015b).
On the other hand, surveys on the public perceptions of social media in disasters dampen the zeal of some proponents of social media in emergencies. A large percentage of Europeans surveyed in 2015 reported that they would not trust nonofficial sources of emergency information on social media (38%). They also felt that emergency management should not trust information from social media (30%). Furthermore, most respondents still indicated that they would rely on television for emergency information (86%). Other results from these studies do indicate some future changes, as younger people were more likely than others to state they would use social media in emergencies (Reuter and Spielhofer Citation2017).
Social media has been used as a warning channel for organizations involved in disasters. It is theorized that emergency managers could extend their risk communication impact and build trust within the community via social media. Research, though, indicates that among these hazard organizations there have historically been only ‘pockets of use and acceptance [of social media]’ (Tapia and Moore Citation2014). Emergency managers generally hold positive views on social media for information sharing, warning communication, and situational awareness, but few organizations frequently used social media to communicate with their publics or trusted the accuracy of information the same or more than news from traditional media sources (Reuter and Kaufhold Citation2018; Reuter et al. Citation2016; Su et al. Citation2013). For example, researchers found in 2012 when Hurricane Sandy occurred that while 100% of state-level emergency management agencies used social media, fewer county (68%) and local emergency management agencies (85%) used social media ‘in some capacity’ (Su et al. Citation2013). Another study shows a low rate of social media use during Hurricane Sandy among 840 police and fire departments within a 10- mile of Sandy’s landfall (Chauhan Citation2014). Limited research indicates that organizational use of social media varies over the course of the disaster and by organizational type (Kankanamge, Yigitcanlar, and Goonetilleke Citation2020). Those organizations that are highly engaged with social media must often ‘bend and adapt policies about what constitutes appropriate public communication in the face of emergency demands,’ creating ad hoc procedures for communicating through new media channels (Hughes et al. Citation2014). As social media use increases worldwide, organizational use of social media for disaster management will have to increase. Understanding the challenges and opportunities in engaging two-way communication effectively via social media by organizations is vital to improving disaster resilience.
3.2. Ground truthing and emergency rescue
Messages posted on Twitter could contain useful first-hand, real-time information on the places affected by the disasters, such as flood depth, power outages, and road damages. This crowdsourced information can serve as ground truth to help verify models such as damage assessment and emergency response. However, as elaborated in the next section, social media users are known to be biased in demographics, with younger, higher income and education, and urban dwellers tweeting more (Li, Goodchild, and Xu Citation2013). The demographic bias of Twitter users implies that social media information is also biased towards places that are of better social-environmental conditions. This bias has impacted the objectivity of scientific research and generated inequity in resource planning and management.
As social media use grows, we see new and expanding roles of its use in disaster events. A distinct new role of Twitter use is that during Hurricane Harvey, people used the Twitter platform to request rescue from flooded homes. Hurricane Harvey hit Houston and its surrounding region in August 2017, dumping over sixty inches of rainfall in some locations and causing widespread flooding. The unprecedented flood was not anticipated, and many residents resorted to social media to request rescue from flooded homes when the 911 system was overloaded and could not be connected (Zou et al. Citation2019b; Mihunov et al. Citation2020; see ). The Time Magazine called Hurricane Harvey ‘The U.S.’s first social media storm’ (Rhodan Citation2017). A recent study leveraged Bidirectional Encoder Representations from Transformers (BERT), one of the latest language models, to develop intelligent VictimFinder tools that can identify rescue requests from Twitter rapidly and accurately (Zhou et al. Citation2022). This new use of Twitter generates many important questions, such as how effective is social media in emergency rescue, what are the challenges, and how to improve Twitter use to make it more efficient for emergency rescue? More importantly, how do we overcome the inequitable access to rescue by the lower socioeconomic group who seldom tweet? ().
3.3. Sentiment and content analysis
Social media data could provide a wealth of information on people’s sentiment and attitude on various events in real time, which traditional data sources such as the Census could not. The number of tweets posted in relation to a disaster event is an indicator of people’s awareness or interest in the event (), and it is expected that people living near the event would tweet more.
Figure 3. County-level ratio indices during Hurricanes Sandy 2012 (left) and Harvey 2017 (right) showing high tweet ratios near the event (After Zou et al. Citation2019b).
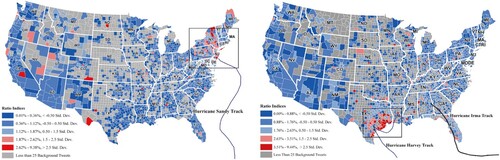
Topic modeling is increasingly used to mine the content of the tweets to detect people’s attitude towards an event (Hoque et al. Citation2022; Huang and Xiao Citation2015; Mihunov et al. Citation2022). The tweet messages posted online could be mined to help understand the state of mind of people in response to disasters and monitor the overall subjective well-being at different localities (Jaidka et al. Citation2020). Sentiment analysis is the process of assigning a sentiment score or category to each message based on its content and is commonly used in Twitter research. The Valence Aware Dictionary and sEntiment Reasoner (VADER) method, a lexicon and rule-based method, is one of the methods used to evaluate the emotional status of each tweet. VADER assigns a sentiment score ranging from −1 (most negative) to 1 (most positive) to each tweet. The VADER method has been applied to poll public attitudes towards breast cancer screening and predict election results with some success (Ramteke et al. Citation2016; Wong et al. Citation2016).
Sentiment analysis was conducted to compare residents’ attitudes during Hurricanes Sandy, Isaac, and Harvey (Wang et al. Citation2021; Zou et al. Citation2018; Zou et al. Citation2019b). It was found that sentiment scores of the three hurricanes were about the same in the preparedness phase, but they deviated greatly in the response and recovery phases. During the response phase, Hurricane Sandy in the northeastern U. S. generated more negative-sentiment tweets than the other two hurricanes in the South, but in the recovery phase, Sandy had a substantially higher sentiment score than that of the other two hurricanes (). This finding suggests a more positive experience in recovery and probably a higher degree of disaster resilience in the northeastern U. S. than in the southern states ().
Figure 4. Mean sentiment scores during Hurricanes Isaac, Sandy, and Harvey (After Wang K et al. 2021).
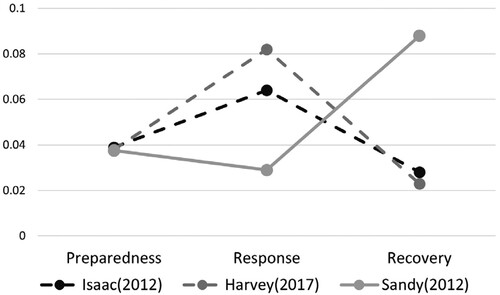
However, studies aimed to link sentiment scores with community resilience predictor variables yielded mixed results (Wang et al. Citation2021, Citation2022). No significant correlation was found between sentiment score and resilient predictor variables (e.g. age, income, education, and so on) in the case of Hurricane Isaac, whereas Twitter use patterns during Hurricane Sandy seemed to indicate that communities with better socioeconomic conditions were associated with more positive sentiment. These mixed findings suggest that more research is needed to examine the underlying factors that would affect the spatial–temporal differences in sentiment during disastrous events. Furthermore, more accurate sentiment characterization algorithms may be needed. Jaidka et al. (Citation2020) evaluated several other sentiment measurement algorithms and found that sentence-level machine learning methods to measure subjective well-being are more accurate than word-level dictionary methods such as VADER. Their study did not test VADER, but their findings further confirmed that Twitter messages could be used to measure subjective well-being of large populations if robust data-driven methods are employed.
3.4. Predictive modeling
Quantitative variables generated from social media data, such as Twitter use ratios, sentiment scores, and indices computed on their spatial–temporal trends, have been applied in various modeling studies. Zou et al. (Citation2018) conducted regression analyses between disaster damage and several independent variables (Twitter indices, sentiment score, socioeconomic variables) during Hurricane Sandy and found that incorporating Twitter indices in the model had increased the damage assessment accuracy by about 10 percent. Wang et al. (Citation2019) calculated the CDC version of social vulnerability index (SVI) for the counties affected by Sandy in the northeastern U. S. and found that the SVI index was a significant predictor of Twitter activities. The finding supports the notion that socially vulnerable communities were digitally left behind in pre-disaster social media responses to Hurricane Sandy. A study of Twitter activities during Hurricane Isaac in Louisiana and Mississippi (in 2012) yielded a similar conclusion. Significant positive correlations were found between Twitter use indices and resilience indicators, pointing to the potential of using Twitter use indices as predictors for disaster resilience (Wang et al. Citation2021). However, the same study also found no significant correlations between sentiment scores (computed using VADER) and resilience indicators, prompting the need for further research such as developing better sentiment algorithms to capture the true sentiment of the words in the messages.
Through mining and classifying the contents of the tweets, it is possible to estimate the socioeconomic characteristics of the senders such as gender, age, and education, as well as their opinions or interests on certain issues (Hinds and Joinson Citation2018). These socioeconomic characteristics, together with the sentiment expressed in the messages, could provide valuable information for studying the success or failure of policies implemented during the COVID19 pandemic. For example, are younger people more reluctant to practice preventive measures such as wearing masks, observing social distance, and taking vaccines? When integrated with other locational data, this real-time human response data enhanced by the estimated demographic characteristics could provide new insights into the best policies for combating the spread of the coronavirus (Hoque et al. Citation2022; Lee, Lam, and Curran Citation2020).
4. Challenges
4.1. False information
Concerns over the use of social media data in disaster research often relate to the validity of such information. Rumors have been a common concern during disasters (U.S. Department of Homeland Security Citation2018), but the growth of ‘fake news’ in social media raises new questions for social media use in disasters. The spreading of fake news – including both misinformation (inaccurate or outdated information) and disinformation (deliberatively fabricated false information) – is especially problematic and may critically affect people and communities during the time of disasters such as hurricanes, flooding, pandemic, or school shooting events (Rajdev and Lee Citation2015). Social media is effective in disseminating useful disaster-related news from authoritative sources. Unfortunately, it can also be a platform for spreading false news in a faster and wider pace (Vosoughi, Roy, and Aral Citation2018; Wang and Ye Citation2018). This dis- or mis-information (DMI) affects not only the sentiment of the people, who are already troubled by the disaster, but also hinders response or recovery activities performed by emergency management agencies, potentially resulting in failed rescue operations or misallocation of vital resources for the affected.
For instance, during Hurricane Harvey a false claim was initially posted on Facebook by a user on August 30, 2017, claiming that the U. S. Federal Emergency Management Agency (FEMA) would only give aid to victims if they had received (unspecified) vaccinations (https://www.snopes.com/fact-check/fema-harvey-vaccines/). It began to spread via Facebook and Twitter. When the fact-checking website, Snopes.com, posted an article debunking this false rumor on September 5, 2017, it has already been shared more than several thousands of times in social media. Many social media users were actively sharing this Snope’s article from September 5th in an effort to counter the spread of the rumor, but it was already too late. There will always be people affected by false rumors due to the time needed for analysis and publication by Snopes.com or any other fact-checking websites. This type of DMI may discourage people who were affected by Hurricane Harvey as well as future events from seeking aid from FEMA and undermine the public trust for the recovery activities performed by FEMA.
When a rumor has a mixture of false, true, or undetermined segments in its narrative, it becomes much harder to debunk. For example, another rumor during Hurricane Harvey asserted that the Red Cross stole donated items from churches in Houston and then sold some of them and burned the others, under the protection of an Obama-era (year 2012) executive order (https://www.snopes.com/fact-check/red-cross-steals-texas/). This type of rumor would denigrate the reputation of Red Cross as a disaster relief organization and may slow down its recovery activities for the affected communities. This rumor was started on September 7, 2017 by a Facebook user who broadcasted a video with the claim. On September 21, 2017, two weeks after the Facebook post, Snopes.com published a debunking article with a label ‘mostly false’ in that the executive order in 2012 did not authorize the government or Red Cross to seize private property. However, there was no evidence to support or disprove the claim that Red Cross stole, sold, or burned the donated items. Social media users started sharing the Snope’s debunked article soon after it was published, but the original rumor had already gained 5.9 million views with more than 5,000 likes and 105,000 shares, potentially affecting a large population. Even worse, tweets about this ‘mostly false’ rumor still appeared in early November 2017, which illustrates the lasting effect of false rumors wandering around social networks.
In addition to the emergency management and disaster relief agencies, political or religious groups and figures have been the targets of false rumors and satirical humor. There was a false rumor spreading in Twitter stating that ‘Black Lives Matter thugs blocking emergency crews from reaching hurricane victims’ and ‘Joel Osteen (the famed pastor of Lakewood Church in Houston, TX) sailed through flooded Houston distributing copies of his book “Your Best Life Now”.’
Despite the growing concerns about the spread and impacts of false news, studies on false news events related to disasters in social media are limited. Vosoughi, Roy, and Aral (Citation2018) conducted an extensive study on false news origins and diffusion in Twitter and found that false news items were more ‘novel’ and more likely to be shared widely than true news. An unexpected finding was that those who spread false news had significantly fewer followers, followed significantly fewer people, were significantly less active, and were less likely to be ‘verified’ users. Their study included news mostly related to politics, terrorism, science and technology, business, and urban legends, with fewer false stories about natural disasters. Friggeri et al. (Citation2014) analyzed about 4,000 rumors that had spread on Facebook and found that fact-checking was effective in curbing rumor propagation. Allcott and Gentzkow (Citation2017) reported that false news spread increased steadily on both Facebook and Twitter in the past few years. However, since the end of 2016, false news interactions have fallen sharply on Facebook while they continue to rise on Twitter, indicating that actions taken by large social media companies could be effective in limiting the spread of false news. Although these studies provide valuable information, the spatial and socioeconomic contexts of false news diffusion have seldom been analyzed. There is a major knowledge gap on the spatial, temporal, and social characteristics of false news events related to disasters. Furthermore, reliable automated approaches to detect false news, as well as strategies to curb its spread are urgently needed.
4.2. Social and geographical disparities
Social media users are known to be biased in social and geographical contexts, with young, better educated, and urban dwellers generally tweeting more (Li, Goodchild, and Xu Citation2013). Results from our several studies on Twitter use during the three hurricanes further corroborate with this statement. During Hurricane Sandy, counties with better socioeconomic conditions tweeted more than poorer counties, and the disparities in Twitter use were most apparent in the response phase (Zou et al. Citation2018). Specifically, counties with higher percentages of young, employed, and better educated population correlated positively with their Twitter use ratios (normalized by the hurricane threat level), whereas percent mobile home contributed negatively to the normalized Twitter use ratios. Percent urban population, however, was not found to be a significant contributor in this study, possibly because the studied counties are mostly urban counties. In another study of Twitter use during Hurricane Sandy, Wang et al. (Citation2019) conducted two different spatial regression analyses between Twitter response by county and their social vulnerability index (as defined by the CDC). The findings show that physically vulnerable communities had more intense Twitter response while socially vulnerable communities had significantly low responses, further substantiating that socially vulnerable communities were digitally left behand in pre-disaster social responses to Hurricane Sandy. Similarly, in the case of Hurricane Isaac (2012), significant positive correlations between Twitter use ratio and resilience indicators were found (Wang et al. Citation2021). Then, five years after Hurricanes Sandy and Isaac, the social and geographical disparities in Twitter use still existed during Hurricane Harvey in 2017. Regression analyses between Twitter use ratio and social-environmental variables further show that counties having better social and geographical conditions tweeted more (Zou et al. Citation2019b).
The implications of social and geographical disparities of social media use to disaster resilience are concerning. In the context of scientific research, these disparities lead to representation bias and pose fundamental questions on the validity of using social media data for scientific research due to their biased samples. In the context of disaster management, policies based on social media responses from a biased sample of tweets will create inequity in resource allocation during disaster response and recovery. The results will further deepen the divide between communities that have and have not, as well as affecting the capacity of have-not communities in disaster response and recovery.
4.3. Technical issues
Real-time or near real-time processing of Twitter data during a disaster event is critical to rapid disaster response and recovery. However, major technical challenges remain. First, although several tools or Application Programming Interfaces (APLs) exist to support real-time collection and processing of social media data (e.g. Twitter APIs for filtering real-time tweets, Amazon Web Services (AMS) Glue for streaming Extract, Transform, and Load (ETL) jobs), integrating and streamlining these tools to make data download and accessible to researchers and disaster responders is difficult. We need to continuously update the settings for data collection and processing (e.g. keywords for filtered streaming) because social media trends, such as popular hashtags on Twitter, are always evolving. If we do not update the settings in a timely manner, we may miss important posts related to a disaster. Such updates usually require continuous and manual monitoring of social media posts to find emerging keywords.
Second, Twitter data are big, noisy, and at times false or inaccurate, making it hard to mine and extract relevant information to use in real-time. Developing efficient algorithms to filter irrelevant information and detect false news is needed, but it remains to be a very difficult task.
Third, extracting accurate locational information from the tweets for spatial analysis is critical to reliable disaster resilience research. Several studies have already pointed to the challenge of extracting locational information, which is related to the issue of balancing between privacy and scientific research needs. There are three common approaches to determine the location of the posted tweets: geotags (tweet from), user profile addresses (user from), and locations mentioned in tweets (tweet about). The reliability of each geolocating method depends on the disaster scenario. For instance, the ‘tweet about’ locations played a vital role in locating victims needing rescue during Hurricane Harvey (Zou et al. Citation2023). In the early days, Twitter provided its users an option to geotag each tweet with a pair of coordinates. However, in June 2019, Twitter removed this precise geotagging function, leaving only the other two options available for geolocating the tweets. Although geotagged tweets are considered the most accurate spatially, they constitute only a small percentage of the entire tweets. During Hurricane Sandy in 2012, about 1-2% of the tweets from the U. S. contain geotagged locations (Zou et al. Citation2018), whereas the global Twitter data in 2020 collected from the Internet Archive has only 0.04% of tweets geotagged (Lin et al. Citation2022). The drop in geotagged tweets could be attributed to the increasing awareness of privacy among Twitter users, leading to a much lower percentage of Twitter users tagging their messages with locations nowadays.
Locational information extracted from the user profiles may not be accurate because of the coarse location the users sometimes provide. The study of Twitter use during Hurricane Isaac in Louisiana and Mississippi shows that two rural counties in the middle of the two states had unexpectedly high Twitter use (Wang et al. Citation2021). This is because when a user specifies his/her profile location with the state name only, the algorithm will make the tweet fall into the centroid of the state, which in this study, they happen to be in rural counties (Avoyelles, LA and Attala, MS). Therefore, they can be easily detected as unreasonable. However, in other study regions where the centroids may fall into urban counties, the extra tweets loaded onto the centroid-urban counties will not be easily detected, thus the locational accuracy of the analysis based on the profile information will be difficult to estimate. Strategies to help improve the accuracy of the locational information of the tweets while maintaining privacy are critical to reliable disaster resilience research and effective emergency management.
4.4. Algorithm bias
Algorithm bias is not uncommon in scientific research and could be due to both data representation bias and bias in the model design. Algorithm bias is exemplified in areas of artificial intelligence (AI) and machine-learning (ML) algorithms (Reva et al. Citation2022). For example, a deep learning-based image detection algorithm consistently turns the face of people of color into the face of white people (Vincent Citation2020). In the realm of disaster management, since social media users are typically not representative of the general population, we need to properly adjust the distribution of training data (e.g. post-stratifying the samples) to reduce bias in the training data for AI applications (Jaidka et al. Citation2020). Because of the social and geographical disparities in social media use, vulnerable social groups often have limited access to social media or limited knowledge about how to get help using social media during disasters. If we do not consider such disparities when we prepare the training data to train AI-based models, the models will likely be working only for frequent social media users who may be less vulnerable to disasters. Similarly, regarding geographical disparities, the AI models may favor urban and well-maintained neighborhoods, leading to more attention and faster disaster responses in the rich neighborhoods and vice versa.
New and cutting-edge research is being carried out currently to find ways to mitigate the bias in AI algorithms, as well as a national effort in developing standards to improve AI applications is being promoted (Reva et al. Citation2022). For instance, rescue requests via Twitter during Hurricane Harvey are biased samples; they do not represent all the vulnerable population that need to be rescued. To mitigate the unfairness, researchers have recently attempted a multivariate Hawkes model to forecast future rescue requests (Shang, Sun, and Lam Citation2020; Zhou and Sun Citation2021). Mihunov et al. (Citation2023) recently applied the fairness criteria (independence, separation, and sufficiency) to evaluate a Random Forest regression model trained to predict rescue request rates and found that imperfect sufficiency based on housing and transportation themes existed. However, more research is needed to better understand rescue request needs to enable implementation of equal probability of being rescued for all vulnerable population in future disasters.
In summary, although progress has been made towards removing some of the challenges, such as improvement in big data processing and new algorithms to correct the social-economic biases of Twitter users (e.g. Hinds and Joinson Citation2018; Giorgi et al. Citation2022), the four challenges largely remain.
5. Proposed strategies
Given the above framework of Twitter uses and challenges in disaster research and management, our third question to be addressed is: what are the practical strategies that can be taken to improve Twitter use to enhance disaster resilience? Through synthesizing the findings from the case studies mentioned in the methodology section (which include studies on tweet analysis and modeling and online surveys of organizations and individuals), we summarize them into a set of strategies that the four sectors of the social media community –organizations, individuals, social media companies, and researchers – could take to improve social media use to increase disaster resilience. lists the proposed strategies for each of the four sectors involved in social media use for disaster research and management.
Table 1. Proposed strategies to improve Twitter use for disaster resilience (see text for detailed description).
5.1. Organizational level
Two surveys of governmental and non-governmental organizations involved in emergency response and recovery in the study regions affected by the three hurricanes were conducted and the main findings have been reported (Kirby Citation2019; Kirby, Reams, and Lam Citation2023). The goal of these survey studies was to examine how these organizations used social media for two-way communications with the public to identify ways to improve social media use at the organization level. The first survey focused on two-way communication during Hurricanes Isaac and Sandy, whereas emergency rescue during Hurricane Harvey was the emphasis of the second survey, reflecting the expanding role of social media use in emergency management five years later (from 2012 to 2017). The surveys classified the respondents into four groups: first responders (FR), governmental agencies (GOV), non-governmental organizations (NGO), and news media organizations (NEWS). The number of survey respondents for the two surveys were 269 and 64, respectively.
Findings from these two surveys offer several new insights, which help formulate our proposed strategies as listed in . First (Strategy 1), survey results suggest an opportunity for these organizations to enhance the overall community disaster resilience by increasing their two-way communications with the public during the mitigation phase of the emergency management cycle. All groups reported their lowest use of social media during this phase. The relatively quiet times between emergencies are conducive to building relationships and hazard mitigation, both collectively and at the household level. This is a logical time to build and expand communication networks and relationships with community stakeholders – relationships that should foster greater public trust in the emergency management organizations. Greater institutional trust will help community members follow the advice of public warning messages more quickly and share the warnings with family and friends. Increasing two-way communications during this important phase could increase hazard awareness and literacy, strengthen relationships with community members, increase social capital, and ultimately, enhance the overall disaster resilience for communities facing increasing exposure to future climate-related hazards.
Second (Strategy 2), both GOV and NGO groups reported that the single most significant challenge they faced was the unknown accuracy of information available through social media. This was followed by insufficient human resources to manage social media use within their organizations and the risks associated with the anonymity of users on social media. Additional problems noted by both groups included the vulnerability of technical infrastructure and problems with Internet connectivity during emergencies, followed by difficulties in learning to use some social media platforms. Also, both groups reported that language and cultural barriers within sub-populations of their communities may limit the effectiveness of their communications with the public through social media. Raising awareness and public trust of official accounts, which serve as reliable sources for true information, would reduce the chance of false news spread.
Third (Strategy 3), survey respondents reported several tactics and strategies they had implemented with their organizations to address the social media challenges. These included adoption of social media use guidelines within their organizations to facilitate faster response to the comments and questions posted by members of the community; assignment of dedicated social-media personnel to conduct more consistent monitoring of incoming messages; leveraging of staff and resources within the organization, including utilization of volunteers in some cases, to meet the higher demand for communication during emergencies; and adoption of specific guidelines for following other social media users. However, the NGOs reported devoting less attention to the verification of the accuracy of information before sharing it with the public. As a result, NGOs may be more susceptible to the inadvertent spreading of misinformation during and after a disaster.
Fourth (Strategy 4), findings from the second survey, which focused on emergency rescue during Hurricane Harvey, show that while the GOV respondents maintained a presence on more social media platforms than the other groups, the FR reported checking their social media accounts most often during the emergency. These findings show that FRs ‘listened’ more consistently to the public but did so through fewer social media platforms. Nearly all rescue requests sent by the public to any of the organizations were communicated through Facebook, and all groups reported regular use of Facebook and Nextdoor to maintain contact with the public and coordinate community resources. Several respondents shared how they used social media successfully to coordinate community resources (e.g. putting out a call for shallow-draw boats via Facebook) to contribute to rescue efforts. Many first responders reported that the neighborhood social media platform Nextdoor was especially useful, enabling them to disseminate information rapidly at the neighborhood level and providing a responsive link for two-way communication between responders and residents. However, the most-reported reason for social media non-use was ‘Not our organization’s mission.’ Interestingly, no respondents selected ‘lack of financial resources’ as an obstacle to non-use. These responses suggest that organizations could use some effort to review their missions and operations to take advantage of social media use in disaster management.
Fifth (Strategy 5), despite examples of effective social media use, the first responder (FR) group reported more general problems in converting social media requests from the public into usable information necessary for rescue missions. The problems were from a range of technical limitations, including having to route calls away from the damaged Public Safety Answering Points (PSAP) system and the use of wired 911 emergency response systems throughout much of the U. S., which were unable to support web-based communication. As a result, incorporating social media communication into the protocols of FRs would require agencies to upgrade PSAP dispatch technologies to a more adaptable system such as Next Generation 911, a web based adaptable PSAP system already being used in parts of the U. S.
Sixth (Strategy 6), multiple survey respondents also suggested that social media platforms could provide additional functions for first responders during emergencies. For example, an application module or a template could be designed and programed to allow first responders or emergency management agencies to collect information about the victims requesting assistance, such as the full address of the rescue location, number of people involved, and/or special needs requirement, as shown in . This is similar to attaching a web form to Facebook’s current Safety Check feature, which could allow responders to compile and display rescue requests geographically, including information about the victims such as age and any health concerns or physical limitations.
Figure 5. An example of Twitter emergency rescue request template (Zou et al. Citation2023).
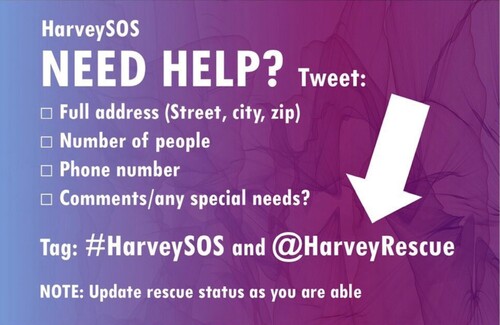
Finally (Strategy 7), a basic GIS such as a map or visual display of the calls for assistance, relevant attributes of the callers, and the rescue status of each call would support more efficient responses.
5.2. Individual level
To understand individual use of social media during disasters, an online survey of 1,000 residents in the Houston metropolitan area in 2017 was conducted by the authors, and some of the main findings are reported here. In general, respondents identified social media as the second most common source of information during Hurricane Harvey, lagging behind traditional media as a trusted source of information. Nearly 60% of respondents said television was their primary source of information, whereas 11% of respondents indicated that social media of any type (Facebook, Twitter, Nextdoor, WhatsApp, and so on) were their primary source of information on the storm. Thus, although social media has been increasing in importance since earlier studies, our survey results indicate that television was still the most common source of disaster information for residents under threat of a disaster.
Of note is that respondents expressed high trust in social media information. On a scale of 1 to 10, respondents rated the information they received via social media during Hurricane Harvey a 7.7 in reliability. They, in contrast, felt that people shared information on social media about half the time without checking whether that information was accurate. Forty-three percent of respondents said that people shared information without checking its accuracy either ‘always’ or ‘most of the time.’ This finding epitomizes the importance of having a mechanism that can be used to check the validity of the news before the false ones propagate into the network (Strategy 8).
Another online survey was conducted, and the results were reported in Mihunov et al. (Citation2020). Unlike the previous surveys, this one focused on Twitter users who resided in Houston and surrounding communities and had requested for rescue during Harvey using Twitter. The survey recruited 195 respondents, which was a result of screening over 2,000 responses who fit into the above-mentioned criteria. Survey results show that most respondents were employed, owned homes, and had higher income and education compared with an average Houston resident. A large percentage of the respondents (87%) were satisfied with their current locations and did not consider moving away from Houston, which may hint at a faster recovery of the region. Also, Twitter users who requested for rescue were of better socioeconomic conditions, further reinforcing the notion of socioeconomic disparities in Twitter use for rescue and the need to have fairness-adjusted algorithms to correct the inequity (Shang, Sun, and Lam Citation2020; Zhou and Sun Citation2021).
Respondents in this rescue-request survey provided useful comments that could be adopted to improve the use of Twitter in emergency rescue and management. First (Strategy 9), respondents suggested that including hashtags (e.g. #hurricaneharveyrescue) in messaging could help organize responses and improve communication dramatically. From the survey, only 25% respondents used hashtags in their messages for rescue requests. Second (Strategy 10), a list of hashtags predetermined by the relevant organizations that have already been widely publicized before hurricanes (during the preparedness phase) would speed up the response and rescue time. Not knowing if the tweets reached the addressee, if the first responders would send help, and how soon would they come for the rescue were the difficulties experienced by the respondents. Likewise, from an organizational point of view, not knowing if the rescue request has been fulfilled poses another difficulty. Thus (Strategy 11), efforts to improve future rescue operations could be better directed at reducing miscommunication between all involved parties. To improve Twitter use for future emergencies, respondents found that raising awareness about Twitter accounts or hashtags that would accept requests for rescue would be most helpful. These are concrete and practical recommendations that could be acted on to improve future rescue operations.
5.3. Twitter company
Although it is within the purview of social media companies to determine how social media platform is designed and whether the data can be made available to the public, it is these companies’ best interest that their platforms are user friendly and used widely. Changing company policies in a rapidly changing social media technology and business model is common, which will affect how emergency organizations and disaster researchers use social media and their data for management and research. For example, Facebook data are generally not available to the public, whereas Twitter made 1% sample data free for the public. In 2021, Twitter released the Academic Research track product, which provided free full-archive search capability for researchers to download up to 10 million tweets per month per account. However, in February 2023, after Elon Musk took over the Twitter company, the Company’s policy of data access changed, and monthly fees will be charged for API access with ID verification (Twitter Citation2023). Twitter’s justification is that account verification and fees charging will reduce the scammers and bots. However, others view such policy change as disrupting public interest research (Hendrix Citation2023).
At the time this paper is written, it is not clear if there will be further changes to data access policies. Despite these challenges, our case studies show that some modifications of current tweet formats by the Company could make disaster responses more efficient. These algorithmic modifications include: (Strategy 12) making the platform easier for organizations to develop templates for inputting key information during emergency response or rescue. Information such as relevant hashtags, rescue addresses, number of people to be rescued, and the final status of whether the request has been fulfilled would be very useful. These are changes that are amenable. (Strategy 13) Working with governmental and non-governmental organizations to enable these changes will benefit their operations.
To combat false news, (Strategy 14) working with researchers and the communities to find the best way to debunk false news is essential. Methods such as assigning a label to tweets that are false or potentially false and including a warning of spreading could help eliminate the potential impacts of the false news in a disastrous event. At the time this paper is written, both Twitter and Facebook are actively testing a feature that the user is being warned before sharing a link to an article that they did not open. The pop-up dialogue presents a message encouraging the user to read the article before sharing. In addition, (Strategy 15) working with emergency organizations and researchers by setting up a central website to debunk false information was also a recommendation made by organizations involved in emergency management (U. S. Department of Homeland Security Citation2018).
Finally (Strategy 16), improving locational accuracy of the tweets is key to successful applications of social media data, but it is probably the most difficult challenge to overcome given the increasing concern of privacy. The locational information in social media is increasingly text-based and generalized to larger areas and greater-known toponyms. New methods including AI are being developed to improve toponym disambiguation when location information is incomplete or uncertain (de Bruijn et al. Citation2017; Nizzoli et al. Citation2020). Address standardization using natural-language processing algorithms has also been used to improve geocoding results (Kucuk Matci and Avdan Citation2018). This is an active research area. Working with the four major social media sectors, including researchers, emergency organizations, and residents, to explore ways to increase locational accuracy while protecting privacy is necessary.
5.4. Researchers
To enable social media research by a wide range of researchers with diverse perspectives, some of the technical challenges will need to be addressed. First (Strategy 17), developing user-friendly open-source software tools for social media data collection and processing is a fundamental step. The tools should be made available to the end-users (e.g. social scientists, first responders) with minimal technical details (e.g. server configurations, programming using APIs) so that they can focus on their core studies/responsibilities. The tools should be made to allow the users to select keywords and regions of interest using a graphical user interface (GUI) to start collecting social media posts in real time. One critical requirement for the tools will be to have an ability to integrate with the latest developments in natural language processing (e.g. BERT, GPT: Generative Pre-trained Transformer) for analyzing social media posts. Building a social media cyberinfrastructure that contains essential data and algorithms will help generate more research that would lead to better understanding of social media data use for disaster response and management.
Second (Strategy 18), the biased demographic representation of social media users implies that modeling and predictions made from social media data alone are prone to bias. Like many social surveys, social media data suffers a ‘selection bias.’ Different adjustment methods have been suggested, though studies have shown that even the most elaborated adjustment techniques were unable to remove most of the bias (Giorgi et al. 2021; Hinds and Joinson Citation2018; Mercer, Lau, and Kennedy Citation2018; Wang et al. Citation2019). Developing an accurate adjustment or weighting scheme to remove the representation bias will increase the reliability of findings based on social media data.
Third (Strategy19), new and cutting-edge research will need to be carried out to find ways to mitigate the bias in AI algorithms. For example, in developing an AI-fairness model for rescue requests during Hurricane Harvey, researchers could identify the fairness requirements by calculating the flood vulnerability of communities and then comparing the computed vulnerability with the empirical rescue tweet density data. The difference between the two variables can be used to adjust the parameters in AI modeling to mitigate the unfairness, leading to a model of equal probability of being rescued according to the vulnerability, not the abundance of the rescue-request tweets (Shang, Sun, and Lam Citation2020; Sun and Lam Citation2019; Zhou and Sun Citation2021). However, as stated in Gevaert et al. (Citation2021), there is still limited understanding of how the fairness issue affects AI modeling in disaster applications, and a combined data science, ethical, and social perspective must be considered to ensure the responsible use of AI in disaster research and management.
Fourth (Strategy 20), to address the challenge of false news, a holistic approach which considers multiple aspects of false news behaviors in social media is essential (Singh et al. Citation2020). These aspects include user-to-user engagement patterns, user-to-claim engagement patterns, user profile data, temporal dissemination patterns of false news events, content of the false news, and associated Twitter posts and hashtags. A deep-learning approach could be used to mine the multiple aspects to detect the likelihood of a false news event (Ruchansky, Seo, and Liu Citation2017). However, success in detecting false news by algorithms has not been high. Another approach could be to develop a ranking of trustworthiness for the news generated by various organizations to help filter false news. This approach is being carried out in a current study of Twitter use related to COVID19 (Hoque et al. Citation2022; Lee, Lam, and Curran Citation2020). Initial efforts on developing a trustworthiness ranking have been made, such as the Global Disinformation Index (GDI) (Srinivasan Citation2019), but these efforts met with lots of challenges and disagreement among organizations on many fronts. More research on this line of direction should help in establishing standards and raising awareness of the danger of false news spreading.
6. Discussion
We organized the proposed strategies according to the four sectors of the social media community, instead of aligning them with the four challenges/uses framework, so that we can place an emphasis on the roles that each sector can play. Moreover, since the different types of Twitter uses and challenges discussed in the framework are interacting closely with each other, a proposed strategy can have impacts on multiple uses and challenges. Thus, it is difficult to isolate and align the impacts of a strategy with a single challenge. For example, Strategy 2, which is to raise awareness of official accounts at the organization level, can also help reduce the chance of false news spread and improve communication and rescue request.
There are limitations to this study. First, we acknowledge that our proposed strategies do not necessarily cover all the potential problems related to social media use for disaster research and management, such as cybersecurity and internet connectivity, and that they are derived from limited evidence and literature. Second, the proposed strategies are designed to be actionable, low-hanging fruit-type actions that could be taken to generate big impacts. We recognize that some of the proposed strategies may have already been brought up or adopted to a certain degree by the four sectors, and if so, findings from this study could serve to reinforce the importance of those proposed actions.
Finally, we observe that of all the proposed 20 strategies, none of them directly addresses the issue of reducing social and geographical disparities of social media use. In other words, what steps should we take to improve the access of underserved communities to social media and other digital means? This is a broad societal issue that will involve social and structural changes on many levels. Reducing the digital divide is difficult but it is an important capacity of community resilience that all sectors of the social media community should aim to build and foster (e.g. Lam et al. Citation2016; Wang et al. Citation2019; Wang et al. Citation2021; Zou et al. Citation2018).
7. Conclusions
Social media platforms are effective means of communication for disaster management, and social media data are valuable for studying and understanding human responses and disaster resilience. We outlined four major contributions of social media data for disaster resilience research and management. They include serving as an effective communication platform, providing ground truth information for emergency response and rescue operations, providing information on people’s sentiments and subjective well-being, and allowing predictive modeling. However, the key challenges of social media research for disaster resilience are the easy spread of false news, social and geographical disparities of Twitter use, technical issues on processing and analyzing big and noisy data, especially regarding the locational accuracy of the tweets, and algorithm bias in AI and other types of modeling. Building on findings from previous case studies and online surveys of Twitter use during three hurricane events (Isaac, Sandy, and Harvey), we proposed 20 strategies that the four sectors of the social media community – organizations, individuals, social media companies, and researchers – could consider taking to improve Twitter use for disaster resilience research and management.
In summary, we found that organizations could utilize the mitigation phase to increase two-way communications with individuals to increase their social capital and hazard awareness and literacy. The mitigation phase is a logical time to review organizational mandates and procedures regarding social media use such as designing relevant hashtags for emergency rescue. Individuals should take the opportunity to get familiar with the resources, hashtags, or templates provided by organizations to help speed up the response and recovery. Social media companies like Twitter could make the platform easier for the user to input necessary information to enable more efficient two-way communications during disasters. All three sectors have expressed serious concerns about the spread of false or malicious news, uneven representation of the users, and privacy and confidentiality. Thus, the task for all sectors is clear, which is to find the best approach to detect false news, remove algorithm bias, reduce digital divide, build data and tools infrastructure to generate more and better studies, and establish standards and criteria to help promote scientific repeatability and reliability. Social media technologies and policies are rapidly changing, and it will remain to be a dominant social force in the future. It would be in our interests as a whole community to make the best use of them to benefit society.
Social media promotion
This paper identifies major knowledge gaps and methodological challenges of social media use in disaster resilience research and proposes 20 strategies to improve social media use to increase disaster resilience.
Acknowledgment
This research is supported by the U.S. National Science Foundation under The Interdisciplinary Behavioral and Social Science Research (IBSS) Program [Award No. 1620451] and the Rapid Response Research (RAPID) Program [Award No. 1762600]. Any opinions, findings, and conclusions or recommendations expressed in this article are those of the authors and do not necessarily reflect the views of the funding agency.
Disclosure statement
No potential conflict of interest was reported by the author(s).
Data availability statement
The authors confirm that the data supporting the findings of this study are available within the article.
Additional information
Funding
References
- Allcott, Hunt, and Matthew Gentzkow. 2017. “Social Media and Fake News in the 2016 Election.” Journal of Economic Perspectives 31 (2): 211–236. https://doi.org/10.1257/jep.31.2.211.
- Brainard, Jeffrey. 2022. “Riding the Twitter Wave.” Science 375 (6587): 1344–1347. https://doi.org/10.1126/science.abq1541.
- Chauhan, Apoorva. 2014. “Online Media Use and Adoption by Hurricane Sandy Affected Fire and Police Departments.” PhD Diss, Utah State University.
- de Bruijn, Jens A., Hans de Moel, Brenden Jongman, Jurjen Wagemaker, and Jeroen C. J. H. Aerts. 2017. “TAGGS: Grouping Tweets to Improve Global Geoparsing for Disaster Response.” Journal of Geovisualization and Spatial Analysis 2 (1): 2. https://doi.org/10.1007/s41651-017-0010-6.
- Enserink, Martin. 2022. “Inside The Vortex.” Science 375 (6587): 1332–1333. https://doi.org/10.1126/science.abq1536.
- FEMA (Federal Emergency Managment Agency). 2006. Principles of Emergency Management: Independent Study Manual. https://www.hsdl.org/?abstract&did=485174.
- Fitzpatrick, Colleen, and Dennis S. Mileti. 1991. “Motivating Public Evacuation.” International Journal of Mass Emergencies & Disasters 9 (2): 137–152. https://doi.org/10.1177/028072709100900202.
- Fortin, Jacey. 2017. “When Disaster Hits and Landlines Fail, Social Media Is a Lifeline.” The New York Times: New York, September 23, 2017. https://www.nytimes.com/2017/09/23/us/whatsapp-zello-hurricane-earthquakes.html.
- Friggeri, Adrien, Lada Adamic, Dean Eckles, and Justin Cheng. 2014. “Rumor Cascades.” Proceedings of the International AAAI Conference on Web and Social Media 8 (1): 101–110. https://doi.org/10.1609/icwsm.v8i1.14559.
- Gevaert, Caroline M., Mary Carman, Benjamin Rosman, Yola Georgiadou, and Robert Soden. 2021. “Fairness and Accountability of AI in Disaster Risk Management: Opportunities and Challenges.” Patterns 2 (11): 100363. https://doi.org/10.1016/j.patter.2021.100363.
- Giorgi, Salvatore, Veronica E. Lynn, Keshav Gupta, Farhan Ahmed, Sandra Matz, Lyle H. Ungar, and H. Andrew Schwartz. 2022. “Correcting Sociodemographic Selection Biases for Population Prediction from Social Media.” Proceedings of the International AAAI Conference on Web and Social Media 16 (1): 228–240. https://doi.org/10.1609/icwsm.v16i1.19287.
- Harrison, Sara E., and Peter A. Johnson. 2016. “Crowdsourcing the Disaster Management Cycle.” International Journal of Information Systems for Crisis Response and Management (IJISCRAM) 8 (4): 17–40. https://doi.org/10.4018/IJISCRAM.2016100102.
- Hendrix, Justin. 2023. “Twitter API Charges Set to Disrupt Public Interest Research.” Tech Policy Press, April 4, 2023. https://techpolicy.press/twitter-api-changes-set-to-disrupt-public-interest-research/.
- Hinds, Joanne, and Adam N. Joinson. 2018. “What Demographic Attributes Do Our Digital Footprints Reveal? A Systematic Review.” Plos One 13 (11): e0207112. https://doi.org/10.1371/journal.pone.0207112.
- Hoque, Misbah U., Kisung Lee, Jessica L. Beyer, Sara R. Curran, Katie S. Gonser, Nina S. N. Lam, Volodymyr V. Mihunov, and Kejin Wang. 2022. “Analyzing Tweeting Patterns and Public Engagement on Twitter During the Recognition Period of the COVID-19 Pandemic: A Study of Two U.S. States.” IEEE Access 10: 72879–72894. https://doi.org/10.1109/ACCESS.2022.3189670.
- Huang, Qunying, and Yu Xiao. 2015. “Geographic Situational Awareness: Mining Tweets for Disaster Preparedness, Emergency Response, Impact, and Recovery.” ISPRS International Journal of Geo-Information 4 (3): 1549–1568. https://doi.org/10.3390/ijgi4031549.
- Hughes, Amanda L., Lise A. A. St. Denis, Leysia Palen, and Kenneth M. Anderson. 2014. “Online Public Communications by Police & Fire Services During the 2012 Hurricane Sandy.” Proceedings of the SIGCHI Conference on Human Factors in Computing Systems, 1505–1514. https://doi.org/10.1145/2556288.2557227.
- Ilieva, Rositsa T., and Timon McPhearson. 2018. “Social-media Data for Urban Sustainability.” Nature Sustainability 1 (10): 553–565. https://doi.org/10.1038/s41893-018-0153-6.
- Jaidka, Kokil, Salvatore Giorgi, H. Andrew Schwartz, Margaret L. Kern, Lyle H. Ungar, and Johannes C. Eichstaedt. 2020. “Estimating Geographic Subjective Well-Being from Twitter: A Comparison of Dictionary and Data-Driven Language Methods.” Proceedings of the National Academy of Sciences 117 (19): 10165–10171. https://doi.org/10.1073/pnas.1906364117.
- Kankanamge, Nayomi, Tan Yigitcanlar, and Ashantha Goonetilleke. 2020. “How Engaging Are Disaster Management Related Social Media Channels? The Case of Australian State Emergency Organisations.” International Journal of Disaster Risk Reduction 48: 101571. https://doi.org/10.1016/j.ijdrr.2020.101571.
- Kankanamge, Nayomi, Tan Yigitcanlar, Ashantha Goonetilleke, and Md Kamruzzaman. 2019. “Can Volunteer Crowdsourcing Reduce Disaster Risk? A Systematic Review of the Literature.” International Journal of Disaster Risk Reduction 35: 101097. https://doi.org/10.1016/j.ijdrr.2019.101097.
- Kantarci, Burak, Kevin G. Carr, and Connor D. Pearsall. 2016. “SONATA: Social Network Assisted Trustworthiness Assurance in Smart City Crowdsensing.” International Journal of Distributed Systems and Technologies (IJDST) 7 (1): 59–78. https://doi.org/10.4018/IJDST.2016010104.
- Kirby, Ryan H. 2019. “The Use of Social Media in Emergency Management by Public Agencies and Non-Governmental Organizations: Lessons Learned From Areas Affected by Hurricanes Isaac, Sandy, and Harvey.” PhD Diss, Louisiana State University.
- Kirby, Ryan H., Margaret Reams, and Nina S.-N. Lam. 2023. “The Use of Social Media by Emergency Stakeholder Groups: Lessons Learned from Areas Affected by Hurricanes Isaac and Sandy.” Journal of Homeland Security and Emergency Management 20 (2): 133–168. https://doi.org/10.1515/jhsem-2021-0031.
- Küçük Matci, Dilek, and Uğur Avdan. 2018. “Address Standardization Using the Natural Language Process for Improving Geocoding Results.” Computers, Environment and Urban Systems 70: 1–8. https://doi.org/10.1016/j.compenvurbsys.2018.01.009.
- Lam, Nina S.-N., Margaret Reams, Kenan Li, Chi Li, and Lillian P. Mata. 2016. “Measuring Community Resilience to Coastal Hazards Along the Northern Gulf of Mexico.” Natural Hazards Review 17 (1): 04015013. https://doi.org/10.1061/(ASCE)NH.1527-6996.0000193.
- Lee, Kisung, Nina S. N. Lam, and Sara R. Curran. 2020. “RAPID: Investigating Public Health and Social Information Disparities During the COVID-19 Pandemic.” National Science Foundation. https://www.nsf.gov/awardsearch/showAward?AWD_ID=2032745.
- Li, Linna, Michael F. Goodchild, and Bo Xu. 2013. “Spatial, Temporal, and Socioeconomic Patterns in the Use of Twitter and Flickr.” Cartography and Geographic Information Science 40 (2): 61–77. https://doi.org/10.1080/15230406.2013.777139.
- Lin, Binbin, Lei Zou, Nick Duffield, Ali Mostafavi, Heng Cai, Bing Zhou, Jian Tao, Mingzheng Yang, Debayan Mandal, and Joynal Abedin. 2022. “Revealing the Linguistic and Geographical Disparities of Public Awareness to Covid-19 Outbreak Through Social Media.” International Journal of Digital Earth 15 (1): 868–889. https://doi.org/10.1080/17538947.2022.2070677.
- Lindell, Michael K., and Ronald W. Perry. 2012. “The Protective Action Decision Model: Theoretical Modifications and Additional Evidence.” Risk Analysis 32 (4): 616–632. https://doi.org/10.1111/j.1539-6924.2011.01647.x.
- Lindsay, Bruce R. 2011. “Social Media and Disasters: Current Uses, Future Options, and Policy Considerations.” Congressional Research Service Washington, DC.
- Liu, Sophia B. 2014. “Crisis Crowdsourcing Framework: Designing Strategic Configurations of Crowdsourcing for the Emergency Management Domain.” Computer Supported Cooperative Work (CSCW) 23 (4): 389–443. https://doi.org/10.1007/s10606-014-9204-3.
- Maron, Dina Fine. 2013. “How Social Media Is Changing Disaster Response.” Scientific American, June 7, 2013. https://www.scientificamerican.com/article/how-social-media-is-changing-disaster-response/.
- Mercer, Andrew, Arnold Lau, and Countney Kennedy. 2018. “For Weighting Online Opt-In Samples, What Matters Most?” Pew Research Center, January 26, 2018. https://www.pewresearch.org/methods/2018/01/26/for-weighting-online-opt-in-samples-what-matters-most/.
- Mihunov, Volodymyr V., Navid H. Jafari, Kejin Wang, Nina S. N. Lam, and Dylan Govender. 2022. “Disaster Impacts Surveillance from Social Media with Topic Modeling and Feature Extraction: Case of Hurricane Harvey.” International Journal of Disaster Risk Science 13 (5): 729–742. https://doi.org/10.1007/s13753-022-00442-1.
- Mihunov, Volodymyr V., Nina S. N. Lam, Lei Zou, Zheye Wang, and Kejin Wang. 2020. “Use of Twitter in Disaster Rescue: Lessons Learned from Hurricane Harvey.” International Journal of Digital Earth 13 (12): 1454–1466. https://doi.org/10.1080/17538947.2020.1729879.
- Mihunov, Volodymyr V., Kejin Wang, Zheye Wang, Nina S. N. Lam, and Mingxuan Sun. 2023. “Social Media and Volunteer Rescue Requests Prediction with Random Forest and Algorithm Bias Detection: A Case of Hurricane Harvey.” Environmental Research Communications 5 (6): 065013. https://doi.org/10.1088/2515-7620/acde35.
- Mileti, Dennis S., and Colleen Fitzpatrick. 1992. “The Causal Sequence of Risk Communication in the Parkfield Earthquake Prediction Experiment.” Risk Analysis 12 (3): 393–400. https://doi.org/10.1111/j.1539-6924.1992.tb00691.x.
- Nizzoli, Leonardo, Marco Avvenuti, Maurizio Tesconi, and Stefano Cresci. 2020. “Geo-Semantic-Parsing: AI-powered Geoparsing by Traversing Semantic Knowledge Graphs.” Decision Support Systems 136: 113346. https://doi.org/10.1016/j.dss.2020.113346.
- Rajdev, Kyumin Le, and Kisung Lee. 2015. “Fake and Spam Messages: Detecting Misinformation During Natural Disasters on Social Media.” Proceedings of the 2015 IEEE/WIC/ACM International Conference on Web Intelligence and Intelligent Agent Technology (WI-IAT) 1: 17–20. https://doi.org/10.1109/WI-IAT.2015.102.
- Ramteke, Jyoti, Samarth Shah, Darshan Godhia, and Aadil Shaikh. 2016. “Election Result Prediction Using Twitter Sentiment Analysis.” Proceedings of the 2016 International Conference on Inventive Computation Technologies (ICICT) 1: 1–5. https://doi.org/10.1109/INVENTIVE.2016.7823280.
- Reuter, Christian, and Marc-André Kaufhold. 2018. “Fifteen Years of Social Media in Emergencies: A Retrospective Review and Future Directions for Crisis Informatics.” Journal of Contingencies and Crisis Management 26 (1): 41–57. https://doi.org/10.1111/1468-5973.12196.
- Reuter, Christian, Thomas Ludwig, Marc-André Kaufhold, and Thomas Spielhofer. 2016. “Emergency Services׳ Attitudes Towards Social Media: A Quantitative and Qualitative Survey Across Europe.” International Journal of Human-Computer Studies 95: 96–111. https://doi.org/10.1016/j.ijhcs.2016.03.005.
- Reuter, Christian, and Thomas Spielhofer. 2017. “Towards Social Resilience: A Quantitative and Qualitative Survey on Citizens’ Perception of Social Media in Emergencies in Europe.” Technological Forecasting and Social Change 121: 168–180. https://doi.org/10.1016/j.techfore.2016.07.038.
- Reva, Schwartz, Vassilev Apostol, K. Greene Kristen, Perine Lori, Burt Andrew, and Hall Patrick. 2022. “Towards a Standard for Identifying and Managing Bias in Artificial Intelligence.” NIST Special Publication, https://doi.org/10.6028/NIST.SP.1270.
- Rhodan, Maya. 2017. “Hurricane Harvey: The U.S.'s First Social Media Storm.” Time Magazine, August 30, 2017. https://time.com/4921961/hurricane-harvey-twitter-facebook-social-media/.
- Ruchansky, Natali, Sungyong Seo, and Yan Liu. 2017. “CSI: A Hybrid Deep Model for Fake News Detection.” Proceedings of the 2017 ACM on Conference on Information and Knowledge Management, 797–806. https://doi.org/10.1145/3132847.3132877.
- Sagun, Aysu, Dino Bouchlaghem, and Chimay J. Anumba. 2009. “A Scenario-Based Study on Information Flow and Collaboration Patterns in Disaster Management.” Disasters 33 (2): 214–238. https://doi.org/10.1111/j.1467-7717.2008.01071.x.
- Shams, Shayan, Sayan Goswami, and Kisung Lee. 2019. “Deep Learning-Based Spatial Analytics for Disaster-Related Tweets: An Experimental Study.” Proceedings of the 2019 20th IEEE International Conference on Mobile Data Management (MDM), 337–342. https://doi.org/10.1109/MDM.2019.00-40.
- Shang, Jin, Mingxuan Sun, and Nina S.N. Lam. 2020. “List-Wise Fairness Criterion for Point Processes.” Proceedings of the 26th ACM SIGKDD International Conference on Knowledge Discovery & Data Mining, 1948–1958. https://doi.org/10.1145/3394486.3403246.
- Singh, Dipak, Shayan Shams, Joohyun Kim, Seung-jong Park, and Seungwon Yang. 2020. “Fighting for Information Credibility: An End-to-End Framework to Identify Fake News During Natural Disasters.” ISCRAM 2020 Conference Proceedings – 17th International Conference on Information Systems for Crisis Response and Management, 90–99. https://www.lsu.edu/chse/slis/about_us/bios/yang.php.
- Sorensen, John H. 2000. “Hazard Warning Systems: Review of 20 Years of Progress.” Natural Hazards Review 1 (2): 119–125. https://doi.org/10.1061/(ASCE)1527-6988(2000)1:2(119).
- Sorensen, John H., and Barbara Vogt Sorensen. 2007. “Community Processes: Warning and Evacuation.” In Handbook of Disaster Research, edited by Havidán Rodríguez, Enrico L. Quarantelli, and Russell R. Dynes, 183–199. New York, NY: Springer New York.
- Srinivasan, Santhosh. 2019. “Rating Disinformation Risk: The GDI Methodology.” Accessed December 1, 2019. https://www.disinformationindex.org/research/2019-12-1-rating-disinformation-risk-the-gdi-methodology/.
- Su, Yee San, Clarence III Wardell, Zoë Thorkildsen, and Monica Giovachino. 2013. “Social Media in the Emergency Management Field 2012 Survey Results”.
- Sun, Mingxuan, and Nina Lam. 2019. “AI-DCL: EAGER: Fairness-aware Informatics System for Enhancing Disaster Resilience.” National Science Foundation. https://www.nsf.gov/awardsearch/showAward?AWD_ID=1927513.
- Sutton, Jeannette, C. Ben Gibson, Nolan Edward Phillips, Emma S. Spiro, Cedar League, Britta Johnson, Sean M. Fitzhugh, and Carter T. Butts. 2015a. “A Cross-Hazard Analysis of Terse Message Retransmission on Twitter.” Proceedings of the National Academy of Sciences 112 (48): 14793–14798. https://doi.org/10.1073/pnas.1508916112.
- Sutton, Jeannette, Cedar League, Timothy L. Sellnow, and Deanna D. Sellnow. 2015b. “Terse Messaging and Public Health in the Midst of Natural Disasters: The Case of the Boulder Floods.” Health Communication 30 (2): 135–143. https://doi.org/10.1080/10410236.2014.974124.
- Sutton, Jeannette, Emma Spiro, Carter Butts, Sean Fitzhugh, Britta Johnson, and Matt Greczek. 2013. “Tweeting the Spill: Online Informal Communications, Social Networks, and Conversational Microstructures During the Deepwater Horizon Oil Spill.” International Journal of Information Systems for Crisis Response and Management (IJISCRAM) 5 (1): 58–76. https://doi.org/10.4018/jiscrm.2013010104.
- Tapia, Andrea H., and Kathleen Moore. 2014. “Good Enough is Good Enough: Overcoming Disaster Response Organizations’ Slow Social Media Data Adoption.” Computer Supported Cooperative Work (CSCW) 23 (4-6): 483–512. https://doi.org/10.1007/s10606-014-9206-1.
- Twitter. 2023. “Twitter Developer Platform.” Accessed April 4, 2023. https://developer.twitter.com/en/docs/twitter-api.
- U. S. Department of Homeland Security. 2018. “Countering False Information on Social Media in Disasters and Emergencies: Social Media Working Group for Emergency Services and Disaster Management.” Accessed June 27, 2023. https://www.dhs.gov/publication/st-frg-countering-false-information-social-media-disasters-and-emergencies.
- Vincent, James. 2020. “What a Machine Learning Tool That Turns Obama White Can (and Can't) Tell Us About AI Bias.” Theverge, Jun 23, 2020. https://www.theverge.com/21298762/face-depixelizer-ai-machine-learning-tool-pulse-stylegan-obama-bias.
- Vosoughi, Soroush, Deb Roy, and Sinan Aral. 2018. “The Spread of True and False News Online.” Science 359 (6380): 1146–1151. https://doi.org/10.1126/science.aap9559.
- Wang, Zijian, Scott Hale, David Ifeoluwa Adelani, Przemyslaw Grabowicz, Timo Hartman, Fabian Flöck, and David Jurgens. 2019. “Demographic Inference and Representative Population Estimates from Multilingual Social Media Data.” Proceedings of the World Wide Web Conference, 2056–2067. https://doi.org/10.1145/3308558.3313684.
- Wang, Kejin, Nina S. N. Lam, and Volodymyr Mihunov. 2023. “Correlating Twitter Use with Disaster Resilience at Two Spatial Scales: A Case Study of Hurricane Sandy.” Annals of GIS 29 (1): 1–20. https://doi.org/10.1080/19475683.2023.2165545.
- Wang, Zheye, Nina S. N. Lam, Nick Obradovich, and Xinyue Ye. 2019. “Are Vulnerable Communities Digitally Left Behind in Social Responses to Natural Disasters? Evidence from Hurricane Sandy with Twitter Data.” Applied Geography 108: 1–8. https://doi.org/10.1016/j.apgeog.2019.05.001.
- Wang, Zheye, Nina S. N. Lam, Mingxuan Sun, Xiao Huang, Jin Shang, Lei Zou, Yue Wu, and Volodymyr V. Mihunov. 2022. “A Machine Learning Approach for Detecting Rescue Requests from Social Media.” ISPRS International Journal of Geo-Information 11 (11): 570. https://doi.org/10.3390/ijgi11110570.
- Wang, Kejin, Nina S. N. Lam, Lei Zou, and Volodymyr Mihunov. 2021. “Twitter Use in Hurricane Isaac and Its Implications for Disaster Resilience.” ISPRS International Journal of Geo-Information 10 (3): 116. https://doi.org/10.3390/ijgi10030116.
- Wang, Zheye, and Xinyue Ye. 2018. “Social Media Analytics for Natural Disaster Management.” International Journal of Geographical Information Science 32 (1): 49–72. https://doi.org/10.1080/13658816.2017.1367003.
- Wong, Kai On, Faith Davis, Osmar Zaïane, and Yutaka Yasui. 2016. “Sentiment Analysis of Breast Cancer Screening in the United States Using Twitter.” Proceedings of the 8th International Conference on Knowledge Discovery and Information Retrieval, 265–274. https://doi.org/10.5220/0006047102650274.
- Yang, Seungwon, and Boryung Ju. 2021. “Library Support for Emergency Management During the Time of Natural Disasters: Through the Lens of Public Library Twitter Data.” Library & Information Science Research 43 (1): 101072. https://doi.org/10.1016/j.lisr.2021.101072.
- Zhou, Zihan, and Mingxuan Sun. 2021. “Multivariate Hawkes Processes for Incomplete Biased Data.” Proceedings of the 2021 IEEE International Conference on Big Data (Big Data), 968–977. https://doi.org/10.1109/BigData52589.2021.9672043.
- Zhou, Bing, Lei Zou, Ali Mostafavi, Binbin Lin, Mingzheng Yang, Nasir Gharaibeh, Heng Cai, Joynal Abedin, and Debayan Mandal. 2022. “VictimFinder: Harvesting Rescue Requests in Disaster Response from Social Media with BERT.” Computers, Environment and Urban Systems 95: 101824. https://doi.org/10.1016/j.compenvurbsys.2022.101824.
- Zou, Lei, Nina S. N. Lam, and Heng Cai. 2019a. “A Framework for Analyzing and Visualizing Twitter Use Patterns During Natural Hazards.” U. S. National Report (U. S. National Committee for the International Cartographic Association).
- Zou, Lei, Nina S. N. Lam, Heng Cai, and Yi Qiang. 2018. “Mining Twitter Data for Improved Understanding of Disaster Resilience.” Annals of the American Association of Geographers 108 (5): 1422–1441. https://doi.org/10.1080/24694452.2017.1421897.
- Zou, Lei, Nina S. N. Lam, Shayan Shams, Heng Cai, Michelle A. Meyer, Seungwon Yang, Kisung Lee, Seung-Jong Park, and Margaret A. Reams. 2019b. “Social and Geographical Disparities in Twitter Use During Hurricane Harvey.” International Journal of Digital Earth 12 (11): 1300–1318. https://doi.org/10.1080/17538947.2018.1545878.
- Zou, Lei, Danqing Liao, Nina S. N. Lam, Michelle A. Meyer, Nasir G. Gharaibeh, Heng Cai, Bing Zhou, and Dongying Li. 2023. “Social Media for Emergency Rescue: An Analysis of Rescue Requests on Twitter During Hurricane Harvey.” International Journal of Disaster Risk Reduction 85: 103513. https://doi.org/10.1016/j.ijdrr.2022.103513.