ABSTRACT
Chatbots can bring innovation in online assistance and communication with customers. Due to the growth of e-commerce, fashion brands have been adopting chatbots to provide personalised consumer experiences. Research in the area of chatbots for fashion e-commerce has addressed technological advancements and consumer behaviour, but little has been done on analysing chatbot features through a holistic point of view. The aim of this paper is to offer an interdisciplinary review through a comprehensive categorisation of recent studies on the theme and inform future research in the area. To achieve that, a theme-based literature review was carried out through the analysis of specialised research. The collected work was categorised addressing both computational and non-computational perspectives. The findings show that Deep Learning, recommendation systems, audio recognition and integration of chatbots with other fashion applications are a few design opportunities to be applied in both research and practice.
1. Introduction
Boosted by the COVID-19 pandemic, the fashion industry has been forced to speed up its digital transformation, bringing traditional offline services online. In the UK, 77% of retail businesses are employing AI in e-commerce through the use of chatbots (Statista, Citation2019).
Studies involving AI and fashion as a discipline have looked at factors that determine consumer acceptance (Rese, Ganster, & Baier, Citation2020; Sanny, Susastra, Roberts, & Yusramdaleni, Citation2020), forecasting and production management (Sun & Zhang, Citation2018), smart textiles (Roh, Chi, & Kang, Citation2010), trend analysis of colour palettes (Lai & Westland, Citation2020), accuracy of image labelling through machine learning (ML) (Park & Choi, Citation2020) and ‘conversational agents’, also known as ‘chatbots’ (Grewal, Roggeveen, & Nordfält, Citation2017).
Chatbots can provide personalised shopping experiences across physical and online channels (Grewal et al., Citation2017) and promote consumer wellbeing (Skjuve, Følstad, Fostervold, & Brandtzaeg, Citation2021). However, the main challenge remains on the complexity of human language and the chatbot’s effectiveness within this context (Pantano & Pizzi, Citation2020).
Chatbots may be viewed as Natural User Interfaces (Tan & Liew, Citation2020) for users to interact with a computer more intuitively through natural language, easing acceptance of e-commerce, especially for fashion items. Thus, chatbots may be seen as an essential, relevant interface element for many fashion e-commerce tasks such as providing recommendations, exploring and searching huge catalogues, complementing virtual fitting room’s features, and delivering (post-sale) customer services ().
When it comes to designing and adopting chatbots within the consumer journey, Pantano and Pizzi (Citation2020) inform that: retailers might want to choose already designed chatbot platforms or to design their own; from a technological point of view, conversational agents are designed to mimic natural language; however, analytical skills to learn from consumer data are still required; and, social media (e.g. instant messengers such as Facebook Messenger) and voice-based AI (utilising audio recognition) such as Siri and Alexa, are the most used platforms. From a computational perspective, there is a trend to improve the responsiveness of the chatbot and patents were mostly designed to provide new algorithms related to Natural Language Processing (NLP), digital communication and consumer management.
Since retailers are willing to opt for designing their own chatbot, it is crucial that managers and designers understand the current technological barriers and opportunities. Given this context, the aim of this paper is to provide design and research opportunities for future fashion chatbots applications by answering the following research questions:
RQ1: Which are the current proposed chatbots design approaches for e-commerce in general, and particularly for fashion applications?
RQ2: How could research on e-commerce chatbots be categorised in an integrated manner?
RQ3: What are the research opportunities of chatbots design to deal with the specificities of fashion e-commerce applications?
The main contributions of this study are: (i) the proposal of an interdisciplinary categorisation with an integrated view of the theme of ‘chatbots in fashion e-commerce’ that can serve as support for guiding professionals and researchers, from academy to industry, interested in understanding such kind of agents; (ii) the provision of a synthesised basis for future reference and research on the theme.
2. Methodology and research design
The theme-based literature review was carried out according to a 3-phase methodology we interactively adjusted from that by Okoli (Citation2015) for simplicity and fidelity to actual procedures for the task at hand. Phases are not necessarily sequential: one may return to a previous phase after (partial) execution of a next phase – e.g. the review results provided a base to categorise chatbot studies and to enhance details of the review’s design and execution and associated analysis (see ).
2.1. Search and selection strategies
To answer the RQs, the search string
(Chatbot OR Dialog System OR Conversational Agent OR Virtual Assistant OR Digital Assistant)
AND
((E-comm* OR Ecomm* OR Retail) OR (Fashion OR Garment OR Apparel OR Clothing OR Cosmetics OR Makeup OR Make-up))
was conducted to collect papers published from 2011 to 2021 on the theme of interest from the following databases: ACM Digital Library, Emerald, Google Scholar, IEEE Xplore Digital Library, Mendeley (Elsevier), Microsoft Academic, SagePub, ScienceDirect, Scopus, Springer, Taylor and Francis Online, IBM’s TechDocs, and Wiley Online Library. This covers 10 years of research in this field (preliminary searches on some of the databases revealed no study on the theme prior to 2014 – see ). To avoid limitations, we adopted a second search string that is not restricted to ‘e-commerce’ AND ‘fashion’ only. Variations for ‘fashion’ were also included such as apparel, garment, clothing, cosmetics, and makeup. As for ‘chatbots’, we utilised dialog system, conversational agent, virtual assistant and digital assistant as variations. A study was excluded if it was: i) written in a language other than English; ii) not available online for download; and iii) addressing the theme of chatbots but not for digital retail purposes. Three types of work were included: i) journal papers, ii) conference papers, and iii) book chapters.
2.2. Categorisation
A first distinction of chatbots’ studies is along the lines of computational aspects (i.e. aspects related to the area of Computer Science or Information Technologies, such as the use of NLP) and non-computational aspects (i.e. all other aspects such as studying consumer acceptance). This first distinction appears in Jurafsky and Martin (Citation2020) and Diederich, Brendel, and Kolbe (Citation2019). We expand on these earlier categorisations as illustrated in .
Computational papers have different categorisations. Here we focus on the high-level categories ().
Table 1. Computational main categories.
Chatbots as conversational recommender systems take a more sophisticated approach, offering a richer set of interactions that help improve preference elicitation and interact with users through natural language (NL). As such, chatbots can provide mechanisms to capture contextual information, as what has been intended by the so-called context-aware recommender systems. In the case of chatbots in fashion e-commerce, other features related to online sales could be required - e,g,, those that engage and persuade customers to buy a product, eventually involving negotiation dialogues, playing the role of a salesperson conversing with a customer (Jusoh, Citation2018). This kind of system deals with the main challenges of natural-language-based interaction, such as NL understanding, information extraction, and NL generation, which can be specific for the fashion domain.
3. Results and discussion
After applying the proposed methodology, 76 out of 5959 papers were selected: 46 describing research on chatbot computational aspects; and 30 being surveys about the state of the art or the user experience using chatbots. Firstly, it is worth noting the growth of research on the theme of chatbots for (fashion) e-commerce since 2014 (see ). Such increasing interest may be explained by the advent of DL and the subsequent interest to comprehend non-computational aspects of chatbots.
Figure 4. Evolution of chatbots for e-commerce research from 2014 to 2020 (2021 numbers omitted because search ended 2021Q1).
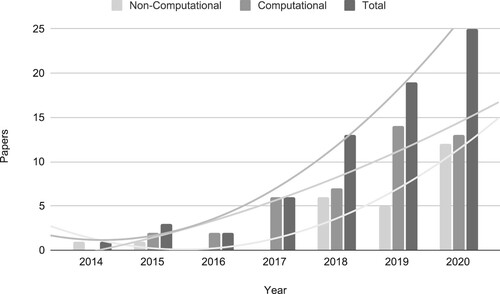
Papers were classified according to the categorisations proposed in Section 2.2 and the results, together with their references, appear in Tables X1 and X2 in an external public repository.Footnote1
3.1. Computational studies
3.1.1. Domain, language and implementation details
Most research on chatbot computational aspects had English as their primary language (76.3%), followed by papers on Indonesian chatbots (6.8%) and other languages like Chinese and Bangla. However, the resulting papers were mostly not fashion-specific (87.7%). Contrastingly, a few papers like Liao, Zhou, Ma, Hong, and Chua (Citation2018) and Vaccaro, Agarwalla, Shivakumar, and Kumar (Citation2018) were fashion-specific. It is also worth mentioning that, while non-computational research mainly employed a diversity of ready-to-use chatbot tools like Amazon Alexa, computational papers usually focus on chatbot development using a specific programming language.
3.1.2. Media
Most recent papers already consider natural language text as the input method (79.4%), except for Aarthi (Citation2020), Pricilla, Lestari, and Dharma (Citation2018) and Wintersberger, Klotz, and Riener (Citation2020) which allow the user to choose from a fixed list of options in specific situations. A few studies (15.1%) are voice-based, and others employ avatars to enhance users’ confidence and perception of the system (Eisman, Navarro, & Castro, 2016).
3.1.3. Dialog control
A turn is each single contribution that composes a dialog (Jurafsky & Martin, Citation2020). Reactive agents respond to user interactions, thus solely exploiting information explicitly provided by the user’s initiative. Conversely, proactive chatbots employ an engagement strategy to interact and influence users, making use of predictions about users’ demands.
Employed dialog turns were mostly reactive, except for Aarthi (Citation2020) and Liu, Jiang, Xiong, Yang, and Ye (Citation2020) systems, which could take control of the dialog flow. The dialog control options in were well-explored. Neural-based controls seem to be a trending research direction. And, the majority are made to solve a specific task, such as answering questions about product characteristics or mediating a purchase. Most papers aimed at solving retail tasks with a minority addressing ‘chit-chat’ chatbots. This aspect could be potentially explored in fashion-specific chatbot research.
To aid users in solving a specific task, chatbots must have a dialog policy, which is responsible for deciding which action the system should take in the next iteration. Although many distinct approaches have been proposed, we identified three main subcategories in the literature:
Predict the user’s intent, and then choose a specific answer in a finite set of predefined responses;
Analyse the similarity between the user’s questions and questions in a dataset, and choose an answer accordingly; and
Sequence-to-sequence DL approaches decode the user intent first, and synthesise a response.
3.1.4. Input processing and natural language understanding
The investigated dialog policies were based on variations of DL architectures such as Recurrent Neural Networks (RNN) and Convolutional Neural Networks (CNN), as shown in . Interest in DL picked up starting in 2017. Nevertheless, classical ML approaches and rule-based methods are still employed in state-of-the-art research.
When user interactions are enacted through buttons or multiple-choice interfaces, the expressed intents and/or other relevant information may be straightforwardly comprehended by the chatbot. However, when human natural language contained in unstructured data is allowed in the form of text (possibly extracted from speech), specific algorithms for Natural Language Understanding (NLU) must be employed. Rule-based algorithms employ handcrafted hard rules by using, for instance, the Artificial Intelligence Mark-up Language (AIML). Classical ML algorithms in general combine the extraction of handcrafted features from unstructured textual data, such as n-gram counts or the Term Frequency–Inverse Document Frequency (TF-IDF) statistical measure. DL algorithms present state-of-the-art performance by employing deep neural network architectures for sequence processing, which mainly include variations of CNN and RNN to recognise complex patterns from data.
Chatbot answers also follow approaches that resemble NLU algorithms, and thus may be categorised as rule-based, when sentences are generated from pre-written templates; or neural-based, when sentences are generated from textual training data. In the latter, variants of CNN, RNN, and Generative Adversarial Networks (GAN) are among the most popular approaches. For a comprehensive introduction to NLP algorithms, we refer the reader to Jurafsky and Martin (Citation2020).
3.1.5. Chatbot answers
Algorithms to generate or to choose predefined chatbot answers have still been employing mostly rule-based approaches, in which answers are selected from a predefined set. Nonetheless, neural-based methods exploiting neural architectures such as RNN, CNN, and GAN have already been proposed, as shown in and . In particular, IR and RNN methods prevail with over 93.1% of the selected papers addressing them ().
3.1.6. Validation
Regarding experiments to measure chatbots’ sentence generation effectiveness, the literature brings mainly two approaches: similarity metrics to compare a chatbot’s answer to that of a human, and/or expert evaluation (human judgment). To quantify the quality of a sentence generated by chatbots, Nie, Wang, Hong, Wang, and Tian (Citation2019) and Gao et al. (Citation2019) applied the BLEU Score (Papineni, Roukos, Ward, & Zhu, Citation2002), a metric for evaluating similarity in automatic language translations based on the word's position in a sentence. Similarly, Chen, Li, Ji, Zhou, and Chen (Citation2019) proposed to use the Distinct-1/2 score (Li, Galley, Brockett, Gao, & Dolan, Citation2016) which is based on the ratios of distinct unigrams and bigrams.
Nevertheless, similarity metrics do not consider syntax and semantics aspects. Therefore, another chatbot validation approach is through the assistance of linguistic (human) experts to evaluate the meaning of the chatbot's sentences. In Nie et al. (Citation2019), Chen et al. (Citation2019) and Qiu et al. (Citation2017), experts judged whether a response fits the question context considering fluency, relevance, informativeness, semantic consistency, and syntax precision. Considering the entire process, Nazir, Khan, Ahmed, Jami, & Wasi, Citation2019 followed Turing’s Loebner principle (Mauldin, Citation1994), where the experts interact with the chatbot for ten minutes to evaluate the chatbot based on context orientation, dynamism, and grammar structure.
3.1.7. Fashion domain-specific results
Multimodal chatbots have been proposed to integrate visual media with textual chatbot interfaces. Liao et al. (Citation2018) proposed a multimodal domain knowledge enriched fashion chatbot that understands the semantics of product image and modifies the attributes during back-end retrieval, offers matching suggestions, and generates responses with different modalities. De Carolis, De Gemmis, and Lops (Citation2015), (Citation2017) exploit multimodality to consider extra-rational consumer factors such as attitudes, emotions, likes and dislikes, to provide refined recommendations accordingly.
Virtual assistants can also be made to mimic a salesperson (Morotti, Donatiello, & Marfia, Citation2020). Sapna et al. (Citation2019) researched chatbots and ML methods to replicate the offline shopping experience.
Fashion specific knowledge is another non-general chatbot aspect for future research to close a large existing gap. The ontology-driven chatbot model based on the semantic web of Nazir et al. (Citation2019) illustrates results that can be expected.
3.2. Non-computational studies
Non-computational studies comprised 20 categories, including consumer trust, acceptance, satisfaction and experience. We also identified that chatbot acceptance has been assessed through models such as the Technology Acceptance Model (TAM) and/or the Use & Gratification Theory (U&G), mainly focusing on perceived usefulness, ease of use, and enjoyment (Kasilingam, Citation2020; Rese et al., Citation2020). Besides enjoyment and utilitarian factors (Rese et al., Citation2020), chatbot acceptance also relies on social presence common in human-human interactions (Chaves & Gerosa, Citation2020). Chatbot acceptance is also influenced by privacy concerns and might also vary across different demographics (Rese et al., Citation2020). Anthropomorphism (Go & Sundar, Citation2019) and privacy (Cheng & Jiang, Citation2020) were two factors that emerged from the review that show an impact on chatbot trustworthiness.
3.2.1. Fashion domain-specific results
To circumvent scarcity of training datasets for the fashion-domain, Sapna et al. (Citation2019) combined a Recommender System (RS) to a Fashion-Knowledgeable Component (FKC) into a chatbot (‘Athena’). Athena’s RS uses the product inventory of the e-commerce site while its FKC uses fashion information collected from social media, models’ photographs and stylists’ curation of fashion items. Athena’s recommendations are made from inventory-style associations and are thus, impersonal. The fashion database needs to be (frequently) aligned to inventory, however.
We have identified a trend towards the use of chatbots as co-creators of value during purchase through different roles specific to fashion (e.g. salesperson or stylist). For example, in-person fashion stylists were implemented to elicit chatbot requirements that facilitate building trust and dealing with uncertainty in e-commerce (Vaccaro et al., Citation2018). Rese et al. (Citation2020) have also found consumer gender differences in fashion e-commerce, indicating a higher use of chatbots by females.
4. Research directions
The main research gaps and needs the literature review unearthed and that serve to base suggestions of chatbot research directions in general and for the fashion domain in particular are presented here along the computational and non-computational perspectives
4.1. Computational perspective
Research for innovatively applying chatbots to e-commerce settings in general will move forward in a three-pronged way:
Evolution of DL approaches for sales assistance: as revealed in , DL approaches have emerged and become the trending research direction for chatbot tasks related to NLP;
Availability of databases to enhance DL training: DL algorithms strongly rely on huge datasets for training. Our literature review points to scarcity of public datasets for chatbots training. Alternatively, Li, Li, and Ji (Citation2021) used a dataset of conversations between customers and customer service; Chen et al. (Citation2019), a dataset with user reviews from e-commerce; and Yu et al. (Citation2017), a generic question pair dataset. The publication of such datasets, for several domains not just fashion, is a promising research direction, including how to deal with data privacy; and,
Investigation of audio-enabled chatbots in Natural User Interfaces (NUIs): using audio cues to evaluate user experience of NUIs is still limited. Prajwal, Mamatha, Ravi, Manoj, and Joisa (Citation2019) and Palma, Seeger, and Heinzl (Citation2020) consider voice-only chatbots; and De Carolis et al. (Citation2015), Eisman et al. (Citation2016) and Tan and Liew (Citation2020) besides voice, have an avatar. Most of these studies convert speech to text for the chatbot, except for De Carolis et al. (Citation2015, Citation2017) that uses audio input directly to enable recognition of voice intonation and prosody to better determine users’ intent by reducing loss of information on cues due to voice-text conversion.
4.1.1. Fashion-domain specific computational research opportunities
Four major opportunities may be highlighted:
More encompassing, real-life, professional-grade datasets on fashion items are needed if fashion chatbots are to be more extensively trained and trusted: given that no large public dataset is readily available, Nazir et al. (Citation2019) alternatively collected data manually from clothing brands websites and from Facebook posts and comments. Others like Liao et al. (Citation2018) proposed to transfer knowledge from richer domains.
Applying chatbots to fashion e-commerce specific needs such as multimedia conversations (e.g. text, voice and images): Pantano, Passavanti, Priporas, and Verteramo (Citation2020) also revealed a lack of innovative technologies in the fashion luxury industry. De Carolis et al. (Citation2015) recommended new clothes based on users’ visual cues, Sapna et al. (Citation2019) make the chatbot ask users their preferences and Liao et al. (Citation2018) proposed a multimodal chatbot to gather user’s visual and textual clothing needs. Future work could explore chatbot’s retrieval of users’ short- and long-term preferences to better recommend fashion products.
Integration of chatbots to other fashion applications in different points within the consumer journey: since chatbots can provide personalised information for consumers across their journey (Vaccaro et al., Citation2018), research that explores such integration, e.g. virtually trying a recommended item on, shows potential. Another opportunity is the integration with Augmented Reality (AR) – e.g. in Virtual Fitting Room (VFR) applications. Moriuchi, Landers, Colton, and Hair (Citation2020) compare e-commerce apps that use chatbots and those which use AR, but they do not propose integration of both to serve the fashion domain.
4.2. Non-computational perspective
Future research on non-computational aspects of chatbots for e-commerce that can be applied in the fashion domain appears more promising in the following 4 areas:
Chatbot acceptance and design for different demographics: Rese et al. (Citation2020) found that females had a negative attitude towards chatbots due to the technology immaturity and privacy issues. Models that measure consumer acceptance should be addressed (e.g. TAM and U&G, mentioned earlier) in future research for chatbot contexts, particularly when considering differences in Womenswear and Menswear categories.
Consumer autonomy and identity in chatbot consumer experience:consumer autonomy (Ameen, Hosany, & Tarhini, Citation2021) is related to the perceived sense of control that consumers have over the interaction with chatbots and it can be attached to motivational factors (e.g. Self-Determination Theory). Future studies might address the role that consumer autonomy and identity play in consumer trust and acceptance, for example, by measuring chatbots’ design approaches that can trigger these states.
Chatbot design, consumer trust and privacy: since consumers might see chatbots with negative eyes if there are privacy concerns, consumer trust is another factor that can be explored further. Aspects such as transparent advice (Vaccaro et al., Citation2018) and problem-solving (Chung, Ko, Joung, & Kim, Citation2020), could be investigated in future research, addressing the role that design plays in this context and if other factors (e.g. social elements, cultural values, self-identity) influence consumers’ trust on chatbots.
Perceived enjoyment, usability, and usefulness: perceived enjoyment and the utilitarian nature of the interaction (e.g. whether useful or not) also influence consumer acceptance. User experience and usability were also factors highlighted by this review. Further studies that analyse the influence of these elements in chatbot design for e-commerce are needed. Other aspects that might be added into this area is the influence of playfulness and gamification in perceived enjoyment of chatbots.
4.2.1. Fashion-domain specific non-computational research opportunities
Promising fashion chatbot research that is essentially non-computational and that can be inferred from the review’s results:
Culture and gender-aware chatbots: fashion retail differs across cultures and individual fit (womenswear vs. menswear), chatbots need to be tailored accordingly. Inclusive studies such as cultural and gender-related research in this field are in order.
Multiuser chatting: Merrilees and Miller (Citation2019) observed that traditional shopping with a companion influences the consumer experience. Alone consumers tend to be more price sensitive. Future studies may explore the way fashion consumers seek for advice from chatbots that could be experimented with by adjusting social factors (e.g. including a friend in the conversation), evaluating the impact of these factors on user acceptance levels, and bring said factors into the dialog when indicators (rejected number of recommendations, say) cross thresholds.
Designbots: Fashion chatbots may be made to behave as a fashion designer, providing a platform to support co-creation of value (Colombi, Kim, & Wyatt, Citation2018). Conversational platforms can provide insights for brands to recognise consumer value (Copulsky, Citation2019), which means that future research in this area can also enhance the consumer experience.
Persuasion: e-commerce chatbots should be able to function as a persuasive salesperson (Jusoh, Citation2018). Building persuasion capabilities into fashion chatbots, given the scarcity of real-life training datasets, will require research to elicit expertise and tactics from fashion offline stylists and sales experts.
Replicating other offline fashion experiences: Research that mines insights from consumer-stylists’ conversations, similarly to the work by Vaccaro et al. (Citation2018), could be also useful to other parts of the fashion supply chain - e.g. design and marketing of new fashion collections.
5. Conclusion
This paper presented an interdisciplinary, comprehensive review of what has been done in the field of conversational agents for fashion and retail e-commerce. Thus, this study adds to specialised literature in the field of chatbot design for fashion e-commerce by providing a comprehensive map of chatbot approaches that can be deployed by retailers. This study also revealed that despite the increasing investment in chatbots for e-commerce in general, research and use in fashion e-commerce are still relatively modest. Thus, further research and development on this topic are still needed.
Data Availability statement
The data that support the findings of this study are openly available at https://figshare.com/s/4ec9f1d08f6a1a362b7c
Disclosure statement
No potential conflict of interest was reported by the author(s).
Notes
References
- The references that follow are cited in the above text. Additional references for the papers filtered from results of the searches over the selected databases are listed at https://figshare.com/s/4ec9f1d08f6a1a362b7c
- Aarthi, N. G. (2020). Chatbot for retail shop evaluation. International Journal of Computer Science and Mobile Computing, 9(3), 69–77.
- Ameen, N., Hosany, S., & Tarhini, A. (2021). Consumer interaction with cutting-edge technologies: Implications for future research. Computers in Human Behavior, 120, 106761.
- Chaves, A. P., & Gerosa, M. A. (2020). How should My Chatbot interact? A survey on social characteristics in human–chatbot interaction design. International Journal of Human–Computer Interaction, 0(0), 1–30.
- Chen, S., Li, C., Ji, F., Zhou, W., & Chen, H. (2019). Review-driven answer generation for product-related questions in E-commerce. Proceedings of WSDM ‘19. ACM, New York, NY, USA, 411–419.
- Cheng, Y., & Jiang, H. (2020). How Do AI-driven chatbots impact user experience? Examining gratifications, perceived privacy risk, satisfaction, loyalty, and continued Use. Journal of Business Economics and Management, 64(4), 592–614.
- Chung, M., Ko, E., Joung, H., & Kim, S. J. (2020). Chatbot e-service and customer satisfaction regarding luxury brands. Journal of Business Research, 117, 587–595.
- Colombi, C., Kim, P., & Wyatt, N. (2018). Fashion retailing “tech-gagement”: engagement fueled by new technology. Research Journal of Textile and Apparel, 22(4), 390–406.
- Copulsky, J. (2019). Do conversational platforms represent the next big digital marketing opportunity? Applied Marketing Analytics, 4(4), 311–316.
- De Carolis, B., De Gemmis, M., & Lops, P. (2015). A multimodal framework for recognizing emotional feedback in conversational recommender systems. ACM International Conference Proceeding series, 2015-September, 11–18.
- De Carolis, B., de Gemmis, M., Lops, P., & Palestra, G. (2017). Recognizing users feedback from non-verbal communicative acts in conversational recommender systems. Pattern Recognition Letters, 99, 87–95.
- Diederich, S., Brendel, A. B., & Kolbe, L. (2019). On conversational agents in Information Systems research: Analyzing the past to guide future work. Wirtschaftsinformatik, 85, 1550–1564.
- Eisman, E. M., Navarro, M., & Castro, J. L. (2016). A multi-agent conversational system with heterogeneous data sources access. Expert Systems With Applications, 53, 172–191.
- Gao, S., Zhao, D., Ren, Z., Yin, D., Zhao, Y., & Yan, R. (2019). Product-aware answer generation in e-commerce question-answering. WSDM, 2019, 429–437.
- Go, E., & Sundar, S. S. (2019). Humanizing chatbots: The effects of visual, identity and conversational cues on humanness perceptions. Computers in Human Behavior, 97, 304–316.
- Grewal, D., Roggeveen, A. L., & Nordfält, J. (2017). The future of retailing. Journal of Retailing, 93(1), 1–6.
- Jurafsky, D., & Martin, J. H. (2020). Speech and language processing (3rd ed. Draft). Upper Saddle River, NJ: Prentice Hall.
- Jusoh, S. (2018). Intelligent conversational agent for online sales. Proceedings of ECAI 2018, 1–4.
- Kasilingam, D. L. (2020). Understanding the attitude and intention to use smartphone chatbots for shopping. Technology in Society, 62, 101280.
- Lai, P., & Westland, S. (2020). Machine learning for colour palette extraction from fashion runway images. International Journal of Fashion design. Technology and Education, 13(3), 334–340.
- Li, J., Galley, M., Brockett, C., Gao, J., & Dolan, B. (2016). A diversity-promoting objective function for neural conversation models. Proceedings of NAACL-HLT2016, 110-119.
- Li, L., Li, C., & Ji, D. (2021). Deep context modeling for multi-turn response selection in dialogue systems. Information Processing & Management, 58(1), 102415.
- Liao, L., Zhou, Y., Ma, Y., Hong, R., & Chua, T. S. (2018). Knowledge-aware multimodal fashion chatbot. Proceedings of ACM MM 2018, 1265–1266.
- Liu, C., Jiang, J., Xiong, C., Yang, Y., & Ye, J. (2020). Towards Building an Intelligent chatbot for customer service: Learning to respond at the appropriate time. ACM SIGKDD KDD, 3377–3385.
- Marulli, F., Pota, M., & Esposito, M. (2018). A comparison of character and word embeddings in bidirectional LSTMs for POS tagging in Italian. KES-IIMSS-18, 14–23.
- Mauldin, M. L. (1994). Chatterbots, tinymuds, and the turing test: Entering the loebner prize competition. AAAI, 94, 16–21.
- Merrilees, B., & Miller, D. (2019). Companion shopping: The influence on mall brand experiences. Marketing Intelligence & Planning, 37(4), 465–478.
- Moriuchi, E., Landers, V. M., Colton, D., & Hair, N. (2020). Engagement with chatbots versus augmented reality interactive technology in e-commerce. Journal of Strategic Marketing, 0(0), 1–15.
- Morotti, E., Donatiello, L., & Marfia, G. (2020, March). Fostering fashion retail experiences through virtual reality and voice assistants. 2020 IEEE VRW (pp. 338-342). IEEE.
- Nazir, A., Khan, M. Y., Ahmed, T., Jami, S. I., & Wasi, S. (2019). A novel approach for ontology-driven information retrieving chatbot for fashion brands. International Journal of Advanced Computer Science and Applications, 10(9), 546–552.
- Nie, L., Wang, W., Hong, R., Wang, M., & Tian, Q. (2019). Multimodal dialog system: Generating responses via adaptive decoders. MM 2019, 1098–1106.
- Okoli, C. (2015). A guide to conducting a standalone systematic literature review. Communications of the Association for Information Systems, 37(1), 43.
- Palma, M. D. C. O., Seeger, A. M., & Heinzl, A. (2020). Mitigating information overload in e-commerce interactions with conversational agents. In F. D. Davis, R. Riedl, J. vom Brocke, P.-M. Léger, A. Randolph, & T. Fischer (Eds.), Information Systems and neuroscience (pp. 221–228). Cham: Springer.
- Pantano, E., Passavanti, R., Priporas, C. V., & Verteramo, S. (2020). The use of new technologies for corporate marketing communication in luxury retailing: Preliminary findings. Qualitative Market Research, 23(3), 503–521.
- Pantano, E., & Pizzi, G. (2020). Forecasting artificial intelligence on online customer assistance: Evidence from chatbot patents analysis. Journal of Retailing and Consumer Services, 55, 102096.
- Papineni, K., Roukos, S., Ward, T., & Zhu, W.-J. (2002). Bleu: A method for automatic evaluation of machine translation. Proceedings of the ACL’02, 311–318.
- Park, S., & Choi, J. Y. (2020). Malware detection in self-driving vehicles using machine learning algorithms. Journal of Advanced Transportation, 2020, 1–9.
- Paul, A., Haque Latif, A., Amin Adnan, F., & Rahman, R. M. (2019). Focused domain contextual AI chatbot framework for resource poor languages. Journal of Information and Telecommunication, 3(2), 248–269.
- Prajwal, S. V., Mamatha, G., Ravi, P., Manoj, D., & Joisa, S. K. (2019). Universal semantic web assistant based on sequence to sequence model and natural language understanding. ICACC, 2019, 110–115.
- Pricilla, C., Lestari, D. P., & Dharma, D. (2018). Designing interaction for chatbot-based conversational commerce with user-centered design. ICAICTA, 2018, 244–249.
- Qiu, M., Li, F. L., Wang, S., Gao, X., Chen, Y., Zhao, W., … Chu, W. (2017). Alime chat: A sequence to sequence and rerank based chatbot engine. ACL 2017 - (long papers), 2, 498–503.
- Rese, A., Ganster, L., & Baier, D. (2020). Chatbots in retailers’ customer communication: How to measure their acceptance? Journal of Retailing and Consumer Services, 56, 102176.
- Roh, J. S., Chi, Y. S., & Kang, T. J. (2010). Wearable textile antennas. International Journal of Fashion design. Technology and Education, 3(3), 135–153.
- Sanny, L., Susastra, A., Roberts, C., & Yusramdaleni, R. (2020). The analysis of customer satisfaction factors which influence chatbot acceptance in Indonesia. Management Science Letters, 10(6), 1225–1232.
- Sapna, C. R., Anagha, M., Vats, K., Baradia, K., Khan, T., Sarkar, S., & Roychowdhury, S. (2019). Recommendence and fashionsence online fashion advisor for offline experience. ACM International Conference Proceeding series, 256–259.
- Skjuve, M., Følstad, A., Fostervold, K. I., & Brandtzaeg, P. B. (2021). My Chatbot Companion-a study of human-chatbot relationships. International Journal of Human-Computer Studies, 149, 102601.
- Statista. (2019). Leading ten areas retailers are using Artificial Intelligence (AI) in their business in the United Kingdom (UK) as of 2019*. Retrieved from https://www.statista.com/statistics/1026052/artificial-intelligence-retailers-area-of-use-in-the-united-kingdom-uk/. Accessed April 13th, 2021
- Sun, Y., & Zhang, Y. (2018). Conversational recommender system. The 41st International ACM SIGIR ‘18. ACM, New York, NY, USA, 235–244.
- Tan, S. M., & Liew, T. W. (2020). Designing embodied Virtual agents as product specialists in a multi-product Category E-commerce: The roles of source credibility and social presence. International Journal of Human–Computer Interaction, 36(12), 1136–1149.
- Vaccaro, K., Agarwalla, T., Shivakumar, S., & Kumar, R. (2018). Designing the future of personal fashion. Conference on human factors in Computing systems - proceedings, 2018-April, 1–11.
- Wintersberger, P., Klotz, T., & Riener, A. (2020, October). Tell Me more: Transparency and time-fillers to optimize chatbots’ waiting time experience. Proceedings of NordiCHI-2020 (pp. 1-6).
- Yan, R. (2018). “Chitty-Chitty-Chat Bot”: Deep Learning for conversational AI. IJCAI, 18, 5520–5526.
- Yu, J., Qiu, M., Jiang, J., Huang, J., Song, S., Chu, W., & Chen, H. (2017). Modelling domain relationships for transfer Learning on retrieval-based question answering systems in E-commerce. Proceedings of WSDM ‘18. ACM, New York, NY, USA, 682–690.