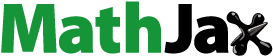
ABSTRACT
This study examines how the complementary relationships among multiple climate change adaptation strategies (improved varieties, irrigation practices and direct-seeded rice, referred to as ‘DSR’) are influenced by access to Extension services, training related to farming practices and weather-related information (referred to as ‘institutional factors’). This study hypothesizes the influence of institutional factors and the resulting effects on their joint adoption using survey data collected from 359 rice producers in Chitwan district of Nepal in 2019. The results indicate: (1) adoption of all the three climate change adaptation practices are complementary to each other; (2) all three institutional factors enhance the likelihood of the adoption of variety, access to Extension and training enhances the likelihood of the adoption of improved irrigation, and information enhances the likelihood of the adoption of DSR and (3) access to Extension and training enhance the complementarities among all three pairs of adaptation practices (variety and irrigation; variety and DSR and irrigation and DSR), while information enhances the complementarity between variety and DSR only. The impact of complementary relationships among multiple climate change adaptation strategies influenced by the institutional factors is particularly valuable for policy makers and government agencies to allocate and prioritize resources.
Introduction
Climate change, which is defined as the long-term variation of the climate that persists for an extended period of time, is one of the greatest challenges to agricultural production and food security in many regions around the world (Intergovernmental Panel on Climate Change [IPCC], Citation2007, Citation2019, Citation2021; Richardson et al., Citation2018). The frequency of the occurrence of extreme weather events such as drought, intense rainfall, floods and storms are increasing over time and their impacts on natural and human systems are observed worldwide (IPCC, Citation2019, Citation2021). For example, climate change is found to be the major determinant of the change in soil erosion (Borrelli et al., Citation2021), and imposes a risk to the livelihood and food security of rural populations, especially in developing countries (Adger et al., Citation2003; Cline, Citation2007; IPCC, Citation2019; Kabir et al., Citation2021; Wheeler & Braun, Citation2013). The vulnerability to food insecurity is projected to increase under all emission scenarios, particularly in parts of Sub-Saharan Africa and South Asia (Richardson et al., Citation2018).
Likewise, producers in Nepal are faced with warming temperatures and precipitation changes over the years. The mean annual temperature in Nepal is projected to increase by 1.8°C by 2030 and 2.8°C by 2060 (McSweeney et al., Citation2010). According to a Food and Agriculture Organization (FAO) report, while more intense rainfall is expected during the rainy season, annual rainfall in Nepal is projected to decrease in the range of 20 mm to 100 mm by 2050 (FAO, Citation2014). The report also indicates about 90% of crop losses in Nepal were associated with weather-related events. In Chitwan, one of Nepal’s rice-producing districts, between 1987 and 2000, producers were faced with extreme precipitation events (>300 mm in a day) and record extreme temperatures of 43°C for maximum and 1.6°C for minimum (Gurung & Bhandari, Citation2009). Rice producers experienced a decline in rice yield due to weather variability in the south-central plains or Terai region of Nepal (Gumma et al., Citation2011; Karna, Citation2014; Malla, Citation2008). Producers in Chitwan district have altered rice farming practices to protect yield as a response to climate change (Gahatraj et al., Citation2018)
Due to limited adaptive capacity, subsistence farming and resource-poor agricultural producers in developing countries are highly vulnerable to the impacts of climate change (Kurukulasuriya & Rosenthal, Citation2013; Morton, Citation2007). Climate change adaptation, which refers to adjustment in natural, socio-economic, or human systems in response to actual or expected climatic stimuli or their effects (IPCC, Citation2007), is one of the key responses to moderate harm or exploit beneficial opportunities (Adger et al., Citation2003). In particular, developing and disseminating appropriate climate change adaptation measures are of utmost importance in mitigating the risks and vulnerability of agricultural production to climate change. Climate change adaptation on a large scale, combined with appropriate mitigation efforts, helps to reduce the negative impacts of climate change, enhance crop production and improve food security (Loboguerrero et al., Citation2019; Richardson et al., Citation2018).
Literature on climate change adaptation practices can be broadly categorized as studies on macro level (i.e. global, national, or regional) impacts and studies on the individual farm or household-level decisions. Studies dealing with the macro-level climate change adaptation focus on simulation of yield impacts of climate change with and without adaptation. For example, Challinor et al. (Citation2014) suggest that climate change adaptation enhances yield by 7–15% depending on crop type across temperate and tropical regions. Most of the literature on the farm and households centre on producers’ perceptions of climate change and determinants of climate change adaptation practices (Deressa et al., Citation2009; Gadédjisso-Tossou, Citation2015; Gbetibouo, Citation2009; Tazeze et al., Citation2012; Thinda et al., Citation2020; Tilahun & Bedemo, Citation2014). These studies focus on how producers adopt one of various climate change adaptation practices at a time (e.g. changing cropping patterns, using improved crop varieties, diversifying crops, and adopting soil and water conservation practices) without accounting for potential interdependence among practices.
Despite the focus on a single practice at a time in the literature, agricultural producers often face the decision to adopt multiple practices together. For example, an agricultural producer may consider adopting multiple practices such as adopting drought-tolerant crop varieties, soil conservation practices, adjustment of time of planting and improved irrigation practices simultaneously as climate change adaptation practices (Khanal et al., Citation2018). Thus, it is more realistic to assume that an agricultural producer faces the decision to adopt a combination of practices to better manage available resources and mitigate climate-induced risk at the same time rather than one practice at a time. Therefore, there is a need to examine the joint adoption of multiple climate change adaptation practices and their interdependence at the farm and household level.
A right mix of multiple climate change adaptation practices is crucial to improve farm productivity and to reduce the negative impacts of climate change (Aryal et al., Citation2018; Kurukulasuriya & Rosenthal, Citation2013; Teklewold et al., Citation2013). Analysing the adoption behaviour of producers by incorporating the interdependence among climate change adaptation practices can be helpful in identifying the factors that enable or limit joint adoption of multiple climate change adaptation practices. Nhemachena and Hassan (Citation2007), Nhemachena et al. (Citation2014) and Mulwa et al. (Citation2017) identified the nature of relationships (complementary or supplementary) among multiple climate change adaptation practices and their determinants. Furthermore, it is important to understand how the complementary relationships among multiple climate change adaptation practices are influenced by access to Extension services, training related to farming practices and access to information about improved farming practices and reliable weather information (referred to as ‘institutional factors’). Despite the importance, studies that examine the influence of the institutional factors and their relationships with multiple climate change adaptation practices are absent indicating a gap in literature. The lack of literature is an impediment for governments or agencies that are often faced with the decision of allocating limited resources to improve climate resiliency of agricultural production practices.
The objective of this study is to examine how the complementary relationships among multiple climate change adaptation practices are influenced by institutional factors. As a case study, we develop an empirical model that hypothesizes the impact of institutional factors on adoption of drought-tolerant and short-duration rice varieties (referred to as ‘variety’), investment in improved irrigation (referred to as ‘irrigation’) and adoption of direct-seeded rice practice (referred to as ‘DSR’), and the resulting effects on their joint adoption using survey data collected from 359 rice producers in Chitwan district of Nepal in 2019. Results will help local government agencies prioritize the investment on the institutional factors among Extension services, training and information that can potentially enhance the uptake of climate change adaptation practices by identifying the complementary relationships.
The rest of paper is organized as follows. The next section describes the methods: survey design, data and regression models. The following section discusses the findings and the last section summarizes and draws conclusions.
Methods
A producer is more likely to adopt climate change adaptation practices if the expected benefit of adoption is greater than non-adoption. Let and
represent the expected benefit for producers with and without adoption respectively, then a producer decides to adopt adaptation practices if the net benefit of adoption (latent variable,
–
is higher than non-adoption. The latent variable is determined by observed household, socio-economic, institutional and farm characteristics, as well as unobserved factors (error terms). We extend this basic conceptual framework to examine complementary relationships among multiple climate change adaptation practices.
To test the hypothesis, we employ a multivariate probit model where the dependent variables represent binary choices of climate change adaptation practices by rice producers: variety, irrigation and DSR. Multinominal logit and multivariate probit are candidate models with multiple binary dependent variables (Gadédjisso-Tossou, Citation2015; Gbetibouo, Citation2009). A major limitation of the multinominal logit model is that it assumes that the binary choices are mutually exclusive. We acknowledge that a rice producer can adopt more than one practice simultaneously and hence did not use a multinomial logit model.
To capture the potential complementarities among the three climate change adaptation practices, following Lin et al. (Citation2005), we apply the multivariate probit model, which can be expressed as follows (observation subscripts suppressed):
(1)
(1)
where denotes binary variables representing three adaptation practices
variety (V),
irrigation (I) and
DSR (D).
is a vector of explanatory variables,
are the unknown parameters and
are random error components. The random error terms are distributed in a multivariate normal distribution with zero conditional mean and unitary variance;
), where
is a n × n covariance matrix as follows:
(2)
(2)
The off-diagonal elements in the covariance matrix represent the correlation (unobserved) among the error terms of the three adaptation practices (i.e. variety, irrigation and DSR). The unknown parameters () are estimated using a simulated maximum likelihood approach in the multivariate probit model (Chib & Greenberg, Citation1998). It should be noted that unlike linear models, the marginal effect or partial derivative for a given explanatory variable is non-linear and not equal to
. Thus, we estimate the marginal effects of explanatory variables to interpret in terms of likelihood of adoption of climate change adaptation practices as follows:
(3)
(3)
where indicates the likelihood of adopting a climate change adaptation practice
(1 = variety, 2 = irrigation and 3 = DSR),
is the normal cumulative density function and
are the model parameters (Hassan, Citation1996).
The three climate change adaptation practices; variety, irrigation and DSR were selected as the dependent variables in the equations based on the National Adaptation Program of Action report (Ministry of Environment, Citation2010) and previous studies (Biggs et al., Citation2013; IRRI, Citation2018; Khanal et al., Citation2018; Kunreuther et al., Citation2014; Manandhar et al., Citation2011; Ministry of Environment, Citation2010; Ministry of Finance, Citation2014, Citation2018; Piya et al., Citation2013). The explanatory variables Xi include institutional factors, household and socio-economic factors, farm characteristics and perception about climate change (see ).
Table 1. Climate change perceptions of rice producers in Chitwan District in the past 10 years (2009–2018).
Institutional factors
We chose three binary variables: access to Extension, training and information as institutional factors based on an extensive literature review. Agrawal (Citation2008) discussed the role of local institutions as Extension service, capacity building and information dissemination; Gbetibouo (Citation2009) includes agricultural Extension and information as institutional factors; Addisu et al., Citation2016 used Extension and climate information as institutional characteristics; Below et al. (Citation2012) and Aryal et al. (Citation2018) used access to agricultural Extension services as a proxy of institutional service. These three institutional factors are key determinants of adoption of climate change adaptation practices (Abdulai & Huffman, Citation2014; Deressa et al., Citation2009; Piya et al., Citation2013). Specifically, agricultural producers can receive information about improved farming practices and its benefits from Extension workers, which facilitates producers’ decisions for adoptions (Deressa et al., Citation2009; Mulwa et al., Citation2017). Likewise, having access to agricultural training programme helps producers gain in-depth knowledge about new farming techniques and to adopt latest technology (Aryal et al., Citation2018). Similarly, access to weather information can help producers to adjust farm operations, and access to information about improved farming practices can also help agricultural producers to select suitable climate change adaptation practices (Mulwa et al., Citation2017).
The role of three institutional factors in the local context (see ) is described below based on communication with local agricultural Extension agents (Chhetri, Citation2019; Dhital, Citation2020):
Extension: Extension agents are responsible for executing the field level Extension activities with individual producers. They implement the governmental plans and programmes (of the Ministry of Agricultural Development) for the agricultural development. During the face-to-face field/farm visit, Extension worker provides technical service related to the improved production technologies, effective plant protection measures and assist producers in making better farm management decisions. Besides technical services, producers can get information on availability of subsidies on seeds, fertilizers, irrigation, farm tools and machinery through Extension contact. Furthermore, Extension agents organize agricultural fairs, farmers field day and farm visits to motivate producers to implement improved farming technologies. Extension services are offered by Extension agents during field visits to rice farms or through the Extension offices at district headquarters. Proximity to the district office plays a role in producers availing Extension services and Extension agents’ ability to travel to farms on field visits. The timeframe for farm visit of Extension office visit typically is 1-day (Singh, Citation1997).
Training: Both the government and non-government organizations can provide climate change adaptation training to a group of producers (typically 20–25). Producers are trained on topics including climate change, its impacts, implications and methods to reduce the negative impacts of climate change in training sessions. The trainings also impart knowledge on better utilization of local resources, new management practices and improved technologies in the context of climate change. Trainings are offered to producers as demonstrations or in-service workshops in adoption of new varieties, technology and management practices. These are typically provided in 2–3 d workshops at a central location to encourage producer participation. In certain occasions, a scholarship or stipend is provided to producers to encourage participation (Singh, Citation1997).
Information: Information is a mass-contact method offered to producers in the form of factsheets, brochures, publications, booklets, progress reports, Television/radio agricultural programmes, weather forecast announcements, extreme weather events and advisories. Literacy and access to print or digital media affects producers’ access to information. Most of the publications are available for producers at the Extension office (Singh, Citation1997) or online for producers who have internet accessibilities.
Table 2. Comparison of institutional factors – Extension, training and information (adapted from Singh, Citation1997).
The local Extension agents avoid duplication of efforts among the three institutional factors to minimize costs and there is no multicollinearity issue among these variables. While local agrovets services may provide services similar to the three institutional factors, we did not collect any information on local agrovets service which is beyond the scope of this study.
Household and socio-economic factors
As household and socio-economic characteristics, we include gender of the producer who is also the head of the household, dependency ratio (ratio of dependent family members under the age of 16 years or above 60 years to the total economically active members aged 16–60), out-migration (to Gulf and Middle East countries for work), producers’ education years, producers’ years of experience in rice farming, annual household income and membership status of producers in cooperatives or producers’ organizations. Gender of the head of the household is one of the important factors influencing household decision to adopt climate change adaptation practices (Aryal et al., Citation2018; Mulwa et al., Citation2017). We use two variables: dependency ratio and out-migration as a proxy for household labour availability. A dependency ratio in the household measures the household labour availability. Among many farm households in Chitwan, younger family members emigrate to work in Middle-East and Gulf countries leaving parents and other family members behind. The out-migration of family members for work may potentially reduce the household labour availability for some producers (Gartaula et al., Citation2012). Household labour availability determines the manpower to implement farm practices that influence the decision to adopt climate change adaptation practices (Ali & Erenstein, Citation2017; Nhemachena et al., Citation2014). Literature also indicates that a family with higher number of economically active family members has a greater propensity to adopt additional labour-intensive farming practices (Deressa et al., Citation2009). Years of education and farming experience are commonly used as determinants of climate change adaptation practices in literature (Ali & Erenstein, Citation2017; Khanal et al., Citation2018; Mulwa et al., Citation2017). A higher educational attainment of the producer who is the head of the household implies better access to information on improved farming practices, and, thus, greater likelihood of adoption of climate change adaptation practices (Deressa et al., Citation2009). Furthermore, annual household income and membership status of producers in cooperatives or organizations are included because they are expected to have a positive influence on adoption of climate change adaptation practices (Ali & Erenstein, Citation2017; Hassan & Nhemachena, Citation2008; Mulwa et al., Citation2017; Nhemachena et al., Citation2014).
Farm characteristics
The farm characteristics, such as number of plots under rice cultivation and rice area are important factors influencing the adoption of climate change adaptation practices (Mulwa et al., Citation2017; Nhemachena et al., Citation2014). Thus, we include total rice area and number of rice plots under cultivation by allowing a single producer with multiple plots of land. We hypothesize that the producers who sold rice in the market in addition to self-consumption are more likely to adopt climate change adaptation practices.
Perceptions about climate change
We include producers’ perception about changes in local weather patterns over time. We collect producers’ perception about changes in local weather patterns over past 10 years in terms of temperature, rainfall, dry periods, floods, hailstorms, unpredictability of weather, groundwater table, onset and end of monsoon. Producers are more likely to adopt climate change adaptation practices if they noticed changes in local weather patterns (Khanal et al., Citation2018; Nhemachena et al., Citation2014; Nhemachena & Hassan, Citation2007). In accordance to literature, we hypothesize that if producers perceive the changes in local weather patterns, they are more likely to adopt multiple climate change adaptation practices.
Model estimation issues: multicollinearity and endogeneity
The three equations of the multivariate probit model are estimated by using simulated maximum likelihood estimation developed by Cappellari and Jenkins (Citation2003) using mvprobit command in STATA 16 (Statacorp, Citation2019). Multicollinearity of explanatory variables are tested by calculating a conditional index value (Belsley, Citation1991) and variance inflation factor (VIF). A conditional index value represents the collinearity of combinations of variables in data. When the value of the conditional index is less than 30, there is no evidence of multicollinearity (Belsley, Citation1991). We did not find evidence of multicollinearity because the condition index value is 9.48, which is not above the threshold level of 30 and the mean VIF is 4.4. We do not have a serious issue of multicollinearity when the VIF value is less than threshold of 10 (Marquardt, Citation1970; Mason et al., Citation1989). A correlation matrix of institutional factors, the variance inflation factors and conditional index values are presented in Appendix 2.
We test how hypothetical changes of institutional factors influence the complementarities of multiple adaptation practices using the post-estimation prediction. To implement the test, first, we estimate the predicted likelihood of climate change adaptation practices based on the results of the three equations of the multivariate probit model (referred to as ‘baseline predicted likelihood’), then we predict the likelihood of adoption of multiple climate change adaptation practices by hypothetically increasing the rice producers with access to Extension, training and information (or increasing institutional factors) through randomly switching their binary values from zeros to ones by 10% for each of the institutional factors using randomtag command in STATA 16 (Statacorp, Citation2019). Then, we test the complementarity relationship by testing how correlated the predicted increase in the probabilities across the three climate change adaptation practices as a result of switching the values of the binary variables, representing access to Extension, training and information from zeros to ones by 10% each. We also conduct sensitivity tests by hypothetically increasing access to institutional factors by 20%, 30% and 40%.
We address the potential endogeneity of binary institutional factors. Our model has a binary dependent variable (adoption decision) and the potential endogenous variables (Extension, Training and Information) are also binary. The multivariate probit model is a joint probability model which accounts interrelationship of adoption decisions (dependent variable) and potential endogeneity issue in such probability model (multivariate model having binary Y, binary X) are relatively less emphasized in the literature. Finding three distinct instrumental variables (IVs) are very challenging in model estimation. There are many studies in the literature that emphasize the difficulty in finding relevant IVs and model estimation in limited dependent variable models (Rivers & Voung, Citation1988; Clarke & Windmeijer, Citation2012; Bollen, Citation2012; Shardell & Ferrucci, Citation2016). We used three instrumental variables:
Ethnicity/social status of the respondent is used as an instrument for the access to Extension following Devkota (Citation2005). Each survey participant indicated their ethnicity – Brahmin/Chettri, Janajati or Dalit. Brahmin/Chhetri have higher social status and resources to access Extension services (Devkota, Citation2005) and ability to travel to Extension office. However, ethnicity does not influence climate change adaptation practices.
We use producers risk attitudes as an instrument for climate change adaptation training. Risk averse producers are expected to participate in training programmes (Kunreuther et al., Citation2014; Nisengwe, Citation2016) than risk neutral or risk-taking producers.
The number of literate family members in the household is used as an instrument for access to agricultural and weather information. Having more educated household members can improve access to agricultural and weather information (Dulal et al., Citation2010) through multiple sources.
We use two approaches to address potential endogeneity problem. First, we used Durbin-Wu-Hausman test of endogeneity in a two-stage framework (). We failed to reject the null hypothesis for variety (p-value = 0.3110), irrigation (p-value = 0.1545) and DSR (p value = 0.1492) indicating the variables are exogenous and affirming that endogeneity is not an issue in our model.
Table 3. Durbin–Wu–Hausman test of endogeneity for variety, irrigation and DSR.
In the second approach, following Rivers and Voung (Citation1988) and Aryal et al., (Citation2018), we first specified the potential endogenous variables as a function of instruments and control variables. Then, we use residuals of the first stage regression as an additional control variable in the model to acquire consistent estimates. If the residuals of the first stage regression are not significant, we do not have an issue of endogeneity (Aryal et al., Citation2018). The residuals of all three variables are not significant in the model and hence we report coefficients without residual terms. A detailed description of the instrumental variables and results from the first stage regressions for potential endogenous variables are provided in Appendix 3.
Since endogeneity is not an issue, we do not believe there are any problems with reverse causality because reverse causality causes endogeneity problem (Cingolani & Crombrugghe, Citation2012; Feng & Wu, Citation2018).
Study area
The study area for this research is Chitwan district, which is located in the south-central Plains (Terai) region of Nepal in South-Asia (see ). We chose Chitwan district as the study area because rice producers in Chitwan district faced high variation in temperature and precipitation (Gurung & Bhandari, Citation2009) in the recent years. Upon consultation with the District Agricultural Extension Office (Agricultural Knowledge Center, Chitwan), three villages in Chitwan were recommended as a study area due to a relatively higher proportion of rice producers. The meteorological station in Rampur located in Chitwan district provided temperature and precipitation records for the study. The proximity of student enumerators from Agricultural and Forestry University in Chitwan solidified the study area. While it could be informative to add other study areas, however, given our limited budget, we could not survey producers outside of Chitwan district.
Figure 1. Study area of three villages in Chitwan district, which is located in the south-central Plains (Terai) region of Nepal.
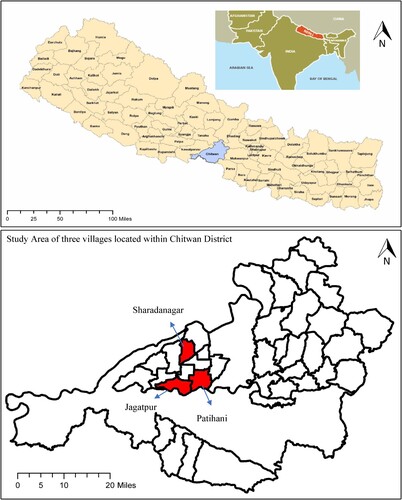
According to the District Agricultural Development Office in Chitwan (DADO, Citation2017), rice is cultivated in 33,900 hectares, which represents 77% of the district’s total arable land. Approximately 67% of households are involved in agricultural activities. The average household size in Chitwan district was 4.38 members with a mean household income in Nepali Rupees (NR) of 169,070 (equivalent to US Dollar, $1,406). 76.98% of the households were literate and 33.69% of the households were female-headed. The average farm size is 0.46 hectares (1.13 acres) which was lower than national average of 0.68 hectares (1.68 acres). The average rice yield was 3.4 metric tons per hectare, which was lower than national average of 3.76 metric tons per hectare. More than half of the producers (55.72%) were members of farmer’s group/co-operatives (Central Bureau of Statistics, Citation2018; DADO, Citation2017).
Survey design
All the data for the multivariate probit model was collected from a field survey of rice producers in Chitwan district, Nepal in June, 2019. First, out of seven administrative units in Chitwan district, the largest administrative unit, Bharatpur was selected, which consists of a total of 69,035 households out of which 45,991 were farming households. Second, in consultation with district agricultural Extension office in Chitwan, three villages where rice is a major crop – Patihani, Jagatpur and Sharadanagar were selected as the target survey area. Third, out of the total number of farming households in three villages, 4,090, the sample size was estimated to be at least 352 at the 95% confidence interval, following the sample size determinization method developed by Krejcie and Morgan (Citation1970).
Survey data
Four undergraduate students of Agriculture and Forestry University, who were proficient in Nepali language and familiar with the landscape and customs of Chitwan were hired as enumerators. They were trained and provided directions to fill the questionnaire before conducting the field survey. The enumerators started at the periphery of the village, selected farming households and progressed towards the centre of the village. Each survey participant was read a consent statement by the enumerator following Institutional Review Board procedures describing their involvement in the study, protecting their privacy and ensuring confidentiality. Upon providing consent that the respondent was 18 years and older, understood the information and agreed to participate in the survey, individual producers answered the survey in a face-to-face interaction with enumerators. A total of 383 producers were contacted, 359 producers (93.7%) answered the survey and 24 producers declined to participate in the survey (6.3%). Surveys were conducted at the producers’ house and at local gathering spots in the three villages. Each survey took approximately 15 min to complete. Summary statistics of the variables used in the multivariate probit model are presented in .
Table 4. Descriptions of variables used in the multivariate probit model.
Triangulation analysis
The relationship among local climate trend data, producers’ perceptions, and survey responses are illustrated in the conceptual model of triangulation in . Rice producers in Chitwan observed climate trends and noted changes in local climate trends over the past several decades, in particular the past 10 years. Through the survey, among many parameters, producers expressed their perceptions on the trends (increased, decreased, or no change) of average temperature, rainfall, dry periods, floods, hailstorms and monsoon onset. The trend analysis of temperature and rainfall data (2009–2018) of Rampur Chitwan supports producers’ perceptions of increasing temperature trends and variability in rainfall patterns. Piya et al. (Citation2012) investigate local communities’ (Chepang) perceptions of climate change in Nepal's rural mid hills. They compared the community responses to the recorded temperature and rainfall trends to see if the perceived changes corresponded to the local climate trends. The survey data suggests that the farm practices were adversely affected by climate change in terms of delay in transplanting rice, reduced irrigation water availability, crop losses due to flooding, increased incidence of diseases/pests, more weeds and grain loss at harvest which in turn reduced the rice yield and net income from rice production. Through the survey, producers also provided their preferences on climate change adaptation practices including – varietal management (drought-tolerant, short-duration, pest-tolerant varieties), investment in improved irrigation practices (new canal construction, change in frequency of irrigation, installing solar water pumps and back-up generators) and use of DSR among other practices. Access to institutional factors such as Extension, training and information increases the likelihood of adopting improved farm practices. We explore complementary relationships among the three climate change adaptation practices that are influenced by the three institutional factors.
Complementarity
In this study, we examine how the complementary relationships among multiple climate change adaptation practices are influenced by institutional factors (). Among the adaptation practices, we explore adoption of drought-tolerant and short-duration rice varieties, investment in improved irrigation practices such as new canals, irrigation timing as well as solar pumps and adoption of DSR practice. Included in the institution factors are Extension farm visit by agricultural agents, office visits by producers, climate-smart and adaptation training, accessing weather, technology and information sources. The socio-economic explanatory variables that influenced varietal, improved irrigation and DSR include gender, dependency ration, out-migration, number years of education, farming years of experience, income and membership of producers’ organizations. The farm characteristics include number of rice plots, amount of rice sold and area of rice under cultivation. The climate-related factors capture rice producers’ perceptions of climate change and variability.
Results and discussion
This section is organized as follows: first, the key findings of the temperature and precipitation trend analysis from Rampur are presented followed by rice producers’ perceptions to climate change and the strategies adapted as a response to climate change. Next, the multivariate probit results describe the complementarity relationship between climate change adaptations and institutional factors. The limitations of the study are noted and the reasons for producers not taking up adaptation practices are identified.
Weather trends, climate change and climate change adaptation
Between 1987 and 2016, Nepal experienced a trend of warming temperature and declining precipitation ( and ). Trend analysis of ten years of climatic data (2009–2018) of Rampur (Chitwan) indicated increases of 0.019°C/year and 0.003°C/year in maximum and minimum temperature, respectively (). Following Piya et al. (Citation2012), a linear trend analysis of maximum and minimum temperature during June–October, which is a rice growing season, between the period of 2009 and 2018 indicated that both maximum and minimum temperatures increased by 0.0255 and 0.0257°C/year at Rampur (Chitwan), respectively (). Between 2009 and 2018, a linear trend analysis of precipitation at Rampur, Chitwan indicated a decline of 42.2 mm (or 1.66 in.) in annual rainfall and 34.6 mm (or 1.36 in.) in seasonal rainfall during June–October ().
Figure 3. Average annual temperature trend of Nepal (1987–2016). Source: World Bank Group (Citation2020).
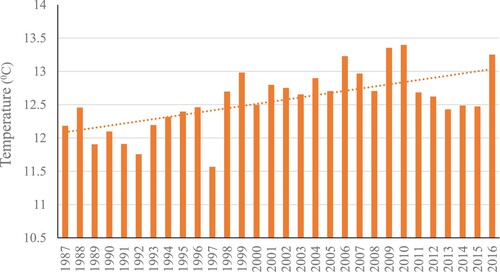
Figure 4. Average annual precipitation trend of Nepal (1987–2016). Source: World Bank Group (Citation2020).
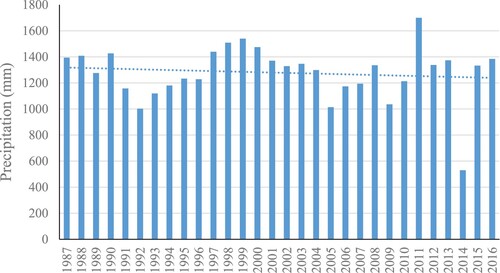
Figure 5. Average annual temperature at Rampur, Chitwan (2009–2018). Source: Department of Hydrology and Meteorology, Citation2019, Kathmandu, Nepal.
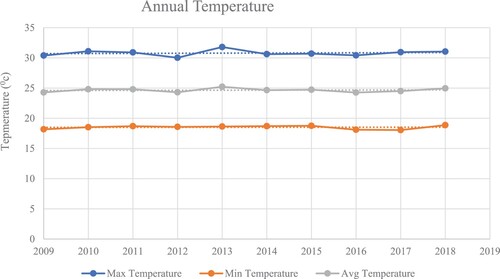
Figure 6. Maximum, minimum and average seasonal temperature (June–October) at Rampur, Chitwan (2009–2018). Source: Department of Hydrology and Meteorology, Citation2019, Kathmandu, Nepal.
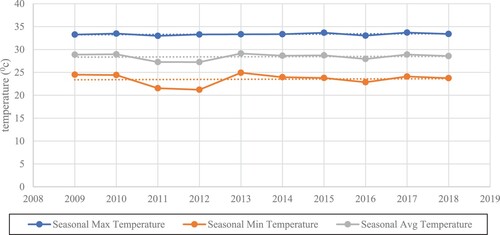
Figure 7. Annual and seasonal rainfall (June–October) at Rampur, Chitwan (2009–2018). Source: Department of Hydrology and Meteorology, Citation2019, Kathmandu, Nepal.
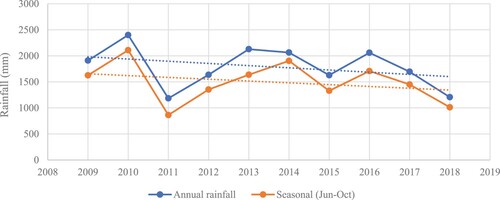
76.6% of rice producers in Chitwan perceived changes in local weather patterns in the past ten years. While 86.9% of producers noted higher summer temperatures, only 61.9% noticed higher temperatures in winter. More than three-fourths of rice producers indicated high intense rainfall and a late onset of monsoon rain but less than a third of producers were affected by floods. Almost all the producers indicated hailstorms and unpredictable weather in the past ten years (see ).
Rice producers in the study area were found to be growing drought-tolerant and short-duration rice varieties. Out of 359, 233 (or 64.9%) producers adopted varietal management. During the household survey, producers reported that they replaced traditional, long-duration rice varieties: Aapjhutte, Anadi, Dhudhraj, Gurdi and Mansara. They also replaced Mansuli variety due to higher disease infestation. Improved varieties including Hardinath, Sukkhadhan-2, Sukkhadhan-4 and Ramdhan as well as hybrid varieties such as Garima, Gorakhanath and Loknath were the most popular varieties cultivated in the study area. Out of 359, 218 (or 60.7%) producers invested in improved water infrastructure and water conservation practices. Producers invested in new irrigation infrastructure like constructing new wells, installing electric motors to pump water, cleaning and maintenance of existing wells and canal construction for better water flow to fields. In some areas, producers also conserved water in lowlands (wetlands also called Ghol in Nepali). Although upland (tadhi in Nepali) may be an important factor in climate change adaptation, our sample did not have any producers growing rice in upland, all the producers cultivated rice in irrigated lowlands. Ridges (earthen structure) were constructed as barriers and the runoff water was re-utilized by pumping it back for irrigation. 56 of 359 (or 15.6%) producers adopted DSR practice. Producers may adopt DSR practice irrespective of the availability of water for land preparation and not depend on the onset of monsoon.
A total of 158 out of 359 (or 44%) producers received training related to climate change adaptation practices. Although local governmental organizations and private institutions organized training related to farming practices, more than half of producers did not have access to such training programmes. Access to Extension services was assessed by ascertaining if Extension agents contacted producers. More than half of producers (54%) had availed agricultural Extension services. The proximity of Extension office to producers may have influenced the participation of producers in training and producers’ access to Extension services. More than half of producers (54%) also had access to technical farming information and weather-related information. Access to technical farming information (including price and market information) and weather update and/or forecasts was assessed by whether producers have access to TV, FM radio, agricultural and weather applications on mobile phones and receiving a text message about farming information and weather updates on a mobile phone.
Information is found to be accurate by 73% of producers and 99% of the producers find it to be useful in adoption of climate change strategies. Over 95% of producers prefer television and FM radio for timely information, more than two-thirds of producers prefer Extension publications as a reliable source of information. Less than one-third of producers prefer mobile applications for weather information and less than 9% of producers rely on internet for weather information.
Multivariate probit results
The Wald test of the three equations of the multivariate probit model (Wald chi2, (42) = 315.30; Prob > = 0.000) is highly significant and rejects the null hypothesis that all regression coefficients are jointly equal to zero, and showing that the model fits the data well. We test the complementary relationship among multiple climate change adaptation practices by using pair-wise correlation coefficients across the residuals of the three equations of the multivariate probit model. The likelihood ratio test (
(3) = 29.38, Prob >
= 0.000) rejects the null hypothesis that the covariance of the residuals of the multivariate probit model equations is equal to zero. Results indicate that all three pairs of residuals were positively correlated (0.617 for variety and irrigation; 0.241 for variety and DSR and 0.085 for irrigation and DSR), suggesting that the three climate change adaptation practices are complementary to each other. The variety and the irrigation practices have the highest correlation, indicating that producers tend to jointly adopt both practices, ceteris paribus. This result has important policy implications that among variety and irrigation, enhancement of adoption of either practice would increase the adoption of the other practice because these practices tend to be adopted together.
The parameter estimates of the three equations of the multivariate probit model are presented in . The results indicate that producers are 15.58% (P < 0.01), 13.14% (P < 0.05) and 9.37% (P < 0.10) more likely to adopt variety if they have access to Extension, training and information, respectively. Imparting knowledge and skills through education, training and information influence producers to use improved rice varieties. These findings align with previous studies where access to Extension services was found to increase the likelihood of adoption of climate change adaptation practices (Deressa et al., Citation2009; Mulwa et al., Citation2017; Nhemachena & Hassan, Citation2007). Access to Extension and training significantly increases the likelihood of adoption of improved irrigation practices by 9.37% (P < 0.05) and 8.77% (P < 0.10), respectively. Gaining more knowledge and awareness through education, training and learning from farming activities encouraged producers to invest in improved irrigation practices resulting in higher rice yield. This result confirms the finding of Aryal et al. (Citation2018), who reported that access to agricultural training enhanced the likelihood of adoption of climate-change adaptation practices. Similarly, having access to information related to weather and improved farming technique significantly increases the likelihood of adoption of DSR practice by 10.35%, which is consistent with the earlier findings of Mulwa et al. (Citation2017) and Aryal et al. (Citation2018). Both of these studies indicated that availability of weather information and information on improved farming practices significantly enhance the adoption of multiple climate change adaptation practices.
Table 5. Empirical results of the multivariate probit model.
As compared with the baseline predicted likelihood, a 10% increase in the rice producers with access to Extension results in the highest increment in the likelihood of adoption of variety (1.38%) followed by training (1.16%) and information (0.84%) (see ). Similarly, a 10% increasein the rice producers with access to Extension results in the highest increment in the likelihood of the adoption of irrigation (0.89%), followed by training (0.88%). In addition, a 10% increase in the rice producers with training results in the highest increments in the likelihood of adoption of DSR (1.24%), followed by information (0.61%) and Extension (0.50%).
Table 6. Predicted likelihood of adoption of multiple climate change adaptation practices by hypothetically increasing institutional factors and pairwise correlation coefficients between the predicted likelihood.
The pairwise correlation coefficients between the predicted likelihood of adoption of multiple climate change adaptation practices across variety and irrigation; variety and DSR and irrigation and DSR are 0.955, 0.529 and 0.631, respectively in the case of increasing access to Extension (see ). Similarly, the pairwise correlation coefficients between the predicted likelihood of adoption of multiple climate change adaptation practices across variety and irrigation; variety and DSR and irrigation and DSR are 0.942, 0.469 and 0.656 respectively in the case of increasing training. The pairwise correlation coefficients between the predicted likelihood of adoption of multiple climate change adaptation practices across variety and DSR is 0.602 in the case of increasing information. These findings suggest that effective Extension and farmer’s training can enhance the complementarities among all three pairs of climate change adaptation practices whereas an increase in information enhances the complementarity between variety and DSR. Results of sensitivity test using 20%, 30% and 40% increase of each of three institutional factors follow similar patterns in terms of magnitude and sign of the institutional factors as in the case of 10% increase (see Appendix 1 for details).
The effects of other variables, including socio-economic, farm characteristics and climate-related variables, on the climate change adaptation practices are also presented in . 45.9% of households had at least one family member migrate to another country for employment. Households with members who out-migrated are 7.43% less likely to adopt variety as a climate change adaptation practice. This might be due to the shortage of household labour in the age group of 16–60 years of age who typically out-migrate for employment.
The average years of farming experience among rice producers in Chitwan was 26 years. Contrary to our expectations, farming experience is less likely (less by 0.35%) to influence DSR adoption. This might be because farmers with more years of experience are relatively older, and it is likely that older producers are reluctant to change and adopt newer climate change adaptation practices. The direction of influence of dependency ratio and rice area is positive in case of adoption of DSR practice, which is realistic that DSR practices require less labour compared to the practice of transplanted rice. International Rice Research Institute (Citation2018) also reported that DSR is relatively less labour-intensive practice than conventional transplanting method. Furthermore, Membership of producers’ organizations enhances the likelihood of adoption of improved irrigation. 68.5% of producers are members of a farmer’s group or a co-operative. Membership of producers’ organizations enhance the likelihood of adoption of improved irrigation by 15.05%. Being a member of a producer’s organization helps to pool available individual resources, transfer technology to peers and influence other producers in the adoption of climate change adaptation practices. Producers having a greater number of rice plots are more likely to adopt variety. Furthermore, rice selling households are more likely to adopt improved irrigation. If producers perceived variability in local weather patterns over the years, they are more likely to adopt varietal selection, invest in improved irrigation practices and adoption of DSR practice. Consistent with existing literature (Deressa et al., Citation2009; Khanal et al., Citation2018; Mulwa et al., Citation2017), results reveal that an increase in the number of years of education of the head of the household, the likelihood of adoption would be enhanced. These results highlight the benefits of better access to education to enhance the uptake of multiple adaptation practices.
A limitation of this study is that we do not directly measure awareness of climate change adaptation strategies. However, survey data indicates the reason producers do not adopt a particular adaptation practice. The survey responses indicate that among non-adopters, 41% of producers did not adopt DSR due to lack of information about the practice. Similarly, 41.1% of producers did not adopt variety due to lack of information while 59.6% of producers did not adopt improved irrigation practices because of lack of funds.
Summary and conclusions
This paper examines how the complementary relationships among multiple climate change adaptation practices are influenced by access to Extension services, training related to farming practices, farming practices and weather-related information (referred to as ‘institutional factors’). Our study examines how the complementary relationships among multiple climate change adaptation strategies (improved varieties, irrigation practices and DSR) are influenced by access to Extension services, training related to farming practices and weather-related information (referred to as ‘institutional factors’). At a macro-level, our study provides valuable information to policymakers on prioritizing limited resources to support and improve specific services to rice producers – Extension, training and information that are effective in the implementation of climate change adaptation strategies.
As a case study, we develop an empirical model that hypothesizes the institutional factors on the adoption of variety, irrigation and DSR, and the resulting effects on their joint adoption using survey data collected from 359 rice producers in the Chitwan district of Nepal in 2019. The results reveal that: (1) adoption of all the three climate change adaptation practices is mutually complementary; (2) all three institutional factors enhance the likelihood of the adoption of variety, access to Extension and training enhances the likelihood of the adoption of improved irrigation and information enhances the likelihood of the adoption of DSR and (3) access to Extension and training enhance the complementarities among all three pairs of adaptation practices (variety and irrigation; variety and DSR; and irrigation and DSR), while information enhances the complementarity between variety and DSR only.
On the basis of the findings summarized above, local government agencies can prioritize the investment on the institutional factors that help rice producer’s adoption of multiple climate change adaptation practices. The result (1) suggests that the three climate change adaptation practices need to be adopted in an organized manner so that the adoption can be done more effectively. For example, when rice producers adopt variety and irrigation practices jointly, they can coordinate their efforts, minimize redundancies and achieve efficient outcomes. The result (2) suggests that it is equally worthy to allocate resources to improve the likelihood of the adoption of the three climate change adaptation practices through Extension agents’ work on technical services, climate change adaptation training programme by the government and non-government organizations and information in the form of factsheets, brochures, publications, booklets and progress reports. The result (3) suggests that investment on improving access to Extension and training is potentially more efficient than improving information accessibility because the former two institutional factors trigger the complementarities among all three pairs of climate change adaptation practices, while the latter institutional factor prompts the complementarity between variety and DSR only. The evaluation of the relative degree of influence of complementary adaptation measures is beyond the scope of this study and can be explored in future studies.
Given the results, improving access to Extension and training is what local government agencies can focus for the uptake of climate change adaptation practices. For example, enhancing the scope of the Community Agricultural Extension Service Center (CAESC) as envisioned by Agricultural Development Strategy (ADS) of Nepal may be the primary target area for investment that aims to improve rice producers’ adoption of climate change adaptation practices. Furthermore, along with regular Extension programmes, local government agencies can develop and implement producers’ capacity-building programme. For example, farmer field school approach, which is a group-based learning approach that teaches how to experiment and solve problems independently (Dhamankar & Wongtschowski, Citation2014) may help producers to improve awareness of climate change and adoption of multiple climate change adaptation practices.
The policy recommendation comes with a caveat because our framework overlooks potential costs associated with improving institutional factors. Though exact cost estimates for improving each of the institutional factors were not available at the time of our survey, we acknowledge that the cost of improving Extension and training programme is generally higher than the cost of improving information in our study area (Dhital, Citation2020). For example, improving information through publishing brochures, factsheets on improved agricultural practices, varieties, weather, price and marketing information is relatively cheaper than developing Extension or training programme. Such information is critical in that improving access to Extension and training to take advantage of their resulting complementary relationships among multiple climate change adaptation practices needs to be carefully evaluated after accounting for the comparisons of the costs associated with them.
To provide more comprehensive recommendations to local government agencies for prioritizing the investment in the institutional factors, future research could explore the complementary relationships among multiple climate change adaptation practices triggered by institutional factors that also consider their costs. Such efforts require cost information that can be incorporated in our three equations of the multivariate probit model. It may be challenging to normalize the cost data that fits in the model because the unit costs for improving access to Extension, training and information are not standardized. Once the normalized cost data that fits in the model is acquired, the variables that capture the interactions between institutional factors and their per unit costs can be included in the three equations, which allows to sort out how the complementary relationships among multiple climate change adaptation practices are influenced by the institutional factors condition upon their costs. This study could also be extended to evaluate how producers would adopt DSR, improved varieties and irrigation practices if governmental funds were provided to support these climate change adaptation strategies.
Acknowledgements
We acknowledge the support and cooperation of rice producers in Chitwan, Nepal.
Disclosure statement
No potential conflict of interest was reported by the author(s).
Additional information
Funding
Notes on contributors
Hari P. Regmi
Hari P. Regmi is a PhD candidate at the Department of Agricultural Economics, Purdue University, West Lafayette, Indiana, USA. His research areas include agricultural policy; forecast evaluation related to agricultural policy; and climate change adaptation.
Sreedhar Upendram
Dr. Sreedhar Upendram is an Assistant Professor in the Department of Agricultural and Resource Economics at the University of Tennessee. His primary areas of research are community economics, economic impact analysis, rural development and natural resource economics. He serves as the statewide Community Economic Development Specialist for the University of Tennessee Extension.
Seong-Hoon Cho
Dr. Seong-Hoon Cho is a Professor at the Department of Agricultural &Resource Economics, University of Tennessee Institute of Agriculture.His main research focuses on promoting sustainability of agriculturaland forested lands by examining economic issues related to land use,ecosystem service provision, and conservation science usingmicroeconomics and econometrics.
Christopher D. Clark
Dr. Christopher Clark is currently serving as Professor and Head of the Department of Agricultural & Resource Economics at the University of Tennessee. His research interests include a variety of topics in environmental and natural resource economics. He teaches graduate and undergraduate courses in natural resource economics and an undergraduate course in agricultural and environmental law. He earned a Ph.D. in Economics from Vanderbilt University and a J.D. from Tulane University.
References
- Abdulai, A., & Huffman, W. (2014). The adoption and impact of soil and water conservation technology: An endogenous switching regression application. Land Economics, 90(1), 26–43. https://doi.org/10.3368/le.90.1.26
- Addisu, S., Fissha, G., Gediff, B., & Asmelash, Y. (2016). Perception and adaptation models of climate change by the rural people of lake Tana Sub-Basin, Ethiopia. Environmental System Research, 5(1), 7. https://doi.org/10.1186/s40068-016-0059-0
- Adger, W. N., Huq, S., Brown, K., Conway, D., & Hulme, M. (2003). Adaptation to climate change in the developing world. Progress in Development Studies, 3(3), 179–195. https://doi.org/10.1191/1464993403ps060oa
- Agrawal, A. (2008). The role of local institutions in adaptation to climate change. World Bank. https://openknowledge.worldbank.org/handle/10986/28274
- Ali, A., & Erenstein, O. (2017). Assessing farmer use of climate change adaptation practices and impacts on food security and poverty in Pakistan. Climate Risk Management, 16, 183–194. https://doi.org/10.1016/j.crm.2016.12.001
- Aryal, J. P., Rahut, D. B., Maharjan, S., & Erenstein, O. (2018). Factors affecting the adoption of multiple climate-smart agricultural practices in the Indo-Gangetic Plains of India. Natural Resources Forum, 42(3), 141–158. https://doi.org/10.1111/1477-8947.12152
- Below, T. B., Mutabazi, K. D., Kirschke, D., Franke, C., Sieber, S., Siebert, R., & Tscherning, K. (2012). Can farmers’ adaptation to climate change be explained by socio-economic household level variables? Global Environmental Change, 22(1), 223–235. https://doi.org/10.1016/j.gloenvcha.2011.11.012
- Belsley, D. A. (1991). A guide to using the collinearity diagnostics. Computer Science in Economics and Management, 4, 33–50. https://link.springer.com/content/pdf/10.1007BF00426854.pdf
- Biggs, E. M., Tompkins, E. L., Allen, J., Moon, C., & Allen, R. (2013). Agricultural adaptation to climate change: Observations from the Mid-Hills of Nepal. Climate and Development, 5(2), 165–173. https://doi.org/10.1080/17565529.2013.789791
- Bollen, K.A. (2012). Instrumental variables in sociology and the social sciences. Annual Review of Sociology, 38, 37–72.
- Borrelli, P., Robinson, D. A., Panagos, P., Lugato, E., Yang, J. E., Alewell, C., Wuepper, D., Montanarella, L., & Ballabio, C. (2021). Land use and climate change impacts on global soil erosion by water (2015–2070). Proceedings of the National Academy of Sciences of the United States of America, 117(26), 21994–22001. https://doi.org/10.1073/pnas.2001403117
- Cappellari, L., & Jenkins, S. P. (2003). Multivariate probit regression using simulated maximum likelihood. The Stata Journal: Promoting Communications on Statistics and STATA, 3(3), 278–294. https://doi.org/10.1177/1536867x0300300305
- Central Bureau of Statistics. (2018). District Profile of Chitwan. Kathmandu, Nepal.
- Challinor, A., Watson, J., Lobell, D., Howden, S., Smith, D., & Chhetri, N. (2014). A meta-analysis of crop yield under climate change and adaptation. Nature Climate Change, 4(4), 287–291. https://doi.org/10.1038/nclimate2153
- Chhetri, P. (2019, June 10). Personal communication. Agricultural Officer. Agricultural Knowledge Center (AKC), Chitwan, Nepal.
- Chib, S., & Greenberg, E. (1998). Analysis of multivariate probit models. Biometrika, 85(2), 347–361. https://doi.org/10.1093/biomet/85.2.347
- Cingolani, L., & Crombrugghe, D. (2012). Techniques for dealing with reverse causality between institutions and economic performance (Working Paper No. 34). Maastricht Economic and Social Research Institute on Innovation and Technology (MERIT), pp. 1–28.
- Clarke, P.S. & Windmeijer, F. (2012). Instrumental variable estimators for binary outcomes. Journal of the American Statistical Association, 107(500), 1638–1652.
- Cline, W. R. (2007). Global warming and agriculture: Impact estimates by country. Center for Global Development and Peterson Institute for International Economics.
- Department of Hydrology and Meteorology. (2019). Historical data (1980-2018) on temperature and precipitation at Rampur, Chitwan. Kathmandu, Nepal.
- Deressa, T. T., Hassan, R. M., Ringler, C., Alemu, T., & Yesuf, M. (2009). Determinants of farmers’ choice of adaptation methods to climate change in the Nile Basin of Ethiopia. Global Environmental Change, 19(2), 248–255. https://doi.org/10.1016/j.gloenvcha.2009.01.002
- Devkota, D. (2005). Gender and ethnicity dimensions in relation to access to and control over household resources in Nepal. Journal of the Institute of Agriculture and Animal Science, 26, 135–141. https://doi.org/10.3126/jiaas.v26i0.666
- Dhamankar, M., & Wongtschowski, M. (2014). Farmer Field Schools (FFS). Note 2. Global Forum for Rural Advisory Services (GFRAS) Good Practice Notes for Extension and Advisory Services. Lindau: GFRAS. https://www.g-fras.org/en/good-practice-notes/19-agri-cooperatives.html
- Dhital, K. (2020, December 5). Personal communication. Agriculture Extension Officer, Ministry of Agricultural Development, Singha Durbar, Kathmandu, Nepal.
- District Agricultural Development Office. (2017). Annual Bulletin - Fiscal year 2015/16. District Agriculture Development Office, Chitwan, Nepal.
- Dulal, H. B., Brodnig, G., Thakur, H. K., & Green-Onoriose, C. (2010). Do the poor have what they need to adapt to climate change? A case study of Nepal. Local Environment, 15(7), 621–635. https://doi.org/10.1080/13549839.2010.498814
- Feng, Q., & Wu, G. L. (2018). On the causality between output and infrastructure: The case of China. Economic Modelling, 74(2018), 97–104. https://doi.org/10.1016/j.econmod.2018.05.006
- Food and Agriculture Organization. (2014). Managing climate risks and adapting to climate change in the agriculture sector in Nepal. Food and Agriculture Organization of the United Nations. http://www.fao.org/3/i3577e/i3577e.pdf
- Gadédjisso-Tossou, A. (2015). Understanding farmers’ perceptions of and adaptations to climate change and variability: The case of the Maritime, Plateau and Savannah regions of Togo. Agricultural Sciences, 6(12), 1441–1454. https://doi.org/10.4236/as.2015.612140
- Gahatraj, S., Jha, R. K., & Singh, O. P. (2018). Impacts of climate change on rice production and strategies for adaptation in Chitwan, Nepal. Journal of Agriculture and Natural Resources, 1(1), 114–121. https://www.nepjol.info/index.php/janr/article/view/22226/18953. https://doi.org/10.3126/janr.v1i1.22226
- Gartaula, H., Niehof, A., & Visser, L. (2012). Shifting perceptions of food security and land in the context of labour out-migration in rural Nepal. Food Security, 4(2), 181–194. https://doi.org/10.1007/s12571-012-0190-3
- Gbetibouo, G. A. (2009). Understanding farmers’ perceptions and adaptations to climate change and variability, the case of the Limpopo Basin, South Africa. International Food Policy Research Institute (IFPRI) Discussion Paper No. 00849. Environment and Production Technology Division, International Food Policy Research Institute, Washington D.C. USA.
- Gumma, M. K., Gauchan, D., Nelson, A., Pandey, S., & Rala, A. (2011). Temporal changes in rice-growing area and their impact on livelihood over a decade: A case study of Nepal. Agriculture Ecosystems & Environment, 2(3–4), 382–392. https://doi.org/10.1016/j.agee.2011.06.010
- Gurung, G. B., & Bhandari, D. (2009). Integrated approach to climate change adaptation. Journal of Forest and Livelihood, 8(1), 90–99.
- Hassan, R. M. (1996). Planting practices of maize farmers in Kenya: A simultaneous equations analysis in the presence of discrete dependent variables. Agricultural Economics, 1(2), 137–149. https://doi.org/10.1111/j.1574-0862.1996.tb00427.x
- Hassan, R., & Nhemachena, C. (2008). Determinants of African farmers’ practices for adapting to climate change: Multinomial choice analysis. African Journal of Agricultural and Resource Economics, 2(1), 83–104. https://doi.org/10.22004/ag.econ.56969
- Intergovernmental Panel on Climate Change. (2007). Climate Change 2007: Impacts, Adaptation and Vulnerability. Contribution of Working Group II to the Fourth Assessment Report of the Intergovernmental Panel on Climate Change [M. L. Parry, O. F. Canziani, J. P. Palutikof, P. J. vander Linden and C. E. Hanson, Eds.]. Cambridge: Cambridge University Press.
- Intergovernmental Panel on Climate Change. (2019). Summary for policymakers. In Climate change and land: An IPCC special report on climate change, desertification, land degradation, sustainable land management, food security, and greenhouse gas fluxes in terrestrial ecosystems [P. R. Shukla, J. Skea, E. Calvo Buendia, V. Masson-Delmotte, H.- O. Pörtner, D. C. Roberts, P. Zhai, R. Slade, S. Connors, R. van Diemen, M. Ferrat, E. Haughey, S. Luz, S. Neogi, M. Pathak, J. Petzold, J. Portugal Pereira, P. Vyas, E. Huntley, K. Kissick, M. Belkacemi, J. Malley, Eds.].
- Intergovernmental Panel on Climate Change. (2021). Summary for policymakers. In Climate Change 2021: The Physical Science Basis. Contribution of Working Group I to the Sixth Assessment Report of the Intergovernmental Panel on Climate Change [Masson Delmotte, V., P. Zhai, A. Pirani, S.L. Connors, C. Péan, S. Berger, N. Caud, Y. Chen, L. Goldfarb, M.I. Gomis, M. Huang, K. Leitzell, E. Lonnoy, J.B.R. Matthews, T.K. Maycock, T. Waterfield, O. Yelekçi, R. Yu, and B. Zhou, Eds.]. Cambridge University Press.
- International Rice Research Institute, Philippines. (2018). Direct-seeded rice consortium: Partnership for the future of rice production. https://dsrc.irri.org/resources/publications
- Kabir, A., Amin, M. N., Roy, K., & Hossain, M. S. (2021). Determinants of climate change adaptation strategies in the coastal zone of Bangladesh: Implications for adaptation to climate change in developing countries. Mitigation and Adaptation Strategies for Global Change, 26(7), 26–30. https://doi.org/10.1007/s11027-021-09968-z
- Karna, P. K. (2014). The impact of climate change on rice production in Nepal (Working paper). South Asian Network for Development and Environmental Economics, Kathmandu, Nepal. https://ideas.repec.org/p/snd/wpaper/85.html
- Khanal, U., Wilson, C., Hoang, V. N., & Lee, B. (2018). Farmers’ adaptation to climate change, its determinants and impacts on rice yield in Nepal. Ecological Economics, 144, 139–147. https://doi.org/10.1016/j.ecolecon.2017.08.006
- Krejcie, R. V., & Morgan, D. W. (1970). Determining sample size for research activities. Educational and Psychological Measurement, 30(3), 607–610. https://doi.org/10.1177/001316447003000308
- Kunreuther, H., Gupta, S., Bosetti, V., Cooke, R., Dutt, V., Ha-Duong, M., Held, H., Llanes-Regueiro, J., Patt, A., Shittu, E., & Weber, E. (2014). Integrated risk and uncertainty assessment of climate change response policies. In Climate Change 2014: Mitigation of Climate Change. Contribution of Working Group III to the Fifth Assessment Report of the Intergovernmental Panel on Climate Change [O. Edenhofer, R. Pichs-Madruga, Y. Sokona, E. Farahani, S. Kadner, K. Seyboth, A. Adler, I. Baum, S. Brunner, P. Eickemeier, B. Kriemann, J. Savolainen, S. Schlömer, C. von Stechow, T. Zwickel and J. C. Minx, Eds.]. Cambridge: Cambridge University Press.
- Kurukulasuriya, P., & Rosenthal, S. (2013). Climate change and agriculture: A review of impacts and adaptations. Agriculture and Rural Development Department, World Bank. https://openknowledge.worldbank.org/handle/10986/16616
- Lin, C. T. J., Jensen, K. L., & Yen, S. T. (2005). Awareness of foodborne pathogens among US consumers. Food Quality and Preference, 16(5), 401–412. https://doi.org/10.1016/j.foodqual.2004.07.001
- Loboguerrero, A. M., Campbell, B. M., Cooper, P. J. M., Hansen, J. W., Rosenstock, T., & Wollenberg, E. (2019). Food and Earth systems: Priorities for climate change adaptation and mitigation for agriculture and food systems. Sustainability, 11(5). https://doi.org/10.3390/su11051372
- Malla, G. (2008). Climate change and its impact on Nepalese agriculture. The Journal of Agriculture and Environment, 9, 62–70. https://doi.org/10.3126/aej.v9i0.2119
- Manandhar, S., Vogt, D. S., Perret, S. R., & Kazama, F. (2011). Adapting cropping systems to climate change in Nepal: A cross-regional study of farmers’ perception and practices. Regional Environmental Change, 11(2), 335–348. https://doi.org/10.1007/s10113-010-0137-1
- Marquardt, D. W. (1970). Generalized inverses, ridge regression, biased linear estimation, and nonlinear estimation. Technometrics. 12: 591–256.
- Mason, R. L. R.F. Gunst, & J.L. Hess. (1989). Statistical Design and Analysis of Experiments: Applications to Engineering and Science. New York: Wiley.
- McSweeney, C., Lizcano, G., New, M., & Lu, X. (2010). The UNDP climate change country profiles: Improving the accessibility of observed and projected climate information for studies of climate change in developing countries. Bulletin of the American Meteorological Society, 91(2), 157–166. https://doi.org/10.1175/2009BAMS2826.1
- Ministry of Environment. (2010). National adaptation plan of action to climate change. Kathmandu, Nepal.
- Ministry of Finance. (2014). Economic survey. Fiscal Year 2013/14. Kathmandu, Nepal.
- Ministry of Finance. (2018). Economic survey. Fiscal Year 2017/18. Kathmandu, Nepal.
- Morton, J. F. (2007). The impact of climate change on smallholder and subsistence agriculture. Proceedings of the National Academy of Sciences, 104(50), 19680–19685. https://doi.org/10.1073/pnas.0701855104
- Mulwa, C., Marenya, P., Rahut, D. B., & Kassie, M. (2017). Response to climate risks among smallholder farmers in Malawi: A multivariate probit assessment of the role of information, household demographics, and farm characteristics. Climate Risk Management, 16, 208–221. https://doi.org/10.1016/j.crm.2017.01.002
- Nhemachena, C., & Hassan, R. (2007). Micro level analysis of farmers’ adaptation to climate change in Southern Africa (IFPRI Discussion Paper No. 00714). Washington, DC: International Food Policy Research Institute.
- Nhemachena, C., Hassan, R., & Chakwizira, J. (2014). Analysis of determinants of farm level adaptation measures to climate change in Southern Africa. Journal of Development and Agricultural Economics, 6(5), 232–241. https://doi.org/10.5897/jdae12.0441
- Nisengwe, J. F. R. (2016). Risk perceptions, attitudes and climate change adaptation behaviors: A case of farmers in Nyabihu District, Rwanda. Master’s thesis, Michigan State University.
- Piya, L., Maharjan, K. L., & Joshi, N. P. (2012). Perceptions and realities of climate change among the Chepang communities in rural mid-hills of Nepal. Journal of Contemporary India Studies: Space and Society, Hiroshima University, 2, 35–50.
- Piya, L., Maharjan, K. L., & Joshi, N. P. (2013). Determinants of adaptation practices to climate change by Chepang households in the rural mid-hills of Nepal. Regional Environmental Change, 13(2), 437–447. https://doi.org/10.1007/s10113-012-0359-5
- Regmi, H. P. (2020). Climate change and variability: Producers’ perceptions and determinants of adaptation strategies in rice farming in Chitwan, Nepal. Master’s thesis, University of Tennessee. https://trace.tennessee.edu/utk_gradthes/6246/
- Richardson, K. J., Lewis, K. H., Krishnamurthy, P. K., Kent, C., Wiltshire, A. J., & Hanlon, H. M. (2018). Food security outcomes under a changing climate: Impacts of mitigation and adaptation on vulnerability to food insecurity. Climatic Change, 147(1–2), 327–341. https://doi.org/10.1007/s10584-018-2137-y
- Rivers, D., & Voung, Q. H. (1988). Limited information estimators and exogeneity tests for simultaneous probit models. Journal of Econometrics, 39(3), 347–366. https://doi.org/10.1016/0304-4076(88)90063-2
- Shardell, M. and Ferrucci, L. (2016). Instrumental variable analysis of multiplicative models with potentially invalid instruments. Statistics in medicine, 35(29), 5430–5447.
- Singh, K. N. (1997). Agricultural extension education. In Handbook of agriculture [ R.R. Lokeshwar, Eds.]. (5th ed., pp. 668–703). Indian Council of Agricultural Research.
- StataCorp. (2019). Stata statistical software: Release 16. StataCorp LLC.
- Tazeze, A., Haji, J., & Ketema, M. (2012). Climate change adaptation practices of smallholder farmers: The case of Babilie district, East Harerghe zone of Oromia regional state of Ethiopia. Journal of Economic and Sustainable Development, 3(14), 1–12. https://scholar.google.com/scholar?cluster=18176727552038859967&hl=en&as_sdt=0,15
- Teklewold, H., Kassie, M., & Shiferaw, B. (2013). Adoption of multiple sustainable agricultural practices in rural Ethiopia. Journal of Agricultural Economics, 64(3), 597–623. https://doi.org/10.1111/1477-9552.12011
- Thinda, K. T., Ogundeji, A. A., Belle, J. A., & Ojo, T. O. (2020). Understanding the adoption of climate change adaptation strategies among smallholder farmers: Evidence from land reform beneficiaries in South Africa. Land Use Policy, 99. https://doi.org/10.1016/j.landusepol.2020.104858
- Tilahun, B., & Bedemo, A. (2014). Farmers’ perception and adaptation to climate change: Heckman’s two stage sample selection model. Ethiopian Journal of Environmental Studies & Management, 7(2), 832–839. https://doi.org/10.4314/ejesm.v7i2.3s
- Wheeler, T., & Braun, J. V. (2013). Climate change impact on global food security. Science, 341(6145), 508–513. https://doi.org/10.1126/science.1239402
- World Bank Group. (2020). Climate change knowledge portal for development practitioner and policy makers. https://climateknowledgeportal.worldbank.org/download-data
Appendices
Appendix 1
Results of sensitivity test using 20%, 30% and 40% increase of each of institutional factors.
Appendix 2
Correlation between institutional factors and multicollinearity issues
To verify if the three institutional factors (Extension, Training and Information) are positively correlated and thus cause multicollinearity, which compromises the reliability of model estimates, we first estimate the correlation coefficients among Extension (referred as ‘EXT’ in the table), Training and Information below.
Table A1. Correlation matrix of institutional factors.
In general, an absolute correlation coefficient greater than 0.7 among two or more independent variables indicates the presence of multicollinearity, which we do not find among the three institutional variables. To reassure the absence of the multicollinearity, we calculated a conditional index value following Belsley (Citation1991) and variance inflation factor (VIF). We did not find evidence of multicollinearity because condition index value is 9.48, which is not above the threshold level 30, and the mean VIF is 4.4 as shown in the table below. The most common rule of thumb is that VIFs exceeding 10 are signs of serious multicollinearity needing correction (Marquardt, 1970; Mason et al., 1989).
Table A2. Variation inflation factors for variables.
Table A3. Conditional index values for variables.
Appendix 3
Instrumental variables and testing for endogeneity
Rivers and Voung (Citation1988) discuss potential endogeneity issues in the limited dependent variable model. Aryal et al. (Citation2018) follow Rivers and Voung (Citation1988) approach to deal with potential endogeneity issues in the multivariate probit model. We follow the similar approach in our study. Following Rivers and Voung (Citation1988) and Aryal et al. (Citation2018), we first specified the potential endogenous variables (Extension, Training and Information) as a function of instruments and controls variables. Then we use residuals of the first stage regression as an additional control variable in the model to acquire consistent estimates. The first stage regression results indicate that all three instruments are significant in the model (see output screenshots), hence we believe that our instruments are reliable and not weak. If the residuals of the first stage regression are not significant, we do not have an issue of endogeneity (Aryal et al., Citation2018). The residuals of all three potential endogenous variables are not significant in the model and hence we report coefficients without residual terms.
First stage regression results for potential endogenous variables are presented below.
Table A4. Extension: instrument is ethnicity.
Table A5. Training: Instrument is risk-averse producers.
Table A6. Information: instrument is the literacy rate in the family.