Abstract
We provide a comparative analysis of how short-run variations in carbon and energy prices relate to each other in the emerging greenhouse gas market in California (Western Climate Initiative [WCI], and the European Union Emission Trading Scheme [EU ETS]). We characterize the relationship between carbon, gas, coal, electricity and gasoline prices and an indicator for economic activity, and present a first analysis of carbon prices in the WCI. We also provide a comparative analysis of the structures of the two markets. We estimate a vector autoregressive model and the impulse--response functions. Our main findings show a positive impact from a carbon shock toward electricity, in both markets, but larger in the WCI electricity price, indicating more efficiency. We propose that the widening of carbon market sectors, namely fuels transport and electricity imports, may contribute to this result. To conclude, the research shows significant and coherent relations between variables in WCI, which demonstrate some degree of success for a first year in operation. Reversely, the EU ETS should complete its intended market reform, to allow for more impact of the carbon price. Finally, in both markets, there is no evidence of carbon pricing depleting economic activity.
KEYWORDS:
Introduction
Carbon markets are a globally recognized solution for the greenhouse gas (GHG) effect problem, which provide, at the same time, financial opportunities. The aim is to promote the reduction of emissions of GHG where it is the most cost efficient.
The first microeconomic computer simulations of cap-and-trade systems for cities and their emissions were designed by Burton and Sanjour between 1967 and 1970 [Citation1,Citation2] for the US National Air Pollution Control Administration (now called the US Environmental Protection Agency [EPA]'s Office of Air and Radiation). In subsequent years, the first “cap-and-trade” system was implemented, under the Clean Air Act, as part of the US Acid Rain Program, and trading started in 1995. After this first example, in the last 20 years carbon markets have become officially implemented in international trade.
The European Union Emission Trading Scheme (EU ETS) was the first, and it is the largest GHG emission trading scheme in place. The EU ETS was built under the Kyoto Protocol mechanism that considers regional trading schemes. A test phase, EU ETS Phase I, lasted from 2005 to 2008. Phase II, 2008–2012, was the first binding phase in which European countries had to fulfill Kyoto obligations. We are now in phase III – 2013–2020. Although some market aspects have changed (new allocation rules, new gases, new activities and new countries), the market principles remain the same.
Other regional emission markets now exist within the USA, New Zealand, Japan, Australia, Canada and China, among others. In the USA, outside the Kyoto Protocol, carbon trading proposals have not yet been taken up at the federal level – although the Waxman–Markey bill came close. Nevertheless, state action on carbon markets continued, of which an example is the Regional Greenhouse Gas Initiative (RGGI). One of the latest emerging GHG markets is in California, linked to the carbon market of Quebec. It is an important instrument to meet the goal of reaching the state's 1990 GHG levels by 2020, as intended by the Western Climate Initiative (WCI), signed in 2007. The market was designed under Assembly Bill 32 (AB32), but it is commonly called WCI. Because of its novelty, this market has not yet been thoroughly described in any research studies. Therefore, we find it relevant to present the most critical features of the Californian emissions trading scheme and the state's energy demand and supply characteristics.
California is the state responsible for the largest share of the USA gross domestic product, 13% in 2012, corresponding to USD 2 trillion. It is one of the largest economies in the world [Citation101]. On energy, California's consumption of 7858.4 trillion BTU (2011) by end-use sectors comes from natural gas (28%), which is hence the largest energy source in the state, followed by motor gasoline excluding ethanol (22%) and fuels (residual, distillate and other; 21%). In smaller shares we find 11% from net interstate flow of electricity, 7% from biomass and other renewables and, finally, 5% from nuclear electric power, 5% from hydroelectric power and 1% from liquid petroleum gas (LPG). Final electricity use totals 21%. On the production of primary energy, California produced around 2624.5 trillion BTU, 2011. Having the largest oil fields in the USA, crude oil and natural gas account for 43% and 11% of California's energy production. Fifteen percent from nuclear electric power and 32% for renewables complete the share.
California's electricity system generates more than 200,000 GWh per year. The current shares of generation per energy source include approximately 63% natural gas, 9% hydroelectric, 18% other renewables, 9% nuclear and 1% coal [Citation102]. In fact, California produces 70% of the electricity it uses. The state imports the remaining amount from the Pacific Northwest (10%) and the US Southwest (20%) [Citation103]. California's challenge on electricity under AB32 is to secure a supply with 33% renewable sources while reducing GHG emissions.
With this energy background, the associated GHG emissions of California come as no surpriseFootnote : of a total of 448 MMT CO2 eq. (million metric tonnes of CO2 equivalent) emitted in 2011, 38% originates in transportation, 23% is from industrial sources, 19% is from electricity generation (10% imported plus 9% in state), 7% is from residential, there is another 7% from agriculture and forestry and 5% is from commercial sectors [Citation104].
California was initially associated with the Western Regional Climate Action Initiative (WRCI), 2007, that aimed to reduce regional GHG emissions to 15% below 2005 levels by 2020, using a cap-and-trade system [Citation105]. However, after the withdrawal of some members, in 2011 the WRCI was materialized in the “Western Climate Initiative” (WCI) [Citation106], a non-profit corporation that helps in the implementation of regional cap-and-trade schemes. California, as a pioneer member of the WRCI, continued its tasks to implement a regional carbon market. Thus, the “California Cap-and-Trade Program” keeps the original WCI milestones. California's emission goal under AB32 is 427 MMT CO2 eq. in 2020 – that is, equaling 1990 estimated emissions [Citation107].
The strategy to achieve the abovementioned reduction (and an 80% reduction in 2050 below 1990 levels) includes the implementation of a cap-and-trade scheme that sets a limit on emitters that are globally responsible for 85% of total GHG emissions reported in the California inventory. Phase 1 of California's carbon market occurred between 2012 and 2014, including, as participants, industrial facilities, electricity generators, electricity importers, and suppliers of carbon dioxide. From 2015 on, suppliers of natural gas, reformulated blendstock for oxygenate blending (RBOB – gasoline), distillate fuel oil, and liquefied petroleum gas are also included. The scheme only covers entities that emit more than 25 kt of CO2. After 2015, sectors included will cover approximately 85% of California's total GHG emissions. In contrast, in the EU ETS, road transport-related activities and electricity imports are not accounted. The European scheme, however, includes the aviation sector, which adds up to almost all energy-intensive industries, and power and heat generation that also participate.
On initial allowance distribution, in California, investor-owned utilities (generators) must hand over the allowances they receive for auctioning. Public and regulated electric utilities, as well as industrial facilities and natural gas distributors, have initially free allocation, to benefit ratepayers. The free allocated amount decreases over time. In the EU ETS, in phase II (2008–2012), there was a free allocation of allowances for nearly 100% of participants. However, in 2013, at the beginning of phase III, about 40% of total allowances were auctioned. The electricity, manufacturing and aviation sectors have different allocation rules. Electricity generators have 100% auctioning, but temporary free allocation is allowed in eight Member StatesFootnote for development reasons (States with a gross domestic product [GDP] per capita below 60% of the EU average may also continue giving free allowances to the energy sector up to 2030).
Central market mechanisms of the California cap-and-trade not considered in the EU ETS are the “cost containment mechanisms” or, more precisely, the allowance auction design, which includes a price floor and an allowance price containment reserve (APCR), to accommodate high peaks in the carbon price. Auctions of California carbon allowances (CCAs) will happen quarterly, and started with a minimum price of USD 10 in 2012, rising 5% annually over inflation. The APCR is a strategic reserve of up to 7% of all emitted permits. These permits will be available for sale at auction dates, priced at USD 40/45/50 in 2013, which will also increase by 5% per year above inflation (i.e., in real terms). Because these sales are set at predefined prices, a measure of knowledge and stability is provided to the market. The APCR acts, thus, as a price volatility control mechanism. The EU ETS, undergoing a reform process, established a market stability reserve (MSR) that will start operations in 2019. The reserve will immediately cut some EU allowance units (EUAs) to be auctioned when an upper threshold in circulation has been surpassed, adjusting the supply of allowances. It also works in the opposite situation, releasing allowances if the flow goes below a lower threshold. The MSR of the EU ETS mainly differs from the APCR of the WCI market in that the control is activated by the volume of banked credits, whereas in APCR it is activated by allowances' prices.
On the borrowing of licenses from future periods, it is allowed in neither WCI nor EU ETS. However, banking of licenses in the WCI market is allowed, within a limit, based on a multiple of the emitter's annual allowance budget. In the EU ETS, banking was allowed without limitations from phase II to phase III.
Finally, AB32 linked its system with that of Quebec, Canada, on January 2014.
The emission permit unit in the WCI is the CCA. To register CCAs, California uses a registry (the Compliance Instrument Tracking System Service, CITSS) and requests annual reporting and verification. The same happens in the EU ETS (using the Union Registry), among other market rules. CCAs are traded in the Intercontinental Futures Exchange US (The ICE Futures US) [Citation108], a leading trade for commodity markets. Only recently, the full time series for ICE CCA 2013 futures was made available by the Climate Policy Initiative [Citation109]. For this reason, there still isn't any research published on actual California carbon prices.
As shown, although both systems are emission trading schemes, the WCI and EU ETS markets have different structures. These differences cause various participants to act in different ways, although with the same goal of efficiently spending their carbon allowances.
Under a cap-and-trade system, emission prices are of the utmost importance. They reflect the “price of pollution,” or the marginal cost of abatement, providing actors with ongoing incentives for technological changes that reduce emissions. As carbon prices represent the equilibrium between demand and supply of emission permits, they are the mirror of abatement decisions. From a more macroeconomic perspective, the carbon price also conveys useful information relevant to climate policy, which can thus consciously decide on the overall emissions goal [Citation4]. Therefore, it is no surprise that a large part of the studies on carbon markets are focussed on price variations, determinations and effects.
The Granger causality test, which tests whether one time series is useful in forecasting another, was initially the most common methodology used to understand the relation between carbon prices and other variables. However, authors using this approach usually only consider a one-way influence of variables, such as the effect of changes in power or gas prices on carbon prices [Citation5,Citation6]. More recent studies use vector autoregressive (VAR) models to detect and overcome the endogeneity problem, usually present in time series analysis, that happens when variables are dependent on each other. They estimate the impact of shocks in energy or carbon prices from a dynamic perspective, considering that all variables influence all others simultaneously [Citation4,Citation7–10]. Kumar et al. [Citation10] apply this methodology to stock prices of clean energy firms, and oil and carbon markets, and Aatola et al. [Citation4] look at the impacts of changes in electricity prices. Finally, Gorenflo [Citation11] also relies on a VAR model to study the lead–lag relationship between spot and futures contracts of carbon allowances, using data for 2006 and 2007.
Most carbon market research has been built mainly on data from the EU ETS. Due to the abovementioned recent availability of CCA prices, to the best of our knowledge, there is no previous econometric analysis of California carbon market prices. Previous studies of WCI have focused on market design features. For example, Fischer et al. [Citation12] proposed the use of auctions for allowance allocation and, later, Peace and Juliani [Citation13] modelled the Lieberman-Warner Bill. A topic that has received more attention is the consideration of electricity imports when accounting GHG emissions, to prevent carbon leakage – an export of production, and therefore emissions, to regions with fewer constraints on GHG emissions. Other authors compare GHG accounting at the load-serving level, using a “first-seller” approach and pure source-based system, which means considering different accounting of emissions from electricity [Citation14–16]. Finally, Thurber and Wolak [Citation17] simulate impacts of high-carbon-price conditions. Other sectoral features of the California carbon market and climate policy have been studied by Sivaraman and Moore [Citation18], who looked at the impact of carbon pricing on photovoltaic (PV) systems, and Fine et al. [Citation19] who studied the result of avoided expenditures originated by gasoline and diesel fuel price spikes due to the existence of a carbon market. In short, California studies look at sectoral aspects of the energy market and impacts of an emissions constraint or carbon price.
Previous results from EU ETS studies confirm the relation of carbon prices with economy fluctuations [Citation8,Citation20]. Causality is found from electricity and gas to carbon prices, and some from carbon and gas to electricity [Citation4,Citation5,Citation7,Citation21,Citation22].
Looking at European data from 2008 to 2014, and California data from 2014 to 2015, we characterize the carbon price interrelation with the most relevant variables influencing this market: energy prices and the economy context. We also include temperatures to serve as a proxy for energy demand, in line with Keppler and Mansanet-Bataller [Citation5]. We specify a dynamic VAR model, which is usually used to analyze and display interdependencies between different time series. With our model, we can estimate impulse–response functions (IRFs) that show the response of carbon prices to impulses in other variables, and the responses of energy prices to impulses in carbon prices, in terms of duration, direction and magnitude.
Our purpose in this paper is to delve into carbon price dynamics. The values of this work are threefold: a first analysis of variations in carbon prices in the new market of California, not previously addressed by other authors. Second is a comparative study of the dynamic interaction of carbon prices with energy prices, between the recent California carbon market, and the EU ETS, an established market. Previous studies on this subject, made for the EU ETS, are limited in terms of variables and temporal dimension. Third, we perform an initial comparative analysis of the structures of the two markets. This information, when related to the interaction of prices in each market, allows us to draw policy conclusions. The results are statistically significant and relevant, and will improve the quality of decision-making of all parties involved in the energy and carbon markets – polluters and regulators included.
This paper proceeds as follows. The second section provides a brief description of the data used and of the applied methodology, and the third section reports and discusses the empirical results. The fourth section concludes.
Data and methods
Selected variables
Controlling GHG emissions through markets implies that emissions are limited, have a price and may be exchanged. The initially imposed overall limit is the fixed emissions allowance supply. Therefore, in the short run, the expected price drivers for emission allowances are on the demand side. This demand for allowances depends on the main emitters' activity, and variables that affect their production. In our specification, that means that the variables to include in the model should be energy prices, the overall economic activity and weather variables.
In the EU ETS, the main emitters include electricity power plants, and cement, steel, glass and paper industries. WCI also includes these energy-intensive and/or high-emission sectors, as explained in the introductory section. However, a significant difference between the WCI market and the EU ETS is in regards to the inclusion of petroleum products and natural gas suppliers, which are not considered in Europe. Also, electricity imports are included in the California trading based on their primary energy source mix. Finally, transport and heating fuel distributors are also included in the market, since January 2015.
Both CA and EU ETS market features and previous work on carbon price causality [Citation4,Citation5,Citation7,Citation20,Citation22–24] justify our variable choices. Our model considers six European variables: European carbon price (European Union Allowance or EUA), electricity price, gas and coal prices, average temperature and a European economy stock market index (Economy) that acts like a proxy for the European economy activity. For WCI we consider seven variables from California: California carbon price (CCA); electricity, gas, coal and gasoline prices; the average state temperature; and an economic activity index – the Dow Jones Utility Index (DJU). All of these variables, as well as their sources, are described in the following paragraphs.Footnote
The years 2008 to 2014 are the time length of this study, representing EU ETS phase II (2008–2012) and two years of phase III (2013–2020). Considering this, as the carbon variable we used the EUA spot price, referring to the emission of 1 tonne of carbon equivalent.Footnote Data for carbon was available from February 26, 2008, up to November 1, 2012, from Bluenext,Footnote and prices from November 2012 until December 31, 2014, were collected from Sendecarbon [Citation111]. All of the following EU data were gathered for these dates.
The WCI market started operations in 2012, with emissions compliance from 2013. The unit quoted on the stock exchange is the CCA Vintage Futures and options on these futures, for 2013, 2014, 2015 and 2016. The products are available at the ICE, and their daily rate is published weekly. In this study, we used the available daily series on the CCA Future Vintage 2013 released by Climate Policy Initiative S. Francisco of the ICE data. Data were available from August 29, 2011, to January 1, 2015. All of the following WCI data were gathered for these dates.
Greenhouse gas emissions considered in the European carbon market come from fossil fuel burning and follow a top-down accounting methodology. We included prices for natural gas, coal and electricity in Europe as energy variables. For all, 1-month future contracts were selected. This choice is in line with the established notion that, in energy, future prices lead spot prices essentially due to the difficulty of storage and the consequent ease of shorting.
Regarding EU natural gas prices, we also used the ICE exchange data. As for coal 1-month future prices, they were also retrieved from the ICE database. EU coal prices are cost, insurance and freight (CIF) with delivery in Amsterdam, Rotterdam and Antwerp (ARA). For electricity, the Phelix prices were retrieved from the European Energy Exchange (EEX). These prices reflect the German/Austrian market area and were selected as representatives of the European electricity prices, because Germany is the largest electricity producer in Europe, which, combined with Austria, reached 680TWh of generated electricity in 2011 [Citation112]. Also, correlation levels between Phelix data and other electricity prices (tested for France and the UK) range from 0.87 to 0.95. So, variations presented through Phelix prices should appropriately represent changes in other European electricity prices.
On the WCI market model, following the same reasoning as for the EU ETS model, we include prices of electricity, natural gas, coal and gasoline as variables.
Regarding the electricity variable, we considered the wholesale day-ahead price of SP15 EZ Generation Hub, located in California. The data source is the ICE exchange. Data were retrieved from the US Energy Information Administration (EIA) information page for 10 major electricity trading hubs in the USA, from August 29, 2011, to November 8, 2013 [Citation113]. For natural gas prices, we used Natural Gas Futures Contract 1, or 1-month futures, available from the US EIA [Citation114]. The source is the New York Mercantile Exchange (NYMEX), and the prices are based on delivery at the Henry Hub in Louisiana. As for coal, Powder River Basin Coal Futures, front month, were also collected from the ICE database. Gasoline prices are Los Angeles Reformulated RBOB Regular Gasoline spot prices, available from the US EIA [Citation115]. One-month futures are only available for the New York Harbor area. We selected the LA prices because spot and futures prices have a correlation value of 0.80, and we consider the geographic reason important for this paper.
Noting that industries included in both EU ETS and WCI market models are energy intensive, and thus their production levels are highly associated with general economic growth, we included a variable that mirrored economic activity. This is in line with several previous authors on the subject [Citation5,Citation25,Citation26]. For this purpose, in the EU ETS we considered the FTS Eurofirst 300 Index (E3X.L), available at YahooFinance. It is a capitalization-weighted price tradable index measuring the performance of Europe's 300 largest companies. Daily price returns were included. In the WCI model, we included a stock exchange index as an economy performance proxy. We chose the DJU [Citation116], a stock index that tracks the financial activity of 15 large US utility companies, also available at YahooFinance.
Finally, following Keppler and M. Mansanet-Bataller [Citation5], we consider average daily temperatures as exogenous variables in our model. For the EU model, they were calculated based on the average daily temperatures from regions of seven representative EU countries (Austria, Germany, France, Italy, the Netherlands, Spain and the United Kingdom), retrieved from the European Climate Assessment & Dataset [Citation117]. It is a weighted average considering the population of each region.Footnote The result is a European average daily temperature index. Similar data were included in the California model. Using the Remote Automated Weather Stations (RAWS) USA Climate Archive [Citation119], we gathered average values from seven representative weather stations (Kneeland, Lassen Lodge, Black Diamond, San Jose, La Panza, Oak Creek, Apple Valley). The daily average of these temperatures was then calculated.
In our econometric specification, all variables are in rates of return (first differences of log data). Stationarity of these time series was established by typical tests. Below, in and , we summarize the descriptive statistics of the abovementioned variables, per model.
The vector autoregressive model
VAR models capture the dynamics among several variables considering the influence of their past values. Through a set of IRFs, and after appropriate restriction identification, it is possible to estimate the dynamic response of a variable to innovations in other variables.
There is a substantial number of relevant references on VAR time series models [Citation27–29]. We will simply provide a brief description of the VAR model. We will focus on a first-order VAR, as the generalization for any number of lags is quite straightforward.
Let be a vector of stationary variables (as previously mentioned, in our application, we consider variables in first log differences). The structural VAR model is:
(1)
where we omit the vector of constants and of exogenous variables for expository purposes; is a matrix lag polynomial and
is a vector with the considered variables
. Matrix
reports the contemporaneous relations between all five variables (six, in the WCI model). So the model allows for a feedback effect because variables at time “t” may affect each other. The model assumes that
, called innovations, are white noise. Unfortunately, EquationEquation (1)
(1) cannot be estimated directly, as ordinary least squares (OLS) would render inconsistent estimates.
Pre-multiplying by , the model may be written in a reduced form:
(2)
EquationEquation (2)(2) delivers the VAR in its reduced form and can be estimated by OLS. Note that the
s have zero mean and constant time-independent variances, but their covariances are not zero, meaning that although they are serially uncorrelated, they are correlated across equations. That is, the shocks in the model are correlated.
The final goal is to estimate how carbon prices respond to innovations in other variables. To derive the IRFs, we follow the methodology proposed by Sims [Citation30] and impose short-run identification restrictions.
The idea is to impose restrictions in the error covariance matrix of EquationEquation 2(2) in order to recover matrix
of EquationEquation 1
(1) . These restrictions impose contemporaneous effects of zero in a predetermined direction. By a convenient ordering of the variables, we basically impose that the covariance matrix is lower triangular where the first equation does not consider any other innovation rather than its own, and the second equation considers the second and the first coming from the addition of the first equation and so on, until the last equation considers them all.
Our ordering choices reflect an initial assumption of the direction in which carbon prices capture information on industrial output levels, on effects of mitigation actions, and on economic circumstances. Also, we consider suggestions from previous authors and block exogeneity Wald tests results presented in the following section. All this information enables us to consider the following initial influence between variables, which triangulates the covariance matrix: first, the prices of variables that are less dependent on others and thus are less elastic – primary energies – and, after, the prices of final energies, the economy index and, finally, the carbon price.
To be more precise, we considered, in the initial impact moment, gas being influenced only by its innovation; then coal to have its own innovation and be influenced by the gas innovation; then electricity, following the same reasoning, having its own innovation, and the ones from coal and gas; then economic activity, gasoline (only in the California model), and finally carbon, following the same logic, by the denoted order. Still, we should stress that, in the end, results of both models were robust to different orderings.
We relied on the likelihood ratio test statistic to decide on the number of lags to include in the VAR model. The test points toward the consideration of 16 days/lags in the European case, and five in the Californian, corresponding roughly to 1 week of daily data for WCI and 3 weeks for EU ETS.
After estimating the VAR, we now turn to the impact multipliers and impulse-response functions. An impulse is what is assumed to trigger the dynamic response of the model. The goal is the analysis of the response, or the propagation mechanism, of the variables, in the following time periods. The IRF shows the effect of a specific innovation to variable (
) on the contemporaneous and future values of all variables.
The process to define these functions starts with the estimated “composite” residuals (linear combinations of uncorrelated innovations) and, from them, rebuilds the original innovations
. Following Sims [Citation30], the process involves representing the VAR model as a vector moving average (VMA), where endogenous variables are defined by
shocks. The VMA allows tracking the shock effects.
To see this, note that EquationEquation (2)(2) may be rewritten as:
(3)
where is a matrix polynomial in the lag operator L.
Then, considering that the VAR model is invertible, it is possible to write it as a vector moving average of infinite order (VMA(∞)):(4)
Having the VMA representation, and keeping in mind that , it is possible to estimate the IRF. Given a unit change in innovation “j,” or impulse, the system reaction to a shock is given by individual reactions of variables “i,” which are called responses:
.
Results
Causality and feedback relations between variables
A central question in VAR models is the endogeneity or exogeneity of variables, as discussed in the previous section. In this study, temperature was the only variable considered exogenous a priori. For all other variables, we ran Granger causality/block exogeneity tests to perceive whether any variable should be treated as exogenous. In these tests, a χ2 Wald statistic is given for each equation for the joint significance of each other lagged endogenous variable in the equation, as well as a statistic for joint significance. The results are described in
Figure 1. Granger causality/block exogeneity Wald tests for the EU Emission Trading Scheme (left) and the Western Climate Initiative (right). Dashed or continuous arrows indicate causality at 10% or 5% significance, respectively. CA: California.
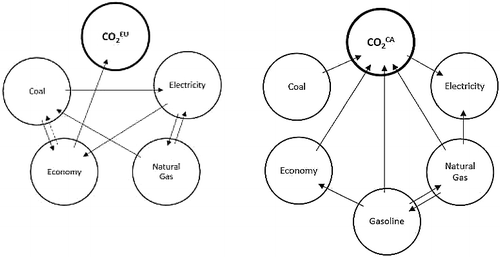
In addition to the results about the endogeneity of the model variables, the VAR Granger causality analysis allows us to make some brief observations. As we may see above in , we found interdependencies in several variables. Recall that the purpose of the Wald test is not to quantify any relation, but instead to identify multiple relations. This analysis allows surpassing the problem of missing variables, present in bivariate causality tests. Additionally, looking at the results of individual and joint significance of lagged endogenous variables, it is reasonable to conclude that no variable should be considered exogenous in this model.
Regarding the EU ETS carbon price, its returns are only significantly caused by the economy returns. Although some authors consider a direct influence of gas and coal prices in carbon prices [Citation7,Citation21], in our EU model, with more data, the natural gas influence becomes indirect. It is, instead, captured through the electricity price. This is possibly due to the surplus of licences that the EU ETS is suffering. On WCI carbon price returns, we find significant direct causality from all variables except electricity. Instead, it is the carbon price returns that show Granger causality toward electricity. This is an indication, in line with the principles of an emissions trading scheme, that states that the carbon price should assemble information from primary energy and the economy, and influence the prices of final energies. Although the relation between carbon and gasoline prices does not follow this idea, it most probably is because the fuel transport sector was only included in the market in 2015.
Also, in the analysis of WCI electricity, gas price has significant explanatory power, a result in line with the findings of Fezzi and Bunn [Citation7] for the EU ETS. This is an adequate result given that natural gas is an important primary energy for thermal electricity production. Natural gas has a lower GHG emission intensity (469 g carbon/kWhe [Citation120]), and more flexible supply, when compared to coal. Another causality relation found of relevance in the WCI is between natural gas and gasoline, in both directions. This is an expected result because both variables are intrinsically related to oil, and their relation is showing this common background.
A final important relation is found between gasoline and the economy. A variable that affects the supply side of most economies is the oil price, so causality from an oil product, such as gasoline, to the economy seems reasonable. Finally, in the WCI market, the economy is also shown to have a role in causing variations in the carbon price returns.
Impulse–response functions
There are a total of 25 (5 × 5 variables) IRFs in the EU ETS model, and 36 in the WCI model. It would be purposeless to show them all. Recalling the goal of this study, to analyze carbon impulses and responses, we will look to the IRFs that show this. For IRF display, we selected the accumulated responses because variables are in first log differences, so the interpretation should be clearer. We also tested for several response periods, and 15 days proved to be enough to show the accumulated response asymptote to a non-zero constant. Also, the WCI market did not show significant IRFs for the first few years of activity. Because of this we only included in the study data from 2014 onward, which show significant relations between variables.
First, we look at the impact that an innovation in primary and secondary energy prices and economy performance has in carbon prices. Second, recalling a carbon market goal of pricing emissions, we analyze the result of a carbon price shock in energy prices.
Carbon response
shows the accumulated response of carbon to one standard deviation innovation in other variables, +/– 2 standard errors. We only present the graphics that show periods of significant influence. They are organized per variable, with the column on the left corresponding to the EU ETS market variables, and the column on the right to the WCI market variables. Note that the last row is an exception, because the economy IRF is not significant in the WCI analysis, and, on the other hand, gasoline was not included in the EU ETS model. All previous rows correspond to a same variable per row.
Figure 2. Accumulated responses of carbon prices to impulses in energy prices and the economy. Continuous line is the impulse–response function; dashed lines are the limits of the 95% confidence interval. EU ETS: European Union Emission Trading Scheme; WCI: Western Climate Initiative.
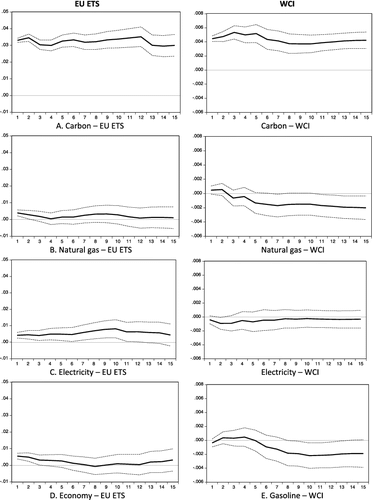
In the first row of , we may see the response of the carbon price to a shock in itself, which results in a distinct positive reaction. It means stakeholders account for previous variations in carbon price when acting in the carbon market, which is a very intuitive consideration for any financial market.
On a more relevant variable, on the second row of , the natural gas IRFs provide us with different results in both markets. In the EU ETS, we see a marginally significant positive impact on carbon returns in the first few periods. This result from the natural gas shock is valid for a market such as the EU ETS that suffers from a surplus of licences. It is also consistent with the definitions of Clean Dark and Spark Spreads that analysts and utilities consider in their decisions for the generation mix. As explained in the data description section, these spreads are linear combinations of electricity, carbon, coal and gas prices, displaying the most cost-efficient option for electricity generation in one period, using either coal or gas power plants. The previous results tell us that changes in the natural gas prices are immediately reflected in the carbon price variation, which, in the case of EU ETS, is a direct positive reflection of variations in energy prices. In contrast, the results from the WCI show us a different result, where we note a significant negative response after the second week that does not fade over time. This result reinforces the idea that when there is a decrease in the price of fossil fuels, carbon prices may rise because the demand for fossil fuels may increase. This result is more in line with an emission trading scheme purpose than the EU ETS positive result. It is also related to the fact that fuel transport has been included in the WCI market since 2015, which does not happen in the EU ETS.
In the electricity IRFs, in the third row of , a situation arises that is slightly similar to that of natural gas. Again, in the EU ETS, the impact is significant during 2 weeks, and increasingly positive. By contrast, in California, an impulse from the electricity price has a slightly significant negative result in carbon in just 1 day, sustaining the Granger causality results presented earlier in . The lack of an overall significance may correspond to the fact that during the considered year there was less need for electricity generators to restrain their emissions significantly, as stated in the California Climate Credit [Citation121].
Regarding the role of the economy, presented in the last row of , in the graph on the left, the contemporaneous and the 2nd day impact in EU carbon returns are positive. That is saying that economy and emissions move in the same direction, which is also a known result of this market. In the economy–carbon relation, a positive result is expected. As carbon emissions mostly come from energy-intensive production, and this production is known to be highly related to economy levels, it is anticipated that a positive change in the economy returns has a positive response from carbon returns. However, we do not find any significant response of the WCI carbon price to a shock in the economy variable. While this does not contradict the result in the Granger analysis, it is an unexpected result, which may be related to the novelty of the market.
Looking at the gasoline variable, also in the last row of in the graph on the right, and only considered in the WCI model, we find a significant response of carbon prices to an impulse in gasoline price, after period 8. This is not surprising given that this variable is closely related to the transport sector, which was only included in the carbon market in January 2015. The rationale remains the same, where a negative relation is expected from fossil fuels to carbon prices, similar to the situation of natural gas, and in line with the carbon market goals. Nevertheless, it should be interesting to re-analyze this feature with future data.
As a final note on impacts of energy variables and the economy, on carbon prices, we emphasize the differences between the EU ETS and WCI: results globally show a negative relation of energy prices to carbon in the WCI, but a positive one in the EU ETS, from which we may draw some conclusions regarding a more evident compliance with carbon market principles in the WCI. However, the economy has a significant and positive role in the EU ETS prices, whereas we do not find such a relation in the WCI. This is an important variable that links the carbon market to the sectors indirectly affected by emissions control. We expect the lack of significance in the WCI results to be related to the novelty of the market, and, thus, temporary.
Carbon impulses
Once more, accumulated responses were selected, and a 15-day response period for the models was considered for the same reasons. In , we represent the second view in the interaction between carbon and energy prices. It shows the accumulated significant response of electricity variables to one standard deviation innovation of carbon returns, +/– 2 standard errors. Following emission market fundamentals, we should anticipate an immediate and positive impact of carbon in the final energies.
Figure 3. Impulses in carbon prices and accumulated responses of selected EU ETS and WCI prices. Continuous line is the impulse–response function; dashed lines are the limits of the 95% confidence interval.
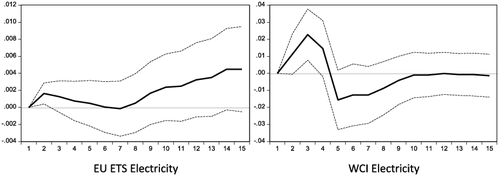
In this carbon-impulse analysis, we find two significant results in the EU ETS, of electricity and coal, and one in the WTI, of electricity. On both EU and California electricity price returns, the carbon impact is only significant in initial days (2nd and 3rd for the EU and California, respectively) and shows positive values, larger in California than in the EU, and becoming insignificant afterward. This means that a positive change in carbon returns causes a positive change in electricity returns. The electricity price reaction is coherent with previous European causality studies [Citation5], and also consistent with market fundamentals, which justify that when the emission price increases, then the cost of producing electricity will also increase because of its inelasticity of demand. The difference in the magnitude of responses between the EU ETS and WCI, which, although small, is 10 times stronger in the WCI, is acceptable because WCI includes all generated and imported electricity emissions. These imported electricity emissions are accounted considering their fuel mix, which includes fossil fuels. Considering that the electricity generation mix is more carbon intensive in the California data than in that considered for our EU model, it was expected that a shock in carbon would affect the WCI electricity price more. This WCI result is also in line with the causality information from , and allows us to consider that the WCI market helps the goal of controlling emissions by effectively penalizing energy consumption. The signal transmission from carbon prices to electricity prices that we show, in a new carbon market such as the WCI, is an important result from this study. In parallel, in the EU ETS, we may also consider that carbon price is on the right path for influencing carbon emissions, although lacking in magnitude.
We also found a slightly significant negative influence of carbon on coal price returns in the EU, in periods 3 and 4. However, this result is very implausible in energy markets, given the physical features of the coal market, and, also, it is not consistent with the previous Granger causality analysis. Given the likelihood that this is a statistical coincidence, we excluded it from and leave it for further studies.Footnote
Concluding remarks
Carbon markets have multiplied around the world in recent years, and are now one of the key policies to fight climate change. Because of this, research on prices of carbon financial assets drew interest, as did the number of polluting facilities facing a GHG emissions cap. Industries covered by GHG markets need to model carbon price variations, on a daily basis. For them, the carbon price is necessary information to manage their portfolio optimization, production costs and risk management in investment decisions [Citation31–33]. Concerned with markets' efficiency, regulators also have an interest in this information.
In this paper, we considered two markets in the analysis: the oldest and largest EU ETS and a first analysis of the carbon prices in WCI, the emerging California emission market. We characterized the EU ETS and the WCI carbon market features, and their short-run relation between carbon prices, energy prices and an economic activity index. We estimated a VAR model considering all variables endogenous, and included temperature as the only exogenous variable. For the EU ETS model, we used daily data from phase II and two years of phase III (2008–2014).Footnote For the WCI market model, we used available data from the compliance period (2014–2015).
Our main findings from the European model are, overall, in line with the situation of a surplus of licenses in the market. On causality, only the economic context shows influence on carbon prices; at the same time, carbon prices do not Granger cause variations in any variable. However, in the IRF dynamic information, taking into consideration all variables roles, we find a significant impact of a carbon price shock on the EU electricity price. When looking into this particular result in the WCI market, we see a similar positive impact from a carbon shock toward electricity, coherent with the WCI model causality result. However, the response of the electricity price is much larger than that of the EU electricity price. Therefore, we may say that even though electricity prices in both markets are responding in the expected direction, the WCI market is gaining in magnitude. Considering that the larger the response in electricity prices, the more efficient the carbon pricing, we may consider that the inclusion of more sectors under the trading scheme may contribute to a more efficient carbon market. The WCI market has a larger scope, including more sectors and emitters than the EU ETS, namely fuel transporters and electricity imports.
On carbon price returns, results globally show a negative relation from energy prices to carbon in the WCI, but a positive one in the EU ETS. There is a primary idea that when fossil fuels prices decrease, the carbon price should increase in response to the possible increase of demand for carbon licences. Considering this indication, we are induced to conclude that the WCI more evidently complies with carbon market principles. This is also possibly related to the low carbon prices in the EU ETS, not limited by any price control mechanisms in the EU ETS, contrary to the top and floor price limits that are in place in the WCI market. In the EU ETS situation, the results do not show an actual response of carbon due to an increase in licenses demand, but only reflect the idea that the EU carbon price is a composite of various energy prices.
On the economy activity variable, we find a significant and positive role in the EU ETS prices, whereas we do not find such a relation in the WCI. This is an important variable that links the carbon market to the sectors indirectly affected by emissions control. Probably, the lack of significance in the WCI results is related to the novelty of the market, and, thus, is temporary.
Overall, although significant relations were found in both markets, consistent with their features and situations, markets could be more efficient if results were larger than the ones obtained. Considering problems such as carbon leakage and surplus of licences, a control of licence quantity in the market is mandatory, and free allocation should be avoided when possible. In the case of WCI, customers receiving their climate credits should save them, to accommodate an expected increase in electricity and gasoline prices, as proposed by the results. It should be an indirect way of supporting an increase in energy prices, without tampering with the climate goal. Regarding the EU ETS, the consideration of fuel suppliers in the market may be a next step.
Finally, there is no evidence in any of the models that penalizing carbon emissions depleted economic activity in the time frame considered. However, this is a relation that should be assessed in the longer term, as done by Sousa et al. [Citation34].
It is important to note that this study was conducted using data from 2008 to 2014–2015, meaning that the current economic and financial crisis has possibly influenced our results. It will be interesting to complete this analysis with an analysis from other carbon markets, namely with different operation and accounting methodologies and economic conditions.
To conclude, looking at our results, we consider that the WCI market demonstrated a successful first year in operation. Conversely, the EU ETS has to fulfill its intentions of a market reform to allow for a more evident control of energy prices.
Acknowledgements
We thank the ICE for providing us the data for natural gas and coal used in this paper. We thank EEX for providing the data on electricity prices used in this paper.
Funding
This work was carried out within the funding with the UID/ECO/03182/2013 reference with the FCT/MEC's financial support through national funding and when applicable co-funded by the FEDER under the PT2020 Partnership Agreement.
Table 1. Descriptive statistics of EU ETS selected variables.
Table 2. Descriptive statistics of WCI selected variables.
Notes
1 Methodologies used in the inventory are consistent with the 2006 Intergovernmental Panel on Climate Change (IPCC) guidelines, and use global warming potential values from the IPCC AR2 [Citation3].
2 Bulgaria, Cyprus, Czech Republic, Estonia, Hungary, Lithuania, Poland and Romania.
3 We did not consider variables as the Clean Spark Spread, the Clean Dark Spread or the “carbon switch” [Citation5], because they are linear combinations of variables already included.
4 EUA future prices were not included because of a spot-future high correlation level (99%).
5 Although Bluenext permanently closed its spot and derivatives trading operations as of December 5, 2012, the environmental trading exchange has hosted the largest number of spot trades, totalling 29.4 million tons (in 2012) [Citation110].
6 Following the methodology used by Tendances Carbone for the European Temperature Index [Citation118].
7 This result is, obviously, available upon request from the authors.
8 Restricting our analysis to 2008–2012 would yield similar results. These results are available upon request.
References
- Burton ES, Sanjour W. A simulation approach to air pollution abatement program planning. Socioecon. Plan. Sci. 4(1), 147–159 (1970).
- Burton ES, Pechan EH, Sanjour W. Solving the air pollution control puzzle. Environ. Sci. Technol. 7(5), 412–415 (1973).
- IPCC. IPCC Second Assessment - Climate Change 1995. Cambridge, NY and Melbourne, Cambridge University Press (1996).
- Aatola P, Ollikainen M, Toppinen A. Price determination in the EU ETS market: theory and econometric analysis with market fundamentals. Energy Econ. 36, 380–395 (2013).
- Keppler JH, Mansanet-Bataller M. Causalities between CO2, electricity, and other energy variables during phase I and phase II of the EU ETS. Energy Policy 38(7), 3329–3341 (2010).
- Creti A, Jouvet P-A, Mignon V. Carbon price drivers: phase I versus phase II equilibrium? Energy Econ. 34(1), 327–334 (2012).
- Fezzi C, Bunn DW. Structural interactions of European carbon trading and energy prices. J. Energy Mark. 2(4), 53–69 (2009).
- Chevallier J. A model of carbon price interactions with macroeconomic and energy dynamics. Energy Econ. 33(6), 1295–1312 (2011).
- Pinho C, Madaleno M. CO2 emission allowances and other fuel markets interaction. Environ. Econ. Policy Stud. 13(3), 259–281 (2011).
- Kumar S, Managi S, Matsuda A. Stock prices of clean energy firms, oil and carbon markets, A vector autoregressive analysis. Energy Econ. 34(1), 215–226 (2012).
- Gorenflo M. Futures price dynamics of CO2 emission allowances. Empirical Econ. 45(3), 1025–1047 (2013).
- Fischer C, Kerr S, Toman M. Using emissions trading to regulate US greenhouse gas emissions: an overview of policy design and implementation issues. Natl. Tax J. 51, 453–464 (1998).
- Peace J, Juliani T. The coming carbon market and its impact on the American economy. Policy and Society27(4), 305–316 (2009).
- Bushnell JB. The Implementation of California AB 32 and its Impact on Wholesale Electricity Markets. CSEM Working Paper. UC Berkeley, Center for the Study of Energy Markets, University of California Energy Institute, UC Berkeley. 170 (2007). Available from: http://www.escholarship.org/uc/item/1qw1c912 (accessed 1 February 2014).
- Bushnell J, Chen Y. Allocation and leakage in regional cap-and-trade markets for CO2. Resour. Energy Econ. 34(4), 647–668 (2012).
- Bushnell J, Chen Y, Zaragoza-Watkins M. Downstream regulation of CO2 emissions in California's electricity sector. Energy Policy 64, 313–323 (2014).
- Thurber MC, Wolak FA. Carbon in the classroom: lessons from a simulation of California's electricity market under a stringent cap-and-trade system. Electr. J. 26(7), 8–21 (2013).
- Sivaraman D, Moore MR. Economic performance of grid-connected photovoltaics in California and Texas (United States): the influence of renewable energy and climate policies. Energy Policy 49, 274–287 (2012).
- Fine J, Busch C, Garderet R. The upside hedge value of California's global warming policy given uncertain future oil prices. Energy Policy 44, 46–51 (2012).
- Alberola E, Chevallier J, Cheze B. Emissions compliances and carbon prices under the EU ETS: a country-specific analysis of industrial sectors. J. Policy Model. 31(3), 446–462 (2009).
- Mansanet-Bataller M, Pardo A, Valor E. CO2 prices, energy and weather. Energy J. 28(3), 73–92 (2007).
- Lutz BJ, Pigorsch U, Rotfuß W. Nonlinearity in cap-and-trade systems: the EUA price and its fundamentals. Energy Econ. 40, 222–232 (2013).
- Sijm J, Chen Y, Hobbs BF. The impact of power market structure on CO2 cost pass-through to electricity prices under quantity competition – a theoretical approach. Energy Econ. 34(4), 1143–1152 (2012).
- Nazifi F. Modelling the price spread between EUA and CER carbon prices. Energy Policy 56, 434–445 (2013).
- Alberola E, Chevallier J. European carbon prices and banking restrictions: evidence from phase I (2005–2007). Energy J. 30(3), 51–79 (2009).
- Chevallier J. Carbon futures and macroeconomic risk factors: a view from the EU ETS. Energy Econ. 31(4), 614–625 (2009).
- Tiao GC, Tsay RS. Model specification in multivariate time series. J. R. Stat. Soc. B 51, 157–213 (1989).
- Amisano G, Giannini C. Chapter 1: from VAR models to structural VAR models. In: Topics in Structural VAR Econometrics. Springer–Verlag GmbH, Heidelberg, 1–28 (1997).
- Enders W. Applied Econometric Time Series. John Wiley & Sons, Danvers, MA (2010).
- Sims CA. Macroeconomics and reality. Econometrica 48(1), 1–48 (1980).
- Roques FA, Newbery DM, Nuttall WJ. Fuel mix diversification incentives in liberalized electricity markets: a mean–variance portfolio theory approach. Energy Econ. 30(4), 1831–1849 (2008).
- Fuss S, Szolgayová J. Fuel price and technological uncertainty in a real options model for electricity planning. Appl. Energy 87(9), 2938–2944 (2010).
- Tolis AI, Rentizelas AA. An impact assessment of electricity and emission allowances pricing in optimised expansion planning of power sector portfolios. Appl. Energy 88(11), 3791–3806 (2011).
- Sousa R, Aguiar-Conraria L, Soares MJ. Carbon financial markets: a time–frequency analysis of CO2 prices. Physica A, Stat. Mech. Appl. 414(0), 118–127 (2014).
Websites
- United States Department of Commerce, Bureau of Economic Analysis (BEA). U.S. Economic Accounts (2015). http://www.bea.gov ( accessed 1 October 2015)
- U.S. Energy Information Administration. California State Profile and Energy Estimates (2015). http://www.eia.gov/state/data.cfm?sid=CA ( accessed 1 October 2015)
- California Energy Commission. California Electricity Data, Facts, & Statistics (2008). http://energyalmanac.ca.gov/electricity/ ( accessed 1 October 2015).
- Air Resources Board of California Environmental Protection Agency. California Greenhouse Gas Emission Inventory (2015). http://www.arb.ca.gov/cc/inventory/data/data.htm ( accessed 1 October 2015).
- Western Climate Initiative. Western Climate Initiative Program Design (2013). http://www.westernclimateinitiative.org/designing-the-program ( accessed 1 October 2015)
- Western Climate Initiative Inc. Western Climate Initiative, Inc. (2014). http://www.wci-inc.org/ ( accessed 1 October 2015).
- Air Resources Board of California Environmental Protection Agency. Cap-and-Trade Program (2015). http://www.arb.ca.gov/cc/capandtrade/capandtrade.htm ( accessed 1 October 2015).
- Intercontinental Exchange Inc. ICE Futures U.S. - California Carbon Allowance Vintage 2013 Future (2015). http://www.theice.com/products/6747556/specs ( accessed 1 October 2015).
- Climate Policy Initiative. Climate Policy Initiative (2015). http://climatepolicyinitiative.org/ ( accessed 1 October 2015).
- Alessandro Vitelli. (2012). NYSE Euronext to Shut Carbon Market After Failed Auction Bid. http://www.bloomberg.com/news/articles/2012-10-26/bluenext-carbon-exchange-to-shut-after-losing-eu-auction-bid-1- ( accessed 1 October 2015))
- SENDECO2. SENDECO2 (2015). http://www.sendeco2.com/es/ ( accessed 1 October 2015).
- BP. Statistical Review of World Energy (2012). http://www.bp.com/en/global/corporate/energy-economics/statistical-review-of-world-energy.html ( accessed 1 October 2015).
- U.S. Energy Information Administration. Electricity Data Browser (2015). http://www.eia.gov/electricity/data/browser/ ( accessed 1 October 2015).
- U.S. Energy Information Administration. Natural Gas Futures Contract 1 (2015). http://www.eia.gov/dnav/ng/hist/rngc1d.htm ( accessed 1 October 2015).
- U.S. Energy Information Administration. Petroleum and other liquids - Spot prices (2015). http://www.eia.gov/dnav/pet/pet_pri_spt_s1_d.htm ( accessed 1 October 2015).
- S&P Dow Jones Indices. Dow Jones Utility Average - Overview (2015). http://www.djaverages.com/index.cfm?go=utility-overview ( accessed 1 October 2015).
- European Climate Assessment & Dataset. European Climate Assessment & Dataset (2015). http://eca.knmi.nl/ ( accessed 1 October 2015)
- CDC Climat Research. Methodology – Tendances carbone (2013). http://www.cdcclimat.com/IMG//pdf/methodologie_tendances_carbone_en_v8.pdf ( accessed 1 October 2015)
- Western Regional Climate Center. RAWS USA Climate Archive (2015). http://www.raws.dri.edu/ ( accessed 1 October 2015).
- Moomaw W, Burgherr P, Heath G et al. 2011: Annex II: Methodology. In: IPCC Special Report on Renewable Energy Sources and Climate Change Mitigation. Edenhofer R.P.-M.O, Sokona Y, Seyboth K, Matschoss P, Kadner S, Zwickel T, Eickemeier P, Hansen G, Schlömer S, von Stechow C (Eds.). Cambridge University Press, Cambridge, United Kingdom and New York. http://srren.ipcc-wg3.de/report/IPCC_SRREN_Annex_II.pdf ( accessed 18 March 2013).
- California Public Utilities Commission. California Climate Credit (2015). http://www.cpuc.ca.gov/PUC/energy/capandtrade/climatecredit.htm ( accessed 1 October 2015)