Abstract
Presently, climate metrics in life-cycle assessment (LCA) aggregate the emission of greenhouse gases in one impact-triggering instant, rather than accommodating its distribution along a timeline. There have been efforts to make the global warming potential (GWP) metric dynamic to study temporary carbon storage in wood products. In this article, we test the use of dynamic LCA on bioenergy production from Jatropha oil in Mali, which offers temporary carbon sequestration in biomass and soil. We built a life-cycle inventory for land conversion to jatropha after cropland and fallow land. The assessment was made for one and for 10 consecutive rotations, and yielded dynamic emission profiles over the years. We found that dynamic LCA is sensitive to variations in emission over time, and that it resulted in lower GWP compared to the conventional static approach following IPCC rules, except for early times of analysis. But the largest variation occurs within dynamic LCA between readings at different times of analysis. The choice of time of analysis in the dynamic LCA methodology has no theoretical grounds and is therefore subjective. In the particular case of this short-lived product, we did not find an added value for the provoked increase in complexity of LCA.
Introduction
The global warming potential (GWP) is the widely accepted indicator recommended by IPCC since 1990 to convey the impact of greenhouse gas (GHG) emissions on climate change [Citation1,Citation2]. The GWP of a GHG reflects the radiative forcing integrated over a time horizon (TH) of a given GHG relative to the integrated radiative forcing of CO2 (EquationEquation 1(1) ) [Citation3]:
(1)
where = the global warming potential of an emission at time 0 of substance i caused by its radiative forcing in the period between time 0 and time horizon TH; ai = radiative efficiency of substance i; Ci(t) = time-dependent decay of substance i.
In life-cycle assessment (LCA), the impact category GWP is then calculated using as characterization factor of the respective GHGs for a chosen TH (EquationEquation 2
(2) ). The LCA impact category GWP reflects the cumulative effect of all GHGs emitted in a life cycle, by summing the GWPs of emitted GHG by their emitted mass:
(2)
Where GWPITH = the global warming potential impact of all emissions during the life cycle caused by their radiative forcing between time 0 and time horizon TH, assuming that their emissions took place in year 0; gi = cumulative emitted mass of substance i throughout the life cycle.
The GWP is a relatively simple metric whose merits include its transparency and its feature of combining the impact of multiple emission pulses of multiple GHGs in one indicator. However, its design has been the target of criticism. The static approach of time implies that all emissions taking place throughout the life cycle are considered to take place at time 0 of the integration interval of EquationEquation 1(1) . When using available impact assessment methods, the practitioner can close the integration interval at 20, 100 or 500 years (). Opting for a shorter TH emphasizes short-term climate change processes, while a longer TH focuses on longer ones. This is because the TH influences the weight of timing of emissions as it serves as a cut-off point, beyond which radiative forcing is ignored.
Figure 1. Difference between static and dynamic global warming potential approaches relative to emission timing and cut-offs at time horizons of 20, 100 and 500 years, and their link to emission pulses and their radiative forcing. The example is a (A) fictitious life cycle with emission pulses consisting of 50 and 25 kg CO2 (black) at years 0 and 40 respectively, and 2 kg CH4 (light grey) at year 124; (B) depicts the radiative forcing of these emission pulses. (C) The DynGWP and (D) the classic GWP of the life cycle, respectively, where black indicates a climate impact of CO2 emissions, light grey that of CH4 and dark grey of both.
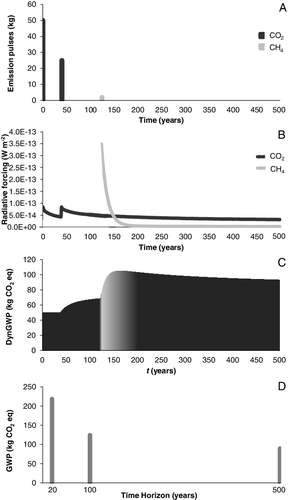
Despite the lack of scientific consensus, a subjective policy-driven choice led to 100 years being the most commonly used TH in GHG accounting and impact studies, including LCA [Citation4]. Regardless of which TH is chosen, the predetermination of 20, 100 and 500 years of time of analysis is perceived as an arbitrary choice [Citation4–6]. In addition, although emission pulses occur at different moments in the life cycle, the GWP does not acknowledge emission timing. Instead, as explained before, it actualizes all emissions to moment 0 of the time of analysis. In addition, the GWP measures the radiative efficiency of that emission pulse in a given interval, beyond which a cut-off is applied. This cut-off point is not related to the de facto duration of the life cycle, as a TH of 100 years can be applied on an emission inventory that spreads over 250 or 600 years, for instance. Hence, the time of analysis is abstract from the temporal boundaries of the life cycle, creating a temporal inconsistency between the method of analysis and the object being analyzed [Citation5,Citation7].
This lack of regard for life-cycle temporal boundaries, allied with the choice of TH, is understood to skew the importance of different emission moments in the LCAs of products with long life cycles because it prevents postponed emissions (e.g., through C sequestration) from being valuated. The significance of emission valuation is explained by the notion that emissions occurring today should be regarded as disadvantageous relative to those occurring in the future, because climate change action ought to be taken as early as possible in order to prevent greater damage [Citation2,Citation8].
Also as a result of its static nature, the GWP LCA category also ignores the changing sensitivity to receiving environments. The rate at which emissions occur and the background concentrations they encounter in the atmosphere will affect their impact on the atmosphere [Citation9,Citation10]. The latter impacts are inherently ignored by the GWP, which has motivated arguments in favor of its replacement with alternative metrics, such as temperature change [Citation2,Citation6,Citation11–14].
There is not yet a consensual methodology to cover those temporal fluctuations, nor to address the issue of TH choice, in LCA [Citation7]. Several authors have come forward with improvements or alternatives to the conventional methodology, either through the use of other metrics or by resolving the GWP in time.
Time-mindful GWP assessments have been proposed by O'Hare and colleagues [Citation15], who oppose the cumulative radiative forcing of a fossil fuel to that of its biofuel substitute. In that study, a comprehensive inventory including GHG emissions from land-use change (LUC) was temporally discriminated through a cost–benefit analysis, thus discounting the value of emissions [Citation15]. Discounting is, evidently, highly sensitive to the discount rate, whose definition is non-consensual. Both International Organization for Standardization (ISO) and UK's Product Carbon Footprint Standard (PAS) guidelines do not accept discounting in LCA [Citation7]. Still, even the use of the classical GWP according to IPCC applies valuing of time preferences, which links to discounting.
Yearly GHG accounting through the entire production chain, including LUC-related emissions, requires measurements in every production-related location and yields a time-specific life-cycle inventory (LCI). An example of such a time-step approach is annual basis carbon accounting, which aims to cover all GHG emissions from source and end-use by making the exhaustive accounting of biogenic and non-biogenic emissions at every year of the life cycle [Citation16]. Although formally presented as an alternative approach to LCA, these annual GHG inventories can rather be seen as the basis of time-specific LCA.
In fact, two other approaches dealing with the static nature of GWP and its use in LCA start from the creation of LCIs in time steps. These methods address the temporal disparity between the GWP's time of analysis TH and the actual duration of the evaluated life cycle. Kendall et al. proposed time-adjusted warming potentials, where emissions are integrated in time between their time of occurrence in the life cycle and a TH. This method maintains the concept of TH as a cut-off but adds THs at 30 and 50 years [Citation17,Citation18].
In 2010, Levasseur and colleagues presented Dynamic LCA, based on the development of the temporally dynamic GWP (henceforth designated DynGWP), which, like the static approach, expresses a radiative forcing per emitted mass of GHG integrated in time of analysis. The crucial difference relative to the IPCC's GWP is that a DynGWP can be calculated for any time t for an emission occurring at a time j, instead of a set of fixed THs for emissions occurring in one single pulse at time 0. This can be seen in the following manner: while the time frame of the time of analysis of the IPCC's GWP consists of three snapshots at 20, 100 and 500 years, the value of DynGWP can be read at any year t of a radiative effect curve up to 2000 years (). In addition, while the IPCC's approach counts all emission pulses as occurring in moment 0 of the time of analysis, DynGWP adds emission pulses to its calculation as they happen ().
DynGWP operates through dynamic characterization factors (DCFs) specific for each GHG and each moment of their decay curve, which are applied on a temporally explicit LCI. The DCFs are analogous to the numerator in EquationEquation 1(1) , as they too consist of the integral over time of the radiative forcing of a GHG. However, the integrated time interval of DCFs runs from the real moment of emission (j) and any year (t) elapsed after it, instead of running between year 0 and a TH as in
(EquationEquation 3
(3) ). The DCF of a GHG is then treated in reference to the radiative forcing of CO2 over the same interval, similarly to the equation describing the
. To calculate a temporally differentiated GWP of a life cycle, DCFS are multiplied by the mass of the emission pulses (g) that have been inventoried in annual time steps (EquationEquation 3
(3) ). Adding up the results yields an annual cumulative GWP at any year up to 2000 years (EquationEquation 3
(3) ):
(3)
Where = the dynamic global warming potential of the life cycle at time t; ai = radiative efficiency of substance i; gi,j = the emitted mass of substance i at time j; Ci(t) = time-dependent decay of substance i.
Dynamic LCA aims to bring consistency between timing of emissions and timing of impact, enabling the differentiation of moments of sequestration and release of GHG emissions, including biogenic C. Hence, it may increase the analytical accuracy of LCA and of valuation of carbon storage. It opened up new debates on decision-making in LCA and introduced new perspectives in the analysis of long life cycles, such as those of forestry or cement products [Citation19–22].
In this article, we evaluate whether dynamic LCA is useful for the case of medium-rotation perennial land-based bioenergy systems generating short-lived products, and how this is affected by the length of the period of land-use occupation. Our case study is a jatropha plantation in central Mali yielding oil for energy generation. We investigate whether the choices of TH on the one hand, and between conventional and dynamic GWP calculation on the other hand, affect the LCA results of a perennial crop. Previous research on this case and methodological duality, assuming a simple one rotation-length life cycle and no emissions from LUC, suggested that TH is the single most important factor to be considered [Citation23]. Here we test these conclusions with the addition of emissions from LUC and for various durations of the life cycle, and therefore short (20 years) and long (more than 200 years) land-occupation periods. The hypothetical scenarios represent two arbitrary periods of one and 10 rotations, respectively, occurring on the same land area in succession. Since there is no clear consensus on the extent of temporal boundaries in the LCA of bioenergy projects, it was here considered that the boundary runs from land clearing at the beginning of one rotation to land clearing at the end of that or the nine following rotations, respectively. Revegetation at the end of cultivation was therefore excluded. The scenarios also compare the conversion of cropland and of fallow land to jatropha.
Methods
Scope and system definition
The system under investigation simulates a hypothetical cradle-to-grave configuration for electricity generation from jatropha-based biodiesel in the town of Koulikoro, Mali. The production chain described here simulates a production system based on operational conditions previously reported for Koulikoro [Citation24].
The cradle-to-cradle system consists of three main stages: cultivation, biofuel production and biofuel use. Cultivation includes the operation of mechanically removing previous vegetation and plowing, and the production and use of fertilizers and pesticides. Weeding and harvesting are done manually. Cultivation takes place in the vicinity of the facilities where extraction of oil takes place. These latter operations include required infrastructure and electricity from local, fossil diesel-based generation. System boundaries include the production of inputs to the system and direct emissions from fertilization, burning fossil diesel for electricity in generators and also tailpipe emissions from the combustion of jatropha oil in generators (Supplementary material, Table S1). The transport of system inputs imported to Koulikoro and the distribution of the oil to end users are excluded. The LCA model was parameterized with direct observations and data from the literature, ecoinvent® version 2.2 (Ecoinvent Center, Switzerland) and GREET 2013 (Argonne National Laboratory, USA) (Table S2).
Allocation to by-products was avoided by system boundary expansion. It was considered that jatropha seed cake, the by-product from oil extraction, avoids the production of N fertilizer, on the basis of equivalent N content.
Life cycle inventory
The GHG emissions related to land use were part of the analysis, including CO2 fluxes related to sequestration/release cycles in biomass and soil. It was considered that a decrease in the stock of the soil and biomass pools between two consecutive years consists of an emission, whereas an increase implies sequestration. Two direct LUC scenarios were evaluated, i.e. jatropha establishment on former cropland and on fallow land.
Carbon sequestration in jatropha roots and stems was assumed to follow the growth curve published by Achten et al. [Citation25] to a maximum C stock of 10.23 t ha–1 when the plantation is 20 years old. The biomass C stocks of adjacent cropland and fallow land [Citation26] were used as stand-ins for previous land use. This study observed that in both land uses, there are trees (e.g. shea trees – Vitellaria paradoxa) that are not felled due to their cultural and economic value. Since fallow land in this location remains undisturbed for long time periods, it accumulates more tree biomass. We assumed that in jatropha plantations the trees present in cropland remain, and that the trees present in fallow land are felled to the same tree density found in cropland. Hence, it was assumed that the conversion from cropland does not entail a C emission, and that the conversion from fallow entails a loss of 6.47 t C ha–1 in the shrub layer and 18.22 t C ha–1 in the tree layer [Citation26] (Table S3).
Soil C stock changes were modeled with RothC version 2.1 [(Coleman and Jenkinson 2008), which has been successfully tested in the Sahel [e.g., Citation29,Citation30]. The model was used to simulate the effect of land conversion from fallow land and from cropland to jatropha, and the land occupation with jatropha. This was done by doing two separate runs, one for the conversion of fallow land and another for the conversion of cropland, starting the model with one of the initial land uses, changing the land cover to jatropha and maintaining jatropha for one or 10 rotations.
RothC requires the input of soil parameters, litter fall and manure inputs for all land uses and climate data. The initial soil C stocks and the clay contents of cropland and fallow land conversion scenarios were approximated by the soil organic carbon (SOC) levels and clay fraction reported for, respectively, cropland and fallow in Koulikoro at 30 cm depth [Citation26] (Table S3). The remaining soil parameters (microbial biomass, decomposable and resistant plant material, and humified and inert organic matter) were estimated with pedotransfer functions designed for use with RothC [Citation30,Citation31]. Total annual C input from fresh leaf litter (and manure on cropland) in the initial land use was retrieved from the literature (Table S4). Cropland was assumed to consist of pearl millet and maize intercropping obeying the cropping calendar in this region of the Sahel, with soil preparation starting in April and harvesting finishing in December [Citation32,Citation33].
C inputs from leaf litter in jatropha plantations was assumed to increase proportionally with tree age until canopy closure at 7 years (Table S4). This timing was gauged according to literature sources and expert observations, taking into account that there is little biomass production [Citation24,Citation26,Citation34]. No pruning is done, so litter input pertains only to leaf shedding. Litter fall intensity was estimated with literature data for 2- and 4-year-old tree plantations in a semi-arid environment in India and extrapolated linearly for years 1 to 7 [Citation35]. Its monthly distribution was assumed to consist of one main shedding period after the rainy season (September–November), while the leaves start sprouting with the onset of the rains in May. The soil was considered uncovered from December to April, until the canopy cover closes. Soil was considered to be covered year round from then onward. Climatic data were extracted from the Local Climate Estimator tool [Citation101]. The modeling was set to start in August, when the soil profile is assumed to be at field capacity [Citation36].
The impact assessment was first performed for one rotation and later also for 10 successive ones. For the consecutive rotations the same emissions from the production chain and biomass were used, whereas C fluxes from soil were modeled with RothC in a continuum over 10 rotations.
The inventory was divided into annual time steps, discriminating emission sources, meaning that for each year in the 21-year rotation, there is a set of emissions from land use and from the operations in the production chain (). In the first year of each rotation the land is prepared to establish jatropha in the next year, which means land clearing in the first rotation after fallow, land preparation in the first year after cropland, and destruction of the old plantation in all consecutive rotations. From year 2 until year 21 of each rotation, jatropha is managed during 20 years. In the third year of management, the yield reaches acceptable levels to start the production of biodiesel. A constant harvest of 1 t ha–1 dry seeds was considered for the rest of the rotation length (17 years). This value was extracted from the predicted yields map of Trabucco et al. [Citation37] and is specific for the region of Koulikoro. This yield proxy was used because sufficient yield measurements are not available and because there is no known empirical relationship between yield and biomass growth for jatropha [Citation24]. When successive rotations were analyzed, the above-described calendar of operations was repeated. The exception is the production of equipment and infrastructure, which only occurs once, at the beginning of the life cycle.
Global warming potential calculation
The result of each annual LCI was converted into an inventory of GHGs. For some processes, direct emission factors (Table S1) were used. The majority of processes were extracted from ecoinvent® (Table S2). Their emission factors were calculated from the inventory of airborne emissions function SimaPro® (PRé, the Netherlands). The GHGs included in this analysis were limited to a subset present in both the SimaPro® inventory and the two impact assessment methods used: the IPCC's Fourth Assessment Report (FAR) and the Dynamic LCA calculation tool [Citation3,Citation102]. The most important GHGs present in the inventory are carbon dioxide (CO2), nitrous oxide (N2O) and methane (CH4), while the remaining gases have vestigial emission loads (see Table S5 for the full list).
The static GWP was calculated by multiplying the IPCC's FAR GWP characterization factors [Citation3] of each GHG, and respective to the IPCC GWP time horizon assessment, with its total emitted mass, and is henceforth referred to as IPCC GWP. The DynGWP was calculated with the DynCO2 tool of Levasseur [Citation102]. The functional unit (FU) of this study is 1 MJ yr–1 of electricity produced during the life cycle in a generator consuming 0.09 L oil MJ–1 [Citation24]. Hence, the final impact reflects an emission profile uniformly distributed in time [Citation38].
Repayment times of carbon debts
In an additional analytical step, the repayment times of the four land conversion and use scenarios were calculated. The aim was to evaluate the impact of using a temporally dynamic approach to reach such performance indicator values.
The repayment time is a measure of how long it takes for a land-based biofuel to neutralize its emission from LUC based on the difference between annual life-cycle emissions of a fossil-based FU compared to if the same FU is fulfilled with a biofuel [Citation39]. It was considered that the carbon debt of the system at hand is the sum of the emissions resulting from biomass clearing and from the SOC lost during the establishment of the jatropha plantation. The static and dynamic GWPs of the life cycle of jatropha were calculated as described above, excluding from the emission inventory the C emissions that consist of the C debt. As such, possible emissions from SOC and jatropha biomass occurring during the life cycle are included in the life-cycle emissions. GWP calculation was performed for an analytical time of 100 years only. It was assumed that the GWP of fossil-based electricity is 0.27 kg CO2 eq MJ–1, which was calculated with SimaPro® based on an appropriate ecoinvent® process.
Results and discussion
Greenhouse gas emission inventory
The main contributor to the GHG emissions and the GWP is LUC, regardless of previous land cover. Accordingly, the single largest mass of GHG emitted is CO2, followed by N2O and then CH4. While a temporal LCI of all included GHGs is presented in Table S5, reports only on these three GHGs, showing the contribution of the different intervening processes in the production system in terms of CO2, N2O and CH4 emissions in one rotation. When fallow land is converted, 86% of emissions arise from biomass clearing, and 12% from SOC losses. SOC losses correspond to circa 88% of total GHG emissions of a full rotation when cropland is converted. The next largest source of emissions is oil extraction: 12% in the case of conversion of cropland and 2% in conversion of fallow land (). The fluxes of CO2 to and from jatropha biomass are not shown in as their balance is zero.
Figure 3. Total mass (kg/FU) of CO2, N2O and CH4 emitted by the stages of electricity production from jatropha in one rotation in the case of conversion of cropland and of fallow land.
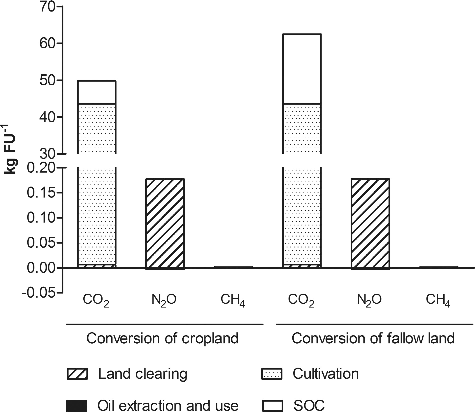
Although these shares are not the main result of this study, they reveal that the emissions from LUC are higher than those of land occupation with jatropha. In terms of the two previous land covers, the overall burden of converting fallow land is greater than the burden of converting cropland, due to the larger initial biomass C stock, which is lost (). This illustrates how sensitive the GWP of a project of this nature is to the land-cover type it replaces.
depicts the evolution of CO2 emissions from soil throughout 10 rotations. These emissions are highest during the first cycle after LUC. This is due to a stage of rapid loss of SOC until the fourth cycle, after which the average SOC in one rotation stabilizes at around 10.5 t C ha–1, regardless of the initial SOC content. This pattern of rapid loss after LUC followed by stabilization has been observed in other studies, as reviewed by Bessou et al. [Citation40]. The overall decline in SOC can be explained by phenomena described in literature included in the modeling inputs [Citation41]. The input of C to the soil from leaf shedding is very low, when compared with wetter climates, for instance. Moreover, there are periods of soil bareness in the beginning of each rotation until the crop reaches maturity, leading to high C losses. CO2 emissions are higher upon the conversion of fallow land due to the higher initial SOC content.
Figure 4. Annual CO2 emission (in t ha–1) from soil of the jatropha plantation during 10 rotations, starting from establishment on cropland (full line) or fallow land (dashed line).
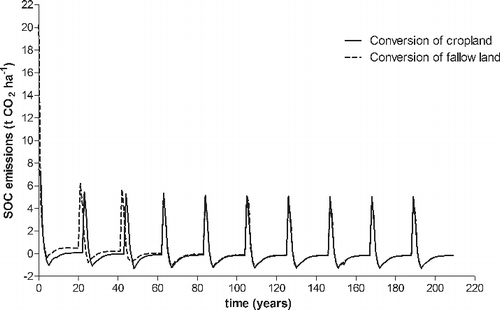
These model outputs have not been validated because a monitoring experiment could not be conducted, but the aim of this simulation was rather to provide indicative values that contribute to the performance of the GWP indicators under study, than to gain in-depth understanding of SOC dynamics under jatropha over time. In addition, because all plantations in the area were young and SOC did not correlate to age, a chronosequence from field measurements could not be derived for a 20-year cycle [Citation26]. However, SOC values measured during a first rotation in fields of several ages fall within the simulated range for the first cycle of 14 to 21 t C ha–1 [Citation26].
The RothC model was chosen due to its robustness, simplicity and low data requirements, all of which match the small body of knowledge available on jatropha cultivation/agronomy. However, it does not consider certain cultivation aspects that possibly influence these results. For instance, soil cover is only represented by a binary value without the possibility to specify its value through time and hence does not reflect spacing, canopy development, variable cover with shed leaves or the presence of understory vegetation. The results are also heavily reliant on assumptions on litter fall data, since it influences how much C is supplied to the soil in jatropha plantations. The lack of field data on the SOC of older plantations and the high spatial variability of such measurements thwart the validation of these results. As such, they should be considered carefully as they are not a thorough assessment of the impact of jatropha on land occupation.
Global warming potential assessment
The charts in show the results of the impact assessment with the IPCC GWP and DynGWP for one and 10 rotation cycles. The IPCC GWP results are calculated and reported for discrete time horizons (20, 100 and 500 years), while the DynGWP is calculated and reported showing continuous curves with a time of analysis to 350 years. The values in correspond to the impact of 1 MJ of electricity generated over an averaged year during the rotation(s') length. For this reason, albeit accumulating more emissions, the GWP of multiple cycles seems to be lower, particularly with dynamic modeling. This is even more evident in the conversion of fallow land. This is because the largest GHG emission pulse, from the lost biomass upon land clearing, smears over the longer life cycle and also decreases considerably along multiple rotation cycles. Within any time of analysis (Table S6), the investment in multiple rotations is more advantageous than in a single one.
Figure 5. Global warming potential (GWP) of Jatropha biodiesel-based electrification upon conversion of cropland and fallow land. (A) GWP according to IPCC and (B) dynamic GWP.
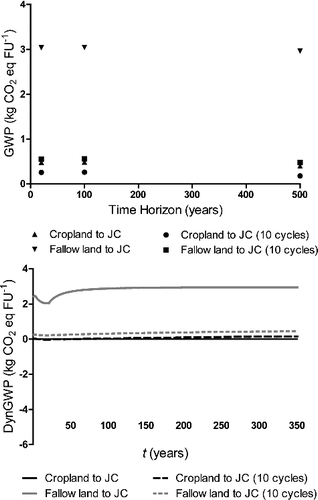
The IPCC GWP varies very little with the different time horizons, being slightly lower at 500 years. On the other hand, the DynGWP decreases with longer times of analysis, plummeting sharply in the first decades of t and stabilizing afterward. The values of DynGWP of the single cycle simulations, after those first decades, are lower than those of the IPCC GWP, while the multi-cycle simulations result in very similar figures throughout (Table S6). The stabilization occurs later in the conversion of cropland than of fallow land. The single largest emission pulse common to all scenarios is the clearing of biomass at the beginning of the plantation. In the single-cycle simulations, it drives the emission peak in the first year followed by a decrease in the DynGWP. This decrease is due to the fact that the sequestration in jatropha biomass offsets the remaining annual emissions, for which reason there is no build-up of GHG concentrations. The decrease is more pronounced in the case of conversion of cropland due to the lower initial emission from biomass clearing. In multi-rotation simulations, successive jatropha clearings are immediately compensated by another round of carbon growth. This irregularity of the emission pulses throughout the years is visible in the DynGWP curves in the small emission pulse from clearing the jatropha plantations. Such curves had not been seen in a previous analysis that excluded the emissions from LUC [Citation23].
This suggests that in the LCA of short-rotation perennials, dynamic LCA can yield lower GWPs in comparison with the classic IPCC estimations. In fact, this discrepancy is inherent to the methods. In the IPCC approach, the net emissions of the entire life cycle reached the atmosphere in the first year and their effect is traceable after 100 and 500 years. Dynamic LCA instantaneously captures the gradual release of GHG alternated with its sequestration. This means that besides the initial peak of GHG release, there is little variation in GHG concentrations originating in this life cycle, in which each emission pulse is partially offset by a moment of sequestration. Because the GHG decay rates and radiative forcings are the same in either methodology, the progression of the DynGWP lags behind.
Effect of emission timing in repayment times
In this bioenergy system, the C debt is not repaid when only one rotation takes place because its life cycle 600 emissions are higher than those of fossil-based energy (). In the case of 10 rotation cycles, considering emission time benefits the performance of jatropha. With DynGWP, the conversion of cropland can be repaid in 16 rather than 130 years and the conversion of fallow land can be repaid in 256 years, while with the IPCC GWP there was no repayment.
Conclusions
From the practitioners' point of view, if standard product profiling is the goal of the LCA, we cannot conclude whether or not this type of life cycle elicits the need to use a temporally specific GWP. The dynamic LCA methodology does not seem to present an advantage in communicating the GWP of this kind of life cycle, since the additional effort does not match an additional gain in results. This is because the dynamic LCA methodology:
Is not fully integrated with available LCA software, which implies the use of an additional, dedicated tool;
Results in greater variation in the scores at different analytical times, i.e. readings taken at different, wide-apart t (such as 20, 100 and 500 years, to match the THs of static GWPs) in dynamic LCA;
Contrarily to what was intended, it does not ease the decision of a cut-off moment;
Increases reporting complexity [Citation21].
In this case study, because it deals with a short-lived product (oil which is consumed immediately for electricity) and because SOC does not accumulate in the plantations, the period of temporary C sequestration in the biomass is short. In this particular case, the practitioner may opt for more immediate metrics, such as the IPCC GWP, which also eases the choice of time of analysis. While this reasoning holds for amortized GHG accounting, which spreads their impact over a time of analysis, it does not address the aim of systematically and readily accounting for the implications of the length of the life cycle and its related land-occupation period. In fact, the effect of choosing a cut-off moment in a time of analysis depends directly on these two aspects. In the specific case of climate-mitigating technologies, such as biofuels, it can be ventured that the carbon debt and repayment time based on a static approach are clearer in communicating the effects of LUC and occupation on the GHG balance of biofuels in time.
However, repayment times must be calculated on the basis of a GWP score, static or dynamic. As seen in the results, considering emission timing can significantly change repayment times. This is in line with the findings of Levasseur et al. [Citation19], although whether or not a dynamic approach defers or shortens repayment times seems to depend on the LCI. Another factor should be the variation of impact scores in different times of analysis herewith reported. Hence, also the adequate use of the repayment of C debts depends on an agreement on the basic GWP assessment methodology.
Nonetheless, dynamic LCA may play a role in the environmental profiling of any type of land-based system in a wider global warming assessment view. Its temporal explicitness matches that of other properties of the land cover, which operate directly on radiative forcing. For instance, there are efforts for recognizing the importance of albedo in climate change as well as for its inclusion in LCA metrics [Citation42–45]. In addition, other temporally dynamic mechanisms are currently excluded from GWP modeling, such as interactions between land cover and land–atmosphere C fluxes, GHG emission rates and the effect of background GHG concentrations [Citation9,Citation10,Citation42,Citation45,Citation46]. A temporally dynamic perspective on GHG accounting is central to realize such inclusive pathways for climate change impact assessment.
Acknowledgements
This work was supported by the Science and Technology Foundation–Portugal [doctoral grant number SFRH/BD/72015/2010] to Joana Almeida.
Table 1. Carbon debt, life cycle emissions and repayment time of the four land replacement scenarios, with the life-cycle emissions assessed with IPCC's GWP and with DynGWP.
References
- Shine KP, Derwent RG, Wuebbles DJ, Morcrette JJ. Radiative forcing of climate. In: Climate Change: The IPCC Scientific Assessment, Houghton JT, Jenkins GJ, Ephraums JJ (Eds). Cambridge University Press, Cambridge, UK (1990).
- Tanaka K, Peters GP, Fuglestvedt JS. Policy update: multicomponent climate policy: why do emission metrics matter? Carbon Manage. 1(2), 191–197. doi:10.4155/cmt.10.28 (2010).
- Intergovernmental Panel on Climate Change (IPCC). Contribution of Working Group I to the Fourth Assessment Report of the Intergovernmental Panel on Climate Change, 2007. Solomon S, Qin D, Manning M et al. (Eds). IPCC, Cambridge, UK and New York, USA (2007).
- Brandão M, Levasseur A, Kirschbaum MUF et al. Key issues and options in accounting for carbon sequestration and temporary storage in life cycle assessment and carbon footprinting. Int. J. Life Cycle Assess.18(1), 230–240. doi:10.1007/s11367-012-0451-6 (2013).
- Levasseur A, Lesage P, Margni M, Deschênes L, Samson R. Considering time in LCA: Dynamic LCA and its application to global warming impact assessments. Environ. Sci. Technol. 44(8), 3169–3174 (2010).
- Kendall A. Climate change mitigation: deposing global warming potentials. Nat. Clim. Chang. 4(5), 331–332. doi:10.1038/nclimate2218 (2014).
- Finkbeiner M, Ackermann R, Bach V et al. Challenges in life cycle assessment: an overview of current gaps and research needs. In: Background and Future Prospects in Life Cycle Assessment. Klöpffer W (Ed.). Springer Science, Dordrecht, The Netherlands (2014).
- Levasseur A, Brandão M, Lesage P et al. Valuing temporary carbon storage. Nat. Clim. Chang. 2(1), 6–8 (2012).
- Reisinger A, Meinshausen M, Manning M. Future changes in global warming potentials under representative concentration pathways. Environ. Res. Lett. 6(2), 024020. doi:doi:10.1088/1748-9326/6/2/024020 (2011).
- Cherubini F, Gasser T, Bright RM, Ciais P, Stromman AH. Linearity between temperature peak and bioenergy CO2 emission rates. Nat. Clim. Chang. 4(11), 983–987 (2014).
- Peters GP, Aamaas B, Lund MT, Solli C, Fuglestvedt JS. Alternative global warming metrics in life cycle assessment: a case study with existing transportation data. Environ. Sci. Technol. 45(20), 8633–8641. doi:10.1021/es200627s (2011).
- Aamaas B, Peters GP, Fuglestvedt JS. Simple emission metrics for climate impacts. Earth Syst. Dyn. 4(1), 145–170. doi:10.5194/esd-4-145-2013 (2013).
- Cherubini F, Bright RM, Strømman AH. Global climate impacts of forest bioenergy: what, when and how to measure? Environ. Res. Lett. 8(1), 014049. doi:10.1088/1748-9326/8/1/014049 (2013).
- Olivié DJL, Peters GP. Variation in emission metrics due to variation in CO2 and temperature impulse response functions. Earth Syst. Dyn. 4(2), 267–286. doi:10.5194/esd-4-267-2013 (2013).
- O'Hare M, Plevin RJ, Martin JI, Jones AD, Kendall A, Hopson E. Proper accounting for time increases crop-based biofuels' greenhouse gas deficit versus petroleum. Environ. Res. Lett. 4, 024001 (2009).
- DeCicco J. Biofuels and carbon management. Clim. Chang. 111(3), 627–640. doi:10.1007/s10584-011-0164-z (2012).
- Kendall A, Chang B, Sharpe B. Accounting for time-dependent effects in biofuel life cycle greenhouse gas emissions calculations. Environ. Sci. Technol. 43(18), 7142–7147. doi:10.1021/es900529u (2009).
- Kendall A. Time-adjusted global warming potentials for LCA and carbon footprints. Int. J. Life Cycle Assess. 17(8), 1042–1049. doi:10.1007/s11367-012-0436-5 (2012).
- Levasseur A, Lesage P, Margni M, Brandão M, Samson R. Assessing temporary carbon sequestration and storage projects through land use, land-use change and forestry: comparison of dynamic life cycle assessment with ton-year approaches. Clim. Chang. 115(3–4), 759–776. doi:10.1007/s10584-012-0473-x (2012).
- Levasseur A, Lesage P, Margni M, Samson R. Biogenic carbon and temporary storage addressed with dynamic life cycle assessment. J. Ind. Ecol. 17(1), 117–128. doi:10.1111/j.1530-9290.2012.00503.x (2013).
- Dyckhoff H, Kasah T. Time horizon and dominance in dynamic life cycle assessment. J. Ind. Ecol. 18(6):799–808. doi:10.1111/jiec.12131 (2014).
- Yang J, Chen B. Global warming impact assessment of a crop residue gasification project: dynamic LCA perspective. Appl. Energy 122(0), 269–279. doi:10.1016/j.apenergy.2014.02.034 (2014).
- Almeida J, Moonen PCJ, Soto I, Achten WMJ, Muys B. Time explicit global warming potential of Jatropha biofuel production in Mali. In: Fulfilling LCA's Promise – Proceedings from the LCA XIII International Conference. Orlando, FL, United States, 68–77, 1–3 October 2013.
- Almeida J, Moonen PCJ, Soto I, Achten WMJ, Muys B. Effect of farming system and yield in the life cycle assessment of jatropha-based bioenergy in Mali. Energy Sustain. Dev. 23, 258–265. doi:10.1016/j.esd.2014.10.001 (2014).
- Achten WMJ, Trabucco A, Maes WH et al. Global greenhouse gas implications of land conversion to biofuel crop cultivation in arid and semi-arid lands – lessons learned from jatropha. J. Arid Environ. 98, 135–145. doi:10.1016/j.jaridenv.2012.06.015 (2013).
- Degerickx J, Almeida J, Moonen PCJ, Vervoort L, Muys B, Achten WMJ. Impact of Jatropha curcas L. plantations on above- and belowground carbon stocks: a field study in Mali. GCB Bioenergy. doi:10.1111/gcbb.12288 (2016) (In Press).
- Coleman K, Jenkinson DS. ROTHC-26.3: A model for the turnover of carbon in soil. Rothamsted Research, Harpenden, UK (2008).
- Takimoto A, Nair VD, Ramachandran Nair PK. Contribution of trees to soil carbon sequestration under agroforestry systems in the West African Sahel. Agrofor. Syst. 76(1), 11–25. doi:10.1007/s10457-008-9179-5 (2009).
- Nakamura S, Hayashi K, Omae H et al. Validation of soil organic carbon dynamics model in the semi-arid tropics in Niger, West Africa. Nutr. Cycl. Agroecosyst. 89(3), 375–385. doi:10.1007/s10705-010-9402-4 (2011).
- Falloon P, Smith P, Coleman K, Marshall S. Estimating the size of the inert organic matter pool from total soil organic carbon content for use in the Rothamsted carbon model. Soil Biol. Biochem. 30(8/9), 1207–1211. doi:10.1016/S0038-0717(97)00256-3 (1998).
- Weihermüller L, Graf A, Herbst M, Vereecken H. Simple pedotransfer functions to initialize reactive carbon pools of the RothC model. Eur. J. Soil Sci. 64(5), 567–575. doi:10.1111/ejss.12036 (2013).
- Shetty SVR, Beninati NF, Beckerman SR. Strengthening Sorghum and Pearl Millet Research in Mali. International Crops Research Institute for the Semi-Arid Tropics, Patancheru, India (1991).
- Assessment Capacities Project (ACAPS). Mali – Food Insecurity Crisis. ACAPS, Geneva, Switzerland (2012).
- Baumert S. Life cycle assessment of carbon and energy balances in jatropha production systems of Burkina Faso [PhD thesis]. University of Bonn (2014).
- Nallathambi Gunaseelan V. Biomass estimates, characteristics, biochemical methane potential, kinetics and energy flow from jatropha curcus on dry lands. Biomass Bioenerg. 33(4), 589–596. doi:10.1016/j.biombioe.2008.09.002 (2009).
- Kaonga ML, Coleman K. Modelling soil organic carbon turnover in improved fallows in eastern Zambia using the RothC-26.3 model. For. Ecol. Manage. 256(5), 1160–1166. doi:dx.doi.org/10.1016/j.foreco.2008.06.017 (2008).
- Trabucco A, Achten WM, Bowe C et al. Global mapping of Jatropha curcas yield based on response of fitness to present and future climate. GCB Bioenerg. 2(3), 139–151 (2010).
- Davis SJ, Burney JA, Pongratz J, Caldeira K. Methods for attributing land-use emissions to products. Carbon Manage. 5(2), 233–245. doi:10.1080/17583004.2014.913867 (2014).
- Fargione J, Hill J, Tilman D, Polasky S, Hawthorne P. Land clearing and the biofuel carbon debt. Science 319(5867), 1235–1238. doi:10.1126/science.1152747 (2008).
- Bessou C, Ferchaud F, Gabrielle B, Mary B. Biofuels, greenhouse gases and climate change. A review. Agron. Sustain. Dev. 31(1), 1–79. doi:10.1051/agro/2009039 (2011).
- Bationo A, Wani SP, Bielders CL, Vlek PLG, Mokwunye AU. Crops residue and fertilizer management to improve soil organic carbon content, soil quality and productivity in the desert margins of West Africa. In: Global Climate Change and Tropical Ecosystems. Lal R, Kimble JM, Stewart BA (Eds). CRC Press, Boca Raton, FL, USA (2000).
- Ollinger SV, Richardson AD, Martin ME et al. Canopy nitrogen, carbon assimilation, and albedo in temperate and boreal forests: functional relations and potential climate feedbacks. Proc. Natl. Acad. Sci. USA 105(49), 19336–19341. doi:10.1073/pnas.0810021105 (2008).
- Muñoz I, Campra P, Fernández-Alba AR. Including CO2-emission equivalence of changes in land surface albedo in life cycle assessment. Methodology and case study on greenhouse agriculture. Int. J. Life Cycle Assess. 15(7), 672–681. doi:10.1007/s11367-010-0202-5 (2010).
- Bright RM, Cherubini F, Strømman AH. Climate impacts of bioenergy: inclusion of carbon cycle and albedo dynamics in life cycle impact assessment. Environ. Impact Assess. Rev. 37(0), 2–11. doi:10.1016/j.eiar.2012.01.002 (2012).
- Port U, Brovkin V, Claussen M. The influence of vegetation dynamics on anthropogenic climate change. Earth Syst. Dyn. 3(2), 233–243. doi:10.5194/esd-3-233-2012 (2012).
- Lammertsma EI, de Boer HJ, Dekker SC, Dilcher DL, Lotter AF, Wagner-Cremer F. Global CO2 rise leads to reduced maximum stomatal conductance in Florida vegetation. Proc. Natl. Acad. Sci. USA 108(10), 4035–4040. doi:10.1073/pnas.1100371108 (2011).
Websites
- Food and Agricultural Organization of the United Nations (FAO). Climpag (2013). www.fao.org/nr/climpag
- Levasseur A. DynCO2 dynamic carbon footprinter (2013). www.ciraig.org/en/dynco2.php