Abstract
Various neuroimaging investigations have revealed that perception of emotional pictures is associated with greater visual cortex activity than their neutral counterparts. It has further been proposed that threat-related information is rapidly processed, suggesting that the modulation of visual cortex activity should occur at an early stage. Additional studies have demonstrated that oscillatory activity in the gamma band range (40–100 Hz) is associated with threat processing. Magnetoencephalography (MEG) was used to investigate such activity during perception of task-irrelevant, threat-related versus neutral facial expressions. Our results demonstrated a bilateral reduction in gamma band activity for expressions of threat, specifically anger, compared with neutral faces in extrastriate visual cortex (BA 18) within 50–250 ms of stimulus onset. These results suggest that gamma activity in visual cortex may play a role in affective modulation of visual processing, in particular with the perception of threat cues.
Cognitive processing resources are preferentially allocated to threat-related cues relative to neutral cues, as the ability to process threatening information rapidly provides potential evolutionary advantages (Davis & Whalen, Citation2001; Vuilleumier & Pourtois, Citation2007). Neuroimaging investigations further reveal that facial displays of threat such as fear or anger engender greater occipital cortex activity in regions such as the lingual gyri when compared to neutral expressions (Noesselt, Driver, Heinze, & Dolan, Citation2005). The sensitivity of the lingual gyri to displays of threat is so acute that it is revealed with highly impoverished displays such as angular shapes that are similar to expressions of anger (Larson, Aronoff, Sarinopoulos, & Zhu, Citation2009). Furthermore, the importance of this region for threat processing is revealed by anatomical studies on nonhuman primates which show it to be one of the few extrastriate regions that has direct afferent connectivity with the amygdala (Amaral & Price, Citation1984). Thus, not only do these findings suggest the presence of highly sensitive mechanisms for the detection of threat but also that such circuitry includes regions of extrastriate cortex (Vuilleumier & Pourtois, Citation2007). To further understand visual processing of threat, investigation of the temporal and frequency dynamics of this activity is necessary. Here, the study of electromagnetic activity by magnetoencephalography (MEG) has proven crucial.
For instance, Ishai, Bikle, and Ungerleider (Citation2006) observed increased MEG positivity in response to faces depicting indirect threat (fear) compared with neutral faces at 230 ms within extrastriate cortex. More recently, Hung et al. (Citation2010) examined the onset of activity in the amygdala and fusiform gyri when subjects were shown task-irrelevant facial displays of fear. It was found that these expressions produced an evoked response in the amygdala at 100 ms post-stimulus onset and in the fusiform cortex a little later at 150 ms. Taken together, such findings again suggest that regions of extrastriate cortex form part of an early (pre-250 ms) visual threat detection network.
Few studies, however, have examined the oscillatory signature of the visual processing of threat, despite some evidence suggesting that gamma activity plays an important role (e.g., Luo, Holroyd, Jones, Hendler, & Blair, Citation2007; Luo et al., Citation2009). Before considering this work, it is helpful to consider the role of gamma in information-processing more generally. Reviewing a number of recent MEG studies, Jensen, Kaiser, and Lachaux (Citation2007) suggest that periods of both neuronal synchrony and/or desynchrony in the gamma-band range are crucial for a variety of complex cognitive processes including attention and working memory. For example, recent evidence from nonhuman primate studies supports the notion that gamma frequency oscillations in visual cortex have a facilitative role in visual perception for attended stimuli (Rotermund, Taylor, Ernst, Kreiter, & Pawelzik, Citation2009). Here, performance in a perceptual task improved as gamma power increased. A similar effect has also been revealed in human subjects with gamma-band power increases demonstrated for voluntary shifts of attention to faces, but not involuntary shifts (Landau, Esterman, Robertson, Bentin, & Prinzmetal, Citation2007). Of further interest, Sokolov, Pavlova, Lutzenberger, and Birbaumer (Citation2004) reported enhancements of induced gamma activity in occipital cortex for attended stimuli, but decreases for unattended stimuli. One suggestion was that gamma activity relates to two separate mechanisms of selective attention, one involving enhancement of the “to be attended” material, and a second involving suppression of competing (i.e., non-attended) material.
Regarding the oscillatory signature of visual processing of threat, MEG studies have revealed that attending to facial displays of fear or anger (Luo et al., Citation2007) or being exposed to the subliminal presentation of such stimuli (Luo et al., Citation2009) results in gamma power changes (increases) in regions of visual cortex and amygdala. This research also provides further support for the rapid visual processing of threat, as the onset and offset of gamma responses in posterior regions largely occurred within 250 ms post-stimulus onset (Luo et al., Citation2009). In the above studies the threat stimuli were task-relevant, so it could be informative to examine gamma responses to task-irrelevant threat, as fMRI evidence indicates that directing attention away from threat-related facial displays can result in suppressed associated fusiform cortex and amygdala activation (Brassen, Gamer, Rose, & Buchel, Citation2010). Thus, the aim of the present study was to use MEG to further explore the gamma response in the visual cortex to task-irrelevant, threat-related stimuli (angry or fearful faces), relative to neutral stimuli (neutral faces). It was hypothesized that if the extrastriate cortex is involved in the rapid detection of threat, then early threat-related modulation of gamma-band activity in this region would be observed (i.e., within 250 ms).
METHOD
Participants
Nine participants (6 women; 3 men, 19–50 years) with no history of neurological injury participated. Two participants were subsequently excluded due to excessive head movement (>5 mm). Previous studies have shown that n = 7 is sufficient to reveal significant gamma-band differences across conditions (Adjamian, Hadjipapas, Barnes, Hillebrand, & Holliday, Citation2008). Experimental procedures were in accordance with the Declaration of Helsinki and received approval from the University of Aston's ethical committee.
Stimuli
Angry, fearful, and neutral faces were selected from the NimStim Face set (Tottenham et al., Citation2009). Eight actors (four men) posing three different expressions (angry, fearful, and neutral) were selected (). In all cases, actors depicted in the stimuli displayed a direct gaze and were photographed straight on. All stimuli were gray-scaled, matched for size, and fitted to an oval-shaped layer of dimensions 2° × 3° (this layer obscured nonfacial features, e.g., hair, earrings). An in-house Matlab script was then used to standardize the faces for luminance and contrast (The Mathworks, Inc., UK).
Figure 1. (A) Angry versus neutral facial expressions: a group SnPM comparison for the time window 50–250 ms demonstrates a power difference in BA 18, bilateral lingual gyrus (p < .05, 23 significant voxels; 6, –87, –12). The threshold was set at p < .10, and significant voxels (i.e., p < .05) are white in color. (B) A time-frequency plot for a significant voxel in BA 18 averaged across participants for the angry face condition demonstrates a decrease in power at approximately 100 ms within the 50–80 Hz range for the angry face stimuli. This power decrease was absent for the neutral facial expressions (results not shown).
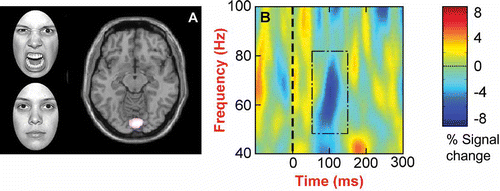
Procedure
Data were collected with a 275-channel, whole-head neuromagnetometer scanner at a sampling rate of 625 Hz, using a third-order gradiometer configuration with an antialiasing filter cutoff of 200 Hz. Participants were seated in an upright position, and three electromagnetic coils were fastened to the participant's nasion and auricular points respectively, to determine head position within the MEG helmet. The display monitor was positioned outside the shielded room and viewed through a window in the room, using a front-silvered mirror. The stimuli were viewed binocularly at an optical viewing distance of 2 m. During the recording session, each subject's head was stabilized within the helmet with an inflatable head-cuff.
On each trial, a single face was presented for 200 ms with a 1.3–1.6 s interstimulus interval (ISI). Stimulus eccentricity corresponded to a visual angle of 1.5° (i.e., central vision). Simultaneously, a stream of mirror-reversed letters (one every 350 ms, no ISI) was presented at central fixation (i.e., letters appeared non-reversed). By a button-press response device, participants were required to respond to the letter X, which appeared on 10% of trials. This task design (1) rendered the faces task-irrelevant and (2) helped maintain an alert attentive state in the participants. Data from trials displaying an X or those where a button-press response was recorded were not analyzed.
For each stimulus type, data from approximately 100 trials were recorded over two experimental runs of 8 min duration. Immediately after data acquisition, a Polhemus Isotrak 3D digitizer was used to map the surface shape of each participant's head and localize the electromagnetic head coils (Medsim, USA). These surface points were then coregistered with the individual participant's anatomical MRI by a surface-matching procedure (Huppertz et al., Citation1998).
Data analysis
Data were bandpass filtered between 0.5 and 100 Hz and DC corrected according to a pre-stimulus baseline, in addition to a 50 Hz powerline filter. Moreover, all data were visually inspected, enabling the removal of trials with eye-blink (e.g., trials in which an extreme dipolar frontal pattern was observed to emerge within the recorded epoch) and/or movement artifacts (e.g., trials in which noise was observed for a period of 25% or more of the recorded epoch). Synthetic aperture magnetometry (SAM) was then used to spatially map task-related power changes in oscillatory brain activity across participants. This method uses the same principle of fixed array-weighted channels as found in contemporary radar systems to scan the brain for the sources of magnetic signals recorded at the scalp (Hillebrand, Singh, Holliday, Furlong, & Barnes, Citation2005). Therefore, SAM requires no a priori assumptions as to the number of sources activated and is ideally suited for the analysis of neuronal activity not strictly time-locked to stimulus onset––i.e., induced activity.
In brief, when using SAM, for each voxel in a predefined source space, an optimal spatial filter is constructed that links activity in that voxel to the MEG system's sensor array. In the present study, each individual's MRI was divided into voxels of 5 × 5 × 5 mm (5 mm grids). The filter output for each voxel was calculated independently as the weighted sum of the sensor signals, yielding a measure of current density as a function of time (Hillebrand et al., Citation2005). This procedure is conceptually similar to the use of an invasive electrode at the neural source location; therefore, the spatial filter is often termed a virtual electrode (Barnes & Hillebrand, Citation2003). The SAM beamformer technique also actively suppresses any undetected noise or artifact sources that may have occurred in spatially removed locations such as around the eyes (e.g., Kinsey, Anderson, Hadjipapas, & Holliday, Citation2011).
For the time windows 50–250 ms (early differences), 250–450 ms (later differences), and 100–600 ms (sustained differences) post-stimulus onset, statistical maps (t maps) of the difference between the facial expressions were calculated for gamma-band oscillations. These analyses involved assessing the difference between spectral power estimates for every voxel with a pseudo-t statistic (Robinson & Vrba, Citation1999), enabling a 3D image of activity to be generated for every participant and time window. Data were then normalized and nonparametric permutation analyses (Singh, Barnes, & Hillebrand, Citation2003) performed with the SnPM toolbox (http://www.fil.ion.ucl.ac.uk/spm/snpm/) to assess significant group effects for voxel-level inferences. Such analyses, unlike random and fixed-effects models, are suited for the robust analysis of data with low degrees of freedom (Singh et al., Citation2003). Additionally, as the SnPM procedure employed included the use of a probability distribution map, generated by the largest T values in the volume rather than the T values at each voxel, the problem of multiple comparisons was circumvented (Nichols & Holmes, Citation2002).
Probability maps for significant group effects (p < .05, corrected) were visualized by using mri3dX http://www.cubric.cf.ac.uk/Documentation/mri3dX/(http://www.cubric.cf.ac.uk/Documentation/mri3dX/), and regions-of-interest (ROIs) were determined from the significant voxel clusters. To examine the specific time course of any changes in oscillatory activity within these ROIs, time-frequency plots were calculated for all individuals, using a Morlet wavelet transform. These plots were created from single-trial activation waveforms for a given ROI, and from these an average time-frequency plot was created. This revealed percent change in energy per time-frequency bin relative to the pre-stimulus phase.
RESULTS
In comparing the facial displays of anger with the neutral facial expressions, SnPM analyses revealed a significant difference in the gamma frequency band within the 50–250 ms time window only. This difference took the form of a significant power decrease (p < .05, corrected for multiple comparisons) in extrastriate visual cortex (BA 18, including lingual gyrus; ). By using the Morlet wavelet transform to examine the exact time course of this difference in gamma activity, group-averaged time-frequency plots for the –200 to 400 ms time period were calculated. These time-frequency plots revealed that the difference in power reflected a decrease in the lower (40–80 Hz) gamma frequency range for angry facial expressions at approximately 100 ms post-stimulus onset (), an effect that was absent for neutral facial expressions. For all other comparisons and time windows, the analyses revealed no significant differences.
While the above analyses demonstrate greater power decreases for anger, the extent to which these changes represent modulation of pre-stimulus versus post-stimulus gamma activity is unclear. Thus, gamma-band activity for angry and neutral faces relative to baseline was examined further.
In each of the seven subjects, guided by our SnPM ROIs, power changes in gamma-band activity prestimulus (–250 to –50 ms) compared with post-stimulus (50–250 ms) onset were investigated for two voxels in BA 18. To avoid confounds associated with using normalized MRI brain templates (Woods, Citation1996), voxels within this ROI were generated with data from each of the participants' own MRI image.
These single-subject ROI analyses revealed gamma-band power decreases in left extrastriate visual cortex (BA 18) for the angry expressions in all participants (as compared to only one participant for the neutral expressions) and a bilateral reduction for the angry faces in this region for five participants (as compared to none for the neutral expressions; see ). For comparison, shows time-frequency plots for one subject for the period –100 to 300 ms. For the angry expressions (), a reduction in power (∼50–80 Hz) was observed from approximately 80–140 ms. However, a similar reduction in power was absent for the neutral expressions (). Given that the group analyses revealed no differences for the fearful faces, these data were not analyzed further.
TABLE 1 A subject-wise overview of frequency fluctuations in the gamma band in the extrastriate cortex. Subject-specific voxel coordinates (top line; bold) show the foci of gamma modulation and the direction of that modulation (PD = power decrease, PI = power increase, NC = no change) for both the right and left hemispheres. For reference, nearest Talaraich coordinates are provided (second line)
Figure 2. Time-frequency plots for a significant voxel in BA 18 for a representative individual demonstrate: (A) A power decrease at approximately 80–150 ms within the 50–80 Hz frequency range for the angry face stimuli; (B) The absence of such an effect in the neutral face stimuli time-frequency plot; and (C) The absence of such an effect in the participant's ‘grand-averaged’ time-frequency plot for the angry stimuli. This demonstrates that the power decrease observed for the angry face stimuli reflect an induced (not evoked) response. In each plot, the stimulus appeared on screen at time zero, and the red/blue colors represent percent change in power.
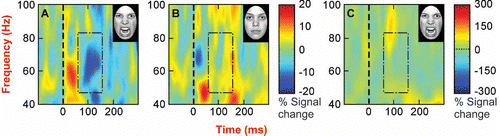
GENERAL DISCUSSION
It was hypothesized that differential modulation of gamma-band activity by threat-related faces as compared to neutral faces would be evident in primary areas of occipital cortex, and that, if observed, these differences would occur within 250 ms of stimulus onset. The findings of this study support the hypotheses with (1) a significant power decrease for facial displays of anger compared to the neutral expressions in extrastriate cortex, including lingual gyrus, and (2) this decrease occurring approximately 100 ms post-stimulus onset (i.e., within the 50–250 ms post-stimulus time window). Moreover, as shown by the individual subject-level analyses, the power decrease in the gamma band for the angry stimuli was observed in BA 18 post-stimulus onset in all subjects.
The current results reveal that perception of facial expressions of anger is associated with modulation of gamma-band activity in the occipital cortex as early as 100 ms post-stimulus onset, a finding consistent with recent MEG research by Luo et al. (Citation2007, 2009). However, while Luo et al. observed power increases, we observed power decreases, a result that is consistent with the methodological differences of our tasks; most notably, in the present research, the stimuli were task-irrelevant––i.e., not the focus of attention. In view of this, parallels can be drawn with recent findings where manipulating the attentional focus away from facial displays of threat resulted in the suppression of amygdala and fusiform cortex activity (Brassen, Gamer, Rose, & Buchel, Citation2010). Additionally, Jerbi et al. (Citation2010) report task-related decreases in gamma-band activity, with strong suppressions of power in areas not related to immediate task demands. Measures of cortical deactivation, then, can be used to identify activity in task-irrelevant areas that needs to be disengaged for appropriate attentional focus to occur. Our data suggest that where threat-related, task-irrelevant emotional stimuli are involved, initial rapid engagement of emotional processing areas requires active suppression as indexed by the gamma-band decreases. As this did not occur during identical presentation of neutral stimuli, it is possible that the threat valence of the stimuli initiated early processing that required subsequent suppression.
Moreover, our results fit well with (1) research implicating the lingual gyrus and extrastriate cortex in threat detection networks; (2) the temporal model of emotional face recognition proposed by Vuilleumier and Pourtois (Citation2007); and (3) the idea that it is periods of both synchrony and desynchrony that enable the rapid detection and processing of significant information (Jensen et al., Citation2007; Jerbi et al., Citation2010). As the lingual gyrus has afferent connectivity with the amygdala (Amaral & Price, Citation1984), our results are also in keeping with the idea that threat circuitry includes regions of primary occipital cortex (Anderson et al., Citation2003). In accordance with this, convergent evidence indicates that early gamma activity observed within visual cortices may reflect mechanisms associated with the fast perceptual processing of aversive stimuli and the initial stage of perceptual processes responsible for recognizing facial expressions of threat (Keil et al., Citation2001; Vuilleumier & Pourtois, Citation2007). Thus, it may be that early oscillatory activity in the gamma frequency range (i.e., that within the first 250 ms of stimulus onset) serves as the mechanism by which selective perceptual processing of threatening facial expressions occurs.
The gamma power decrease may reflect a suppression of visual processing resources that have initially been focused away from task-relevant information (in this instance, the central stream of letters) toward task-irrelevant direct threat cues (angry faces). This account would seem consistent with well-documented findings that threatening faces, especially angry faces, receive prioritized processing that, behaviorally, is manifest in many ways (e.g., rapid reorienting of attention, efficient threat detection) (Maratos, Mogg, & Bradley, Citation2008). It has also been suggested that gamma power decrease reflects an active suppression (or dampening down) of neuronal activity in response to negative information (Vidal et al., Citation2010). Therefore, given that the angry faces were task-irrelevant, the power decreases could reflect inhibition of the hypothesized threat-detection system (in regions of early visual cortex) to enable an individual to focus upon the task at hand. Alternatively, it is possible that our results represent a suppression of gamma synchrony during the central letter task when the angry faces were present. Again, however, such a result would suggest threat superiority––i.e., reprioritization of processing resources from the central letter task to the task-irrelevant (angry) faces.
The absence of an observable gamma response to fearful faces may stem from either qualitative or quantitative differences between the different types of threat cues. Fearful and angry faces are indirect and direct signals of threat, respectively, as fearful faces provide less information about the source of danger (Whalen et al., Citation2001). Fearful and angry faces may also differ in emotional intensity or arousal, with angry faces being more emotionally potent. Thus, there may be a threshold effect for changes in gamma activity, which could be investigated in future studies, as by using morphed angry and fearful facial expressions which manipulate emotional intensity (Mogg, Garner, & Bradley, Citation2007). Another stimulus dimension which would be useful to investigate further is the perceptibility of the stimuli. For example, when the face stimuli used here were blurred (by low-pass filtering), MEG results indicated modulation of theta activity by threat-related expressions (Maratos, Mogg, Bradley, Rippon, & Senior, Citation2009), suggesting an association between theta activity and processing of more ambiguous threat cues. Finally, the present work may also be extended by manipulating task relevance within a single study; this was not practical in the present investigation.
Conclusion
The present data reveal that task-irrelevant threat (facial expressions of anger) is associated with a power decrease in the gamma-band within 250 ms of stimulus onset in the occipital cortex (in keeping with neural theories of visual processing of threat––e.g., Davis & Whalen, Citation2001). Taken together with recent research into the role of gamma in information processing, our research reveals, for angry faces at least, that gamma desynchrony, in addition to gamma synchrony, is key when considering neural networks associated with the perceptual processing of this threat cue.
Acknowledgments
We thank Luke Phillips, Sian Worthen, and Arjan Hillebrand for assistance. This research was supported by the Wellcome Trust (grant no. 051076 awarded to K. Mogg in collaboration with B. Bradley).
REFERENCES
- Adjamian , P. , Hadjipapas , A. , Barnes , G. R. , Hillebrand , A. and Holliday , I. E. 2008 . Induced gamma activity in primary visual cortex is related to luminance and not color contrast: An MEG study . Journal of Vision , 8 : 1 – 7 .
- Amaral , D. G. and Price , J. L. 1984 . Amygdalo-cortical projections in the monkey (Macaca fascicularis) . Journal of Comparative Neurology , 230 : 465 – 496 .
- Anderson , A. K. , Christoff , K. , Panitz , D. , De Rosa , E. and Gabrieli , J. D. E. 2003 . Neural correlates of the automatic processing of threat facial signals . Journal of Neuroscience , 23 : 5627 – 5633 .
- Barnes , G. R. and Hillebrand , A. 2003 . Statistical flattening of MEG beamformer images . Human Brain Mapping , 18 : 1 – 12 .
- Brassen , S. , Gamer , M. , Rose , M. and Buchel , C. 2010 . The influence of directed covert attention on emotional face processing . NeuroImage , 50 : 545 – 551 .
- Davis , M. and Whalen , P. J. 2001 . The amygdala: Vigilance and emotion . Molecular Psychiatry , 6 : 13 – 34 .
- Ewbank , M. P. , Lawrence , A. D. , Passamonti , L. , Keane , J. , Peers , P. V. and Calder , A. J. 2009 . Anxiety predicts a differential neural response to attended and unattended facial signals of anger and fear . NeuroImage , 44 : 1144 – 1151 .
- Hillebrand , A. , Singh , K. D. , Holliday , I. E. , Furlong , P. L. and Barnes , G. R. 2005 . A new approach to neuroimaging with magnetoencephalography . Human Brain Mapping , 25 : 199 – 211 .
- Hung , Y. , Smith , M. L. , Bayle , D. J. , Mills , T. , Cheyne , D. and Taylor , M. J. 2010 . Unattended emotional faces elicit early lateralized amygdala-frontal and fusiform activations . NeuroImage , 50 : 727 – 733 .
- Huppertz , H.-J. , Otte , M. , Grimm , C. , Kristeva-Feige , R. , Mergner , T. and Lucking , C. H. 1998 . Estimation of the accuracy of a surface matching technique for registration of EEG and MRI data . Electroencephalography and Clinical Neurophysiology , 106 : 409 – 415 .
- Ishai , A. , Bikle , P. C. and Ungerleider , L. G. 2006 . Temporal dynamics of face repetition suppression . Brain Research Bulletin , 70 : 289 – 295 .
- Jensen , O. , Kaiser , J. and Lachaux , J. P. 2007 . Human gamma oscillations associated with attention and memory . Trends in Neurosciences , 30 : 317 – 324 .
- Jerbi , K. , Vidal , J. R. , Ossandon , T. , Dalal , S. S. , Jung , J. Hoffman , D. 2010 . Exploring the electrophysiological correlates of the default mode network with intracerebral EEG . Frontiers in Systems Neuroscience , 4 doi: doi: 10.3389/fnsys.2010.00027
- Keil , A. , Muller , M. M. , Gruber , T. , Wienbruch , C. , Stolarova , M. and Elbert , T. 2001 . Effects of emotional arousal in the cerebral hemispheres: A study of oscillatory brain activity and event-related potentials . Clinical Neurophysiology , 112 : 2057 – 2068 .
- Kinsey , K. , Anderson , S. J. , Hadjipapas , A. and Holliday , I. E. 2011 . The role of oscillatory brain activity in object processing and figure-ground segmentation in human vision . International Journal of Psychophysiology , 79 : 392 – 400 .
- Landau , A. N. , Esterman , M. , Robertson , L. C. , Bentin , S. and Prinzmetal , W. 2007 . Different effects of voluntary and involuntary attention on EEG activity in the gamma band . Journal of Neuroscience , 27 : 11986 – 11990 .
- Larson , C. L. , Aronoff , J. , Sarinopoulos , I. C. and Zhu , D. C. 2009 . Recognizing threat: A simple geometric shape activates neural circuitry for threat detection . Journal of Cognitive Neuroscience , 21 : 1523 – 1535 .
- Luo , Q. , Holroyd , T. , Jones , M. , Hendler , T. and Blair , J. 2007 . Neural dynamics for facial threat processing as revealed by gamma band synchronization using MEG . NeuroImage , 34 : 839 – 847 .
- Luo , Q. , Mitchell , D. , Cheng , X. , Mondillo , K. , McCaffrey , D. Holroyd , T. 2009 . Visual awareness, emotion, and gamma band synchronization . Cerebral Cortex , 19 : 1896 – 1904 .
- Maratos , F. A. , Mogg , K. and Bradley , B. P. 2008 . Identification of angry faces in the attentional blink . Cognition & Emotion , 22 : 1340 – 1352 .
- Maratos , F. A. , Mogg , K. , Bradley , B. P. , Rippon , G. and Senior , C. 2009 . Coarse threat images reveal theta oscillations in the amygdala: A magnetoencephalography study . Cognitive, Affective, & Behavioral Neuroscience , 9 ( 3 ) : 133 – 143 .
- Mogg , K. , Garner , M. and Bradley , B. P. 2007 . Anxiety and orienting of gaze to angry and fearful faces . Biological Psychology , 76 : 163 – 169 .
- Nichols , T. E. and Holmes , A. P. 2002 . Nonparametric permutation tests for functional neuroimaging: A primer with examples . Human Brain Mapping , 15 : 1 – 25 .
- Noesselt , T. , Driver , J. , Heinze , H. J. and Dolan , R. 2005 . Asymmetrical activation in the human brain during processing of fearful faces . Current Biology , 15 : 424 – 429 .
- Robinson , S. E. and Vrba , J. 1999 . “ Functional neuroimaging by synthetic aperture magnometry ” . In Recent advances in biomagnetism , Edited by: Yoshimoto , T. 302 – 305 . Tokyo, Japan : Tokyo University Press .
- Rotermund , D. , Taylor , K. , Ernst , U. A. , Kreiter , A. K. and Pawelzik , K. R. 2009 . Attention improves object representation in visual cortical field potentials . Journal of Neuroscience , 29 : 10120 – 10130 .
- Singh , K. D. , Barnes , G. R. and Hillebrand , A. 2003 . Group imaging of task-related changes in cortical synchronisation using nonparametric permutation testing . NeuroImage , 19 : 1589 – 1601 .
- Sokolov , A. , Pavlova , M. , Lutzenberger , W. and Birbaumer , N. 2004 . Reciprocal modulation of neuromagnetic induced gamma activity by attention in the human visual and auditory cortex . NeuroImage , 22 : 521 – 529 .
- Tottenham , N. , Tanaka , J. W. , Leon , A. C. , McCarry , T. , Nurse , M. Hare , T. A. 2009 . The NimStim set of facial expressions: Judgments from untrained research participants . Psychiatry Research , 168 : 242 – 249 .
- Vidal , J. R. , Ossandón , T. , Jerbi , K. , Dalal , S. S. , Minotti , L. Ryvlin , P. 2010 . Category-specific visual responses: An intracranial study comparing gamma, beta, alpha, and ERP response selectivity . Frontiers in Human Neuroscience , 4 doi: doi: 10.3389/fnhum.2010.00195
- Vuilleumier , P. and Pourtois , G. 2007 . Distributed and interactive brain mechanisms during emotion face perception: Evidence from functional neuroimaging . Neuropsychologia , 45 : 174 – 194 .
- Whalen , P. J. , Shin , L. M. , McInerney , S. C. , Fischer , H. , Wright , C. I. and Rauch , S. L. 2001 . A functional MRI study of human amygdala responses to facial expressions of fear versus anger . Emotion , 1 : 70 – 83 .
- Woods , R. P. 1996 . Modelling for inter-group comparisons of imaging data . NeuroImage , 4 : S84 – S94 .