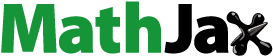
Abstract
The present study aimed to assess the phenotypic variation of milk yield (MY) and quality traits in Italian Jersey (IJ) breed. Sources of variation were investigated through a linear mixed model, including the fixed effects of days in milk (DIM), parity, calving season, milking frequency, recording type, the interaction between DIM and parity, and the random effects of herd-test-day (HTD), cow and the residual. Results highlighted the high contents of milk fat (5.18%), protein (4.08%) and casein (3.16%) of IJ cows. Somatic cell score, averaging 3.35 units, should be lowered through specific managerial actions. Phenotypic variances of MY and milk quality traits were mainly due to cow effect, whereas phenotypic variance of milk urea nitrogen (MUN) content was mainly due to HTD effect, meaning that managerial conditions, especially feeding, are very important to explain the variation of MUN. In conclusion, the present study allowed to characterise milk of IJ cows at population level and to identify environmental factors associated with variation of MY and quality traits, which will be useful to adjust phenotypic records in genetic evaluation of Jersey breed.
Factors affecting milk quality traits of Italian Jersey cows have been investigated.
Phenotypic variance of milk composition and SCS was mainly due to cow effect, and that of MUN was mainly driven by herd-test-day effect.
Significant environmental factors identified in the present study will be used to adjust phenotypic records in genetic analysis.
Highlights
Introduction
Among dairy cow breeds, Jersey is characterised by relatively low milk yield (MY). Nevertheless, Jerseys are endowed with a number of favourable production related traits, such as high milk solids production per unit of feed, high feed conversion efficiency per kilogram of liveweight and high reproductive performance (Hickson et al. Citation2006). Jerseys are worthy also with regard to management aspects, since their productivity and udder conformation are suitable for grazing systems (Lopez-Villalobos et al. Citation2014) and once a day milking (Rocha et al. Citation2018). Furthermore, Jerseys experiment less heat stress load compared with other cattle breeds (Smith et al. Citation2013).
Jersey cows were introduced in Italy in the early 1980s and the Italian Jersey (IJ) herd book was established 10 years later. Since 1999, the Italian Holstein Association (ANAFI, Cremona, Italy) is responsible for the IJ genetic evaluation and herd book maintenance. From 2007 to 2016, the number of Jersey cows farmed in Italy increased from 6391 to 7009 (ANAFI, Citation2016), milk production increased from 5953 to 6521 kg/lactation, but fat and protein content decreased from 5.20 to 4.93%, and from 4.00 to 3.94%, respectively (ANAFI, Citation2016). Giving the high milk solids content, Bland et al. (Citation2015) investigated the effect of blending Jersey and Holstein milk; results showed enhanced milk composition, as well as improved Cheddar cheese yield and sensory properties.
There is a lack of studies that characterise milk quality traits of IJ at population level. A study is required to provide a comprehensive insight into IJ milk quality traits in the context of Italian dairy industry, where 70% of milk is used to produce cheese. Therefore, the aim of the present study was to investigate the sources of variation of MY, composition traits, milk urea nitrogen (MUN) and somatic cell score (SCS) in IJ breed.
Materials and methods
Data collection and editing
The initial dataset was provided by ANAFI and included 527,585 test-day records from 24,447 IJ cows in 1625 herds, recorded from 1994 to 2016. Milk samples were alternatively collected during morning or evening milking, every 4 (AT4) or 5 weeks (AT5) and analysed for fat, protein, casein, and lactose percentages, and MUN content (mg/dL) using mid-infrared spectroscopy. Somatic cell count (SCC, cells/mL) was determined using a Fossomatic (Foss Electric A/S, Hillerød, Denmark), and values of SCC were transformed to SCS to achieve normality and homogeneity of variances through the formula SCS =3 + log2 (SCC/100,000).
Data from 2005 to 2016 were selected because few observations were available from previous years. Days in milk (DIM) were restricted to be between 5 and 480 d, and parity between 1 and 6. Records with MY <2 kg/d were discarded. Lactations with at least 3 test-day records and herd-test-day (HTD) with at least 3 cows were considered. Samples exceeding four standard deviations from the mean of each trait were considered as missing values. After editing, 417,006 test-day records from 20,434 cows and 600 herds were available for analysis. In the dataset, the DIM and parity averaged 171 d and 2.50, respectively.
Statistical analysis
Descriptive statistics were obtained using the MEAN procedure of SAS version 9.4 (SAS Institute Inc., Cary, NC) and analysis of variance was carried out on a subset extracted from the previously edited dataset considering a herd random sampling approach within records collected between 2014 and 2016. The subset accounted for 13,476 test-day records from 1152 cows and 36 herds, and reflected the variability of the edited data for all the studied traits. Shapiro–Wilk’s test showed that all traits were normally distributed. The following linear mixed model was used (MIXED procedure of SAS):
where yijklmno is the dependent variable; μ is the overall mean of the population; DIMi is the fixed effect of the ith class of stage of lactation of the cow (i = 1 to 13, the first being a class from 5 to 30 d, followed by classes of 30 d each, and the last being a class from 360 to 480 d); Pj is the fixed effect of the jth parity of the cow (j = 1 to 6); Sk is the fixed effect of the kth season of calving (k = winter, spring, summer or autumn); Nl is the fixed effect of the lth number of milkings per day (l = 2 or 3); Tm is the fixed effect of the mth milk recording type (m = AT4 or AT5); (DIM × P)ij is the fixed effect of the interaction between DIM and parity; HTDn is the random effect of the nth HTD (n = 1 to 1150) ∼N(0, σ2HTD); cowo is the random effect of the oth cow (o = 1 to 1152) ∼N(0, σ2cow); and eijklmno is the random residual ∼N(0, σ2e).
Pearson correlations between the traits were computed on the subset using the CORR procedure of SAS.
Results and discussion
Descriptive statistics of MY, composition, MUN and SCS
MY averaged 20.11 kg/d (Table ) which was lower than the average MY of IJ cows reported by Moioli et al. (Citation2007), probably because of the longer DIM period considered in the present study. Lopez-Villalobos et al. (Citation2014) and Coffey et al. (Citation2016) reported lower MY of Jersey cows in New Zealand and Ireland, respectively, which is likely attributable to the lower feeding level of grazing cows in these two pasture-based systems. MY of IJ followed the typical pattern during the lactation, with a peak between 30 and 50 d after calving, followed by a progressive decrease up to the end of lactation (Figure S1). Means of fat, protein and casein were 5.18, 4.08 and 3.16%, respectively (Table ), which were greater than averages reported by Capper and Cady (Citation2012) in US Jersey cows and Penasa et al. (Citation2014) in Italian Brown Swiss, Holstein Friesian and Simmental cows. Milk of IJ cows had lower fat content, and similar protein and casein contents than milk of New Zealand Jerseys (Auldist et al. Citation2004). Casein is the only milk constituent reacting to rennet addition and curd formation occurs because of the aggregation of casein micelles, which consequently enclose milk fat and proteins. For this reason, the high contents of protein and casein of IJ milk may be of interest for Italian dairy industry, since more than 70% of milk is used for cheese manufacturing (Pretto et al. Citation2012). In addition, fat, protein and casein ratios are crucial for milk coagulation properties and cheese yield (Auldist et al. Citation2004; Pretto et al. Citation2013). All the major milk constituents, including fat, protein and casein with the exception of lactose, were less concentrated between 30 and 50 DIM (Figure S1), as a result of a dilution effect.
Table 1. Descriptive statistics of milk yield, composition traits, MUN and SCS of Italian Jersey cows.
The average content of MUN observed in the present study was 23.51 mg/dL (Table ). This result was consistent with findings of Penasa et al. (Citation2014) and Niero et al. (Citation2015) in the milk of different Italian dairy cattle breeds, and slightly lower than the value reported by Bobbo et al. (Citation2014) in the milk of IJ cows reared in multi-breed herds. Conversely, MUN content was approximately twofold than MUN content reported by Miglior et al. (Citation2006) in Ayrshire and Holstein cows. The great variability of MUN across different studies and conditions was expected, since this compound is closely linked to the herd management and to the feeding strategies, as well as to the breed and the physiological status of animals, including DIM, parity and the efficiency of nitrogen utilisation (Miglior et al. Citation2006). MUN showed a slight increasing trend up to 150 DIM and was almost stable during the remaining part of lactation (Figure S1). This pattern was partially in agreement with findings of Miglior et al. (Citation2006) who observed a nadir around 30 DIM and a peak around the middle of lactation followed by a slight increase over the remaining part of the lactation.
SCS averaged 3.35 units (Table ). This value was slightly greater than SCS reported by Bobbo et al. (Citation2014) in the milk of IJ cows, but considerably lower than that reported by Sneddon et al. (Citation2016) in New Zealand Jerseys. In a comparison with other dairy breeds reared in Italy, SCS of IJ cows was close to the SCS reported in Holstein Friesian, but greater than that of Brown Swiss and Simmental breeds (Penasa et al. Citation2014). A decrease of SCS in IJ milk would be desirable in order to enhance and stand out its notable protein, casein and fat content, that might be of great interest especially for the Italian dairy sector (Pretto et al. Citation2013; Niero et al. Citation2016). The curve of SCS across lactation was opposite to that of MY and lactose percentage (Figure S1), with a nadir in early lactation, followed by a gradual increase up to the end of lactation, in agreement with Hagnestam-Nielsen et al. (Citation2009). The increase of SCS could be due to a greater incidence of mammary inflammation in cows at the end of lactation, when animals are more susceptible to pathogen infections (Green et al. Citation2004).
Analysis of variance
F-values and significance of fixed effects included in the analysis of MY, composition traits, MUN and SCS of IJ cows are reported in Table . DIM, parity and their interaction were significant in explaining the variation of all studied traits (p < .05). Milking frequency and calving season were significant for all traits (p < .05), with the exception of lactose content in the case of milking frequency, and lactose content and SCS in the case of calving season. Recording type was significant only for MY, lactose and MUN content (p < .05).
Table 2. F-values and significance of fixed effects, and proportion of total variance accounted by herd-test-day (σ2HTD, %) and cow (σ2cow, %) effects for milk yield, composition traits, MUN and SCS (n = 13,476).
HTD accounted for 5.99% (SCS) to 53.09% (MUN) of the phenotypic variance of the studied traits. On the other hand, the proportion of phenotypic variance explained by the effect of cow ranged from 6.28% (MUN) to 33.26% (MY; Table ). Overall, variance explained by cow effect was greater for MY, protein, casein, and lactose percentages, and SCS, whereas HTD variance was greater for fat percentage and especially for MUN content. The latter result supports that management strategies have a strong influence on MUN content. Accordingly, Rajala-Schultz and Saville (Citation2003) observed that the proportion of MUN variance explained by herd and test-day was much more relevant than the proportion of variance explained by cow effect. Once again, results of the present study are in agreement with Miglior et al. (Citation2006) who reported that MUN is tightly connected to herd management, and are a further confirmation that MUN is a valid tool to monitor the efficiency of protein utilisation of the diet, also in IJ herds.
Correlations
Pearson correlation coefficients (r) between the studied traits are presented in Table . All correlations were significant (p < .001). MY was weakly negatively correlated with fat, protein, casein, and SCS (r between −0.26 and −0.20), in agreement with the opposite trend of MY and milk quality traits throughout the lactation, confirming a dilution effect as observed in other dairy breeds (Visentin et al. Citation2017). Fat was moderately positively correlated with protein (r = 0.50) and casein (r = 0.52), and the correlation between protein and casein was close to unity, in agreement with previous studies (e.g. Visentin et al. Citation2018). SCS was moderately negatively correlated with lactose, confirming the previously discussed opposite trends across lactation (Figure S1), and in agreement with previous phenotypic studies (e.g. Lindmark-Månsson et al. Citation2006). Accordingly, Pyörälä (Citation2003) reported that clinical and subclinical mastitis, that are often associated with an increased SCS, lead to a reduced synthetic ability of the enzyme systems of the milk secretory cells, with particular concern to a decrease in lactose percentage.
Table 3. Pearson correlations coefficients between milk yield, composition traits, MUN and SCS (n = 13,476).
Conclusions
The IJ milk showed desirable fat, protein and casein contents for cheese production. Phenotypic variances of MY and composition traits were mainly driven by cow effect, whereas more than 50% of the phenotypic variance of MUN content was due to HTD effect, underlying the important role of farm and thus feeding management on MUN. Environmental factors associated with variation of the studied traits will be used to adjust phenotypic records when estimating genetic parameters and predicting breeding values for these traits at the population level.
Aknowledgements
The first author wants to thank the ‘Fondazione Ing. Aldo Gini’ (Padova, Italy) for funding the research period at Massey University (Palmerston North, New Zealand). This study was supported by “Latteco project”, sottomisura 10.2 of the PSRN-Biodiversity 2014–2020.
Disclosure statement
The authors declare that there is no conflict of interest associated with the paper. The authors alone are responsible for the content and writing of this article.
References
- ANAFI. 2016. Herd book statistics of the Italian Jersey breed. [accessed 2018 Jan 8]. http://www.anafi.it/Jersey/.
- Auldist MJ, Johnston KA, White NJ, Fitzsimons WP, Boland MJ. 2004. A comparison of the composition, coagulation characteristics and cheesemaking capacity of milk from Friesian and Jersey dairy cows. J Dairy Res. 71:51–57.
- Bland JH, Grandison AS, Fagan CC. 2015. Effect of blending Jersey and Holstein-Friesian milk on Cheddar cheese processing, composition, and quality. J Dairy Sci. 98:1–8.
- Bobbo T, Cecchinato A, Cipolat-Gotet C, Stocco G, Bittante G. 2014. Effect of breed and dairy system on milk composition and udder health traits in multi-breed dairy herds. Acta Agr Kaposvár. 18:81–88.
- Capper JL, Cady RA. 2012. A comparison of the environmental impact of Jersey compared with Holstein milk for cheese production. J Dairy Sci. 95:165–176.
- Coffey EL, Horan B, Evans RD, Berry DP. 2016. Milk production and fertility performance of Holstein, Friesian, and Jersey purebred cows and their respective crosses in seasonal-calving commercial farms. J Dairy Sci. 99:5681–5689.
- Green MJ, Green LE, Schukken YH, Bradley AJ, Peeler EJ, Barkema HW, de Haas Y, Collis VJ, Medley GF. 2004. Somatic cell count distributions during lactation predict clinical mastitis. J Dairy Sci. 87:1256–1264.
- Hagnestam-Nielsen C, Emanuelson U, Berglund B, Strandberg E. 2009. Relationship between somatic cell count and milk yield in different stages of lactation. J Dairy Sci. 92:3124–3133.
- Hickson RE, Lopez-Villalobos N, Dalley DE, Clark DA, Holmes CW. 2006. Yields and persistency of lactation in Friesian and Jersey cows milked once daily. J Dairy Sci. 89:2017–2024.
- Lindmark-Månsson H, Bränning C, Aldén G, Paulsson M. 2006. Relationship between somatic cell count, individual leukocyte populations and milk components in bovine udder quarter milk. Int Dairy J. 16:717–727.
- Lopez-Villalobos N, Spelman RJ, Melis J, Davis SR, Berry SD, Lehnert K, Holroyd RE, MacGibbon AKH, Snell RG. 2014. Estimation of genetic and crossbreeding parameters of fatty acid concentrations in milk fat predicted by mid-infrared spectroscopy in New Zealand dairy cattle. J Dairy Res. 81:340–349.
- Miglior F, Sewalem A, Jamrozik J, Lefebvre DM, Moore RK. 2006. Analysis of milk urea nitrogen and lactose and their effect on longevity in Canadian dairy cattle. J Dairy Sci. 89:4886–4894.
- Moioli B, Contarini G, Avalli A, Catillo G, Orrù L, De Matteis G, Masoero G, Napolitano F. 2007. Short communication: effect of stearoyl-coenzyme A desaturase polymorphism on fatty acid composition of milk. J Dairy Sci. 90:3553–3558.
- Niero G, De Marchi M, Masi A, Penasa M, Cassandro M. 2015. Short communication: characterization of soluble thiols in bovine milk. J Dairy Sci. 98:6014–6017.
- Niero G, Penasa M, Gottardo P, Cassandro M, De Marchi M. 2016. Selecting the most informative mid-infrared spectra wavenumbers to improve the accuracy of prediction models for detailed milk protein content. J Dairy Sci. 99:1853–1858.
- Penasa M, Tiezzi F, Sturaro A, Cassandro M, De Marchi M. 2014. A comparison of the predicted coagulation characteristics and composition of milk from multi-breed herds of Holstein-Friesian, Brown Swiss and Simmental cows. Int Dairy J. 35:6–10.
- Pretto D, De Marchi M, Penasa M, Cassandro M. 2013. Effect of milk composition and coagulation traits on Grana Padano cheese yield under field conditions. J Dairy Res. 80:1–5.
- Pretto D, López-Villalobos N, Penasa M, Cassandro M. 2012. Genetic response for milk production traits, somatic cell score, acidity and coagulation properties in Italian Holstein–Friesian population under current and alternative selection indices and breeding objectives. Livest Sci. 150:59–66.
- Pyörälä S. 2003. Indicators of inflammation in the diagnosis of mastitis. Vet Res. 34:565–578.
- Rajala-Schultz PJ, Saville WJA. 2003. Sources of variation in milk urea nitrogen in Ohio dairy herds. J Dairy Sci. 86:1653–1661.
- Rocha JF, Lopez-Villalobos N, Burke JL, Sneddon NW, Donaghy DJ. 2018. Factors that influence the survival of dairy cows milked once a day. N Z J Agric Res. 61:42–56.
- Smith DL, Smith T, Rude BJ, Ward SH. 2013. Short communication: comparison of the effects of heat stress on milk and component yields and somatic cell score in Holstein and Jersey cows. J Dairy Sci. 96:3028–3033.
- Sneddon NW, Lopez-Villalobos N, Davis SR, Hickson RE, Shalloo L, Garrick DJ. 2016. Supply curves for yields of dairy products from first-lactation Holstein Friesian, Jersey and Holstein Friesian-Jersey crossbred cows accounting for seasonality of milk composition and production. Proc N Z Soc Anim Prod. 76:139–143.
- Visentin G, De Marchi M, Berry DP, McDermott A, Fenelon MA, Penasa M, McParland S. 2017. Factors associated with milk processing characteristics predicted by mid-infrared spectroscopy in a large database of dairy cows. J Dairy Sci. 100:3293–3304.
- Visentin G, Penasa M, Niero G, Cassandro M, De Marchi M. 2018. Phenotypic characterisation of major mineral composition predicted by mid-infrared spectroscopy in cow milk. Ital J Anim Sci. 17:549–556.