Abstract
Farming suffers from a crisis based on lack of profitability. This situation is more relevant in small farms. Farmers have implemented local breeding programmes that have shown good results in terms of biodiversity preservation, increase of technological innovation and improvements in farm’s viability. The objective of this study is to compare technological characteristics among groups of farms, according to their breeding programmes and the viability of dairy sheep system. Furthermore, the role of technologies by means of a canonical discriminant model was analysed. The information from 157 dairy sheep farms in Castilla La Mancha, Spain was used. Technologies were grouped in six technological areas: Management, animal feeding, animal health and biosecurity, land use, milking equipment and dairy, reproduction and genetic. Four canonical discriminant models have been used to classify farms according to technological indicators [Model 1], structural [Model 2], productive [Model 3] and a global model including all the variables (18) [Model 4], by considering the genetic programme and their viability. Afterwards, the relationship among the discriminated groups was analysed using cluster analysis. The canonical model built according to the set of technologies classified a 69.43% of farms [Model 1]. Therefore, the farms that implement a breeding programme (BP) increase the likelihood of viability. First, BP requires higher levels of technological innovation in management and genetics-reproduction. Then, such advances should be implemented in milking equipment and animal health and biosecurity. To conclude, BP is a key factor in the success of technological adoption.
Technology adoption plays a strategical role in sheep production systems.
Breeding program promotes higher global technological level.
Likelihood of viability in dairy sheep farms increases with implementation of a breeding program.
Highlights
Introduction
Mediterranean dairy sheep production responds to an integrated mixed system with synergies among activities, characterised by a family subsistence organisation that profits from the system’s own resources to transform them into milk and meat; typical characteristics from the milk sheep production in Castilla La Mancha (Caballero Citation2009; Toro-Mujica et al. Citation2012). In the last two decades, sheep farms have experienced a structural crisis as a consequence of the constant loss of competitiveness and profitability (Rivas et al. Citation2015). For these reasons, the industry is immersed in a deep transformation based on reducing the number of farms and increasing the flock size with progressive professionalisation and productive specialisation (Milán et al. Citation2011; Rivas et al. Citation2014; Toro-Mujica et al. Citation2015a). Different factors have contributed to this process, among them: the high price of inputs, restrictions to pastures, absence for a generational change, price of milk, the existence of marginality risks, high opportunity Labour force cost, and the substitution of farming by other economic activities that provide higher levels of profitability (Toro-Mujica et al. Citation2012; Morantes et al. Citation2017).
High levels of diversity in the dry land mixed system promote some different responses to the crisis from producers: 33.7% of farms have abandoned milk activity and the rest show a trend towards the intensification and specialisation of production (Toro-Mujica et al. Citation2015a, Citation2015b). In this sense, innovation could be a tool to increase the farm’s competitiveness, viability and sustainability (Perea et al. Citation2014; De-Pablos et al. Citation2018). It would be of special interest to assess the best innovations and practices of management provided by farmers, to recognise the key factors in the success or failure of technological adoption (De Pablos-Heredero, et al. Citation2015). Torres et al. (Citation2014) and Rangel et al. (Citation2017b) identified technologies applied in mixed system cattle farms in Ecuador and Mexico and Rivas et al. (Citation2015) did the same for sheep farms in dry land in Spain.
The most frequent strategy to improve local breeds until the date has been to run an official breeding programme (BP) (Delgado et al. Citation2018). According to zootechnical regulation, a breeding programme includes a set of actions aimed at the conservation, improvement and promotion of certain breeds. In the case of the Manchega sheep breed, the purpose of the breeding scheme is to determine the best breeding males and females for quantity and quality of milk to transmit the desired productive characteristics to the next generation. Apart from this, the success of BP requires technological changes in farms (García et al. Citation2016, Toro-Mújica et al. Citation2015a). These structural and technological changes imply a risk in the viability of the farms, since they impact their multifunctional character and decrease the degree of complementarity among different activities (Ryschawy et al. Citation2013; Masayasu et al. Citation2017). Hence, deepening in the knowledge of farm’s technological needs, a BP can favour the increase of competitiveness of smallholders in mixed systems in different countries.
The following research questions arise: (i) Do farms that develop a BP increase the likelihood of viability? (ii) Could the increase of viability be associated to technological adoption? Therefore, the purpose of this research is to support the fact that the success or failure of an official breeding programme (BP) will depend on the right levels of adoption of technological innovations at different farm’s areas.
The objective of this study is to identify main similar and different technological characteristics among four groups of farms by comparing their breeding programmes and their viability, based on the case of Manchego sheep system in Spain. Initially, the role of innovation was determined by comparing the classifying power of technological variables facing the structural and productive ones. Canonical discriminant analyses and the Mahalanobis distances were used. Later on, the relationship among four groups (viable farms with BP, non-viable farms with BP, viable farms without BP and non-viable farms without BP), were analysed through cluster analysis.
Material and methods
Study area and population
The study area was the Spanish region of Castilla La Mancha (38°-41°N; 1°-5°W), whose surface area of roughly 800,000 ha is distributed as follows: 70% arable land, mostly given over rainfed crops (95%), 20% woodland and 10% natural pastureland. The land is mostly flat. Climate is Continental Mediterranean with dry winters and hot and dry summers. Rainfall is concentrated in autumn and spring, and is highly irregular, annual rainfall figures varying between 400 and 1000 mm. Mean winter temperatures range around 5 °C, while mean summer temperatures average is 25 °C (Caballero, Citation2009). The study population was 907 farms of Manchego sheep from ‘La Mancha’ region, gathered under the protected designation of origin (PDO) ‘Queso Manchego’. Rivas et al. (Citation2015), described how a dry land sheep mixed system is characterised by diversified familiar farms (cereal, wine grapes, ewe, cheese, among others), with low incomes.
Official breeding programme (BP)
The National Association of breeders of Manchega sheep breed (AGRAMA) manages an official breeding programme (BP), whose main objectives are to improve genetics and the performance of farms from three areas of action: genealogical information registration, milk recording and artificial insemination (AGRAMA, Citation2011). In Spain, there are 151 livestock breeds for whom a BP programme has been implemented by their corresponding Associations; 66 of small ruminants (Perea et al. Citation2018). The integration in breeding programme enables the conservation of local breeds under a productive model of minimum cost, which favours the utilisation of autochthonous resources, the preservation of the ecosystem and respect to rural livelihoods. Farms were randomised selected (integrated and non-integrated in a breeding programme). Those farms with indirect participation into the BP (purchasing breeding stock, etc.) or the farms with less than two years using the BP were eliminated.
Dairy farm viability
The viability of each farm was based on its economic results, and it was calculated according to the ability of the business to generate, over the long term, sufficient profits for guaranteeing the maintenance of the family unit, according to Toro-Mujica et al. Citation2012, Rivas et al. Citation2015 and Perea et al. Citation2014). Economic returns were calculated for each year, as the sum of cash flow and the opportunity cost of familiar Labour. If a farm generated a positive economic return from 2011 to 2013, it would be considered as viable (1), and non-viable in other situations (0).
Data collection and study design
A random sample of 17.2% (157 farms) of the whole population was selected. The information was collected by using in situ visits to the farms in 2012 and 2013. To evaluate the hypotheses posed, the sample was segmented into four groups: viable farms that have implemented a BP (n = 50), viable farms that have not implemented a BP (n = 41), non-viable farms that have implemented a BP (n = 13), non-viable farms that have not implemented BP (n = 53).
Three set of variables were defined: those related with technological areas (Ti), those related with farm structure (Si), and those related with productive performance (Pi). Technological indicators included the variables management (T1), animal feeding (T2), animal health and biosecurity (T3), land use (T4), milking equipment and dairy (T5), reproduction and genetic (T6). Each technological index groups a set of innovations, technologies and organisational practices shown in Supplementary Table 1 (Rivas et al. Citation2014, Citation2015). It was based on the proportion of innovations implemented in each farm over all innovations identified, with values from 0 to 100%. The variables used in the structure indicator were: surface (S1), ewes (S2), own surface (S3), family Labour (S4), external feeds (S5) and stocking rate (S6). The variables included in the productive returns were: Lamb yield (P1), sanitary cost (P2), milk yield (P3), lambing interval (P4), lambs sold (P5) and feed cost (P6). Most frequently used variables were selected in the characterisation of sheep systems in La Mancha (Toro-Mujica et al. Citation2012; Rivas et al. Citation2015; Caballero, Citation2009).
Rivas et al. (Citation2015) widely describe Manchego dairy mixed system in Castilla La Mancha. The mean flock size was 868 sheep in 1117.7 ha total farm surface; 83% for grazing and the rest corresponded to cultivated surface. 58% of farms applied unifeed and the consumption of concentrate was around 0.8 kg/ewe/d. In 82% of farms three season mating take place and the rest maintain the ram with the ewes permanently throughout the year. The mean production was of 133.4 kg/ewe per lactation, 1.4 lambs per parity, 3.4 annual work units (57.1% familiar). The mean revenue per sheep was 336.8 €/ewe, while the mean unit cost was 2.2 €/kg of milk. The common descriptive characteristics of studied variables and groups are shown in Table .
Table 1. Descriptive statistics of technological, structural and productive variables in Manchega sheep breed.
Statistical analysis of results
Preliminary testing of data was carried out to determine outliers to be discarded before further analysis. As data presented different units of measurements, they were standardised to zero mean and a standard deviation unit. Differences and similarities in the structure, performance and technological level obtained for each group of farms were analysed using canonical discriminant analysis (Tabachnick and Fidell Citation1996). The first step in the canonical discriminant analysis was to determine the a priori basis for grouping the farms.
The analysis was performed on four models: model 1 related to technological areas (Ti), model 2 related to farm structure (Si), model 3 related to productive performance (Pi) and a fourth model containing the whole group of variables (Ti+Si+Pi).
The efficiency of the discriminative power of a given model was determined by the test of significance of the Wilks' lambda value. The capacity of prediction for each model was tested using the absolute assignment of farms to the preassigned group. The distances among groups were determined using the Mahalanobis distances and their statistical significance. Step-wise discriminant analysis was used to determine the discrimination ability of the variables, either forward or backwards. A complete description of the method can be found in Zurita–Herrera et al. (Citation2011), among others.
The second step was the study of the concrete relationships that exist among the discriminated groups. The cluster analysis was appropriate because it provides not only a simple representation of the groups but also a quantification of the relationships among these groups (Delgado et al. Citation2018). The joining tree clustering method, which is based on a plot that shows linkage distances along the vertical axis has been applied. Every node in the representation points out where a cluster is defined. When the data have a structure, with groups of similar elements, this grouping (structure) is established in the hierarchical tree in the form of different branches.
Clustering analysis was carried out among the groups formed with farms belonging to each group (viability and BP). These analyses were developed using the individual Mahalanobis distances performed in the canonical discriminant analysis and were carried out for the same sets of variables analysed by the canonical discriminant analysis. A complete description of the method can be found in Zurita–Herrera et al. (Citation2011) and Caballero-Villalobos et al. (Citation2018). All statistical analyses were performed using the SPSS Inc (SPSS Statistics Citation2010).
Results
Table shows the general results of the canonical discriminant analysis with all the variables measured (18) [Model 4] and also with each set of variables: technological (6) [Model 1], structural (6) [Model 2] and productive indicators (6) [Model 3]. In all cases, discrimination among groups was evident because the F statistics of the Wilks' lambda were always significant for the discriminant variables. The models 4 and 1 with all the variables (Ti+Si+Pi) and with six technological areas (Ti), classify 80.89% and 69.43% of cases correctly. Both models show high discriminant capability, although technological areas (Ti) showed higher F-statistic and higher deviation concerning the group mean and greater classification capability. Table shows the discriminant power of the all variables utilised in each of the four models and Figure shows the classification of farms by canonical components. From a visual perspective it can be observed that the means among those groups are very different along the axis (Technological variables, Ti and all the variables, Ti+Si+Pi). First dimension (CAN1) discriminates farms with or without BP, and CAN2 discriminates means among viable and non-viable farms (Supplementary Table 2). The model 4 comprising all the variables and the model 1 with technologies showed a great capability to discriminate farms according to their integration or lack of integration in a Breeding programme (BP), while the classification of farms depending on viability was barely measured.
Figure 1. Representation of results in each discriminant model for the groups of farms (Viability and BP) in Manchega sheep breed, defined by the axis built by the first canonical variables (CAN 1 and CAN 2).



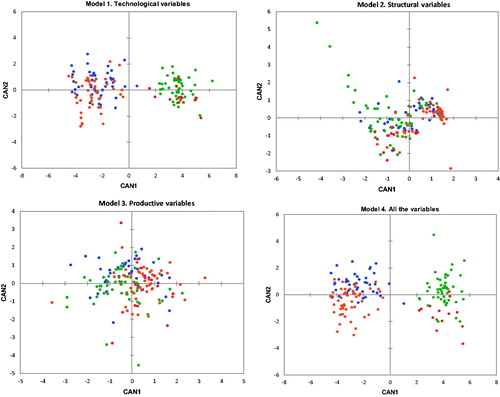
Table 2. Results of the canonical discriminant analysis in Manchega sheep breed.
Table 3. Individual results for variables included in the four canonical discriminant models (variables with the highest discriminate power are bold) and correlations of each variable with the canonical variables.
This outcome is supported by the Mahalanobis distances among groups and in the classification matrix of each farm in its preassigned group (Figure and Supplementary Table 3). The Mahalanobis distances among viable and non-viable farms were 2.340 and 4.258, while between farms with and without BP was 42.000 and 50.155. In addition, 96.7% of classification errors occurred with respect to viability, while there was only one misclassification regarding the existence of BP. This is more evident in the graphical representation of the results in two dimensions. The existence of BP clearly discriminated the groups, while the viability maintained a certain degree of overlapping.
Figure 2. Plot of the cluster analysis developed for the four groups of farms evaluated in Manchega sheep breed.
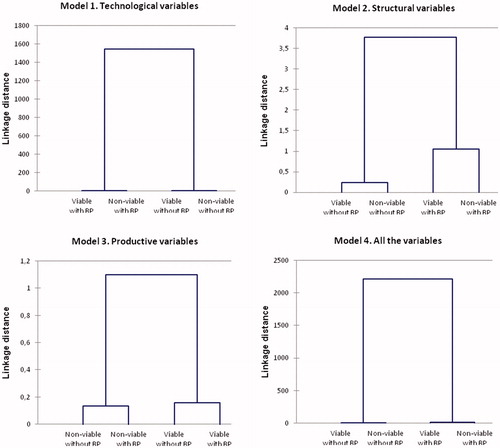
Cluster analysis supported these results (Figure ), as the distances outcome in a clear division between groups according to the existence of BP (linkage distance =2,216.5), while they showed greater similarity among viable and non-viable groups (linkage distance =9.1 for groups without BP and 2.8 for groups with BP). Wilks’ lambda tests indicated a higher discriminating ability for the variables related to technology (Table ).
The variables with greater discriminant ability are highlighted in Table . Variables that discriminated between pairs of groups were identified using stepwise discriminant analysis (Figure ). The variables of management technologies, surface and flock size differentiated between five pairs of groups. The variables health and biosecurity technologies, land use technologies, milking equipment, reproduction and genetic technologies and external feeds differentiated between four pairs of groups.
Table 4. Variables that discriminated between pair of groups using step – wise discriminant analysis in Manchega sheep breed.
Discussion
Breeding programmes (BPs) usually were evaluated on a long term basis, according to a set of zootechnic parameters integrated in a breeding plan; for example, Arias et al. (Citation2016) somatic cell counts (SCC) and milk yield in Manchega sheep; León et al. (Citation2012) type of kidding, lactation number, kidding season in Murciano-Granadina goats, among others. In this research, transversal data instead of longitudinal ones were used. Farms where BP has been implemented to farms where BP has not been implemented (Table ) and viable farms and non-viable farms were compared.
The technology canonical model (Management (T1), animal feeding (T2), animal health and biosecurity (T3), land use (T4), milking equipment and dairy (T5), reproduction and genetic (T6) shows higher levels of discriminatory power (69,43%) than the structural (49,04%) and productive models (50,96%) (Table ). Results show that farms with BP present higher technological innovation levels in the different areas and this implies an increase in the chances to be viable. In Table the structural variables (ewes, familiar Labour and external feeds) were significant in the canonical discriminant analysis too. The application of BP implies higher technological levels and demands at the same time of a minimal dimension, even though dimension is not just enough to increase the technology and the farm’s viability (Rivas et al. Citation2015; Toro-Mújica et al. Citation2015b).
According to Le Gal et al. (Citation2011), Ryschawy et al. (2013) and Ceyhan et al. (Citation2017), there are different strategies to improve competitiveness: (i) Large scale farms and specialised (ii) Small farms with sustainable intensification. Both strategies require of structural changes, the adoption of technologies and business process redesign and a deep transformation of the organisational structure (Morantes et al. Citation2017; De-Pablos-Heredero et al. 2018).
i) Large scale farms. They tend to increase production volumes, herd size, and productivity. According to Toro-Mújica et al. (Citation2012) production is being migrated towards technical and scale efficiencies. However, large scale farms decrease biodiversity and system’s resilience (Toro-Mújica el al. Citation2012 and Ryschawy et al. Citation2013). (ii) Small farms. According to Philippidis et al. (Citation2014), Toro-Mújica et al. (Citation2015b), De-Pablos-Heredero et al. (2018) farms could be oriented to a sustainable intensification at minimal cost by benefiting from flexibility, the existence of dynamic capabilities and localisation barriers. Small farms can reach an efficient allocation of resources, locate production at a level of increasing returns and shift the mean costs curve to more competitive areas (Ceyhan et al. Citation2017; Rangel et al. Citation2017a; García et al. Citation2016).
Next paragraph links results obtained from discriminant and cluster analysis (Table ) with 38 technological innovations identified in Supplementary Table 1 (Rivas et al. Citation2014, Citation2015). In this case, the Manchega breed BP has been focussing on increasing the milk production through genetic improvement and reproductive techniques (T6) from 1975. The improvement in T6 requires a strong technical support and the application of a process management programme (PMP) (Morantes et al. Citation2017). The BP started with structural changes in management (T1) and reproductive and genetic technologies (T6), according to the results of discriminant analysis and the factor loadings from canonical analysis as shown in Table (T1: F, 426.115, R2, 0.805 and CAN1, 0.993; T6: F, 158.586, R2, 0.772 and CAN1, 0.914). This organisation of technologies agrees with the results found by Rangel et al. (Citation2017a). Diverse studies (Wolfová et al. Citation2009, among others) indicate that the systematization of the reproductive process is very important to the productivity of livestock farms since it constitutes the conducting factor. The BP for reproductive and genetic control should be organised from the management area (Le Gal et al. Citation2011). Morantes et al. (Citation2017) indicated that it is essential to establish a technical support for developing the BP and balance the fitness of the reproductive procedure to the type of livestock and farming management.
Therefore, the improvement of the breed within a BP also entails an organisational change in the integral process of managing the farm in a process management programme (PMP) (García et al. Citation2016). PMP is based on the systemisation and standardisation of organisational routines (systems for the productive records, reproductive systems, etc.) and its use in the decision-making process oriented to the reproductive planning, health programmes, milk quality, land use, etc. The management of processes comprises different dimensions and its study requires of a holistic and dynamic approach, where the existent interactions among the different elements in the system gather together (De-Pablos-Heredero et al. 2018). Discriminant analysis between pair of groups results (Table ) were analysed according to Solano et al. (Citation2006) and Millan et al. (Citation2011). The adoption of technological innovations and organisational practices in animal feeding (T2) and land use (T4) increased milk production and decreased the dependence of external inputs.
Future studies should be oriented to deepen in the synergies and interactions between the management of processes, the economic development and the environmental sustainability addressed by De-Pablos-Heredero et al. (2018), Perea et al. (Citation2014) and Rangel et al. (Citation2017b) in the long run. Apart from this, Torres et al. (Citation2014) indicated that management process system contributes to the corporate responsibility, by orienting the processes according to the needs of producers and it constitutes a factor for social sustainability.
Conclusions
The canonical discriminant model built according to the technological set classified a 69.43% of Manchega sheep farms. The technological innovations used were management, animal feeding, animal health and biosecurity, land use, milking equipment and dairy, reproduction and genetic. The likelihood of viability of the dairy sheep farms that implement a breeding programme increased.
BP promotes higher global technological level, and reproduction-genetic and management are the areas to which it has been applied at a large extent. A second group of technologies is required to improve those ones associated to animal health and milking equipment. Finally, the technological level in the land use and in feeding is extremely low, and there is a lack of the application of management process programmes in these areas. The results showed that dairy sheep system presents multiple interactions (synergies and trade-offs) among technologies and it is necessary to deep in their knowledge to increase their sustainable viability without losing the mixed-dry system attributes.
Supplemental Material
Download MS Word (108 KB)Disclosure statement
The authors declare that this article journal is the result of their own work and has not been submitted to any other publishing organisations. Finally, the authors would like to declare that they have no conflict of interest.
Additional information
Funding
References
- AGRAMA. 2011. National Association of breeders of Manchega sheep breed. Programa de mejora de la raza ovina Manchega. http://www.agrama.org (accessed 01 mach 2018).
- Arias A, Oliete B, Seseña S, Jiménez L, Palop L, Pérez-Guzmán M, Arias R. 2016. Importance of on-farm management practices on lactate-fermenting Clostridium spp, spore contamination of total mixed ration of Manchega ewe feeding. Determination of risk factors and characterization of Clostridium population. Small Ruminant Res. 139:39–45.
- Caballero R. 2009. Stakeholder interactions in Castilla La Mancha Spain’s cereal-sheep system. Agr Hum Values. 26:219–231.
- Caballero-Villalobos J, Figueroa A, Xibrraku K, Angón E, Perea JM, Garzón A. 2018. Multivariate analysis of the milk coagulation process in ovine breeds from Spain. J. Dairy Sci. 101:10733–10742.
- Ceyhan V, Cana S, Yıldırım Ç, Türkten H. 2017. Economic structure and services efficiency of Turkish beekeepers’ association. EJSD. 6:53–64.
- De Pablos C, Montes JL, García A. 2018. Sustainability in smart farms: its impact on performance. Sustainability. 10:1713–1729.
- De Pablos C, Haider S, García A. 2015. Relational coordination as an indicator of teamwork quality: potential application to the success of e-learning at universities. IJET. 10:4–8.
- Delgado JV, Landi V, Barba C, Fernández J, Goméz M, Camacho ME, Martínez A, Navas FJ, León JM. 2018. Murciano-Granadina goat: a Spanish local breed ready for the challenges of the twenty-first century. In: Simoes J, Gutiérrez C, editors. Sustainable goat production in adverse environments. Volume 2. Local goat breeds. Cham, Switzerland: Springer International Plubishing. pp. 205–219.
- García A, Rivas J, Rangel J, Espinosa JA, Barba C, De Pablos-Heredero C. 2016. A methodological approach to evaluate livestock innovations on small-scale farms in developing countries. Future Internet. 8:1–17.
- Le Gal PY, Dugué P, Faure G, Novak S. 2011. How does research address the design of innovative agricultural production systems at the farm level? Agr Syst. 104:714–728.
- León JM, Macciotta NPP, Gama LT, Barba C, Delgado JV. 2012. Characterization of the lactation curve in Murciano-Granadina dairy goats. Small Ruminant Res. 107:76–84.
- Masayasu A, Guillaume M, Moraine M, Ryschawy J. 2017. An analytical framework for assessing crop-livestock integration (CLI) beyond the farm level. In: Proceedings of the 4th IEEE International Ecosummit “Ecological Sustainability: Engineering Change”; Aug 28 Sept 1; Montpelier.
- Milán MJ, Caja G, González-González R, Fernández-Pérez AM, Such X. 2011. Structure and performance of awassi and assaf dairy sheep farms in northwestern Spain. J Dairy Sci. 94:771–784.
- Morantes M, Dios-Palomares R, Peña ME, Rivas J, Perea J, García A. 2017. Management and productivity of dairy sheep production systems in Castilla-La Mancha, Spain. Small Ruminant Res. 149:62–72.
- Perea J, De Pablos C, Angón E, Giorgis A, García A. 2014. Using farmer decision making profiles and managerial capacity as predictors of farm viability in Argentina dairy farms (La Pampa. ). Rev Cient-Fac Cien V. 24:509–517.
- Perea J, Barba C, Luque M, González A, Angón E, García A. 2018. Scientific knowledge and conservation policies: interrelationships in the Spanish livestock autochthonous endangered breeds. Rev FCA UNCUYO. 50:171–184.
- Philippidis G, Sanjuán AI, Ferrari E, M'barek R. 2014. Employing social accounting matrix multipliers to profile the bioeconomy in the EU member states: is there a structural pattern?. Span J Agric Res. 12:913–926.
- Rangel J, Espinosa J, De Pablos C, Barba C, Vélez A, Rivas J, García A. 2017a. Adoption of innovations and organizational practices in management animal feeding and reproduction in dual-purpose bovine of small farms in Mexico. Rev Cient-Fac Cien V. 27:44–55.
- Rangel J, Espinosa JA, De Pablos C, Rivas J, Perea J, Angón E, García A. 2017b. Is the increase of scale in the tropics a pathway to smallholders? Dimension and ecological zone effect on the mixed crop-livestock farms. Span J Agric Res. 15:1–10.
- Rivas J, García A, Toro-Mujica P, Angón E, Perea J, Morantes M, Dios-Palomares R. 2014. Caracterización técnica social y comercial de las explotaciones ovinas manchegas centro-sur de España. Rev Mex Cienc Pecu. 5:291–306.
- Rivas J, Perea J, Angón E, Barba C, Morantes M, Dios-Palomares R, García A. 2015. Diversity in the dry land mixed system and viability of dairy sheep farming. Ital J Anim Sci. 14:179–186.
- Ryschawy J, Choisis N, Choisis JP, Gibon A. 2013. Paths to last in mixed crop-livestock farming: lessons from an assessment of farm trajectories of change. Animal. 7:673–681.
- Solano C, León H, Pérez E, Tole L, Fawcett RH, Herrero M. 2006. Using farmer decision-making profiles and managerial capacity as predictors of farm management and performance in Costa Rican dairy farms. Agr Syst. 88:395–428.
- SPSS Statistics. 2010. Statistical package for the social sciences IBM SPSS Statistics 19, SPSS, Inc, Chicago, USA.
- Tabachnick BG, Fidell, LS. 1996. Using multivariate statistics. Harper Collins College Publishers. New York. pp. 80–97.
- Toro-Mujica P, Aguilar C, Vera R, Rivas J, García A. 2015a. Sheep production systems in the semi-arid zone: Changes and simulated bio-economic performances in a case study in Central Chile. Livest Sci. 180:209–219.
- Toro-Mújica P, García A, Aguilar C, Vera R, Perea J, Angón E. 2015b. Economic sustainability of organic dairy sheep systems in central Spain. Ital J Anim Sci. 14:193–201.
- Toro-Mújica P, García A, Gómez G, Perea J, Rodríguez V, Angón E. 2012. Organic dairy sheep farms in south-central Spain: typologies according to livestock management and economic variables. Small Ruminant Res. 104:28–36.
- Torres Y, Rivas J, De Pablos C, Perea J, Toro-Mújica P, Angón E, García A. 2014. Identification and implementation of technological packages for dual purpose cattle. a case study of Manabí-Ecuador. Rev Mex Cienc Pecu. 5:393–407.
- Wolfová M, Wolf J, Krupová Z, Margetín M. 2009. Estimation of economic values for traits of dairy sheep: II. Model application to a production system with one lambing per year. J Dairy Sci. 92:2195–2203.
- Zurita-Herrera P, Delgado JV, Argüello A, Camacho ME. 2011. Multivariate analysis of meat production traits in Murciano-Granadina goat kids. Meat Sci. 88:447–453.