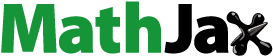
Abstract
Practice of layer poultry farming and commercial egg production relies on the optimal use and improvement of the welfare and genetically determined functional abilities of laying hens, their efficient intake of feed and its components, adaptation to housing conditions and resistance to infectious diseases including salmonellosis. Previous studies were focussed on relationships of chicken performance and resistance with the expression profiles of individual genes involved in metabolic processes and immune system, or with genetic markers that can be closely associated with these processes in chickens. In this study, mathematical models of coherent changes in laying hens were developed for the expression of eight genes involved in immunity and metabolism, on the one hand, and biochemical and immunological blood parameters, on the other hand, in response to Salmonella infection and administration of a phytobiotic Intebio. The proposed modelling approach can be a further basis for an in-depth research of the relationship between the gene expression, functional state and welfare of poultry, impact of pathogenic microorganisms and use of immunomodulatory drugs.
Improved egg production and resistance rely on hens’ potential in gene expression and metabolism.
We developed mathematical models for coherent responses of hens’ gene expression and blood indices to Salmonella infection and phytobiotic intake.
This approach can be used for further studying relationship between gene expression, functional state, impact of pathogens and antimicrobial drugs.
HIGHLIGHTS
Introduction
Salmonellosis remains a serious problem both in poultry industry and in public health care sector (Mughini-Gras et al. Citation2014), while the development of new approaches and veterinary drugs to combat the Salmonella bacteria continues to be relevant (Akbari et al. Citation2008; Yang et al. Citation2015).
In chickens (Gallus gallus), welfare and overall functional status of the organism can be described quite complicatedly and depends both on the individual genetic characteristics and genetic potential, and on the influence of environmental factors experienced by birds in actual growing, housing and feeding conditions. One of the important components of poultry welfare and functional status are the expression levels of certain genes and the state of metabolism, which, in turn, can be estimated via blood biochemical and immunological parameters (e.g. Avzalov Citation2003; Kletikova and Pronin Citation2014; Topuriya Citation2017). Herewith, in response to various environmental factors affecting the bird’s organism, certain genes involved in a number of metabolic pathways can be activated or suppressed.
As an example of earlier searches for association of the functioning of single genes with economically important traits in poultry, one can mention previous genetic studies on activity of serum enzymes. For instance, tissue-nonspecific alkaline phosphatase controlled by the ALPL gene has long been known to be polymorphic and have two allele types, F (fast) and S (slow), found in chickens of different breeds. It was observed on electrophoregrammes that the activity of the dominant fast type was 4–5 times greater than that of the recessive slow type (e.g. Bondarenko and Kutnyuk Citation1975; Bondarenko Citation1976). Under the influence of stress factors, an increase in alkaline phosphatase activity was shown in laying hens (Kletikova and Pronin Citation2014). At the population level, applicability of polymorphic blood groups at 6 genetic loci (Bondarenko and Podstreshny Citation1996) and erythrocyte antigens, when defining population genetic information profiles by the level of entropy (Katerinich et al. Citation2014), was also demonstrated.
Recently, we showed the immunomodulating properties of a novel feed additive Intebio based on essential oils and its positive impact on the welfare of laying hens (Dubrovin et al. Citation2019). When Intebio was added to the ration of laying hens, a more effective launch of immune response, increased resistance, and improved egg weight were observed. Immune response in birds challenged with Salmonella Enteritidis (SE) and fed the additive was more pronounced, and a lowering of egg production curve with age proceeded more smoothly.
In the present work, an attempt was made to develop mathematical models in order to evaluate the effects of intake of phytobiotic Intebio and SE infection in laying hens on the coherent regulation of genes and metabolic responses that seem to be instrumental in maintaining functional state and welfare of poultry.
Materials and methods
Experimental design
To assess the effects of administration of essential oils-based phytobiotic Intebio and SE challenge on functional state and welfare of laying hens, a correlation analysis was performed between the expression data of eight genes and 23 blood biochemical/immunological parameters (Table ) obtained for 24 females of the Lohmann White cross in a study as previously described by Dubrovin et al. (Citation2019). In short, the experiment included chickens over 300 days old that were subjected to infection with a SE epizootic strain and Intebio supplementation in the diet. The phytobiotic intake experiment was started at 346 days of age of layer hens, and at 367 days of age they were inoculated with the SE strain. At 1-day post infection, tissue samples of the hens’ caecum were taken for the further laboratory analysis and used for gene expression examination. Total RNA from the caecal samples was isolated using the Aurum™ Total RNA Mini Kit (BioRad, USA), and reverse transcription reaction was performed to produce cDNAs from RNA templates by employing the iScript™ Reverse Transcription Supermix Kit (Bio-Rad) according to the manufacturer’s instructions.
Table 1. Correlation coefficients (r)* between gene expression and blood biochemical/immunological parameters.
It was hereby assumed that taking into account the aggregated data for the entire experimental sampling would quite noticeably facilitate conformity of the correlation results. Thus, provision of a much greater variability of the analysed data was expected, expanding thereby feasibilities of mathematical description of probable variants for coherent changes in gene expression and blood biochemical/immunological indices, which would reflect the involvement of certain metabolic pathways in maintaining the functional state and welfare of the bird’s body.
Gene expression analysis
Differential expression was evaluated for the following genes involved in immunity, transport and metabolism (Table ): AvBD10, avian β-defensin 10 (antimicrobial peptide); IL6, interleukin 6 (response to inflammation and infection); IL8L1, interleukin 8-like 1 (inflammatory activity); SLC5A1, solute carrier family 5 member 1 (sodium/glucose transport); CA2, carbonic anhydrase 2 (HCO3− and CO2 transport, nitrogen metabolism and eggshell formation); CALB1, calbindin 1 (calcium ion transport); RARRES1, retinoic acid receptor responder 1 (negative regulation of endopeptidase activity and eggshell matrix protein); and BPIFB3, BPI fold containing family B member 3 (innate immune response and eggshell matrix protein). Prior to assessing correlation coefficients (r), expression values of the eight studied genes were normalised in between the samples relative to beta actin used as a household gene.
Results and discussion
The results of the correlation analysis are presented in Table . For the subsequent mathematical analysis, pairs of parameters were chosen that showed a correlation of at least 0.5 (i.e. at |r| > 0.5; Table ). Graphic dependencies for the corresponding mathematical functions reflecting pairwise coherent changes in gene expression and blood indices are given in the Supplemental Material, Figures SM1–SM8. For example, for the IL8L1 gene expression, the following biochemical/immunological parameters meet this criterion (Table ): the levels of urea (−0.551), urea nitrogen (−0.547), alpha amylase (0.508), glucose (0.752), calcium (−0.560), and immunoglobulins IgA (−0.647) and IgG2 (0.850). A more detailed mathematical analysis of the corresponding correlations is depicted in the graphs in the Supplemental Material, Figure SM1 (A–G), each of which was approximated by the most accurate mathematical dependence.
To estimate the multiple correlation of these blood indices with IL8L1, their values were approximated by an equation based on the product of power functions:
(1)
(1)
where U, NU, Gl, and Ca are concentrations of urea, urea nitrogen, glucose and calcium, respectively; and Am, IgA, and IgG2 are activities of alpha amylase and immunoglobulins IgA and IgG2 in the blood of laying hens.
The accuracy of approximation by Equation (Equation1(1)
(1) ) is quite high (R2 = 0.855) and exceeds the maximum calculation accuracy (R2 = 0.785) for a single pairwise dependence (see the Supplemental Material, Figure SM1G), ensuring the relationship closeness at R = 0.925 (Table ). As a result, this calculation formula can be taken for mathematical description of correlated changes in the levels of IL8L1 gene expression and blood biochemical/immunological parameters, which to a certain extent reflects the contribution of these variables to the bird’s functional state.
Table 2. Accuracy of calculating the relationship of gene expression with biochemical/immunological blood parameters based on their multiple correlation.
Similar mathematical processing of the data was carried out for other genes taken into account recalculation options for both pairwise combinations and multiple correlation relations.
For the AvBD10 gene expression (see the Supplemental Material, Figure SM2), the highest correlations with the content of urea (r = −0.531) and urea nitrogen (r = −0.528) were found (Table ). Taking into consideration the nature of their dispersion and the evaluation of single pairwise relationships of the AvBD10 gene expression with these two blood indices, a multiple correlation dependence was obtained as follows:
(2)
(2)
Accuracy of the above formula (2) is rather low and clearly inferior to single pairwise calculations for the parameters U and NU; however, it gives an idea of the degree of contribution of each of the indicators to the overall dependence. The exponent at U is quite small and its contribution to the combined multiple correlation dependence is only 1%. Thus, one can suggest that the content of nitrogen urea is a more significant blood index for the coherent AvBD10 gene expression. Nevertheless, we tried to improve the accuracy of this calculation by selecting other parametric equations, with the result that the following formula provided a greater accuracy:
(3)
(3)
The closeness level of the multiple relation of AvBD10 gene expression with the content of urea and urea nitrogen in the blood was R = 0.709 (Table ), enabling the calculation formula (3) be adopted for interpreting consistent changes in these components of the bird’s functional state.
Similar to the AvBD10 gene, the highest correlations of the IL6 gene expression (see the Supplemental Material, Figure SM3) were also determined with the content of urea (r = −0.720) and urea nitrogen (r = −0.690). The analysis of multiple correlation performed similarly to the calculation of AvBD10 gene expression made it possible to compare two kinds of calculation formulas:
(4)
(4)
and
(5)
(5)
Among these equations, formula (5) turned out to be more accurate (with the relation closeness of the parameters being R = 0.733; Table ), as a result of which this formula was adopted to describe the coherent changes of these correlated indices of the functional status of laying hens.
Expression of the SLC5A1 gene (see the Supplemental Material, Figure SM4) was most associated with the following blood biochemical/immunological parameters in the studied birds (Table ): content of urea (r = −0.574), urea nitrogen (r = −0.586) and calcium (r = −0.566), and activities of alpha amylase (r = 0.606) and immunoglobulins IgA (r = −0.554) and IgG2 (r = −0.605). The multiple regression model enabled an increased accuracy in calculating the SLC5A1 gene expression (R = 0.746; Table ) after approximating the experimental data with the following dependence:
(6)
(6)
Correlation analysis of CA2 gene expression (see the Supplemental Material, Figure SM5) revealed its relationship with glucose content (r = −0.552) and lysozyme activity (r = 0.521; Table ). The analysis of the closeness of the multiple relationships between these indicators resulted in the following calculation formula (with the accuracy at R = 0.720; Table ):
(7)
(7)
where La is the level of lysozyme activity.
Analysis of CALB1 gene expression (see the Supplemental Material, Figure SM6) revealed its relatively strong correlation with only one blood immunological indicator, activity of immunoglobulin IgG2 (r = −0.506; Table ). The resulting calculation formula is not very accurate (R = 0.538; Table ), and its use is more likely to be appropriate only for an approximate assessment of correlated changes in the expression of this gene and activity of immunoglobulin IgG2:
(8)
(8)
Correlation analysis of the dependence of RARRES1 gene expression on blood biochemical parameters (see the Supplemental Material, Figure SM7) made it possible to detect its highest relationships with the levels of urea (r = −0.581), urea nitrogen (r = −0.588) and alpha amylase (r = 0.505; Table ). The multiple correlation between these indicators, as described by the product of power functions, was represented by the following formula, which turned out to be the most accurate, ensuring the closeness of the relationship between these values at R = 0.829 (Table ):
(9)
(9)
Relatively high correlations of the BPIFB3 gene (see the Supplemental Material, Figure SM8) were found with the following blood biochemical/immunological indices (Table ): content of alanine aminotransferase (r = −0.573), glucose (r = 0.552) and bilirubin (r = −0.509), and activities of beta-lysine (−0.515) and immunoglobulins IgM (r = −0.670) and IgG2 (r = 0.623). The analysis of the closeness of the multiple relationships between these parameters allowed us to derive the following calculation formula:
(10)
(10)
where Alt is alanine aminotransferase activity; Br is bilirubin content; IgM is activity of immunoglobulin IgM and Bla is beta-lysine activity.
Formula (10) in its accuracy exceeds the accuracy of calculations using single pairwise relationships, ensuring the closeness of the multiple relationship at R = 0.870 (Table ), and can be used as a basis for characterising the coherent changes in the BPIFB3 gene expression and blood biochemical/immunological indices correlated with it.
The practical use of the regression equations allows for a fairly accurate determination of the correlation of consistent changes in gene expression and various blood biochemical/immunological parameters (R = 0.709–0.937), except for the CALB1 gene (R = 0.538), for which only one pairwise correlation with a single blood immunological indicator was established (Table ). Perhaps, in the future an additional study should be done on peculiarities of this gene expression relative to changes in certain components of the bird’s functional status.
No essential correlation was found for alkaline phosphatase and few other blood indices (Table ). The most informative blood biochemical/immunological parameters were the content of urea, urea nitrogen and glucose, and activity of immunoglobulin IgG2 that most strongly correlated with coherent changes in the expression of eight genes studied in this experiment. This can serve as a basis for further deeper studies on biologically meaningful relationship of blood biochemical/immunological characteristics with genetic parameters, which would bring to a better understanding of various components that ensure the functional state and welfare of the bird’s body (Cogburn et al. Citation2003, Citation2007; Avzalov Citation2003; Topuriya Citation2017).
Sanitary and epidemiological aspects are of great importance for the dynamic development of poultry industry and an increase in the consumption of poultry meat and eggs, since the safety of poultry products is increasingly coming to the fore. In this study, we have proposed and examined one of the modelling-based analytical approaches that can be used in practice and for further research in this area.
The main objectives and advantages of mathematical modelling relevant to various biological processes can be considered as (i) a more evident understanding of the fundamental functioning mechanisms of these processes, and (ii) a solution of certain applied problems more related to the purpose of a specific study.
As for mathematical modelling of gene expression, the fundamental grounds of this approach have been well studied and, as reviewed by some authors (Yīlmaz et al. Citation2005; Ay and Arnosti Citation2011; Robert Citation2019), can be represented by the following major models: differential, stochastic (probabilistic), kinetic and thermodynamic ones. The main benefits of such options for the mathematical structuring of gene expression are transition from a qualitative description to a quantitative estimation and understanding of the laws of gene expression functioning.
Nevertheless, the question of the practical determination of the expression level of any particular genes examined in a particular study often remains open, given the limited possibility of carrying out the necessary measurements of all parameters that affect the course of gene expression process, suggesting other ways for rapid analysis. In this regard, the mathematical dependencies presented by us here would be indispensable, since they allow to quickly and accurately calculate all the necessary factors of gene expression by using the minimum set of initial parameters that can be obtained in any correspondent biological laboratory.
Conclusions
Overall, results of the mathematical modelling can be of practical interest as well as a prerequisite for a more thorough study on correlation between gene expression and blood biochemical/immunological parameters of the examined biological organisms, especially under conditions of infection with Salmonella and other pathogenic microorganisms, as well as testing veterinary drugs that can improve poultry resistance, performance and welfare.
Ethical approval
Animal care and experimental protocol were set up according to the European Convention for the Protection of Vertebrate Animals used for Experimental and other Scientific Purposes, ETS No 123 and was authorised by the relevant national authority. The ethical policies have been adhered to and the appropriate ethical review committee approval has been received.
Supplemental Material
Download MS Word (119.7 KB)Disclosure statement
No potential conflict of interest was reported by the author(s).
Additional information
Funding
References
- Akbari MR, Haghighi HR, Chambers JR, Brisbin J, Read LR, Sharif S. 2008. Expression of antimicrobial peptides in cecal tonsils of chickens treated with probiotics and infected with Salmonella enterica serovar Typhimurium. Clin Vaccine Immunol. 15(11):1689–1693.
- Avzalov RH. 2003. Hematological and immunological parameters of chickens in different age periods, depending on the use of biologically active preparations. Vestnik OSU. 5(6):156–160.
- Ay A, Arnosti DN. 2011. Mathematical modeling of gene expression: a guide for the perplexed biologist. Crit Rev Biochem Mol Biol. 46(2):137–151.
- Bondarenko YV. 1976. Genetic polymorphism of egg and whey proteins of chickens in connection with the direction of selection and level of productivity [dissertation]. Kharkov (Ukraine): Candidate of Biological Sciences.
- Bondarenko YV, Kutnyuk PI. 1975. Genetic polymorphism of domestic fowls and turkeys for plasma alkaline phosphatase. Abstracts of the XIX Conference of Young Scientists and Graduate Students on Poultry Science, Moscow; p. 14–15.
- Bondarenko YV, Podstreshny AP. 1996. Genetic monitoring of chicken populations. Abstracts of the 2nd International Conference on Molecular Genetic Markers of Animals, Agrarna Nauka, Kiev. p. 47–48.
- Cogburn LA, Wang X, Carre W, Rejto L, Porter TE, Aggrey SE, Simon J. 2003. Systems-wide chicken DNA microarrays, gene expression profiling, and discovery of functional genes. Poult Sci. 82(6):939–951.
- Cogburn LA, Porter TE, Duclos MJ, Simon J, Burgess SC, Zhu JJ, Cheng HH, Dodgson JB, Burnside J. 2007. Functional genomics of the chicken—a model organism. Poult Sci. 86(10):2059–2094.
- Dubrovin AV, Ilina LA, Novikova OB. 2019. Influence of essential oils based feed additive on the egg productivity and immune response of laying hens while infection of Salmonella enteritidis epizootic strains. Izvestiya SPbGAU. 1(54):107–111.
- Katerinich OO, Tkachik TE, Bondarenko YV. 2014. Methodical approaches to the genetic-informational profile of the immunogenetic structure of meat and egg chickens. Suchasne ptakhivnytstvo. (3):12–13.
- Kletikova LV, Pronin VV. 2014. Blood biochemical status of the «Hajseks Brown» cross chickens grown in a high-tech enterprise. RVJ PA. (1):5–6.
- Mughini-Gras L, Enserink R, Friesema I, Heck M, van Duynhoven Y, van Pelt W. 2014. Risk factors for human salmonellosis originating from pigs, cattle, broiler chickens and egg laying hens: a combined case-control and source attribution analysis. PLoS One. 9(2):e87933.
- Robert P. 2019. Mathematical models of gene expression. Probab Surveys. 16(0):277–332.
- Topuriya LYu. 2017. The functional condition of the organism of quails under the influence of Germivit. Agrar vestn Urala. 13(167):12–15.
- Yang C, Chowdhury MA, Huo Y, Gong J. 2015. Phytogenic compounds as alternatives to in-feed antibiotics: potentials and challenges in application. Pathogens. 4(1):137–156.
- Yīlmaz FB, Öktem H, Weber G-W. 2005. Mathematical modeling and approximation of gene expression patterns. Oper Res Proc. 2004:280–287.