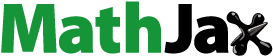
Abstract
The consequences of climate change on agriculture have generated concern among researchers and decision-makers, especially regarding the effects it will have on this sector. The phenomenon is expected to affect the productivity of livestock systems, even more so in grazing livestock, where cattle and pastures are directly impacted by climatic variables. This study evaluated the climatic influence on bulk tank milk production and quality in 38 tropical dairy farms, using Pearson’s partial correlation and canonical correlation analyses. Farm production level and milk quality traits were merged with meteorological information obtained from climatological stations distributed around the Valle del Cauca department, Colombia. According to farms’ milk production levels, four evaluation groups (EG) were established for the analysis, and within each group, the available information between 2.0 and 8.5 years was included. Pearson’s partial correlations among productive and climatic variables were scarce and low (r <± 0.10) within each EG. On the other hand, canonical correlation analyses between productive and climatic variable sets presented a linear increase since the establishment of EGs from 1 to 4 (r ranging from 0.39 to 0.59). The magnitude of canonical correlation coefficients depended on bulk tank dairy farm production levels, being the most productive systems the most susceptible, especially for meteorological variables related to temperature and relative humidity. As a consequence, dairy farms with higher performances must define mitigation strategies to reduce the weather effects. Multivariate correlations are recommended to evaluate the relationship between milk production, composition, and environmental variables in grazing dairy systems of tropical areas.
Livestock farming in the tropics are more vulnerable to climate change.
Heat stress has an impact on the productivity of dairy cows.
Dairy production systems not intensive under grazing conditions and adapted breeds of ecology zones are not affected by adverse meteorological conditions.
Highlights
Introduction
Milk production under the current conditions of climate change constitutes a profound challenge for dairy systems, especially for those in livestock systems under grazing conditions (Scharf et al. Citation2011; Lopes et al. Citation2016). Climate is one of the main factors that affect the behaviour, physiology, and productive efficiency of animals (Hammami et al. Citation2013). The climatic variables that most influence the comfort of animals in the tropics are ambient temperature, solar radiation, wind speed, relative humidity, and precipitation (Polsky and von Keyserlingk Citation2017; Mylostyvyi and Chernenko Citation2019). The effects of single variables or combinations can lead to unfavourable situations known as heat stress, negatively impacting ingestion, digestion, respiration, and blood circulation, among other physiological processes (Herbut and Angrecka Citation2018), which, in turn, impact milk production (productivity and quality) and dairy cow reproduction (Pavani et al. Citation2015; Liu et al. Citation2019).
It was estimated that climate change has caused an increase of 35% in the costs of livestock production in intertropical climate zones (Thamo et al. Citation2017). In tropical Latin America, livestock farming represents one of the most important lands uses from a social, economic, and environmental point of view which are usually developed in conditions characterised by high air temperatures and humidity, making livestock production more vulnerable to climate change, hence generating a loss of employment, animals, and profitability (Landers Citation2007; Oosting et al. Citation2014). In Colombia, more than 95% of production systems are based on grazing (Carulla and Ortega Citation2016; Molina et al. Citation2016).
The exposure of cattle to environmental conditions is influenced by climatic variables, generating direct effects on their performance and behaviour (Scharf et al. Citation2011), and indirectly impacting the quantity and quality of forage, resulting in consequences such as variability in milk bulk composition (Stürmer et al. Citation2018).
Although different works have demonstrated the relationship between climate and milk production and quality (Barrera et al. Citation2015; Mylostyvyi and Chernenko Citation2019), few studies have jointly addressed the relationships between meteorological variables and milk production and characteristics (Milani et al. Citation2015b; Gabbi et al. Citation2017; Stürmer et al. Citation2018).
Multivariate analysis methodologies such as symmetric canonical correlation are used to analyse functions, relations, or expressions that can allow comprehensive form, to reduce without loss of generality, variables of different types, making your use wide and diverse, thus helping the study of the variables with reliability in the complex production systems (Costa et al. Citation2018). Canonical correlation and other multivariate methods had been used to analyse the relationship between milk composition and climatic conditions (Stürmer et al. Citation2018).
The objective of this study was to evaluate the relationship between weather variables and milk tank volume and quality (fat, protein, bacterial count in terms of colony-forming units, and somatic cell count) of grazing dairy farms located in the geographical zone of Valle del Cauca, Colombia, under low tropical conditions, using partial correlation and canonical correlation analysis.
Material and methods
Production systems
The bulk tank milk production and composition information were obtained from 38 dairy farms located in the geographical zone of Valle del Cauca, Colombia, in low tropic conditions between 3’30 to 4’78 N and 76’21 to 76’46 W. These production systems usually base their animal feeding directly on rotational grazing of available pastures. The predominant pastures are Cynodon plectostachyus, Megathyrsus maximus, Brachiaria decumbens or Brachiaria Brizantha (to a lesser extent), and native pasture such as Paspalum spp. (Molina et al. Citation2017; Morales-Vallecilla and Ortiz-Grisales Citation2018). Pure breeds and multiracial cows were found among dairy farms. Bos taurus × Bos indicus crosses represented 70% of the animal population, whereas Holstein, Jersey, Normando, Lucerna, and Gyrolando breeds represented the remaining 30%. All farms used machine milking twice a day, and the milk collected was cooled. Adequate livestock farming practices were carried out with different levels of technology, according to the production system facilities.
The information used in this work was obtained from the database of one of the milk-purchasing companies of the region. The milk production information was obtained from fortnightly records of total bulk tank milk production (MP; in kg), milk fat (as %), milk protein (as %), milk total solids (TS; as %), colony-forming units (CFU/ml), and somatic cell count (SCC/ml) variables, analysed since 2010 to mid of 2018. Every Once every fifteen days, samples of bulk tank milks were taken from each farm for laboratory analysis, to estimate quality and microbiological variables. The sample was fresh milk free of preservatives. On the same day, the average MP of each farm was estimated by dividing the amount of milk delivered by the number of milked cows in each farm. Fat and protein were determined following the Ekomilk ultrasound technology (Bulteh, Stara Zagora, Bulgary). Total solids were estimated using the Richmond formula
where T: total solids; L: lactodensimeter reading, and F: Fat (Patel and Boghra Citation2018). CFU/ml was analysed through the Standard Plate Count SPC method by surface seeding, with a previous dilution of 10−3 (Laird et al. Citation2004). Somatic cell count was determined using the Delaval optical fluorescence counter (DeLaval International AB, Tumba, Sweden).
Climatic information
Climate information was obtained from the automated meteorological network of sugarcane research centre Cenicaña; it has approximately 30 meteorological stations distributed around the geographical zone. The closest meteorological station was assigned to each farm, ensuring that the distance between them did not exceed 20 km. Daily values of mean temperature (Med_T; °C), minimum temperature (Min_T; °C), maximum temperature (Max_T; °C), mean relative humidity (Med_RH; %), maximum relative humidity (Max_RH; %), minimum relative humidity (Min_RH; %), precipitation (PP; mm), solar radiation (SR; cal/cm2), wind speed (WS; m/s), and temperature and humidity index (THI) were used. The THI was estimated from daily mean temperature and relative humidity as suggested by Gomes da Silva et al. (Citation2007). Historic climatic information from 2010 to mid-2018 was obtained and averaged fortnightly to match them with bulk tank milk production and composition variables of the farms. During this process, some climatic information was excluded due to missing values in productive variables. In the final database, 39% of the farms presented information from January 2010 to June 2018, and the remaining had at least two consecutive years of information.
Statistical analysis
Fortnightly data of bulk tank milk production and its chemical and microbiological composition (MP, fat, protein, TS, CFU, and SCC) were gathered with environmental variables (Max_T, Med_T, Min_T, Max_RH, Med_RH, Min_RH, PP, SR, WS, and THI) in each farm. Data outliers were removed according to box plot representation and biological meaning. Later, descriptive statistics were estimated for each variable within the farms, using the InfoStat software (InfoStat Citation2019). Fortnightly, bulk tank milk production presented a high variability among dairy systems, with values between 3440 and 21197 kg. To reduce the variation and establish homogeneous groups of farms according to with the average fortnightly bulk tank milk production level, four evaluation groups (EG) were created: EG 1 (1,000–5,000 kg/fortnight), EG 2 (5,001–10,000 kg/fortnight), EG 3 (10,001–15,000 kg/fortnight), and EG 4 (>15,000 kg/fortnight).
To evaluate the association between bulk tank milk production, its composition (chemical and microbiological) and environmental variables of each EG, Pearson’s partial correlation, and canonical correlation was used, as suggested by Stürmer et al. (Citation2018). To avoid confusing the information patterns, the four EGs were analysed independently, and in each case, variables such as MP, protein, TS, CFU, SCC, PP, SR and WS were transformed to the square root or log10 to meet the assumption of multivariate normality (Sherry and Henson Citation2005). Variable histogram plots were utilised to guarantee the normal distribution. The Max_RH variable was eliminated in all the EGs, as it does not fulfil the assumption.
Pearson’s partial correlation allows estimating the association between two quantitative variables after eliminating the influence of other variables (Vargha et al. Citation2013). In this case, the procedure allows to control the effect of categorical farm and fortnight variables, and remove their residual correlation (Stürmer et al. Citation2018). The estimated partial correlations were used to identify bivariate associations among bulk tank milk production, its composition, and meteorological variables. To identify a significant linear trend, only correlation values equal or greater than ± 0.30 were selected for further analysis. Partial correlations were estimated using PROC GLM of SAS 9.4 (SAS Inc SI Citation2011).
Associations between variables were also evaluated from a multivariate approach. Bulk tank milk production along with the chemical and microbiological variable sets was related with the respective meteorological variable sets through a canonical correlation analysis applied to each EG, using the PROC CANCORR of SAS 9.4 (SAS Inc SI, Citation2011). In this case, when high bivariate correlation (r > 0.80) occurred within each variable group, just one was selected for the analysis, to avoid multicollinearity, as a consequence of linear combination between variables within each set (Manly Citation2004). Canonical correlation analysis creates weighted synthetic variables (also known as canonical functions) within the evaluated groups of characteristics and applies the Pearson correlation method on both synthetic variables. The first canonical function creates the two synthetic variables with the largest possible correlation, thus representing the first canonical correlation. The residual variance left over by the first function is utilised to create a second canonical function, where two more synthetic variables, which are correlated as strongly as possible, are constructed. These new synthetic variables are uncorrelated with the preceding ones (double orthogonality). The process of constructing canonical functions continues until the minimum number of variables in the smaller group are attained or when the total variance of the original variables is explained (Manly Citation2004; Sherry and Henson Citation2005).
To estimate the proportion of variance shared between bulk tank milk production—composition and environmental variable sets across all functions—an effect size index was estimated in each EG (Sherry and Henson Citation2005). Finally, functions that were significant (P < .05) and explained a reasonable amount of the shared variability between the variable sets were interpreted. In these functions, the canonical redundancy statistics available in PROC CANCORR output were analysed to identify how well the environmental canonical variables can predict the original bulk tank milk production and composition variables (SAS Inc SI Citation2011).
Results and discussion
Descriptive statistics of the bulk tank milk production and composition of the 38 evaluated farms are shown on Table . Bulk milk production averaged 3440, 7283, 12022, and 21197 kg/fortnightly for EGs 1, 2, 3, and 4, respectively. EGs 1, 2, and 3 included 42%, 29%, and 16% of the studied farms, respectively, whereas the remaining 13% were in EG 4. This is a common trend since most of the dairy systems in the studied geographical zone belong to small producers, where more productive and technologically advanced systems are scarce (Carulla and Ortega Citation2016; Molina et al. Citation2017; Morales-Vallecilla and Ortiz-Grisales Citation2018). Although the bulk tank milk production was the main difference between EGs, the milk quality parameters were similar. Fat, protein and TS levels ranged between 3.75–3.79%, 3.14–3.22%, and 12.32–12.51%, respectively, whereas CFU and SCC showed a higher variability, with values ranging between 230.18 and 828.11 (×1000 CFU/ml) and between 439.07 and 523.60 (×1000 cells/ml), respectively. In general, the climatic behaviour was characterised by hot days with an average Max_T and Max_RH of 30.6 °C and 97%, respectively, and low precipitation rates (3.4 mm). Productive and climatic conditions found in this analysis were in line with other studies carried out in the same geographical zone (Molina et al. Citation2016; Morales-Vallecilla and Ortiz–Grisales Citation2018). The heterogeneity observed among farms related to milk production and composition complies with typically tropical conditions, where different animal management, genetics and physiology states are present (Ramírez et al. Citation2019).
Table 1. Descriptive statistics of bulk tank milk production, composition and environmental variables of the 38 dairy systems evaluated.
Scarce and low levels of univariate correlations were found between bulk tank milk production, composition, and climatic variables. Among milk chemical and microbiological traits, a positive relationship between fat and TS was found in all the EGs (0.58–0.72). Higher correlation coefficients of 0.88 and 0.92 were reported by Sourabh et al. (Citation2017) and Bondan et al. (Citation2018), respectively. The same positive tendency was observed for protein and TS in EGs 1 and 3 (0.39–0.41, respectively). These are expected associations since milk fat and protein are the main components of total solids.
Correlations between climatic variables varied within the groups. Broadly, all evaluated groups presented significant and negative relationships between Min_T and Max_T (−0.30 to −0.65), Med_T and Med_RH (−0.66 to −0.97), and Max_T and Min_RH (−0.60 to −0.77); meanwhile, positive associations between Med_T and THI (0.61 to 0.99), and Med_RH and THI (0.35–0.96) were observed. Within the EG 4, direct associations between Min_T with PP and Med_T (0.45 and 0.62, respectively), Max_T with Med_RH and Med_T (0.66 and 0.70, respectively), Med_T and Min_RH (0.45), Med_RH and Min_RH (0.85), and SR and WS (0.33) were also found (Picinin et al. Citation2019).
Bivariate correlations among bulk tank milk production, composition, and climatic variables were low and non-significant (P > .05), with most of the values below ± 0.10. These results contrast with the study of Picinin et al. (Citation2019), where rainfall and high temperatures exert an influence on raw milk volume and quality of dairy farms located under similar environmental conditions of the present study.
According to this univariate approach, environmental variables are not related to the volume and composition of the raw milk collected fortnightly within the evaluated production systems. These results suggest, to some extent, that climatic conditions do not influence the performances of grazing dairy cows under tropical conditions. Low correlations may be linked to the particular management, breeds, and microclimate condition of the farms within each EG (Ruiz et al. Citation2019). Similar weak correlations between milk production and climate variables were reported in other studies (Milani et al. Citation2015; Gabbi et al. Citation2017; Mylostyvyi and Chernenko Citation2019).
The relationship between bulk tank milk production, composition (chemical and microbiological), and climatic variables was explored deeply in this study with a second multivariate approach. Previous correlation analysis among climatic variables in each EG indicated high associations with THI and Med_T variables (>0.90). In those groups, to avoid multicollinearity, only THI and Med_T were considered for subsequent analysis, since they have a well-known effect on dairy cows’ performance and heat load (Gomes da Silva et al. Citation2007; Hammami et al. Citation2013; Gabbi et al. Citation2017; Mylostyvyi and Chernenko Citation2019).
Canonical correlation results obtained in the present work are shown in Table . All multivariate tests (Wilks’ Lambda, Pillai’s Trace, the Hotelling-Lawley Trace, and Roy’s greatest root) were significant (p < .0001 in all cases) within each of the EGs, suggesting that a latent structure could be identified using the multivariate approach. These results were accompanied by an analysis of effect size indexes, to guarantee the practical significance of the study. Effect size represents the variance proportion shared between the group of studied variables, and large values are always desirable. The effect size was estimated as 1–Wilks’ Lambda coefficient (Sherry and Henson Citation2005), obtaining values of 0.21, 0.40, 0.43, and 0.47 for EGs 1, 2, 3, and 4, respectively. Even when all the EGs presented significant multivariate test results, only two of them attained a relevant effect size, EGs 3 and 4.
Table 2. Canonical correlation analysis and associated statistics between bulk tank milk production, composition and environmental variables.
The first canonical correlation coefficients observed between EG presented a linear increase as bulk tank milk production improved within the groups (Figure ), with values ranging between 0.39 and 0.59 (Table ). Although the sample sizes were smaller in EGs 3 and 4 compared to EGs 1 and 2, these values still remain significant and higher than those reported in other studies (Aliç Ur Al and Baritçi Citation2013; Stürmer et al. Citation2018). Converse to what was found in previous correlations analysis, these results demonstrated how production systems with higher bulk tank milk volumes are more influenced by meteorological conditions; similar findings were reported by Haygert-Velho et al. (Citation2018). This affirmation agrees with other studies where milk production was reduced per unit increase in THI, and the magnitude varies among dairy systems characteristics, environmental conditions, and raised breeds (Promket et al. Citation2020; Ruiz et al. Citation2019). Usually, in intensive systems under tropical conditions, high productive dairy cows are more sensitive to hot temperatures, mainly because of the high metabolic rate (Rhoads et al. Citation2011; Gantner et al. Citation2017). Cows exposed to high temperatures in tropical ambiances reduce feed intake and increase their metabolic maintenance requirements by 7–25%, causing a decrease in both nutrient supply and nutrient available used for milk synthesis (Ramírez et al. Citation2019). Maintenance requirements increase due to the rise in basal metabolism caused by activation of the thermoregulatory system (Gaughan and Mader Citation2014; McCafferty et al. Citation2017). All these changes negatively impact the individual productive capacity of dairy cows, which can be directly reflected in the amount of milk collected in the production system during a specific time period (Haygert-Velho et al. Citation2018; Ramírez et al. Citation2019).
Figure 1. Relationship between a linear combination of bulk tank milk production – composition and environmental variables obtained in the first canonical functions of each EG.
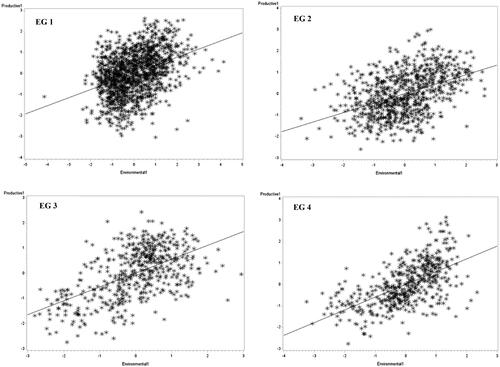
In this study, significant canonical functions explaining a representative amount of variance between bulk tank milk production, composition, and climatic variable sets (the highest squared canonical correlation coefficients ) were chosen for further interpretation. This criterion was established because some functions may not explain the relationship between the variable sets enough, making their interpretation questionable and increasing the risk of highlighting effects not important or replicable in other studies (Sherry and Henson Citation2005). This is the case of EGs 1 and 2: even when several canonical functions were significant, most of the variance explained within each group was low, just like their effect size (0.21 and 0.40 for groups 1 and 2, respectively). Canonical correlations coefficients and associated statistics are found in Table . According to the squared canonical correlation coefficients, the first canonical functions among all groups explained most of the variance shared between bulk tank milk production, composition, and climatic variables, with values between 0.15 and 0.35. To avoid redundant results interpretation, only these functions were selected for further analysis. Table shows the standardised canonical coefficients of the first function associated to each group. In this case, it is preferable to interpret the standardised coefficients since variables are not measured in the same units (SAS Inc SI Citation2011). The analysis of these coefficients together with the correlations between the original variables (milk characteristics and climatic conditions), with their respective canonical variable, allows for the identification of the parameters that most contributed to each canonical function; however, their interpretation must be carried out carefully since, as suggested by Moraes et al. (Citation2015), where a correlation is found, its causality cannot always be predicted.
Table 3. Standardised canonical coefficients, correlation of original variables and their canonical function and canonical redundancy statistic obtained within the first canonical function of each Evaluated Group (EG).
The first canonical correlations among all groups were positive with increasing values since EGs 1–4 (Table ), suggesting that the relationship between the linear combination of bulk tank milk production, composition, and environmental variables was strengthened as bulk tank milk volume increased among groups. Stürmer et al. (Citation2018) also found a strong canonical correlation (0.95) between milk production, composition, and price with climatic variables in systems with bulk tank milk production close to 14500/fortnightly, similar to the volumes observed in the farms belonging to EGs 3 and 4, where the highest canonical correlations coefficients were obtained (0.55 and 0.59, respectively).
The EG 1 presented a canonical correlation of 0.39, with an explained variance of 15%. Standardised canonical coefficients showed that the first canonical variable for bulk tank milk characteristics is a weighted difference between CFU/ml (−0.585), protein (−0.390), and SCC (0.555). Environmental standardised coefficients indicated that Max_T (1.841), Min_RH (1.60), and Min_T (−0.734) were the variables that most contributed to this component. Correlations between original variables and their canonical function also presented the highest associations (−0.60–0.57 and −0.23–0.35, for bulk tank milk characteristics and climatic variables, respectively). These results can be interpreted to mean that high Max_T and Min_RH, and lower Min_T are associated with bulk milk tanks with lower protein and CFU, and higher SCC. Previous climatic conditions are comparable to those found in tropical dry seasons, with high ambient temperature and relative humidity. Under summer conditions, Bertocchi et al. (Citation2014) reported lower protein content and high SCC in the bulk milk data of 3,328 dairy farms. Bouraoui et al. (Citation2002) also observed the same tendency in lactating Holstein cows, while Renna et al. (Citation2010) reported a reduction in milk protein content during the hottest months of the year in grazing cows.
The EG 2 presented a 0.44 canonical correlation and 20% explained variance. Variables like MP, TS, and CFU presented the most contributing standardised coefficients associated with the milk characteristics component (−0.653, 0.479, and 0.396, respectively); meanwhile, the Max_T, Min_RH, and WS variables were for the climatic canonical function (−1.109, −0.835, and 0.650, respectively). Correlations between original variables and their canonical function also presented the highest associations (−0.71–0.49 and −0.30–0.56, for bulk tank milk characteristics and climatic variables, respectively). Within the EG 2, results suggest that low Max_T and Min_RH, and high WS conditions are associated with a reduction in bulk tank milk production and an increase in TS and CFU levels. Some studies report the negative effect of temperature, relative humidity, and wind speed on milk production (Zuluaga and Restrepo Citation2009; Lambertz et al. Citation2014; Promket et al. Citation2020), while Ramírez et al. (Citation2019) described an inverse relation between TS and the milk produced, supporting the observed fact that lower milk volumes are associated with higher TS due to the concentration effect of milk solids.
In EG 3, a 0.55 canonical correlation and 30% explained variance were found. The highest standardised coefficients in canonical bulk tank milk characteristics were observed in TS (−0.661) and CFU (0.832), while WS (0.989) and Min_RH (−0.308) were for the environmental canonical function. Additionally, TS, CFU, WS, and Min_RH showed a strong association with their respective canonical functions (−0.48, 0.84, 0.93, and −0.11 respectively). A conclusion that may arise from these results is that a high WS and lower Min _RH have corresponded with lower bulk tank TS and an increase in CFU. A similar inverse association between relative humidity and CFU had been reported in other studies ( Zuluaga and Restrepo Citation2009; Stürmer et al. Citation2018). A direct relationship between relative humidity and TS was found by Stürmer et al. (Citation2018), contrary to what was obtained within this group. No associations between wind speed and TS or CFU were identified. The influencing variables within this group are similar to those found in EG 2, reinforcing the effect of relative humidity and wind speed, mainly on milk TS and CFU variables.
Finally, the EG 4 showed a canonical correlation of 0.59 and a 35% explained variance. Bulk tank milk characteristics within this group were represented mainly by SCC (0.518), TS (0.430), and protein (0.353); correlations between these variables and their canonical functions were significant (0.48, 0.67, and 0.71, respectively). The highest standardised coefficients related to the environmental canonical function were observed in Med_RH (0.863), Min_T (−0.418), and SR (0.364), with correlation coefficients between these variables and their canonical function of 0.68, −0.40, and 0.57, respectively. These results suggest that environmental conditions with high Med_RH and SR, and low Min_T are associated with increases in bulk tank milk protein, TS, and SCC levels. Zuluaga and Restrepo (Citation2009) showed positive correlations between relative humidity with milk protein and SCC. Stürmer et al. (Citation2018) found negative associations among minimum temperature and milk protein, and TS and SCC. Relative humidity and temperature variables used to construct temperature and humidity indexes have shown a positive correlation with SCC (Bertocchi et al. Citation2014). Solar radiation also positively influences milk composition, especially the protein and TS percentages (Hill and Wall Citation2015;; Stürmer et al. Citation2018).
According to previous results, climatic conditions from the studied geographical zone differentially influence the bulk tank milk production and composition among the EGs, which can be attributed to differences in dairy systems management, proper milking practices implementation, animal genetics, and microenvironmental conditions of the farms associated to each group. Climatic variables like Max_T, Min_T, Med_RH, Min_RH, WS, and SR, together with bulk tank milk volume, protein, TS, CFU, and SCC variables constituted the main factors that explained the canonical correlations found. It is important to highlight that temperature and relative humidity conditions were the most frequented climatic variables influencing bulk tank milk production and composition between the EGs. This observation agrees with other research reports demonstrating that these climatic variables are the most frequent factors impacting the production level and comfort of grazing animals (Gomes da Silva et al. Citation2007; Polsky and von Keyserlingk Citation2017; Mylostyvyi and Chernenko Citation2019); however, other climatic variables such as wind speed and solar radiation can also have a significant influence, so they should be considered in future studies (Gauly et al. Citation2013). These results demonstrate how the climatic variables interact to differentially influence the productivity of the farms and the milk physicochemical and microbiological characteristics, even with production systems located under the same study area.
The relevant result observed with the multivariate approach is that the meteorological variables are correlated with bulk tank milk production, composition, and hygienic conditions in tropical dairy systems based on grazing (Rc = 0.39–0.59). The magnitude of the association varied, depending on the bulk tank milk volume of the dairy farm, being the most productive systems, the most susceptible to climatic conditions. In tropical areas, high ambient temperatures and relative humidity are common, increasing the occurrence risk of heat stress cases in dairy cows, affecting the dairy farm productivity directly, especially those with bulk tank milk production levels above 15,000 kg/fortnight. To reduce the heat stress impact and face new climate change scenarios, these systems require establishing heat stress mitigation practices like the use of trees in grazing areas, implementation of cooling systems (e.g. fans, sprinkles, self-controlled showers) during milking, improving water availability and quality, diet manipulation, and animal selection for heat stress resistances (Lopes et al. Citation2016; Gantner et al. Citation2017; Polsky and von Keyserlingk Citation2017).
The canonical redundancy statistics presented in Table examined how well the canonical variables can predict the original ones (SAS Inc SI Citation2011). In this study, it is useful to identify the amount of variation in bulk tank milk production and composition variables that is accounted for by the linear combination of meteorological variables. Results showed that canonical redundancy statistics increased between 3 and 9% from EG 1 to 4. Only the 9% value found in EG 4 was similar to the 10.2% reported by Stürmer et al. (Citation2018), which can be attributed to the fact that in both situations, the dairy farms included in the analysis presented a bulk tank milk production equal or above 15,000 kg or l/fortnightly. The canonical redundancy coefficients obtained indicate that a linear combination of climatic variables explained at least 3% and a maximum of 9% of the variance of bulk tank milk production and quality variables, depending on the farm production level. These findings are in line with the previous canonical correlation analysis results, where the increasing influence of climatic variables on bulk tank milk characteristics was emphasised.
The positive relationship between bulk tank milk production and composition with environmental variables observed in the present study with canonical correlation analysis is similar to that found with other multivariate methods like cluster, factor, and discriminant analysis (Shiker Citation2012; Haygert-Velho et al. Citation2018; Stürmer et al. Citation2018). These findings corroborate the importance of multivariate approaches to fully understanding the complex influence of several climatic variables on a particular dairy production scenario.
Conclusions
Compared with univariate analysis, multivariate approaches allow for a deeper exploration of factors, contributing to relationship analyses among the climatic variables and bulk tank milk production. Canonical correlation analysis found a significant association between climatic variable sets and bulk tank milk production, and quality in tropical grazing-based dairy systems. The effect and magnitude of climatic influence depended on the bulk tank milk volume of dairy farms, with the most productive systems being the most influenced. Climatic variables like Max_T, Min_T, Med_RH, Min_RH, WS, and SR, along with bulk tank milk volume, protein, TS, CFU/ml, and SCC characteristics were the main factors contributing to the observed canonical correlations. Temperature and relative humidity remained the most important variables affecting bulk tank milk production and quality, even though other variables such as wind speed and solar radiation also deserve attention for further studies. As a result, systems with high production levels must establish practices to mitigate the impact of climatic variables that affect animals’ performance and the herd’s milk yield in tropical environments.
Acknowledgments
The work was carried out as part of the project “Quantification of climate impacts on dairy cattle systems in the low tropic through data mining and modeling”, Announcement 776-2017, MinCiencias.
Disclosure statement
No potential conflict of interest was reported by the authors.
Data availability statement
The data that support the findings of this study are available from the corresponding author upon reasonable request.
References
- Barrera GA, Angeli N, Machado L, Cardoso F, Gonzalez F. 2015. Relationships between heat stress and metabolic and milk parameters in dairy cows in southern Brazil. Trop Anim Health Prod. 47(5):889–894.
- Bertocchi L, Vitali A, Lacetera N, Nardone A, Varisco G, Bernabucci U. 2014. Seasonal variations in the composition of Holstein cow's milk and temperature-humidity index relationship . Animal. 8(4):667–674.
- Bondan C, Folchini JA, Noro M, Quadros LD, Machado MK, González DH. 2018. Milk composition of Holstein cows: a retrospective study. Cienc Rural. 48(12):1–8.
- Bouraoui R, Lahmar M, Majdoub A, Djemali M, Belyea R. 2002. The relationship of temperature–humidity index with milk production of dairy cows in a Mediterranean climate. Anim Res. 51(6):479–491.
- Carulla JE, Ortega E. 2016. Dairy production systems of Colombia: challenges and opportunities. Arch. Latinoam Prod Anim. 24(2):83–87.
- Costa BA, Dantas MR, Alvarenga BM, Peripolli V, Tanure BC, McManus C. 2018. Programs for best dairy management practice in Brazil and their applications. J Clean Prod. 176:1026–1033.
- Gabbi AM, Peripolli V, Cobuci JA, Fischer V, Costa JB, McManus C. 2017. Can meteorological variables affect milk production in different lactation orders of dairy cows in the Cfb climatic zone? A case study in Southern Brazil. Arch Zootec. 66(254):271–278.
- Gantner V, Bobic T, Gantner R, Gregic M, Kuterovac K, Novakovic J, Potocnik K. 2017. Differences in response to heat stress due to production level and breed of dairy cows. Int J Biometeorol. 61(9):1675–1685.
- Gaughan JB, Mader TL. 2014. Body temperature and respiratory dynamics in un-shaded beef cattle. Int J Biometeorol. 58(7):1443–1450.
- Gauly M, Bollwein H, Breves G, Brügemann K, Dänicke S, Daş G, Demeler J, Hansen H, Isselstein J, König S, et al. 2013. Future consequences and challenges for dairy cow production systems arising from climate change in Central Europe – a review. Animal. 7(5):843–859.
- Gomes da Silva R, Façanha Morais D, Guilhermino M. 2007. Evaluation of thermal stress indexes for dairy cows in tropical regions. R Bras Zootec. 36(4 suppl):1192–1198.
- Hammami H, Bormann J, M'hamdi N, Montaldo HH, Gengler N. 2013. Evaluation of heat stress effects on production traits and somatic cell score of Holsteins in a temperate environment. J Dairy Sci. 96(3):1844–1855.
- Haygert-Velho I, Conceição G, Cosmam L, Alessio D, Busanello M, Sippert M, Damiani C, Almeida AP, Velho JP. 2018. Multivariate analysis relating milk production, milk composition, and seasons of the year. An Acad Bras Cienc. 90(4):3839–3852.
- Herbut P, Angrecka S. 2018. Relationship between THI level and dairy cows’ behaviour during summer period. Ital J Anim. Sci. 17(1):226–233.
- Hill DL, Wall E. 2015. Dairy cattle in a temperate climate: the effects of weather on milk yield and composition depend on management. Animal. 9(1):138–149.
- InfoStat 2019. Grupo InfoStat, FCA. Argentina: Universidad Nacional de Córdoba.
- Laird, D.T.; G. Lenarz; F.M. Scher; T.E. Graham and R. Reddy (2004). Microbiological count method (chapter 6): In Standard methods for the examination of dairy products, 17th edition. AP HA. Washington D C, USA, pp. 153–185.
- Lambertz C, Sanker C, Gauly M. 2014. Climatic effects on milk production traits and somatic cell score in lactating Holstein-Friesian cows in different housing systems. J. Dairy Sci. 97(1):319–329.
- Landers J. 2007. Sistemas tropicales de agricultura-ganadera en la agricultura de conservación la experiencia en Brazil. Roma: FAO.
- Liu J, Li L, Chen X, Lu Y, Wang D. 2019. Effects of heat stress on body temperature, milk production, and reproduction in dairy cows: a novel idea for monitoring and evaluation of heat stress – a review. Asian-Australas J Anim Sci. 32(9):1332–1339.
- Lopes BL, Eckstein C, Pina SD, Carnevalli AR. 2016. The influence of trees on the thermal environment and behaviour of grazing heifers in Brazilian Midwest. Trop Anim Health Prod. 48(4):755–761.
- Manly BF. 2004. Análisis estadístico multivariado. Londres: Chappman & Hall.
- McCafferty DJ, Pandraud G, Gilles J, Fabra-Puchol M, Henry PY. 2017. Animal thermoregulation: a review of insulation, physiology and behaviour relevant to temperature control in buildings. Bioinspir Biomim. 13(1):011001.
- Milani MR, Hense A, Rahmani E, Ploeger A. 2015. A pilot investigation of the relationship between climate variability and milk compounds under the Bootstrap Technique. Foods. 4(3):420–439.
- Milani MR, Hense A, Rahmani E, Ploeger A. 2015b. A survey of the relationship between climatic heat stress indices and fundamental milk components considering uncertainty. Climate. 3(4):876–900.
- Molina BA, Sanchez GH, Campos GR, Atzori A, Morales JD. 2017. Dynamic estimation of greenhouse gas emissions from bovine livestock of Valle del Cauca, Colombia. Acta Agron. 66(3):422–429.
- Molina BA, Silva AF, Perilla DS, Sánchez GH. 2016. Caracterización del ambiente térmico para la actividad ganadera bovina en el Valle del Cauca, Colombia. Acta Agron. 65(4):406–412.
- Moraes LE, Kebreab E, Strathe AB, Dijkstra J, France J, Casper DP, Fadel JG. 2015. Multivariate and univariate analysis of energy balance data from lactating dairy cows. J Dairy Sci. 98(6):4012–4029.
- Morales-Vallecilla F, Ortiz-Grisales S. 2018. Productividad y eficiencia de ganaderias lecheras especializadas en el Valle del Cauca (Colombia). Rev Med Vet. 65(3):252–268.
- Mylostyvyi R, Chernenko O. 2019. Correlations between environmental factors and milk production of Holstein Cows. Data. 4(3):103.
- Oosting SJ, Udo HM, Viets TC. 2014. Development of livestock production in the tropics: farm and farmers' perspectives. Animal. 8(8):1238–1248.
- Patel KJ, Boghra VR. 2018. Modifications in Richmond formula for calculating Solids-not-fat/Total solids percent in cows' milk in Gujarat State. Asian Journal of Dairy & Food Research J Dairy Foods Home Sci. 37(4):278–282.
- Pavani K, Carvalhais I, Faheem M, Chaveiro A, Reis VF, Da Silva MF. 2015. Reproductive performance of holstein dairy cows grazing in dry-summer subtropical climatic conditions: effect of heat stress and heat shock on meiotic competence and in vitro fertilization. Asian-Australas J Anim Sci. 28(3):334–342.
- Picinin LC, Bordignon-Luiz M, Cerqueira MP, Toaldo IM, Souza FN, Leite MO, Fonseca LM, Lana AM. 2019. Effect of seasonal conditions and milk management practices on bulk milk quality in Minas Gerais State-Brazil. Arq Bras Med Vet Zootec. 71(4):1355–1363.
- Polsky L, von Keyserlingk AG. 2017. Invited review: effects of heat stress on dairy cattle welfare. J Dairy Sci. 100(11):8645–8657.
- Promket D, Kenchaiwong W, Ruangwittayanusorn K. 2020. Effects of climate change on milk yield and milk composition in Thai crossbred Holstein cows. GEOMATE. 18(67):108–113.
- Ramírez EJ, Rodríguez MJ, Huerta MR, Cárdenas CA, Juárez BJ. 2019. Tropical milk production systems and milk quality: a review. Trop Anim Health Prod. 51(6):1295–1305.
- Renna M, Lussiana C, Malfatto V, Mimosi A, Battaglini ML. 2010. Effect of exposure to heat stress conditions on milk yield and quality of dairy cows grazing on Alpine pasture. In Proceedings of 9th European IFSA Symposium, 4–7 July 2010. Vienna (Austria). p. 1338–1348.
- Rhoads R, La Noce J, Wheelock JB, Baumgard L. 2011. Short communication: alterations in expression of gluconeogenic genes during heat stress and exogenous bovine somatotropin administration. J Dairy Sci. 94(4):1917–1921.
- Ruiz JI, Vargas B, Abarca S, Hidalgo HG. 2019. Heat stress effect on dairy cattle production in Costa Rica. Agronomy Mesoamerican. 30(3):733–750.
- SAS Inc SI 2011. CSAS/STAT® 9.3 Guía del usuario. Cuidado. NC: SAS Institute Inc.
- Scharf B, Leonard MJ, Weaber RL, Mader TL, Hahn GL, Spiers DE. 2011. Determinants of bovine thermal response to heat and solar radiation exposures in a field environment. Int J Biometeorol. 55(4):469–480.
- Sherry A, Henson RK. 2005. Conducting and interpreting canonical correlation analysis in personality research: a user-friendly primer. J Pers Assess. 84(1):34–37.
- Shiker AK. 2012. Multivariate statistical analysis. Br J Sci. 6(11):55–66.
- Sourabh Y, Kumar CS, Hankar SS, Sudheer J. 2017. Correlation between milk constituents and somatic cell counts in Holstein Friesian crossbred cattle. Int J Agri Sci. 9(7):3840–3842.
- Stürmer M, Busanello M, Velho JP, Heck VI, Haygert-Velho IM. 2018. Relationship between climatic variables and the variation in bulk tank milk composition using canonical correlation analysis. Int J Biometeorol. 62(9):1663–1674.
- Thamo T, Addai D, Pannell DJ, Robertson MJ, Thomas DT, Young JM. 2017. Climate change impacts and farm-level adaptation: economic analysis of a mixed cropping–livestock system. Agric Syst. 150:99–108.
- Aliç Ur Al D, Baritçi İ. 2013. Determination of relationship between some udder and body traits of Holstein cows by canonical correlation analysis. Kocatepe Vet J. 6 (1):11–11.
- Vargha A, Bergman LR, Delaney HD. 2013. Interpretation problems of the partial correlation with nonnormally distributed variables. Qual Quant. 47(6):3391–3402.
- Zuluaga JJ, Restrepo BF. 2009. Efecto meteorológico sobre la producción y calidad de la leche en dos Municipios de Antioquia – Colombia. Rev Lasallista Investig. 6(1):50–57.