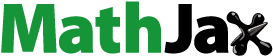
Abstract
Single-step genomic best linear unbiased predictor (ssGBLUP) is a methodology for estimating breeding values jointly for genotyped and non-genotyped animals. Since its development in the early 2010s, ssGBLUP faced challenges like modelling missing pedigrees, efficiently computing accuracies, ensuring the compatibility between genomic and pedigree information, implementing large-scale genetic evaluations, and using non-genotyped animals for genome-wide association studies, among others. Because of the extensive research and the availability of efficient software packages, those challenges for ssGBLUP were solved. Nowadays, ssGBLUP is the chosen methodology estimating values in almost all livestock populations. This review aims to report the progress of ssGBLUP, outline the current state of the art, and hypothesise about the future of this methodology.
Single-step genomic BLUP is the most popular methodology for genetic evaluations including genotyped and non-genotyped animals.
The development of theories and efficient software allows to use single-step for virtually any real dataset.
Continuous research in single-step will allow the use of massive amount of data like video recording, omics, among others.
Highlights
Introduction
Single-step genomic best linear unbiased predictor (ssGBLUP) became the most popular methodology for genetic evaluations, including genotyped and non-genotyped individuals. Since its early development at the beginning of the 2010s, it has been shown to produce accurate estimated breeding values in animals, plants, and humans. This study aimed to review the history of single-step genomic evaluations and provide insights into the future of single-step genetic evaluations.
Preludes of single-step genetic evaluations
According to the classical genetic theory (Fisher Citation1919), the phenotype of an individual is explained by an overall population mean
its genetic merit
plus an error term
:
(1)
(1)
Also, its genetic merit is equal to the sum of the additive (), dominance (
and epistatic effects (
(Falconer and Mackay, Citation1996):
(2)
(2)
Given the, in general, small magnitude of the dominance and epistatic effects, and in consequence:
(3)
(3)
where
is the breeding value of the ith individual. Therefore, if the additive effects at the quantitative trait loci (QTL) are known, the breeding value of the individual can be accurately obtained from (Fernando and Grossman Citation1989):
(4)
(4)
where
is the ith row of the design matrix
for the vector of fixed effects
is the centred genotype of the ith individual at the jth QTL, and
is the effect of the jth QTL. However, the genotypes at the QTL are unknown and, therefore, EquationEquation (4)
(4)
(4) cannot be used for estimation purposes. In the early 2000s, the availability of panels of thousands of single-nucleotide polymorphism (SNP) and the pioneering study from Meuwissen et al. (Citation2001) encouraged using genomic information for genetic evaluations. Given the large number of SNP, it is expected that they are in linkage disequilibrium (LD) with the QTL affecting a specific trait (Meuwissen et al. Citation2001). Therefore, the breeding values of the animals can be obtained from:
(5)
(5)
where
is the effect of the jth SNP in the panel. Then, the conceptual EquationEquation (5)
(5)
(5) leads to two families of equivalent statistical models, known as genomic best linear unbiased predictor (GBLUP) and single nucleotide polymorphism best linear unbiased predictor (SNP-BLUP) (Strandén and Garrick Citation2009). The structure of the GBLUP models is:
(6)
(6)
where
is the vector of phenotypes,
is the vector of breeding values,
is the vector of errors,
is a design matrix,
is the genomic relationship matrix, and
is the additive genetic variance. On the other hand, the structure of the SNP-BLUP models’ family is:
(7)
(7)
where
is the centred SNP content matrix,
is the vector of SNP effects,
is the covariance matrix for the marker effects, and
is the SNP variance. By comparing EquationEquations (6)
(6)
(6) and Equation(7)
(7)
(7) , the reader can note that both models are equivalent when
For most applications,
where
is the minor allele frequency at the ith SNP (VanRaden Citation2008). Analogously to the equivalence between EquationEquations (6)
(6)
(6) and Equation(7)
(7)
(7) , two families of single-step models exist.
Although EquationEquations (6)(6)
(6) and Equation(7)
(7)
(7) are simple, they were not used straightaway because only a small portion of the animals from the livestock populations had available genotypes due to the high genotyping cost. Since most animals were not genotyped, methods for incorporating the genomic information from a small set of animals were necessary for genetic evaluations.
Before the development of single-step methodologies, genomic selection was carried out by the so-called multiple-step procedures (e.g. Boichard et al. Citation2002; Cole et al. Citation2009; Harris and Johnson Citation2010). Besides their differences in details and implementation, most of the multiple-step genetic evaluations involve the following steps: (i) run a pedigree-based genetic evaluation, (ii) obtain pseudo-phenotypes for genotyped animals such as daughter yield deviations (DYD), deregressed proofs (DRP), or adjusted estimated breeding values (EBV), (iii) calculate direct genomic values (DGV) for genotyped animals using a genomic model based on the pseudo-phenotypes obtained in the previous step, and (iv) combine EBV and DGV for genotyped animals using a selection index methodology.
Although its easy implementation, multiple-step genetic evaluations have several drawbacks. These disadvantages include biased or inaccurate predictions for genotyped animals, absence of gain in accuracy for non-genotyped animals, and incompatibility between estimated breeding values for genotyped and non-genotyped animals. We refer the reader to Misztal et al. (Citation2009) and Patry and Ducrocq (Citation2011) for more details on these disadvantages.
History and theory of single-step genetic evaluations
First stages
Given the disadvantages of multi-step genetic evaluations, Misztal et al. (Citation2009) proposed to include both genotyped and non-genotyped animals in a single genetic evaluation by replacing the numerator relationship matrix (A) with a new covariance matrix () that combines genomic and pedigree relationships. With such a matrix, estimated breeding values for non-genotyped and genotyped animals can be obtained from the following model:
(8)
(8)
However, Legarra et al. (Citation2009) noticed that was not a proper covariance matrix because it may not be positive semi-definite. Additionally, the derivation by Misztal et al. (Citation2009) did not consider the distribution of breeding values of ungenotyped animals conditioned on breeding values of genotyped animals. Consequently, Legarra et al. (Citation2009) proposed the currently used single-step covariance matrix
which has the following structure:
(9)
(9)
where the subscripts 1 and 2 represent the blocks pertaining to the non-genotyped and genotyped animals, respectively. Letting
and
be the vector of breeding values for non-genotyped and genotyped animals, respectively, the structure of
represents the covariance of the conditional distribution of
on
given that
was updated by marker information. Henderson (Citation1975) used such a structure to model the effects of selection, which can be traced back to Pearson (Citation1903).
Since Legarra et al. (Citation2009) correctly defined the joint covariance matrix for non-genotyped and genotyped animals, the MME for a single-step genetic evaluation with the model (8) are:
(10)
(10)
Note that the inverse of is needed in (10). However, when single-step was developed, its algebraic structure remained unknown. Although Misztal et al. (Citation2009) proposed different computational approaches for altered versions of (10) without requiring
none of them were as computationally efficient as equations EquationEquation (10)
(10)
(10) .
However, Aguilar et al. (Citation2010) and Christensen and Lund (Citation2010) discovered that the structure of was simpler than the structure of
The authors found that the algebraic expression for
is:
(11)
(11)
where, henceforth, the superscript ij of a matrix refers to the ijth block of its inverse. Due to its simple structure, the above matrix allows using EquationEquation (10)
(10)
(10) for various models for routine genetic evaluations. The genomic relationship matrix is singular when the number of genotyped animals exceeds the number of markers, and in the presence of clones or monozygotic twins. Thus, a small fraction of a positive definite matrix is added to
to ensure its non-singularity in a procedure known as blending (VanRaden Citation2008). In ssGBLUP, the most common choice for blending is
where
A less common but computationally more efficient alternative is using the identity matrix instead of
When the choice is
the underlying genomic model is equivalent to a marker effects model with a residual polygenic effect (RPG) with a covariance matrix equal to
(Christensen and Lund Citation2010).
The compatibility between genomic and pedigree information
The first applications of ssGBLUP to commercial datasets revealed the presence of inflation in the GEBV for genotyped animals (Forni et al. Citation2011; Christensen et al. Citation2012). Also, Aguilar et al. (Citation2010) implicitly recognised it and proposed a scaling factor for reducing the overestimation of GEBV. Vitezica et al. (Citation2011) and Christensen (Citation2012) identified that the origin of the problem was in the difference between the genetic base of pedigree and genomic relationship matrices. Since the genotyped animals were not genotyped at random, their base population was different from the pedigree’s base population. Consequently, this generated incompatibility between and
To overcome this incompatibility, Vitezica et al. (Citation2011) proposed adjusting the genetic base of
to that of
resulting in:
(12)
(12)
where
that is, the average difference between
and
Some implementations may use
instead of EquationEquation (12)
(12)
(12) . This is the method proposed by Powell et al. (Citation2010), closely related to the one proposed by Vitezica et al. (Citation2011).
Christensen (Citation2012) presented two different approaches to ensure the compatibility between the genomic and pedigree relationship matrices. The first follows the ideas from Vitezica et al. (Citation2011) of adjusting He proposed to adjust the genetic base of
by using a simple regression of the form:
(13)
(13)
As mentioned by the author, the coefficients can be estimated either by least squares (VanRaden Citation2008) or by equating the averages of the matrices and their diagonals (Christensen et al. Citation2012; Gao et al. Citation2012). It turns out that both the method of Vitezica et al. (Citation2011) and Christensen (Citation2012) are identical when and
The second approach proposed by Christensen (Citation2012) consisted of adjusting the complete numerator relationship to the genetic base of
The author claimed that such an approach depends on two parameters,
and
which can be estimated by maximum likelihood. After being estimated,
is modified such that
and
is calculated as
For generalising the method of Christensen (Citation2012), Legarra et al. (Citation2015) introduced the concept of metafounders. They defined the metafounders as pseudo-individuals that relate pedigree founders and different populations. To use metafounders in single-step, the genomic relationship matrix is constructed with allele frequencies equal to 0.5, and the numerator relationship matrix is modified by including a multidimensional parameter, called The matrix
with metafounders is:
(14)
(14)
where the subscript
denotes that a matrix was constructed using metafounders, and the subscript 0.5 denotes that it was calculated with allele frequencies equal to 0.5. In genetic evaluations with metafounders,
reflects the covariance between the allele frequencies in the base populations and can be estimated from pedigree and genomic information (Garcia-Baccino et al. Citation2017). In the case of a single metafounder,
and the approach of Legarra et al. (Citation2015) is the same as the method of Christensen (Citation2012). As they can relate a priori unrelated base populations, metafounders are especially helpful for multibreed single-step genomic evaluations (Junqueira et al. Citation2020; Kluska et al. Citation2021).
Missing pedigrees
Genetic evaluations use unknown parent groups (UPG) for modelling missing pedigrees for classical animal models. UPG can be fitted as covariates in the model equation (Westell et al. Citation1988) or by modifying the inverse of the covariance matrix for the random effects (Quaas Citation1988). This second approach is more used than the first one because of its computational efficiency and is the chosen one when modelling missing pedigrees in ssGBLUP. In its introduction, Misztal, Vitezica et al. (Citation2013) pointed out that single-step genomic evaluations had problems when UPG modelled missing pedigrees. They claimed that, at that moment, commercial implementations of ssGBLUP with UPG showed significant ranking changes for selection candidates, poor convergence rate, and biased UPG solutions when fitted as suggested by Quaas (Citation1988). However, these problems were not observed when UPG were included as a separated covariate in the model equation. Thus, Misztal, Vitezica et al. (Citation2013) applied the Quaas-Pollack transformation (Quaas and Pollak Citation1981) to the model equations with UPG as explicit covariates for deriving single-step with UPG. Holding the model in EquationEquation (8)(8)
(8) , the MME for ssGBLUP with UPG are:
(15)
(15)
where
is a matrix assigning animals to UPG,
represents the UPG solutions and
is the BLUP of
The distinctive feature of EquationEquation (15)
(15)
(15) compared to a situation where UPG are included only in
is the multiplication
located in the third diagonal block of the left-hand side of EquationEquation (15)
(15)
(15) , where
is the block of
pertaining to the genotyped animals. Misztal, Vitezica et al. (Citation2013) discussed more specific details and implementation procedures for EquationEquation (15)
(15)
(15) .
Starting from the hypothesis that the information in is complete, Tsuruta et al. (Citation2019) proposed eliminating the UPG contributions for the genomic relationships and Masuda, Tsuruta et al. (Citation2021) showed that this is equivalent to modelling first the missing pedigrees and second the inclusion of genomic information. In contrast, the approach of Misztal, Vitezica et al. (Citation2013) is the opposite. In this setting, the multiplication
is replaced by
Although both approaches give similar results, the second approach is more computationally efficient with many UPG.
Since they are a generalisation of UPG, metafounders can also model missing pedigrees in ssGBLUP. This method has the advantage of modelling missing pedigrees and simultaneously accounting for the incompatibility between genomic and pedigree information. Masuda, Tsuruta et al. (Citation2021) presented a similar approach (i.e. encapsulated UPG) based on UPG instead of metafounders. However, the encapsulated UPG method does not guarantee the compatibility between and
per se; thus, tuning methods are still required.
Individual accuracies
The accuracy of an individual’s GEBV is defined as the correlation between the estimated and true breeding value under a conceptual repeated sampling. According to the BLUP theory (Henderson Citation1984, p. 41), the individual accuracies can be obtained as a function of the diagonal elements of the inverse of the coefficient matrix in EquationEquation (10)(10)
(10) . Approximation methods need to be used when the coefficient matrix is too large to be inverted. Most of the approximation methods have the following steps: (i) calculate accuracies for a model without genomic information, (ii) calculate genomic accuracies, (iii) remove double-counting of information (i.e. phenotypes of genotyped relatives), and (iv) propagate genomic information to non-genotyped animals.
Misztal, Tsuruta et al. (Citation2013) proposed to calculate the amount of information under ssGBLUP models from the diagonal of the matrix:
(16)
(16)
where
is a diagonal matrix of effective number of records or effective record contributions, and
is the ratio between the error and genetic variance. The effective number of records are obtained from genotyped animals’ pedigree accuracies. After calculating the diagonal elements of
the accuracy of the ith genotyped animal is calculated as
Accuracies for non-genotyped animals are then obtained by updating the effective record contributions with weights derived from the calculated accuracy for genotyped animals.
Instead of inverting EquationEquation (16)(16)
(16) , Liu et al. (Citation2017) proposed calculating genomic accuracies based on an SNP-BLUP model and removing double-counting of information by deriving weights from pedigree accuracies calculated only with genotyped animals. In their study, they also proposed adjustment factors to avoid accuracy overestimation. These factors can be calculated by cross-validation from previous data. Edel et al. (Citation2019) suggested a similar strategy with additional steps to simplify the structure of the matrices to be inverted. The most time-consuming task for these methods is the calculation of genomic accuracies; therefore, different strategies were developed to reduce such time (Ben Zaabza et al. Citation2020, Citation2021; Bermann, Lourenco et al. Citation2021).
Marker effects in ssGBLUP
By the equivalence between SNP-BLUP and GBLUP, the marker effects from the former can be obtained as a linear function of the GEBV of the latter and vice versa. Keeping the notation from EquationEquations (6)(6)
(6) and Equation(7)
(7)
(7) , the BLUP for the marker effects
can be calculated as (Strandén and Garrick Citation2009):
(17)
(17)
where
is the BLUP of the vector of breeding values. In single-step, since the statistical model is conditional on the genomic information, EquationEquation (17)
(17)
(17) can be used for estimating the marker effects. However, the genomic relationship matrix in ssGBLUP is usually blended and tuned. If tuning is applied before blending,
is overwritten as:
(18)
(18)
Then, EquationEquation (17)(17)
(17) is modified as follows:
(19)
(19)
In single-step, the estimates of marker effects have a dual purpose. For genetic evaluations, they can be routinely used for calculating interim or indirect predictions, whereas they can also be used for genome-wide association studies (GWAS).
When new genotyped animals without phenotypes or progeny enter the database between genetic evaluations, indirect predictions (IP) estimate their breeding values without performing the complete genetic evaluation; hence, saving computing time. Suppose these genotyped animals have a SNP content matrix centred with the same allele frequencies as in the original genetic evaluation, the GEBV of the indirectly predicted animals are:
(20)
(20)
where
is the mean for genotyped animals. Including it in EquationEquation (20)
(20)
(20) is for avoiding biased indirect predictions (Lourenco et al. Citation2018; Pimentel et al. Citation2019).
The indirect predictions calculated in EquationEquation (20)(20)
(20) are known as direct genomic values (DGV), which do not consider polygenic effects. Therefore, they are an approximation when the underlying model considers a high proportion of residual polygenic effect. To consider polygenic effects for indirect predictions, Liu et al. (Citation2016) presented the following expression:
(21)
(21)
where
is the block of the numerator relationship matrix relating the indirectly predicted animals with the animals used for calculating marker effects in EquationEquation (19)
(19)
(19) .
A significant drawback of the standard GWAS methods is that only genotyped individuals can be used (i.e. EMAXX from Kang et al. Citation2008; Kang et al. Citation2010). Since the number of genotyped animals in livestock populations can be small, GWAS for livestock species suffer from the lack of statistical power and large standard errors associated with QTL detection. Thus, Wang et al. (Citation2012) suggested using single-step for GWAS, developing a method called single-step GWAS (ssGWAS). This allows for including records for non-genotyped animals and for using multiple-trait and complex models. The method works as follows:
Set
Run ssGBLUP with
where
and
is the minor allele frequency at the jth SNP.
Back-solve the GEBV to SNP effects using EquationEquation (19)
(19)
(19) .
Calculate the weight for the jth marker as
(Zhang et al. Citation2010).
Normalise
for the genetic variance being constant across iterations.
Go to step 2 for ssGWAS1 or to step 3 for ssGWAS2.
The computational difference between ssGWAS1 and ssGWAS2 is that the former requires running ssGBLUP once per iteration, whereas the latter only at the first iteration. The choice depends on the stability of GEBV in consecutive iterations. Since this is an ad-hoc algorithm, specific methods for calculating p-values for the marker effects as in the standard GWAS were developed (Aguilar et al. Citation2019). This procedure, known also as ssGWAS, is as follows:
Calculate
Set and solve the MME from EquationEquation (10)
(10)
(10) .
Calculate the matrix of prediction error (co) variances for genotyped animals
Back-solve the SNP effects from the estimated breeding values using EquationEquation (19)
(19)
(19) .
Calculate the variance of the SNP effects’ estimates as
where
is the column of
corresponding to the ith marker.
Calculate the p-value for the ith SNP as
where
is the cumulative density function of the standard normal distribution.
As stated by Aguilar et al. (Citation2019) the estimates and variances of SNP effects calculated from ssGWAS can be transformed to fixed regression estimates as calculated from EMMAX (Bernal Rubio et al. Citation2016).
Marker effects from equivalent models
As for the equivalence between GBLUP and SNP-BLUP, two families of equivalent models to ssGBLUP that estimate the marker effects instead of the breeding values of the animals or both effects together exist. Intending to present computational strategies to improve single-step genomic evaluations, Legarra and Ducrocq (Citation2012) proposed several models equivalent to ssGBLUP. Building from their work, two families of marker effects single-step models were developed: the so-called single-step Bayesian Regression (ssBR; Fernando et al. Citation2014) and single-step SNP-BLUP (ssSNP-BLUP; Liu et al. Citation2014, Taskinen et al. Citation2017).
Fernando et al. (Citation2014) reviewed the theoretical derivation of ssGBLUP in Legarra et al. (Citation2009) and introduced ssBR as an alternative to avoid the inversion of and
in ssGBLUP. Recalling that the subscripts 1 and 2 refer to the non-genotyped and genotyped animals, respectively, the general equation for ssBR is:
(22)
(22)
where
is the expected breeding value for genotyped animals, and
is a vector of imputation errors for non-genotyped animals. The term
is equivalent to the adjustment proposed by Vitezica et al. (Citation2011), differing in that the first considers
as fixed and the second as random. If multivariate normality is assumed for
and
EquationEquation (22)
(22)
(22) yields MME of size equal to the sum of the number of fixed effects, markers, and non-genotyped animals plus one. However, EquationEquation (22)
(22)
(22) is flexible for implementing a Gibbs sampler when a prior distribution different from a normal distribution is assumed for the marker effects (Gianola Citation2009). MME resulting from EquationEquation (22)
(22)
(22) usually involve a diagonal covariance matrix; hence, their inversion is trivial. However, the parts of the MME corresponding to the incidence matrices of the model are dense. Therefore, large matrix multiplications or specific solving strategies (Strandén and Garrick Citation2009) are needed. Regardless of the estimation procedure, the BLUP of the breeding values is:
(23)
(23)
In contrast to ssBR, the ssSNP-BLUP models do not change the incidence matrix for the statistical model. Consequently, the model structure is the same as in EquationEquation (8)(8)
(8) . However, in ssSNP-BLUP, the vector of breeding values has the marker effects appended to it. Therefore,
from EquationEquation (11)
(11)
(11) is extended to:
(24)
(24)
The above covariance matrix yields the following MME:
(25)
(25)
As proposed by Liu et al. (Citation2014), the MME in EquationEquation (25)(25)
(25) can be separated into two different equations:
(26)
(26)
and
(27)
(27)
However, using EquationEquations (26)(26)
(26) and Equation(27)
(27)
(27) leads to models with convergence problems (Mäntysaari et al. Citation2020). Clearly, EquationEquations (24)–(27) are not defined if
Thus, in these settings, ssSNP-BLUP always involves a residual polygenic effect or implicit blending.
Using ssGBLUP, ssBR, or ssSNP-BLUP for obtaining estimates of the marker effects depends on the assumed prior, the statistical model, computational resources, and user preference.
Large-scale genetic evaluations
At the first stages of the implementation of single-step genomic evaluations, EquationEquation (11)(11)
(11) was easy to calculate and plug into the MME. Following Henderson’s rules (Henderson Citation1976; Quaas Citation1976),
is easy to calculate for any number of animals, and
and
were easy to construct, the latter by Colleau (Citation2002), and invert because of the small number of genotyped animals. As the number of genotyped animals grew, the inversion of both matrices turned unfeasible given the usual computational resources for genetic evaluations.
A logical choice to overcome the inversion of is to use ssBR or ssSNP-BLUP, which are models that do not involve
However, they involve complex products of the form
where
and
are matrices or vectors required for estimating the breeding values for non-genotyped animals. Fernando et al. (Citation2014) suggested that such products can be calculated by solving the sparse linear system of equations
using preconditioned conjugate gradient (PCG). However, the resulting matrix
known as the matrix of imputed genotypes, is dense and large to store in memory. For solving this issue in ssBR, Fernando, Cheng, Golden et al. (Citation2016) presented the hybrid model, which directly fits the breeding values for non-genotyped animals plus the marker effects. In ssSNP-BLUP, Taskinen et al. (Citation2017) introduced equivalent models that, instead of storing the matrix of imputed genotypes, impute them ‘on-the-fly’. In ssGBLUP, products of the form
where
is a vector, are needed. Very efficient methods based on the equality
allow calculating
for many genotyped animals (Masuda et al. Citation2017; Strandén et al. Citation2017).
Although ssGBLUP requires this method is widely used for genomic evaluations. This is because it can accommodate more complex models than ssBR and the software development is more straightforward because any program for pedigree-based genetic evaluations can be modified to ssGBLUP by replacing
by
Even though ssSNP-BLUP uses the same incidence matrices as ssGBLUP, memory requirements might be higher depending on the implementation, and convergence problems due to ill-conditioned systems of equations were reported (Vandenplas, Eding et al. Citation2018, 2019). However, convergence problems can be solved using a second-level preconditioner (Vandenplas et al. Citation2019).
Mimicking the ideas of Henderson (Citation1976) and Quaas (Citation1988) for computing Misztal, Legarra et al. (Citation2014) introduced the Algorithm for Proven and Young (APY) to compute a sparse representation of
hereafter denoted as
The idea underlying APY is that genotyped animals can be divided into proven or core animals (c) and young or noncore animals (n). Then, the breeding values of the noncore animals
can be written as a linear combination of the breeding values of the core animals
plus an error term
(28)
(28)
where
is the regression coefficient matrix of
on
Assuming that
and taking variances of both sides of EquationEquation (28)
(28)
(28) :
(29)
(29)
Then, the inverse of EquationEquation (29)(29)
(29) is:
(30)
(30)
The first diagonal block is a dense square matrix of size equal to the number of core animals, whereas the off-diagonal block is a dense rectangular matrix of size equal to the number of core animals times the number of noncore animals. Lastly, the second diagonal block is a diagonal matrix. If the number of core animals is much less than the number of noncore animals, EquationEquation (30)(30)
(30) is sparse, and it can be calculated for many genotyped animals (Masuda et al. Citation2016).
At the beginning of APY implementations, the division between proven and young animals was based on phenotypic and progeny information. However, Fragomeni et al. (Citation2015) empirically showed that the definition of proven and young is not critical for the method to work. Thus, the groups were named core and noncore, and the core animals can be chosen as a random sample from the genotyped animals. Pocrnic et al. (Citation2016) related the number of core animals with the number of eigenvalues explaining a certain percentage of the variance of the spectrum of Misztal (Citation2016) hypothesised that the number and type of animals selected to be core depend on the number of independent chromosome segments present in the population. Thus, the core animals can be chosen at random if they represent all the independent chromosome segments in the population. However, selecting the type and number of core animals in APY is still a topic of discussion and research (Bradford et al. Citation2017; Vandenplas, Calus et al. Citation2018; Nilforooshan and Lee Citation2019). Fernando, Cheng, Garrick et al. (Citation2016) presented a similar approach to APY but based on an orthogonal decomposition of
As an alternative to APY, Mäntysaari et al. (Citation2017) developed ssGTBLUP, which is equivalent to ssSNP-BLUP when the equations for the marker effects are absorbed into the MME. When is blended as
where
is usually proportional to the identity matrix or
Then,
is obtained following the Woodbury formula as:
(31)
(31)
where
is a matrix of size equal to the number of markers times the number of genotyped animals, and
is the Cholesky factor of
Solving the MME by PCG requires multiplying a vector
from right to left in EquationEquation (31)
(31)
(31) . Therefore, it is not needed to compute
but
and
The first product is easy given that
is the identity matrix or
and the matrix
is calculated before solving the MME. For a further reduction in computing time, Mäntysaari et al. (Citation2017) re-defined
where
is the eigendecomposition of
Using this definition, the authors suggested applying a low-rank approximation to EquationEquation (31)
(31)
(31) by eliminating the eigenvectors corresponding to the eigenvalues close to zero, resulting in an approximation to ssGBLUP as in APY. In the same fashion, Ødegård et al. (Citation2018) presented a low-rank approximation of
based on the singular value decomposition of
Variance component estimation
Since the model structure of ssGBLUP is the same as the animal model but replacing by
any method for estimating variance components and reliabilities applied to the animal model can be employed in ssGBLUP. We refer the reader to Hofer (Citation1998) for a review of these methods.
When all the animals are genotyped, the variance components obtained from a GBLUP are equal to those calculated without genomic information when the number of markers increases towards infinity and the pedigree information is complete (Cuyabano et al. Citation2018). Given a finite number of markers, Cuyabano et al. (Citation2018) showed that the heritability calculated with a GBLUP model is likely underestimated because the markers do not capture all the genetic variation. Although it is not proven and a formal proof would be out of the scope of this review, we expect that with blending or a residual polygenic effect, the variance components calculated with a ssGBLUP model should not be biased (Cesarani, Pocrnic et al. Citation2019). Also, the estimated variance components should match those estimated with an animal model, subject to pedigree completeness and sample size. In practice, estimates may or not match (Hidalgo et al. Citation2020) depending on the statistical model, sample size, selection, and genotyping strategies. These hypotheses should hold for any variance components estimation method given their convergence.
ssGBLUP applied to livestock genetic evaluations
Researchers, private companies, and breeders’ associations applied ssGBLUP to nearly all animal livestock species. Breeding values were estimated with ssGBLUP for a wide variety of statistical models in species such as dairy (e.g. Cesarani, Masuda et al. Citation2021) and beef cattle (e.g. Lourenco, Tsuruta et al. Citation2015; Lee et al. Citation2017), pigs (e.g. Christensen et al. Citation2012), broiler chickens (e.g. Lourenco, Fragomeni et al. Citation2015), laying hens (e.g. Yan et al. Citation2018), sheep (e.g. Cesarani, Gaspa et al. Citation2019; Nilforooshan Citation2020), goat (e.g. Teissier et al. Citation2018), turkey (e.g. Emamgholi Begli et al. Citation2021), rainbow trout (e.g. Gonzalez-Pena et al. Citation2016), buffalo (e.g. Aspilcueta-Borquis et al. 2015 ; Cesarani, Biffani et al. Citation2021), catfish (e.g. Garcia et al. Citation2018), honey bees (e.g. Gupta et al. Citation2013), horses (e.g. Dugué et al. Citation2021), and rabbits (e.g. Mancin et al. Citation2021), among others. The models used in ssGBLUP studies include single and multiple-trait models, with or without permanent environmental and maternal effects (e.g. Lourenco, Tsuruta et al. Citation2015), random regression (e.g. Oliveira et al. Citation2019), reaction norm (e.g. Zhang et al. Citation2019), threshold (e.g. Bermann, Legarra et al. Citation2021), and survival models (e.g. Vallejo et al. Citation2019).
Present and future challenges
Currently, ssGBLUP is the method of choice for genomic evaluations in most livestock species, where not all the animals in the evaluations have genotypes. The current state of the art allows implementing most of the models used in animal breeding for a very large number of genotyped animals. This is possible due to the wide availability of efficient software packages (BLUPF90, Misztal, Tsuruta et al. Citation2014; BOLT, Garrick et al. Citation2018; DMU, Madsen et al. Citation2014; Mix99, Lidauer et al. Citation2015; MixBLUP, Mulder et al. Citation2012). Applying complex models such as social interaction models or including dominance, epistasis, and genotype by environment interactions is still challenging when the number of genotypes is large (Varona et al. Citation2018).
Current research topics in single-step include modelling missing pedigrees (Masuda, VanRaden et al. Citation2021), integrating markers selected from whole-genome sequence data (Liu et al. Citation2020), incorporating omics features such as transcriptomics and metabolomics in the genetic evaluations (Christensen et al. Citation2021), crossbreed evaluations (Alvarenga et al. Citation2020; VanRaden et al. Citation2020), GWAS (Aguilar et al. Citation2019), among others.
For genomic evaluations, we foresee a massive amount of information in the form of omics, images and videos of animals’ behaviour, and whole-genome sequence. This information may help define new phenotypes and improve phenotype prediction. However, it is not clear whether this data would help increase the accuracy of ssGBLUP predictions. Nonetheless, the key would be to find a parsimonious deal between incorporating these new sources of information and the simplicity and efficiency of ssGBLUP genomic evaluations. These new data create the need to increase the efficiency and flexibility of ssGBLUP continuously. Thus, ongoing research aims to make ssGBLUP an efficient tool for constantly growing datasets. These research topics include an approximation of theoretical accuracies of estimated breeding values, improving convergence of the solving algorithms, increasing the efficiency for categorical traits analysis, and calculating p-values for large-scale ssGWAS.
The availability of software and various datasets allow testing different models and methodologies based on single-step. Almost all studies draw conclusions based on cross-validation (Thompson Citation2001; Gianola and Schön Citation2016; Legarra and Reverter Citation2018). However, cross-validation results depend highly on the data and the animals selected for the validation set. Thus, cross-validation studies may not consider peculiarities of animal breeding such as population structure, selection, selective genotyping, among others. We believe that there is space for research on different methods for model selection in ssGBLUP. New approaches would complement cross-validation and allow making trustworthy decisions for ssGBLUP research or genomic evaluations.
Disclosure statement
No potential conflict of interest was reported by the author(s).
Additional information
Funding
References
- Aguilar I, Legarra A, Cardoso F, Masuda Y, Lourenco D, Misztal I. 2019. Frequentist p-values for large-scale-single step genome-wide association, with an application to birth weight in American Angus cattle. Genet Sel E. 51(1):28.
- Aguilar I, Misztal I, Johnson DL, Legarra A, Tsuruta S, Lawlor TJ. 2010. Hot topic: a unified approach to utilize phenotypic, full pedigree, and genomic information for genetic evaluation of Holstein final score. J Dairy Sci. 93(2):743–752.
- Alvarenga AB, Veroneze R, Oliveira HR, Marques D, Lopes PS, Silva FF, Brito LF. 2020. Comparing alternative single-step GBLUP approaches and training population designs for genomic evaluation of crossbred animals. Front Genet. 11:263.
- Ben Zaabza H, Mäntysaari EA, Strandén I. 2020. Using Monte Carlo method to include polygenic effects in calculation of SNP-BLUP model reliability. J Dairy Sci. 103(6):5170–5182.
- Ben Zaabza H, Mäntysaari EA, Strandén I. 2021. Estimation of individual animal SNP-BLUP reliability using full Monte Carlo sampling. JDS Communications. 2(3):137–141.
- Bermann M, Legarra A, Hollifield MK, Masuda Y, Lourenco D, Misztal I. 2021. Validation of single-step GBLUP genomic predictions from threshold models using the linear regression method: an application in chicken mortality. J Anim Breed Genet. 138(1):4–13.
- Bermann M, Lourenco D, Misztal I. 2021. Efficient approximation of reliabilities for single-step genomic BLUP models with the Algorithm for Proven and Young. J. Anim. Sci. 100(1):skab353.
- Bernal Rubio YL, Gualdron Duarte JL, Bates RO, Ernst CW, Nonneman D, Rohrer GA, King A, Shackelford SD, Wheeler TL, Cantet RJC, et al. 2016. Meta-analysis of genome-wide association from genomic prediction models. Anim Genet. 47(1):36–48.
- Boichard D, Fritz S, Rossignol MN, Boscher MY, Malafosse A, Colleau JJ. 2002. Implementation of marker-assisted selection in dairy cattle. In ‘Proceedings of the 7th world congress of genetics applied to livestock production, Montpellier, France’. Communication no. 22–03.
- Bradford HL, Pocrnić I, Fragomeni BO, Lourenco D, Misztal I. 2017. Selection of core animals in the Algorithm for Proven and Young using a simulation model. J Anim Breed Genet. 134(6):545–552.
- Cesarani A, Biffani S, Garcia A, Lourenco D, Bertolini G, Neglia G, Misztal I, Macciotta NPP. 2021. Genomic investigation of milk production in Italian buffalo. Ital. J. Anim. Sci. 20(1):539–547.
- Cesarani A, Gaspa G, Correddu F, Cellesi M, Dimauro C, Macciotta NPP. 2019. Genomic selection of milk fatty acid composition in Sarda dairy sheep: effect of different phenotypes and relationship matrices on heritability and breeding value accuracy. J Dairy Sci. 102(4):3189–3203.
- Cesarani A, Masuda Y, Tsuruta S, Nicolazzi EL, VanRaden PM, Lourenco D, Misztal I. 2021. Genomic predictions for yield traits in US Holsteins with unknown parent groups. J Dairy Sci. 104(5):5843–5853.
- Cesarani A, Pocrnic I, Macciotta NPP, Fragomeni BO, Misztal I, Lourenco D. 2019. Bias in heritability estimates from genomic restricted maximum likelihood methods under different genotyping strategies. J Anim Breed Genet. 136(1):40–50.
- Christensen OF, Börner V, Varona L, Legarra A. 2021. Genetic evaluation including intermediate omics features. Genetics. 219(2):iyab130.
- Christensen OF, Lund MS. 2010. Genomic prediction when some animals are not genotyped. Genet. Sel. Evol. 42(1):2.
- Christensen OF, Madsen P, Nielsen B, Ostersen T, Su G. 2012. Single-step methods for genomic evaluation in pigs. Animal. 6(10):1565–1571.
- Christensen OF. 2012. Compatibility of pedigree-based and marker-based relationship matrices for single-step genetic evaluation. Genet Sel Evol. 44(1):37.
- Cole JB, VanRaden PM, O′Connell JR, Van Tassell CP, Sonstegard TS, Schnabel RD, Taylor JF, Wiggans GR. 2009. Distribution and location of genetic effects for dairy traits. J Dairy Sci. 92(6):2931–2946.
- Colleau JJ. 2002. An indirect approach to the extensive calculation of relationship coefficients. Genet. Sel. Evol. 34(4):409–421.
- Cuyabano B, Sørensen AC, Sørensen P. 2018. Understanding the potential bias of variance components estimators when using genomic models. Genet Sel E. 50(1):41.
- Dugué M, Dumont Saint Priest B, Crichan H, Danvy S, Ricard A. 2021. Genomic correlations between the gaits of young horses measured by accelerometry and functional longevity in jumping competition. Front Genet. 12:619947.
- Edel C, Pimentel E, Erbe M, Emmerling R, Götz KU. 2019. Short communication: calculating analytical reliabilities for single-step predictions. J Dairy Sci. 102(4):3259–3265.
- Emamgholi Begli H, Schaeffer LR, Abdalla E, Lozada-Soto EA, Harlander-Matauschek A, Wood BJ, Baes CF. 2021. Genetic analysis of egg production traits in turkeys (Meleagris gallopavo) using a single-step genomic random regression model. Genet Sel E. 53(1):61.
- Falconer D, Mackay T.Introduction to Quantitative Genetics, Longman Essex, New York 1996. -, -p. 111–111. 3rd .
- Fernando R, Grossman M. 1989. Marker assisted selection using best linear unbiased prediction. Genet Sel Evol. 21(4):467.
- Fernando RL, Cheng H, Garrick DJ. 2016. An efficient exact method to obtain GBLUP and single-step GBLUP when the genomic relationship matrix is singular. Genet Sel E. 48(1):80.
- Fernando RL, Cheng H, Golden BL, Garrick DJ. 2016. Computational strategies for alternative single-step Bayesian regression models with large numbers of genotyped and non-genotyped animals. Genet Sel E. 48(1):96.
- Fernando RL, Dekkers JC, Garrick DJ. 2014. A class of Bayesian methods to combine large numbers of genotyped and non-genotyped animals for whole-genome analyses. Genet Sel Evol. 46 (1):50.
- Fisher RA. 1919. The causes of human variability. The Eugenics Rev. 10(4):213–220.
- Forni S, Aguilar I, Misztal I. 2011. Different genomic relationship matrices for single-step analysis using phenotypic, pedigree and genomic information. Genet Sel Evol. 43(1):1.
- Fragomeni BO, Lourenco DA, Tsuruta S, Masuda Y, Aguilar I, Misztal I. 2015. Use of genomic recursions and algorithm for proven and young animals for single-step genomic BLUP analyses-a simulation study. J Anim Breed Genet. 132(5):340–345.
- Gao H, Christensen OF, Madsen P, Nielsen US, Zhang Y, Lund MS, Su G. 2012. Comparison on genomic predictions using three GBLUP methods and two single-step blending methods in the Nordic Holstein population. Genet Sel Evol. 44(1):8. 8.
- Garcia A, Bosworth B, Waldbieser G, Misztal I, Tsuruta S, Lourenco D. 2018. Development of genomic predictions for harvest and carcass weight in channel catfish. Genet Sel E. 50(1):66.
- Garcia-Baccino CA, Legarra A, Christensen OF, Misztal I, Pocrnic I, Vitezica ZG, Cantet RJ. 2017. Metafounders are related to Fst fixation indices and reduce bias in single-step genomic evaluations. Genet. Sel. E. 49(1):34.
- Garrick DJ, Garrick DP, Golden BL. 2018. An introduction to BOLT software for genetic and genomic evaluations. In Proceedings of The 11th World Congress on Genetics Applied to Livestock Production; February (Vol. 11, p. 973).
- Gianola D, de los Campos G, Hill WG, Manfredi E, Fernando R. 2009. Additive genetic variability and the Bayesian alphabet. Genetics. 183(1):347–363.
- Gianola D, Schön CC. 2016. Cross-Validation Without Doing Cross-Validation in Genome-Enabled Prediction. G3 (Bethesda). 6(10):3107–3128.
- Gonzalez-Pena D, Gao G, Baranski M, Moen T, Cleveland BM, Kenney PB, Vallejo RL, Palti Y, Leeds TD. 2016. Genome-Wide Association Study for Identifying Loci that Affect Fillet Yield, Carcass, and Body Weight Traits in Rainbow Trout (Oncorhynchus mykiss). Front Genet. 7:203.
- Gupta P, Reinsch N, Spötter A, Conrad T, Bienefeld K. 2013. Accuracy of the unified approach in maternally influenced traits-illustrated by a simulation study in the honey bee (Apis mellifera). BMC Genet. 14:36.
- Harris BL, Johnson DL. 2010. Genomic predictions for New Zealand dairy bulls and integration with national genetic evaluation. J Dairy Sci. 93(3):1243–1252.
- Henderson CR. 1975. Best Linear Unbiased Estimation and Prediction under a Selection Model. Biometrics. 31(2):423–447.
- Henderson CR. 1976. A Simple Method for Computing the Inverse of a Numerator Relationship Matrix Used in Prediction of Breeding Values. Biometrics. 32(1):69–83.
- Henderson CR. 1984. Applications of linear models in animal breeding. University of Guelph Press, Guelph, Canada.
- Hidalgo J, Tsuruta S, Lourenco D, Masuda Y, Huang Y, Gray KA, Misztal I. 2020. Changes in genetic parameters for fitness and growth traits in pigs under genomic selection. J. Anim. Sci. 98(2):skaa032.
- Hofer A. 1998. Variance component estimation in animal breeding: a review†. J Anim Breed Genet. 115(1-6):247–265.
- Junqueira VS, Lopes PS, Lourenco D, Silva FFE, Cardoso FF. 2020. Applying the metafounders approach for genomic evaluation in a multibreed beef cattle population. Front Genet. 11:556399.
- Kang HM, Sul JH, Service SK, Zaitlen NA, Kong SY, Freimer NB, Sabatti C, Eskin E. 2010. Variance component model to account for sample structure in genome-wide association studies. Nat Genet. 42(4):348–354.
- Kang HM, Zaitlen NA, Wade CM, Kirby A, Heckerman D, Daly MJ, Eskin E. 2008. Efficient control of population structure in model organism association mapping. Genetics. 178(3):1709–1723.
- Kluska S, Masuda Y, Ferraz J, Tsuruta S, Eler JP, Baldi F, Lourenco D. 2021. Metafounders May Reduce Bias in Composite Cattle Genomic Predictions. Front Genet. 12:678587.
- Lee J, Cheng H, Garrick D, Golden B, Dekkers J, Park K, Lee D, Fernando R. 2017. Comparison of alternative approaches to single-trait genomic prediction using genotyped and non-genotyped Hanwoo beef cattle. Genet Sel E. 49(1):2. 2.
- Legarra A, Aguilar I, Misztal I. 2009. A relationship matrix including full pedigree and genomic information. J Dairy Sci. 92(9):4656–4663.
- Legarra A, Christensen OF, Vitezica ZG, Aguilar I, Misztal I. 2015. Ancestral relationships using metafounders: finite ancestral populations and across population relationships. Genetics. 200(2):455–468.
- Legarra A, Ducrocq V. 2012. Computational strategies for national integration of phenotypic, genomic, and pedigree data in a single-step best linear unbiased prediction. J Dairy Sci. 95(8):4629–4645.
- Legarra A, Reverter A. 2018. Semi-parametric estimates of population accuracy and bias of predictions of breeding values and future phenotypes using the LR method. Genet. Sel. E. 501:53.
- Lidauer M, Matilainen K, Mantysaari E, Pitkanen T, Taskinen M, Stranden I. 2015. Technical Reference Guide for MiX99 Solver. Natural Resources Institute Finland: Jokioinen, Finland.
- Liu A, Lund MS, Boichard D, Karaman E, Guldbrandtsen B, Fritz S, Aamand GP, Nielsen US, Sahana G, Wang Y, et al. 2020. Weighted single-step genomic best linear unbiased prediction integrating variants selected from sequencing data by association and bioinformatics analyses. Genet Sel E. 52(1):48.
- Liu Z, Goddard ME, Hayes BJ, Reinhardt F, Reents R. 2016. Technical note: equivalent genomic models with a residual polygenic effect. J Dairy Sci. 99(3):2016–2025.
- Liu Z, Goddard ME, Reinhardt F, Reents R. 2014. A single-step genomic model with direct estimation of marker effects. J Dairy Sci. 97(9):5833–5850.
- Liu Z, VanRaden PM, Lidauer MH, Calus MP, Benhajali H, Jorjani H, Ducrocq V. 2017. Approximating genomic reliabilities for national genomic evaluation. Interbull Bull. 51:75–85.
- Lourenco DA, Fragomeni BO, Tsuruta S, Aguilar I, Zumbach B, Hawken RJ, Legarra A, Misztal I. 2015. Accuracy of estimated breeding values with genomic information on males, females, or both: an example on broiler chicken. Genet. Sel. E. 47(1):56.
- Lourenco DA, Tsuruta S, Fragomeni BO, Masuda Y, Aguilar I, Legarra A, Bertrand JK, Amen TS, Wang L, Moser DW, et al. 2015. Genetic evaluation using single-step genomic best linear unbiased predictor in American Angus. J Anim Sci. 93(6):2653–2662.
- Lourenco DAL, Legarra A, Tsuruta S, Moser D, Miller S, Misztal I. 2018. Tuning indirect predictions based on SNP effects from single-step GBLUP. Interbull Bull. 53:48–53.
- Madsen P, Jensen J, Labouriau R, Christensen OF, Sahana G. 2014. DMU—A Package for Analyzing Multivariate Mixed Models in quantitative Genetics and Genomics. In Proceedings of the 10th World Congress of Genetics Applied to Livestock Production, Vancouver, BC, Canada, 17–22 August.
- Mancin E, Sosa-Madrid BS, Blasco A, Ibáñez-Escriche N. 2021. Genotype Imputation to Improve the Cost-Efficiency of Genomic Selection in Rabbits. Animals. 11(3):803.
- Mäntysaari EA, Evans RD, Strandén I. 2017. Efficient single-step genomic evaluation for a multibreed beef cattle population having many genotyped animals. J Anim Sci. 95(11):4728–4737.
- Mäntysaari EA, Koivula M, Strandén I. 2020. Symposium review: single-step genomic evaluations in dairy cattle. J Dairy Sci. 103(6):5314–5326.
- Masuda Y, Misztal I, Legarra A, Tsuruta S, Lourenco DA, Fragomeni BO, Aguilar I. 2017. Technical note: avoiding the direct inversion of the numerator relationship matrix for genotyped animals in single-step genomic best linear unbiased prediction solved with the preconditioned conjugate gradient. J. Anim. Sci. 95(1):49–52.
- Masuda Y, Misztal I, Tsuruta S, Legarra A, Aguilar I, Lourenco D, Fragomeni BO, Lawlor TJ. 2016. Implementation of genomic recursions in single-step genomic best linear unbiased predictor for US Holsteins with a large number of genotyped animals. J Dairy Sci. 99(3):1968–1974.
- Masuda Y, Tsuruta S, Bermann M, Bradford HL, Misztal I. 2021. Comparison of models for missing pedigree in single-step genomic prediction. J. Anim. Sci. 99(2):skab019.
- Masuda Y, VanRaden PM, Tsuruta S, Lourenco D, Misztal I. 2021. Invited review: unknown-parent groups and metafounders in single-step genomic BLUP. J Dairy Sci.105(2):923-939.
- Meuwissen TH, Hayes BJ, Goddard ME. 2001. Prediction of total genetic value using genome-wide dense marker maps. Genetics. 157(4):1819–1829.
- Misztal I, Legarra A, Aguilar I. 2009. Computing procedures for genetic evaluation including phenotypic, full pedigree, and genomic information. J Dairy Sci. 92(9):4648–4655.
- Misztal I, Legarra A, Aguilar I. 2014. Using recursion to compute the inverse of the genomic relationship matrix. J Dairy Sci. 97(6):3943–3952.
- Misztal I, Tsuruta S, Aguilar I, Legarra A, VanRaden PM, Lawlor TJ. 2013. Methods to approximate reliabilities in single-step genomic evaluation. J Dairy Sci. 96(1):647–654.
- Misztal I, Tsuruta S, Lourenco DAL, Masuda Y, Aguilar I, Legarra A, Vitezica Z. 2014. Manual for BLUPF90 family of programs.
- Misztal I, Vitezica ZG, Legarra A, Aguilar I, Swan AA. 2013. Unknown-parent groups in single-step genomic evaluation. J Anim Breed Genet. 130(4):252–258.
- Misztal I. 2016. Inexpensive Computation of the Inverse of the Genomic Relationship Matrix in Populations with Small Effective Population Size. Genetics. 202(2):401–409.
- Mulder HA, Lidauer M, Strandén I, Mäntysaari EA, Pool MH, Veerkamp RF. 2012. MiXBLUP Manual. Animal Breeding and Genomics Centre, Wageningen UR Livestock Research, Lelystad, The Netherlands
- Nilforooshan MA, Lee M. 2019. The quality of the algorithm for proven and young with various sets of core animals in a multibreed sheep population1. J Anim Sci. 97(3):1090–1100.
- Nilforooshan MA. 2020. Application of single-step GBLUP in New Zealand Romney sheep. Anim Prod Sci. 60(9):1136–1144.
- Ødegård J, Indahl U, Strandén I, Meuwissen T. 2018. Large-scale genomic prediction using singular value decomposition of the genotype matrix. Genet Sel E. 50(1):6. 6.
- Oliveira HR, Lourenco D, Masuda Y, Misztal I, Tsuruta S, Jamrozik J, Brito LF, Silva FF, Schenkel FS. 2019. Application of single-step genomic evaluation using multiple-trait random regression test-day models in dairy cattle. J Dairy Sci. 102(3):2365–2377.
- Patry C, Ducrocq V. 2011. Evidence of biases in genetic evaluations due to genomic preselection in dairy cattle. J Dairy Sci. 94(2):1011–1020.
- Pearson K. 1903. Mathematical contributions to the theory of evolution. —XI. On the influence of natural selection on the variability and correlation of organs. Philosophical Transactions of the Royal Society of London. Series A, Containing Papers of a Mathematical or Physical Character 2001–66.
- Pimentel E, Edel C, Emmerling R, Götz KU. 2019. Technical note: methods for interim prediction of single-step breeding values for young animals. J Dairy Sci. 102(4):3266–3273.
- Pocrnic I, Lourenco DA, Masuda Y, Misztal I. 2016. Dimensionality of genomic information and performance of the Algorithm for Proven and Young for different livestock species. Genet. Sel. E. 48(1):82.
- Powell JE, Visscher PM, Goddard ME. 2010. Reconciling the analysis of IBD and IBS in complex trait studies. Nat Rev Genet. 11(11):800–805.
- Quaas RL, Pollak EJ. 1981. Modified equations for sire mod- els with groups. J. Dairy Sci. 64(9):1868–1872. https://doi.org/10.3168/jds.S0022-0302(81)82778-6.
- Quaas RL. 1976. Computing the Diagonal Elements and Inverse of a Large Numerator Relationship Matrix. Biometrics. 32(4):949–953.
- Quaas RL. 1988. Additive Genetic Model with Groups and Relationships. J. Dairy Sci. 71(5):1338–1345.
- Strandén I, Garrick DJ. 2009. Technical note: derivation of equivalent computing algorithms for genomic predictions and reliabilities of animal merit. J Dairy Sci. 92(6):2971–2975.
- Strandén I, Matilainen K, Aamand GP, Mäntysaari EA. 2017. Solving efficiently large single-step genomic best linear unbiased prediction models. J Anim Breed Genet. 134(3):264–274.
- Taskinen M, Mäntysaari EA, Strandén I. 2017. Single-step SNP-BLUP with on-the-fly imputed genotypes and residual polygenic effects. Genet Sel E. 49(1):36.
- Teissier M, Larroque H, Robert-Granié C. 2018. Weighted single-step genomic BLUP improves accuracy of genomic breeding values for protein content in French dairy goats: a quantitative trait influenced by a major gene. Genet Sel E. 50(1):31.
- Thompson R. 2001. Statistical validation of genetic models. Livestock Production Science. 72(1-2):129–134. Volume IssuesPages ISSN 0301-6226,.
- Tsuruta S, Lourenco DAL, Masuda Y, Misztal I, Lawlor TJ. 2019. Controlling bias in genomic breeding values for young genotyped bulls. J Dairy Sci. 102(11):9956–9970.
- Vallejo RL, Cheng H, Fragomeni BO, Shewbridge KL, Gao G, MacMillan JR, Towner R, Palti Y. 2019. Genome-wide association analysis and accuracy of genome-enabled breeding value predictions for resistance to infectious hematopoietic necrosis virus in a commercial rainbow trout breeding population. Genet. Sel. E. 51(1):47.
- Vandenplas J, Calus M, Eding H, Vuik C. 2019. A second-level diagonal preconditioner for single-step SNPBLUP. Genet Sel E. 51(1):30.
- Vandenplas J, Calus M, Ten Napel J. 2018. Sparse single-step genomic BLUP in crossbreeding schemes. J Anim Sci. 96(6):2060–2073.
- Vandenplas J, Eding H, Calus M, Vuik C. 2018. Deflated preconditioned conjugate gradient method for solving single-step BLUP models efficiently. Genet Sel E. 50(1):51.
- VanRaden PM, Tooker ME, Chud T, Norman HD, Megonigal JH, Haagen IW, Jr., Wiggans GR. 2020. Genomic predictions for crossbred dairy cattle. J Dairy Sci. 103(2):1620–1631.
- VanRaden PM. 2008. Efficient methods to compute genomic predictions. J. Dairy Sci. 91(11):4414–4423.
- Varona L, Legarra A, Toro MA, Vitezica ZG. 2018. Non-additive Effects in Genomic Selection. Front Genet. 9:78.
- Vitezica ZG, Aguilar I, Misztal I, Legarra A. 2011. Bias in genomic predictions for populations under selection. Genet Res (Camb). 93(5):357–366.
- Wang H, Misztal I, Aguilar I, Legarra A, Muir WM. 2012. Genome-wide association mapping including phenotypes from relatives without genotypes. Genet Res (Camb). 94(2):73–83.
- Westell RA, Quaas RL, Van Vleck LD. 1988. Genetic groups in an animal model. J. Dairy Sci. 71(5):1310–1318.
- Xiang T, Christensen OF, Legarra A. 2017. Technical note: genomic evaluation for crossbred performance in a single-step approach with metafounders. J Anim Sci. 95(4):1472–1480.
- Yan Y, Wu G, Liu A, Sun C, Han W, Li G, Yang N. 2018. Genomic prediction in a nuclear population of layers using single-step models. Poult Sci. 97(2):397–402.
- Zhang Z, Kargo M, Liu A, Thomasen JR, Pan Y, Su G. 2019. Genotype-by-environment interaction of fertility traits in Danish Holstein cattle using a single-step genomic reaction norm model. Heredity (Edinb). 123(2):202–214.
- Zhang Z, Liu J, Ding X, Bijma P, de Koning DJ, Zhang Q. 2010. Best linear unbiased prediction of genomic breeding values using a trait-specific marker-derived relationship matrix. PLoS One. 5(9):e12648.