Abstract
For many years, microbiology was based on culture-dependent methods to study microorganisms; however, the latest technologies are culture-independent and their development has generated large amounts of new data, allowing researchers to understand the true nature and complexity of animals’ microbiomes. The myriad of microorganisms living in a symbiotic relationship with animals, primarily in their gastrointestinal tract (GIT), interact with the host animal in many different ways, ultimately affecting the animal’s health and productivity. The purpose of this article is to address the use of microbiome information through the lens of animal science. It covers some best practices for performing microbiome studies, from proper sample collection to useful data analysis tools and packages. It also provides examples of how the microbiome can interact with the host to impact its health and productivity. Lastly, we present some future perspectives for animal microbiome research and how the information generated can be utilised for more accurate comparison across studies and integrated with other branches of the animal sciences so the data produced can have a larger, more beneficial impact.
DNA sequencing costs have sharply dropped in the last two decades, enabling more accessibility for studies to utilise nucleotide sequencing technologies to investigate microbiomes.
There is increasing evidence that the microorganisms living on, and especially inside a host animal, can affect animal health and performance-related traits.
This review addresses utilising microbiome information in Animal Science and suggests some best practices for performing microbiome studies, which spans from sample collection to data analysis.
Highlights
Introduction
Present-day technologies have allowed us to comprehend the extraordinary number of microbes living on and within all livestock animals (Shang et al. Citation2018; O’Hara et al. Citation2020). These microorganisms harbour an enormous collection of genes, which vastly outnumber the number of genes contained in the host animal’s own genome (Bashiardes et al. Citation2016; Kogut Citation2019). Consequently, they can interact with and affect many processes in the animal’s body, and potentially modulate animal health and performance. As an illustration, many of the microorganisms located in the gastrointestinal tract (GIT) act as a consortium to degrade certain portions of the digesta that would otherwise not be utilised by the host animal and convert it into useful end products, such as volatile fatty acids (VFA) and water-soluble vitamins (B-complex vitamins and vitamin C;(Pham et al. Citation2021)), which are later utilised by the animal.
Several approaches are currently available for studying the microbiome, which can be technically defined as the collection of all genetic material from the microbes within a given environment (Turnbaugh et al. Citation2007; Allaband et al. Citation2019), and the microbiota, defined as the collection of all microorganisms living within a defined environment (Allaband et al. Citation2019). These approaches typically use sample-specific barcodes that allow hundreds of communities to be analysed simultaneously in multiplex (Caporaso et al. Citation2010). In this method, the barcodes can be defined as short, known DNA sequences that are attached to the samples, making it possible to retrieve information about the samples at later stages by searching for those known sequence of nucleotides. Regardless ofthe approach used to obtain such information (e.g. shotgun metagenomic sequencing or simply using a marker gene), the resulting datasets are normally large, complex, and typically require specific bioinformatics approaches to be disentangled (Caporaso et al. Citation2010; Callahan et al. Citation2016; Bolyen et al. Citation2019). Thus being, in addition to affordability, a wider adoption and integration of microbiome data in animal science studies may require new software that are more user-friendly and allow the end-user to run the analysis in a remote server without the need to invest in powerful computing equipment. In order to increase the prevalence of microbiome studies related to the animal sciences, this layer of complexity must be removed from the potential end-users which are more familiar with biological processes than bioinformatics. Fortunately, projects like the metagenomics RAST server (Meyer et al. Citation2008) and Galaxy (Afgan et al. Citation2018) represent solid steps in reducing the complexity required for data analysis.
In addition to pure microbiome studies, there has been a widespread adoption and popularisation of the ‘multi-omics’ approaches in all biological sciences in recent years. Such popularisation is the result of a combination of many factors, among which affordability is certainly included. The remarkable progress observed in nucleotide sequencing technologies has not only accelerated the entire process, but has also sharply decreased the cost of obtaining genetic information (Goodwin et al. Citation2016). For instance, data gathered by the National Human Genome Research Institute for the last two decades reveals a striking reduction in DNA sequencing costs, achieved mainly due to the transition to newer (next-generation) DNA sequencing technologies (Figure ).
With the expected exponential growth of the world’s population, the efficiency of food production from animals and crops must increase (Berry and Crowley Citation2013). Thus, it is no surprise that the entire field of animal science is committed to improving animal health, productivity and the quality of animal products while decreasing the use of inputs and chemicals (such as antibiotics) and reducing excretions to the environment. Presently, there is a plethora of evidence indicating that the use of microbiome information can help address these issues more precisely (Ding et al. Citation2019; Kogut Citation2019; Xue et al. Citation2020; Lourenco et al. Citation2021).
One means to increase food production from animals is naturally by increasing the efficiency of animal-producing systems. Moreover, since feed costs make up approximately 75% of production costs in beef operations, 40–60% of production costs in dairy systems, and up to 70% of the total cost share of swine production (Bach Citation2012; Finneran et al. Citation2012; Mintert Citation2021), improving feed efficiency is clearly a means to increase animal production while also increasing profitability. Considerable research has focussed on elucidating the factors influencing animals’ feed efficiency, and in ruminants, some estimates indicate that 19% of the variation in feed efficiency is a result of diet and its digestibility; however, 81% of this variation remains not fully understood (Herd and Arthur Citation2009). The microbes inhabiting the GIT appear to be reasonable candidates for explaining (at least in part) this remaining variation in feed efficiency.
This review aims to address the use of microbiome information in the context of the animal sciences and indicate some best practices for carrying out microbiome studies, from the collection of samples to data analysis. We will also show some examples that demonstrate how the microbiome can impact animal health and productivity, as well as present some future perspectives on studies involving animal microbiomes.
Obtaining microbiome data
Due to the nature of many animal science studies (i.e. performed in the field and not a controlled environment), the collection of samples may represent the first challenge when investigating microbiomes. In fact, sample collection can be a significant source of errors and introduction of confounding factors, which can affect the results and interpretation of an entire study (Bharti and Grimm Citation2021). Thus being, obtention of reliable samples for microbiome studies begin by using DNA/RNA-free containers and other materials, such as swabs and gloves. It is important to note that DNA/RNA-free and sterile are not synonyms, since sterile material may still contain DNA or RNA fragments, which may end up as extraneous contaminants. Besides using nucleotide-free collection equipment, the samples should be frozen immediately after collection and stored as soon as possible (preferably at −80 °C) to conserve all the microbial diversity (Bharti and Grimm Citation2021). Utilisation of nucleic acid stabilisers is a practice that should be used whenever possible. The quantity of specimen used in microbiome analysis is typically less than 1 g for most protocols. Additionally, it has been shown that the microbial community profile of the liquid and solid portions of ruminal samples are different (Henderson et al. Citation2013), so, in order to minimise error and standardise comparisons across studies, microbiome investigators should also consider the type of sample they plan to collect.
Another important source of bias in microbiome studies is the method used for nucleic acid extraction and purification. Ideally, the method used should effectively capture all types of microbes present in the samples. In a study that compared fifteen different methods to extract DNA, Henderson et al. found that the absolute microbial numbers determined in the same set of samples varied by more than 100-fold depending on the DNA extraction method used (Henderson et al. Citation2013). One of the most challenging types of bacteria to obtain an accurate representation of during the extraction process are the Gram-positives. Though both Gram-positive and Gram-negative cell walls have a peptidoglycan layer, the peptidoglycan layer of Gram-positive cells has a greater quantity, thickness and the degree of crosslinking (Mahalanabis et al. Citation2009) making it much harder to lyse open and extract DNA from Gram-positive cells (Mahalanabis et al. Citation2009; Abbas and AL Musawi Citation2016). Mechanical, and specially hybrid (mechanical plus enzymatic) extraction protocols that utilise bead-beating techniques can lead to increased abundance of Gram-positive phyla compared to enzymatic extractions (Rothrock et al. Citation2014), since they are better able to break through the peptidoglycan layer. However, excessive mechanical disruption of the cells can be detrimental and end up fragmenting the DNA. As DNA extraction kits introduce biases that affect all the samples equally, these biases can be controlled and mitigated by the use of the same batch of reagents and by including proper negative (‘blank-swab’, ‘blank-extraction’ and ‘blank-library’) and positive (‘mock communities’) technical controls (Fidler et al. Citation2020).
Before using DNA samples in downstream analyses, the quality and concentration must be determined. Although the elution buffer can impact the results, typically, DNA purity can be evaluated by spectrophotometry assessing the UV absorbance of the eluate at 260 and 280 nm. The ratio of absorbance at 260 nm to absorbance at 280 nm is calculated, and DNA is considered ‘pure’ if this ratio is between 1.7 and 1.9. Ratios lower than 1.6 may indicate presence of proteins or other contaminants. Conversely, ratios that are significantly greater than 1.9 may indicate presence of RNA (Lucena-Aguilar et al. Citation2016). DNA concentration can be determined using an equation derived from the Lambert–Beer law, in which absorbance at 260 nm is proportional to the concentration of DNA in solution, and values between 0.1 and 1.0 are considered reliable (Lucena-Aguilar et al. Citation2016; Koetsier and Cantor Citation2019). Utilisation of fluorescence-based methods may yield results that are more accurate than UV absorbances, especially when dealing with lower concentrations. Moreover, if necessary, DNA length and integrity can be evaluated by pulsed-field gel electrophoresis through agarose gel.
DNA primers and sequencing technologies
Following extraction/purification, the microbial DNA is amplified, most commonly by using a phylogenetic marker gene such as the 16S rRNA gene due to its highly conserved and variable regions as well as an extensive database of reference sequences. The highly conserved regions of the gene are used for primer design; whereas, the hypervariable regions are used to identify the phylogenetic characteristics of the microorganisms. Because of these unique features, the 16S rRNA gene has become the most widely used marker gene for profiling prokaryotic communities, particularly bacteria (Klindworth et al. Citation2013; Yang et al. Citation2016; Johnson et al. Citation2019; Bharti and Grimm Citation2021).
The 16S rRNA gene contains approximately 1500 base pairs and has nine hypervariable regions which are separated by the highly conserved regions (Figure ). Although sequencing the entire gene is an option, most studies end up targeting only one or just a few hypervariable regions of the gene for taxonomic determination, mainly due to cost and practicality. However, the accuracy of analyses involving the 16S rRNA gene depends strongly on the choice of primers (Klindworth et al. Citation2013). Moreover, using some particular regions of the gene may be more informative depending on the microbiome of interest and the experimental objectives (Illumina Citation2013; McCann et al. Citation2014). Which region of the gene to sequence is an active area of research, but there is data indicating that, overall, targeting the V3–V4 region yields best results (Illumina Citation2013; Klindworth et al. Citation2013; Thijs et al. Citation2017). For example, Thijs et al. found that the primer pair 341f/785r (which is used to amplify the V3–V4 region) showed the highest taxonomic coverage, produced the highest number of bacterial OTUs, phylogenetic richness and diversity and had the best agreement with qPCR data, indicating that this primer pair is one of the most promising for microbiome studies (Thijs et al. Citation2017). Primers for other microorganisms, such as Archaea, Fungi and Protozoa are also available. Klindworth et al. described a series of primers that can simultaneously amplify Bacteria and Archaea (Klindworth et al. Citation2013); however, primers designed specifically for Archaea, such as the primer pair Met630F and Met803R typically have greater coverage (higher than 90%) of the archaeal domain (Wallace et al. Citation2015). Protozoal populations can be studied using PCR approaches that amplify the 18S rRNA gene as described in detail by Sylvester et al. (Citation2004). Similarly, Fungi can be studied either by amplification of the 18S rRNA gene (Banos et al. Citation2018), or by fungal-specific PCR primers developed for analysis of the internal transcribed spacer (ITS) regions of fungal ribosomal DNA (Martin and Rygiewicz Citation2005).
Figure 2. Schematic representation of the 16S rRNA gene depicting the conserved and variable regions and some potential sections of the gene to be amplified by primers.
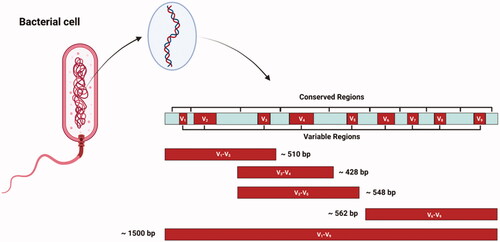
Currently, there are a few major players in the DNA sequencing platform market, namely: Illumina, Pacific Biosciences (PacBio, Menlo Park, CA), Oxford Nanopore Technologies, and Ion Torrent/Thermo Fisher Scientific. Several studies have addressed the details and the differences between these platforms (Allali et al. Citation2017; Heikema et al. Citation2020; Nygaard et al. Citation2020; Zhang et al. Citation2020). Aside from the technology each platform uses, another important aspect that differs is that they focus either on short (up to 300 bases) or long (up to 25,000 bases) reads, each with its own advantages and limitations. At the present time, long reads (e.g. PacBio) cost more than short reads (e.g. Illumina) on a per-sample basis; however, they provide a better taxonomic resolution. Still, to date, the vast majority of microbiome studies in animal science have been performed using Illumina platforms.
Processing microbiome data
The recent advances in DNA-sequencing technologies have also generated a challenge: processing the large amount of data that is generated. Currently, there are numerous software packages available for processing microbiome data including: Mothur (Schloss et al. Citation2009), QIIME (Caporaso et al. Citation2010), Phyloseq (McMurdie and Holmes Citation2013), DADA2 (Callahan et al. Citation2016) and QIIME 2 (Bolyen et al. Citation2019). Many are available as an R package (e.g. Phyloseq); whereas, others consist of comprehensive software packages which allow users to go from raw sequencing data to ready-to-publish data and figures (e.g. QIIME, Mothur and QIIME 2). Tutorials and extensive documentation on how to use these software packages are freely available online; however, they require certain bioinformatics knowledge by users, which often hinders a wider use of these tools. Additional paid tools are also available, but they are not as popular as the ones previously mentioned. Lastly, web-based analysis platforms, such as Galaxy and the metagenomics RAST server are good free options for making analysis more easily accessible to researchers (Meyer et al. Citation2008; Afgan et al. Citation2018), but are not widely used in animal science studies.
Regardless of which software/package is used for processing microbiome data, some key components must be observed during the analysis including quality-checking, dereplicating/clustering and filtering the DNA sequences to make them free of chimaeras and other possible sources of error. Quality-filtering of sequencing data typically begins by excluding low-quality sequences by selecting a minimum quality score (Phred score) and working only with high-quality sequences in downstream analyses (Bokulich et al. Citation2013). Filtering the chimeric DNA sequences formed during PCR amplification is also a common practice, which is accomplished using bioinformatics software (Edgar et al. Citation2011; Callahan et al. Citation2016). Some tool for phylogenetic alignment, generating phylogenetic trees, performing taxonomic classifications and computing alpha and beta-diversities must also be part of the analysis, since this kind of information is normally very relevant. A comprehensive overview/evaluation of microbiome analysis tools is beyond the scope of the current publication; however, the interested reader is referred to the publications by Chappidi et al. and Kim et al., both of which cover in detail the use of computational analysis of microbiome sequencing data with step-by-step instructions on how to use some relevant software (Chappidi et al. Citation2019; Kim et al. Citation2020).
In order to overcome some of the limitations of techniques, such as 16S rRNA gene or fungal ITS, shotgun DNA sequencing may be used. Shotgun sequencing involves randomly breaking up the DNA into small fragments, which are then sequenced individually. By using some bioinformatic approaches, the small DNA sequences are later reassembled to reconstitute the genome, which is achieved by looking for overlaps in the DNA sequences. In addition to detecting prokaryotes at a deeper taxonomic level (Anyansi et al. Citation2020), shotgun sequencing can detect eukaryotic organisms (e.g. protozoa) and even viruses.
Microbial diversity indices
Not only does microbiome data provide valuable information about individual microbes, but it also gives insight into the microbial populations as a whole. This allows meaningful conclusions to be made about differences in the microbial composition among samples. Alpha diversity indices summarise the structure of a community with respect to its richness (number of taxonomic groups), evenness (distribution of abundances of the groups) or both (Willis Citation2019). The resulting indices are used to compare differences within individual samples (McCann et al. Citation2014), and are usually represented by Shannon index, Faith’s phylogenetic diversity index, or Simpson’s index, although several others can be computed. Metrices of species evenness (e.g. Pielou’s evenness) and richness (e.g. Chao1) are also commonly found in literature dealing with microbial communities. The number of unique OTU (or ASV) detected in a sample is also considered a primary metric of microbial richness, although it differs from Chao1 in the fact that Chao1 attempts to estimate the total number of species in a sample while adjusting for the sampling effort (Chao Citation1984). Thus, the use of Chao1 as a richness estimator is encouraged to minimise bias, as it tries to account for unobserved species (Willis Citation2019).
As briefly mentioned above, Operational Taxonomic Unit (OTU) or Amplicon Sequence Variant (ASV) are common basic units on which microbiome studies are based on. In summary, OTUs are clusters of reads that are formed by computational algorithms based on a pre-defined sequence similarity threshold, which is usually set at 97%, and intends to serve as a proxy for species in taxonomic profiling applications. OTUs are normally generated by two methods: closed-reference or de novo methods. Closed-reference OTUs are generated by comparing the sequence reads to the ones from a reference database, whereas, de novo OTUs are formed by grouping reads as a function of their pairwise sequence similarities (Callahan et al. Citation2017). The concept of ASV has been introduced more recently in microbiome analyses, and it presents several benefits over OTUs, such as the ability to infer sample sequences more accurately, and resolve differences to the precision of one nucleotide (Callahan et al. Citation2016). Thus, microbiome analysis using ASV instead of OTU have better resolution can allow better taxonomic classifications, and there is sufficient evidence showing that ASV should replace OTU as the standard unit of marker-gene analysis and report (Callahan et al. Citation2017).
The other kind of diversity typically quantified in microbiome studies is the beta. Beta diversity represents the differences between two or more spatial units (Magurran Citation2003), and even though it is a concept first developed by ecologists, it may bring valuable insights into microbiome studies. The general hypotheses about beta diversity are: 1) species composition is uniform over large areas; 2) species composition fluctuates in a random way; and 3) species distributions are related to environmental conditions (Legendre et al. Citation2005). In animal microbiome studies beta diversity is usually represented by indices like Bray–Curtis metric, Jaccard distance or UniFrac distances (Lozupone and Knight Citation2005). The latter method has the advantage of accounting for the phylogenetic information of samples in the calculation, so it is highly recommended for microbiome studies. In most cases, beta diversity is visualised through the use of a principal coordinate analysis plot (as seen in Figure ), though other visualisation methods can also be used.
Figure 3. Alpha and beta-diversity of steers finished in feedlot. A) Bray–Curtis dissimilarity observed in the rumen. B) Bray–Curtis dissimilarity observed in the faeces. C) Shannon diversity index observed in the rumen. Weaning = weaning day; Feedlot1 = after adaptation to feedlot diet; Feedlot2 = last week at feedlot; Slaughterhouse = the day steers were killed.
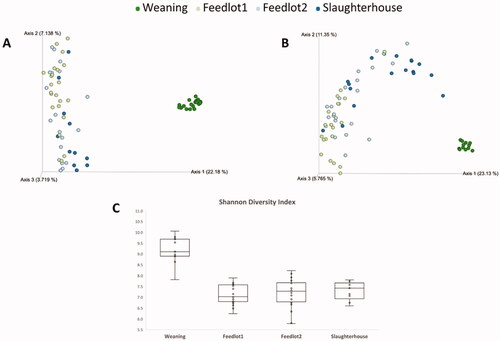
Additional considerations concerning microbiome research
Although typically only a few hundred milligrams of raw material are utilised in the extraction/purification of microbial DNA, investigators normally make use of collections to gather samples for other analysis, such as VFA (Buffa et al. Citation2020; Lourenco, Kieran, et al. Citation2020), resulting in larger quantities being collected. However, even the portion of the collection designated exclusively for microbiome analysis should be greater (10–40 times) than what is required by the DNA extraction procedure, to account for better homogenisation of samples and possible re-runs. As previously mentioned, samples must be frozen soon after collection, ideally using a ‘flash freezing’ method, such as immersion in liquid nitrogen to stop all microbial activity and preserve the original sampling conditions (Lourenco, Kieran, et al. Citation2020). In ruminant animals, samples are normally obtained from fistulated animals or by stomach tubbing, but less-invasive approaches, such as using buccal swabs have been used and show promising results (Kittelmann et al. Citation2015). In other animals, such as swine and chicken, faecal material is typically used as proxy of GIT microbiome since it does not require killing the animals for collections, however, there is some dissimilarity between the faecal and caecal material. Although faecal samples capture a large percentage of the microbial diversity present in caeca, the proportion of species between faeces and caeca may differ. Despite these differences, with enough sequencing depth, faecal samples can be effectively used to detect some shifts of caecal microbiota (Stanley et al. Citation2015).
In order to detect reliable (i.e. genuine and replicable) differences in microbiome studies, it is imperative to use sample sizes large enough to detect an hypothesised difference with sufficient statistical power (Mattiello et al. Citation2016). Typically, this translates into collecting as many samples as possible; however, due to limitations (such as cost), this is not always feasible. Casals-Pascual et al. simulated that the number of individuals needed to find significant differences in alpha-diversity between groups can be as low as 20, but the probability of detecting such difference is less than 30%. However, to find these differences with a statistical power of 80%, 55 individuals per group would be required (Casals-Pascual et al. Citation2020).
An additional method used to identify microbes within the GIT is by using a ‘culturomics’ approach which uses live culturing methods to identify bacterial isolates. This method utilises matrix-assisted laser desorption/ionisation time-of-flight mass spectrometry (MALDI-TOF MS) instead of the use of pyrosequencing or metagenomics (Lagier et al. Citation2012). The advantages of culturomics are that only live bacteria are isolated, can study more than eubacteria and gives insight into how microbes interact (Greub Citation2012). On the other hand, a major limitation is that a large portion of microbes (approximately only 23% of the microorganisms found in the rumen) can be cultured. However, by utilising different growth mediums (undefined and defined media) and dilution factors, culturability of ruminal microorganisms was increased to 40% (Zehavi et al. Citation2018). Nonetheless, culturomics can be a valuable approach to expand our knowledge of microbes that are present at low abundances, which other identification methods can miss (Greub Citation2012).
Of note, other microorganisms like fungi, virus and protozoa play important biological roles and interact with their host animals in many different ways, however, they are not as commonly studied as bacteria in animal science studies. The popularisation of ‘universal’ DNA barcode marker for fungi, such as the ITS region (Schoch et al. Citation2012) may help more animal science studies investigate fungi in a quicker and cost-effective way.
Microbiome effects on the host animal
Recently, there has been a major drive towards understanding the complex synergistic relationship that exists between the GIT microbial population and the host. The GIT microbiota interacts with all aspects of the body through the different gut-organ axes. Some examples of these axes include: the gut-brain axis, the gut-heart axis, the gut-kidney axis, the gut-liver axis, the gut-adipose axis, the gut-bone axis, the gut-skin axis and the gut-lung axis (Ahlawat et al. Citation2020). Any alterations within the gut microbial population can have cascading detrimental effects on the health of the host through these different axes. Thus, it is imperative we understand the mechanisms of the microbiota within the GIT to ensure the health and well-being of animals and humans alike.
When the diet of an animal is altered, the GIT microbiota tends to be altered as a result. Perhaps one of the most dramatic dietary shifts that occur in food-producing animals is the one experienced in beef cattle that are finished in feedlot. These animals transition from a predominantly forage-based diet to a concentrate-based feedlot-finishing ration. During this transition, the GIT microbiota may become unstable and result in dysbiosis and many dietary illnesses, so a proper dietary transition is of utmost importance (USDA/APHIS Citation1995; Nagaraja and Chengappa Citation1998). Even when such transition is properly performed, marked differences in the overall microbiome of the GIT is observed in feedlot cattle. Figure illustrates this fact, as it shows the permanent results concerning alpha and beta-diversity of the microbiome of cattle finished in feedlot.
Similarly to what is shown in Figure , many studies have found that the microbial composition of the GIT is altered by age and diet (Zhou et al. Citation2009; Durso et al. Citation2017; Welch et al. Citation2021). Welch et al. (Citation2021) found that the faecal microbial composition of beef steers at weaning had a distinct microbial profile compared to yearling and after the feedlot-finishing trial. Additionally, they observed that the microbial diversity index and microbial evenness were higher at weaning, which can mainly be attributed to dietary differences. In simple terms, there are two main classes of bacterial species found within the GIT of cattle: cellulolytic and amylolytic bacteria (Mizrahi Citation2013). The amounts of these bacterial species present are determined by the nutrients provided to them by the diet, which gives certain bacteria a competitive advantage. Earlier in life when ruminants are consuming mainly a forage-based diet, cellulolytic bacteria including Ruminococcus albus, R. flavefaciens and Fibrobacter succinogenes tend to have high abundance in the rumen since they are better able to degrade and utilise forages (Flint and Bayer Citation2008; Flint et al. Citation2008; Hobson and Stewart Citation2012). Likewise, the faecal microbial population is made up of mainly the family Ruminococcaceae (Welch et al. Citation2021), which has many genes present that can break down cellulose, hemicellulose and xylan, making this family particularly adapted to degrading plant materials (Ding et al. Citation2001; Rakotoarivonina et al. Citation2005; Brulc et al. Citation2009; Biddle et al. Citation2013). However, once cattle arrive at the feedlot, they are shifted to a predominately concentrate diet and GIT fermentation patterns must rapidly change as well. Instead of degrading mainly cellulose and hemicellulose, fermentation must shift to mainly degrading starch and soluble sugars (McAllister Citation2000; Fernando et al. Citation2010; Carberry et al. Citation2012). After this switch to a high concentrate diet, amylolytic bacteria dominate with Ruminobacter amylophilus, Streptococcus bovis, Succinomonas amylolytica, Butyrivibrio fibrosolvens, Selenomonas ruminantium and many species from the genus Prevotella being some of the most numerous species present (Krause et al. Citation2003).
In order to prevent large shifts in the gastrointestinal microbiota which can lead to dysbiosis, early intervention strategies can be implemented to prime the gastrointestinal populations before exposure to stressors (weaning, diet change, etc.) to pre-emptively avoid GIT distress. Faecal matter transplants (FMTs) are useful tools to introduce healthy microorganisms into a host to either develop or restore healthy microbial populations in the gut. Research has shown that piglets treated with FMT from their mother in early life resulted in improved growth performance, less intestinal permeability and increased beneficial bacteria and energy availability (Cheng et al. Citation2019). In ruminants, inoculation with rumen fluid before and during weaning can modulate both the rumen and colon, preventing dysbiosis (Yu et al. Citation2020). Not only can introducing live population of microbes via FMT or rumen fluid modulate the gastrointestinal microbiota, but introducing feed (creep feeding) to animals prior to weaning can impact their GIT populations as well, making them better prepared for stressful conditions like weaning, commingling and diet changes (Lourenco et al. Citation2019; Lourenco, Maia, et al. Citation2020). To maintain homeostasis in the GIT microbiota while the host is undergoing stressful events, early intervention strategies can be incorporated into the animal’s management whether they are ruminants or monogastrics to prevent GIT dysbiosis and maintain host health.
Microbiome and feed efficiency
Research has revealed a link between animal microbiota and feed efficiency (Stanley et al. Citation2012; Shabat et al. Citation2016; Welch et al. Citation2020). In the foregut of ruminants, and in the hindgut of monogastric animals, the residing microbial population breaks down feedstuffs and converts it into useful products, such as VFA and water-soluble vitamins, which are then utilised by the host animal. Among other things, a greater production (and composition of this pool) of useful compounds, such as VFA and vitamins can have an impact on animals’ feed efficiency.
Yan et al. (Citation2017) recorded the individual performance of 252 laying hens and found that their caecal microbial composition was highly correlated with feed efficiency, with greater presence of Lactobacillus and Akkermansia, and lower abundance or Faecalibacterium in the group of hens with better feed efficiency (Yan et al. Citation2017). In swine, out of a group of 138 animals, Reyer et al. found 19 times more Saccharopolyspora in the ileum, and five times more Akkermansia in the caecum of the pigs with best feed efficiency (Reyer et al. Citation2020). In dairy cows, it has been suggested that specific microbiome-dependent mechanisms are tightly linked to cows’ ability to extract energy from their feed (Shabat et al. Citation2016). More specifically, a ruminal microbiome enriched with some specific microbial taxa, such as Megasphaera elsdenii and Coprococcus catus can result in better energy and carbon channelling to the animal (through production of end products, such as propionate), while lowering methane emissions (Shabat et al. Citation2016). In beef cattle, studies have reported different microbial profiles in the rumen (Myer et al. Citation2015) and hindgut (Welch et al. Citation2020) of animals differing in feed efficiency, but more work needs to be done to establish a clear picture of which taxa (or group of taxa) consistently impacts and modulates feed efficiency. However, research performed by Li et al. (Citation2019) using metagenomic and metatranscriptomic approaches concluded that the differences in the ruminal microbiomes of cattle with different feed efficiency tended to be specific to each breed, indicating that not only the microbiome, but microbiome-host breed interactions are in fact the main contributors to the variation observed in cattle feed efficiency (Li et al. Citation2019).
Effects of the microbiome on animal health and immunity
It has been established that the GIT microbiota is crucial for the development of the mucosal immune system in monogastrics (Mulder et al. Citation2011) and ruminants (Malmuthuge and Guan Citation2017; O’Hara et al. Citation2020). The epithelial cells in the GIT are joined by an intercellular junction called tight junctions which are comprised proteins (e.g. occludin, claudins, and zonula occludens) and junctional adhesion molecules. The tight junctions allow the passage of nutrients, ions and water through to the bloodstream while preventing the passage of microbes and their peptides (Sawada et al. Citation2003; Rajasekaran et al. Citation2008). In bovines, right after birth, the tight junction proteins are not fully formed and passage through into the bloodstream is high. This increases the calf’s ability to absorb nutrients, immunoglobulins and leukocytes from the colostrum (Bush and Staley Citation1980; Besser and Gay Citation1994). However, this permeability begins to decrease around 24–36 h after birth, and continues to decrease during the calf’s first month (Araujo et al. Citation2015). Research has shown that the presence of some specific bacteria, such as Lactobacillus spp. and Bifidobacterium spp. and their metabolites, can promote the activation of these tight junction proteins (Ewaschuk et al. Citation2008; Miyauchi et al. Citation2012; Sultana et al. Citation2013), which can be particularly important during the first few weeks of life, when the intestinal permeability is progressively decreasing.
Proinflammatory (e.g. interleukin [IL]-8) and anti-inflammatory (e.g. IL-10) cytokines are upregulated during the first week of a calf’s life (Liang et al. Citation2016). These are important because IL-8 works to activate and attract neutrophils into the interstitial space in the body (Stillie et al. Citation2009), and IL-10 prohibits proinflammatory molecules (e.g. INF-g, TNF-α and IL-6) from activating and prevents the immune system’s ability to recognise antigens (Iyer and Cheng Citation2012). Some bacterial species aid the immune system in these functions: both Lactobacillus and Bifidobacterium stimulate activation of IL-10 which prevents the immune system from activating a greater proinflammatory response (Smits et al. Citation2005). These two beneficial bacterial genera play an important role in the early stages of the establishment of the GIT microbiota, which can help modulate the animal’s immune response and prevent any unnecessary inflammatory responses to ‘self’ antigens.
Besides aiding in the establishment of animal’s immune system early in life, the GIT microbial population continues to play a vital role in maintaining and regulating the immune system during the adult stage. As animals are weaned, both their GIT microbiota and the expression of genes related to intestinal immunity are impacted (Marchiando et al. Citation2010). The expression of immune genes has been linked to the abundance of luminal bacteria correlated with health in dairy calves (Okumura and Takeda Citation2018). IgA, a secretory immunoglobulin that plays a large role in intestinal immunity, aids the immune system in regulating the relationship between both beneficial and pathogenic bacteria (Nakajima et al. Citation2018). If this immunoglobulin is removed from the GIT, a dysbiosis may occur and the immune system may upregulate the expression of proinflammatory cytokines (Cunningham-Rundles Citation2001), however, recovery of IgA restores intestinal homeostasis, characterised by a return of commensal bacterial species and reduction of inflammation (Shroff et al. Citation1995).
Presence of mucus is another key feature of the gastrointestinal system that helps maintaining health and proper functioning by providing a barrier to the tight junctions and maintaining gut integrity (Bergstrom et al. Citation2010; Okumura and Takeda Citation2018). The GIT microbial population plays a vital role in the production of intestinal mucus. When comparing germ-free and conventionally raised mice, the mice without a functional microbiota had less mucus lining their intestinal epithelial cells (Szentkuti et al. Citation1990; Enss et al. Citation1992), but when germ-free mice were exposed to bacterial molecules (lipopolysaccharides and peptidoglycans), their intestinal mucus production restabilised (Enss et al. Citation1992, Citation1996). These results highlight the importance of the GIT microbiota and its metabolites in promoting and maintaining intestinal mucus production.
Limitations and future perspectives of microbiome studies
Studies involving the evaluation complex microbial communities (such as animal microbiomes) using high-throughput sequencing have allowed a better understanding of biological systems; however, such studies normally have an inherent limitation since they only provide a ‘snapshot’ of the microbial population present in a given sample at the time the sample was collected, and this fact in itself constitutes a limitation. For this reason, replication/reproduction of microbiome studies, as well as the collection of as many samples as feasible within each study is recommended since it would allow a collection of multiple ‘snapshots’, and consequently help the determination of certain patterns (in case any is present) in the type of sample being studied. On the other hand, caution should be taken when comparing data from different microbiome studies (Henderson et al. Citation2013; Pollock et al. Citation2018), especially if the used methodologies differ significantly, as previously discussed (i.e. if there is no consistency regarding the method of DNA extraction, library preparation, sequencing platform and bioinformatics analysis).
In order to overcome some of those inherent limitations, ideally, microbiome evaluations should be performed in conjunction with other approaches, such as metatranscriptomics, proteomics and metabolomics investigations, as well as host phenotypic evaluations. The literature has many examples showing that animals with different phenotypes have different microbial profiles (Xue et al. Citation2018; Clemmons et al. Citation2019; Wallace et al. Citation2019); however, there is still a lack of knowledge on the exact biological mechanisms driving those differences, as well as the direction of the causality. Moreover, despite the considerable advances made in recent years, there is still insufficient information on the diversity of molecules produced by microorganisms (Han et al. Citation2021). As illustrated in Figure , studies that utilise only microbial DNA as input address only the first layer of the biological complexity observed in different animal phenotypes. Therefore, multi-omics studies appear to be the natural way to integrate microbiome studies, and most likely will become more popular in animal science research.
Figure 4. The several layers of biological complexity that can be explored using ‘omics’ approaches.
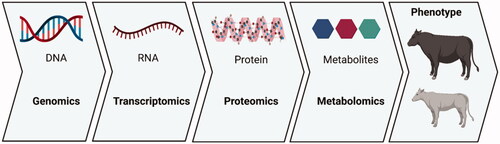
One useful way in which microbiome information can be utilised (and likely will become more popular in animal production systems) is in the development of probiotics and prebiotics. The term probiotic can be broadly defined as ‘live microorganisms that when administered in adequate amount confer a health benefit on the host’ (FAO/WHO Citation2006). In animal science, probiotics are sometimes referred to as direct fed microbials (DFMs). On the other hand, prebiotics are non-digestible food ingredients that beneficially affect the host by selectively stimulating the growth and/or activity of one or a limited number of bacterial species already resident in the GIT (Gibson and Roberfroid Citation1995). Thus, by either introducing beneficial bacteria into the GIT, or by selecting for beneficial bacteria by providing nutrients that stimulate their growth, pro and prebiotics can provide many benefits to the host animal, including: stabilising the gut microbial population (through competitive exclusion) to prevent development of pathogens and reduce digestive disorders (Wisener et al. Citation2015); improving animal performance, feed conversion and fibre digestion (Zhang et al. Citation2016); reducing ruminal methane production (Alazzeh et al. Citation2012); increasing milk yield (Ayad et al. Citation2013); and reducing the incidence of mastitis (Espeche et al. Citation2012).
Conclusions
Animals host a myriad of different microorganisms, especially in their GIT. Those microorganisms encode numerous metabolic pathways and can produce many compounds that can affect diet digestibility, utilisation, gut health and integrity and many other aspects of the host animal. As a consequence, the composition of an animal microbiota can have an impact on the animal’s energetic requirements (and other nutrients required) for maintenance and production, with eventual economic implications. Precise selection and manipulation of microbiomes for improved animal performance is an ambitious goal, but the tools that are currently available (and at increasingly affordable costs) are definitely helping in achieving that objective. However, microbiome studies must be carried out in a meticulous way to avoid the introduction of biases, which may occur at all methodological stages, from sampling to bioinformatic analysis. In addition, microbiome investigations should be coupled with other ‘omics’ approaches (transcriptomics, metabolomics, etc.) for a more accurate understanding of the complex biology responsible for the differences observed in animal phenotypes. Current and future research will likely solidify the attributes of ‘good’ or ‘desirable’ microbiomes; and hopefully in a not-so-distant future, we will be able manipulate animal microbiomes in a very precise way, so, by combining the knowledge acquired in other areas like animal genetics and nutrition, we will be able to have a greater control over animal health and productivity.
Ethical approval
This article is a review and not an experimental study; therefore, it did not perform any studies with human or animal subjects.
Acknowledgements
The authors thank Mr. Osman Yasir Koyun for drawing most of the figures presented in this article.
Disclosure statement
No potential conflict of interest was reported by the author(s).
Data availability statement
The data used to support the findings of this study are available from the corresponding author upon request.
References
- Abbas HF, AL Musawi IH. 2016. Evaluation three methods of the extraction and purification of bacterial DNA of Gram positive and Gram negative bacteria. World J Exp Biosci. 4:899–65.
- Afgan E, Baker D, Batut B, van den Beek M, Bouvier D, Čech M, Chilton J, Clements D, Coraor N, Grüning BA, et al. 2018. The Galaxy platform for accessible, reproducible and collaborative biomedical analyses: 2018 update. Nucleic Acids Res. 46(W1):W537–W544.
- Ahlawat S, Asha , Sharma KK. 2020. Gut–organ axis: a microbial outreach and networking. Lett Appl Microbiol. 72(6):636–668.
- Alazzeh AY, Sultana H, Beauchemin KA, Wang Y, Holo H, Harstad OM, McAllister TA. 2012. Using strains of propionibacteria to mitigate methane emissions in vitro. Acta Agric Scand A Anim Sci. 62(4):263–272.
- Allaband C, McDonald D, Vázquez-Baeza Y, Minich JJ, Tripathi A, Brenner DA, Loomba R, Smarr L, Sandborn WJ, Schnabl B, et al. 2019. Microbiome 101: studying, analyzing, and interpreting gut microbiome data for clinicians. Clin Gastroenterol Hepatol. 17(2):218–230.
- Allali I, Arnold JW, Roach J, Cadenas MB, Butz N, Hassan HM, Koci M, Ballou A, Mendoza M, Ali R, et al. 2017. A comparison of sequencing platforms and bioinformatics pipelines for compositional analysis of the gut microbiome. BMC Microbiol. 17(1):19.
- Anyansi C, Straub TJ, Manson AL, Earl AM, Abeel T. 2020. Computational methods for strain-level microbial detection in colony and metagenome sequencing data. Front Microbiol. 11:1925.
- Araujo G, Yunta C, Terré M, Mereu A, Ipharraguerre I, Bach A. 2015. Intestinal permeability and incidence of diarrhea in newborn calves. J Dairy Sci. 98(10):7309–7317.
- Ayad M, Benallou B, Saim M, Smadi M, Meziane T. 2013. Impact of feeding yeast culture on milk yield, milk components, and blood components in Algerian dairy herds. J Vet Sci Technol. 4(2):1–5.
- Bach A. 2012. Ruminant nutrition symposium: optimizing performance of the offspring: nourishing and managing the dam and postnatal calf for optimal lactation, reproduction, and immunity. J Anim Sci. 90(6):1835–1845.
- Banos S, Lentendu G, Kopf A, Wubet T, Glöckner FO, Reich M. 2018. A comprehensive fungi-specific 18S rRNA gene sequence primer toolkit suited for diverse research issues and sequencing platforms. BMC Microbiol. 18(1):190.
- Bashiardes S, Zilberman-Schapira G, Elinav E. 2016. Use of metatranscriptomics in microbiome research. Bioinform Biol Insights. 10:BBI.S34610.
- Bergstrom KSB, Kissoon-Singh V, Gibson DL, Ma C, Montero M, Sham HP, Ryz N, Huang T, Velcich A, Finlay BB, et al. 2010. Muc2 protects against lethal infectious colitis by disassociating pathogenic and commensal bacteria from the colonic mucosa. PLoS Pathog. 6(5):e1000902.
- Berry D, Crowley J. 2013. Cell biology symposium: genetics of feed efficiency in dairy and beef cattle. J Anim Sci. 91(4):1594–1613.
- Besser TE, Gay CC. 1994. The importance of colostrum to the health of the neonatal calf. Vet Clin North Am Food Anim Practi. 10(1):107–117.
- Bharti R, Grimm DG. 2021. Current challenges and best-practice protocols for microbiome analysis. Brief Bioinform. 22(1):178–193.
- Biddle A, Stewart L, Blanchard J, Leschine S. 2013. Untangling the genetic basis of fibrolytic specialization by lachnospiraceae and ruminococcaceae in diverse gut communities. Diversity. 5(3):627–640.
- Bokulich NA, Subramanian S, Faith JJ, Gevers D, Gordon JI, Knight R, Mills DA, Caporaso JG. 2013. Quality-filtering vastly improves diversity estimates from Illumina amplicon sequencing. Nat Methods. 10(1):57–59.
- Bolyen E, Rideout JR, Dillon MR, Bokulich NA, Abnet CC, Al-Ghalith GA, Alexander H, Alm EJ, Arumugam M, Asnicar F, et al. 2019. Reproducible, interactive, scalable and extensible microbiome data science using QIIME 2. Nat Biotechnol. 37(8):852–857.
- Brulc JM, Antonopoulos DA, Berg Miller ME, Wilson MK, Yannarell AC, Dinsdale EA, Edwards RE, Frank ED, Emerson JB, Wacklin P, et al. 2009. Gene-centric metagenomics of the fiber-adherent bovine rumen microbiome reveals forage specific glycoside hydrolases. Proc Natl Acad Sci USA. 106(6):1948–1953.
- Buffa G, Mangia NP, Cesarani A, Licastro D, Sorbolini S, Pulina G, Nudda A. 2020. Agroindustrial by-products from tomato, grape and myrtle given at low dosage to lactating dairy ewes: effects on rumen parameters and microbiota. Ital J Anim Sci. 19(1):1462–1471.
- Bush LJ, Staley TE. 1980. Absorption of colostral immunoglobulins in newborn calves1. J Dairy Sci. 63(4):672–680.
- Callahan BJ, McMurdie PJ, Holmes SP. 2017. Exact sequence variants should replace operational taxonomic units in marker-gene data analysis. Isme J. 11(12):2639–2643.
- Callahan BJ, McMurdie PJ, Rosen MJ, Han AW, Johnson AJA, Holmes SP. 2016. DADA2: high-resolution sample inference from Illumina amplicon data. Nat Methods. 13(7):581–583.
- Caporaso JG, Kuczynski J, Stombaugh J, Bittinger K, Bushman FD, Costello EK, Fierer N, Peña AG, Goodrich JK, Gordon JI, et al. 2010. QIIME allows analysis of high-throughput community sequencing data. Nat Methods. 7(5):335–336.
- Carberry CA, Kenny DA, Han S, McCabe MS, Waters SM. 2012. Effect of phenotypic residual feed intake and dietary forage content on the rumen microbial community of beef cattle. Appl Environ Microbiol. 78(14):4949–4958.
- Casals-Pascual C, González A, Vázquez-Baeza Y, Song SJ, Jiang L, Knight R. 2020. Microbial diversity in clinical microbiome studies: sample size and statistical power considerations. Gastroenterology. 158(6):1524–1528.
- Chao A. 1984. Nonparametric estimation of the number of classes in a population. Scand J Stat. 11(4):265–270.
- Chappidi S, Villa EC, Cantarel BL. 2019. Using mothur to determine bacterial community composition and structure in 16S ribosomal RNA datasets. Curr Protoc Bioinformatics. 67(1):e83.
- Cheng CS, Wei HK, Wang P, Yu HC, Zhang XM, Jiang SW, Peng J. 2019. Early intervention with faecal microbiota transplantation: an effective means to improve growth performance and the intestinal development of suckling piglets. Animal. 13(3):533–541.
- Clemmons BA, Martino C, Powers JB, Campagna SR, Voy BH, Donohoe DR, Gaffney J, Embree MM, Myer PR. 2019. Rumen bacteria and serum metabolites predictive of feed efficiency phenotypes in beef cattle. Sci Rep. 9(1):19265.
- Cunningham-Rundles C. 2001. Physiology of IgA and IgA deficiency. J Clin Immunol. 21(5):303–309.
- Ding X, Lan W, Liu G, Ni H, Gu JD. 2019. Exploring possible associations of the intestine bacterial microbiome with the pre-weaned weight gaining performance of piglets in intensive pig production. Sci Rep. 9(1):15534. https://doi.org/10.1038/s41598-019-52045-4.
- Ding SY, Rincon MT, Lamed R, Martin JC, McCrae SI, Aurilia V, Shoham Y, Bayer EA, Flint HJ. 2001. Cellulosomal scaffoldin-like proteins from Ruminococcus flavefaciens. J Bacteriol. 183(6):1945–1953.
- Durso LM, Miller DN, Schmidt TB, Callaway T. 2017. Tracking bacteria through the entire gastrointestinal tract of a beef steer. https://dx.doi.org/10.2134/ael2017.05.0016.
- Edgar RC, Haas BJ, Clemente JC, Quince C, Knight R. 2011. UCHIME improves sensitivity and speed of chimera detection. Bioinformatics. 27(16):2194–2200.
- Enss ML, Grosse-Siestrup H, Schmidt-Wittig U, Gärtner K. 1992. Changes in colonic mucins of germfree rats in response to the introduction of a "normal" rat microbial flora. Rat colonic mucin. J Exp Anim Sci. 35(3):110–119.
- Enss ML, Schmidt-Wittig U, Müller H, Mai UE, Coenen M, Hedrich HJ. 1996. Response of germfree rat colonic mucous cells to peroral endotoxin application. Eur J Cell Biol. 71(1):99–104.
- Espeche MC, Pellegrino M, Frola I, Larriestra A, Bogni C, Nader-Macías MEF. 2012. Lactic acid bacteria from raw milk as potentially beneficial strains to prevent bovine mastitis. Anaerobe. 18(1):103–109.
- Ewaschuk JB, Diaz H, Meddings L, Diederichs B, Dmytrash A, Backer J, Langen ML-v, Madsen KL. 2008. Secreted bioactive factors from Bifidobacterium infantis enhance epithelial cell barrier function. Am J Physiol Gastrointest Liver Physiol. 295(5):G1025–G1034.
- FAO/WHO. 2006. Probiotics in food. Health and nutritional properties and guidelines for evaluation. Rome, Italy: FAO/WHO.
- Fernando SC, Purvis HT, Najar FZ, Sukharnikov LO, Krehbiel CR, Nagaraja TG, Roe BA, De SU. 2010. Rumen microbial population dynamics during adaptation to a high-grain diet [Article. Appl Environ Microbiol. 76(22):7482–7490.
- Fidler G, Tolnai E, Stagel A, Remenyik J, Stundl L, Gal F, Biro S, Paholcsek M. 2020. Tendentious effects of automated and manual metagenomic DNA purification protocols on broiler gut microbiome taxonomic profiling. Sci Rep. 10(1):3419.
- Finneran E, Crosson P, O'kiely P, Shalloo L, Forristal D, Wallace M. 2012. Stochastic simulation of the cost of home-produced feeds for ruminant livestock systems. J Agric Sci. 150(1):123–139.
- Flint HJ, Bayer EA. 2008. Plant cell wall breakdown by anaerobic microorganisms from the mammalian digestive tract. Ann NY Acad Sci. 1125(1):280–288.
- Flint HJ, Bayer EA, Rincon MT, Lamed R, White BA. 2008. Polysaccharide utilization by gut bacteria: Potential for new insights from genomic analysis. Nat Rev Microbiol. 6(2):121–131.
- Gibson GR, Roberfroid MB. 1995. Dietary modulation of the human colonic microbiota: introducing the concept of prebiotics. J Nutr. 125(6):1401–1412.
- Goodwin S, McPherson JD, McCombie WR. 2016. Coming of age: ten years of next-generation sequencing technologies. Nat Rev Genet. 17(6):333–351.
- Greub G. 2012. Culturomics: a new approach to study the human microbiome. Clin Microbiol Infect. 18(12):1157–1159.
- Han S, Van Treuren W, Fischer CR, Merrill BD, DeFelice BC, Sanchez JM, Higginbottom SK, Guthrie L, Fall LA, Dodd D, et al. 2021. A metabolomics pipeline for the mechanistic interrogation of the gut microbiome. Nature. 595(7867):415–420.
- Heikema AP, Horst-Kreft D, Boers SA, Jansen R, Hiltemann SD, De Koning W, Kraaij R, De Ridder MAJ, Van Houten CB, Bont LJ, et al. 2020. Comparison of illumina versus nanopore 16S rRNA gene sequencing of the human nasal microbiota. Genes. 11(9):1105.
- Henderson G, Cox F, Kittelmann S, Miri VH, Zethof M, Noel SJ, Waghorn GC, Janssen PH. 2013. Effect of DNA extraction methods and sampling techniques on the apparent structure of cow and sheep rumen microbial communities. PLoS One. 8(9):e74787.
- Herd R, Arthur P. 2009. Physiological basis for residual feed intake. J Anim Sci. 87(14):E64–E71.
- Hobson PN, Stewart CS. 2012. The rumen microbial ecosystem. Berlin, Germany: Springer Science & Business Media.
- Illumina 2013. 16S metagenomic sequencing library preparation. Part # 15044223 Rev. B. [accessed]. https://support.illumina.com/documents/documentation/chemistry_documentation/16s/16s-metagenomic-library-prep-guide-15044223-b.pdf
- Iyer SS, Cheng G. 2012. Role of interleukin 10 transcriptional regulation in inflammation and autoimmune disease. Crit Rev Immunol. 32(1):23–63.
- Johnson JS, Spakowicz DJ, Hong BY, Petersen LM, Demkowicz P, Chen L, Leopold SR, Hanson BM, Agresta HO, Gerstein M, et al. 2019. Evaluation of 16S rRNA gene sequencing for species and strain-level microbiome analysis. Nat Commun. 10, 5029. https://doi.org/10.1038/s41467-019-13036-1.
- Kim H, Kim S, Jung S. 2020. Instruction of microbiome taxonomic profiling based on 16S rRNA sequencing. J Microbiol. 58(3):193–205.
- Kittelmann S, Kirk MR, Jonker A, McCulloch A, Janssen PH, Schloss PD. 2015. Buccal swabbing as a noninvasive method to determine bacterial, archaeal, and eukaryotic microbial community structures in the rumen. Appl Environ Microbiol. 81(21):7470–7483.
- Klindworth A, Pruesse E, Schweer T, Peplies J, Quast C, Horn M, Glöckner FO. 2013. Evaluation of general 16S ribosomal RNA gene PCR primers for classical and next-generation sequencing-based diversity studies. Nucleic Acids Res. 41(1):e1.
- Koetsier G, Cantor E. 2019. A practical guide to analyzing nucleic acid concentration and purity with microvolume spectrophotometers. Ipswich (MA): New England Biolabs Inc.
- Kogut MH. 2019. The effect of microbiome modulation on the intestinal health of poultry. Anim Feed Sci Technol. 250:32–40.
- Krause DO, Denman SE, Mackie RI, Morrison M, Rae AL, Attwood GT, McSweeney CS. 2003. Opportunities to improve fiber degradation in the rumen: microbiology, ecology, and genomics. FEMS Microbiol Rev. 27(5):663–693.
- Lagier JC, Armougom F, Million M, Hugon P, Pagnier I, Robert C, Bittar F, Fournous G, Gimenez G, Maraninchi M, et al. 2012. Microbial culturomics: paradigm shift in the human gut microbiome study. Clin Microbiol Infect. 18(12):1185–1193.
- Legendre P, Borcard D, Peres-Neto PR. 2005. Analyzing beta diversity: partitioning the spatial variation of community composition data. Ecol Monogr. 75(4):435–450.
- Li F, Hitch TCA, Chen Y, Creevey CJ, Guan LL. 2019. Comparative metagenomic and metatranscriptomic analyses reveal the breed effect on the rumen microbiome and its associations with feed efficiency in beef cattle. Microbiome. 7(1):6.
- Liang G, Malmuthuge N, Bao H, Stothard P, Griebel PJ, Guan LL. 2016. Transcriptome analysis reveals regional and temporal differences in mucosal immune system development in the small intestine of neonatal calves. BMC Genomics. 17(1):602.
- Lourenco JM, Callaway TR, Kieran TJ, Glenn TC, McCann JC, Stewart RL. 2019. Analysis of the rumen microbiota of beef calves supplemented during the suckling phase. Front Microbiol. 10:1131.
- Lourenco JM, Hampton RS, Johnson HM, Callaway TR, Rothrock MJ, Azain MJ. 2021. The effects of feeding antibiotic on the intestinal microbiota of weanling pigs. Front Vet Sci. 8:601394.
- Lourenco JM, Kieran TJ, Seidel DS, Glenn TC, Silveira M, Callaway TR, Stewart RL. Jr. 2020. Comparison of the ruminal and fecal microbiotas in beef calves supplemented or not with concentrate. PLoS One. 15(4):e0231533.
- Lourenco JM, Maia FJ, Bittar JHJ, Segers JR, Tucker JJ, Campbell BT, Stewart RL. 2020. Utilization of exogenous enzymes in beef cattle creep feeds. J Appl Anim Res. 48(1):70–77.
- Lozupone C, Knight R. 2005. UniFrac: a new phylogenetic method for comparing microbial communities. Appl Environ Microbiol. 71(12):8228–8235.
- Lucena-Aguilar G, Sánchez-López AM, Barberán-Aceituno C, Carrillo-Ávila JA, López-Guerrero JA, Aguilar-Quesada R. 2016. DNA source selection for downstream applications based on DNA quality indicators analysis. Biopreserv Biobank. 14(4):264–270.
- Magurran AE. 2003. Measuring biological diversity. Hoboken (NJ): John Wiley & Sons.
- Mahalanabis M, Al-Muayad H, Kulinski MD, Altman D, Klapperich CM. 2009. Cell lysis and DNA extraction of gram-positive and gram-negative bacteria from whole blood in a disposable microfluidic chip. Lab Chip. 9(19):2811–2817.
- Malmuthuge N, Guan LL. 2017. Understanding host-microbial interactions in rumen: searching the best opportunity for microbiota manipulation. J Anim Sci Biotechnol. 8(1):8.
- Marchiando AM, Graham WV, Turner JR. 2010. Epithelial barriers in homeostasis and disease. Annu Rev Pathol. 5(1):119–144.
- Martin KJ, Rygiewicz PT. 2005. Fungal-specific PCR primers developed for analysis of the ITS region of environmental DNA extracts. BMC Microbiol. 5(1):28.
- Mattiello F, Verbist B, Faust K, Raes J, Shannon WD, Bijnens L, Thas O. 2016. A web application for sample size and power calculation in case-control microbiome studies. Bioinformatics. 32(13):2038–2040.
- McAllister T. 2000. Learning more about rumen bugs: genetic and environmental factors affecting rumen bugs. Southern Alberta Beef Rev. 2(1).
- McCann JC, Wickersham TA, Loor JJ. 2014. High-throughput methods redefine the rumen microbiome and its relationship with nutrition and metabolism. Bioinform Biol Insights. 8:109–125. eng.
- McMurdie PJ, Holmes S. 2013. Phyloseq: an R package for reproducible interactive analysis and graphics of microbiome census data. PLoS One. 8(4):e61217.
- Meyer F, Paarmann D, D’Souza M, Olson R, Glass EM, Kubal M, Paczian T, Rodriguez A, Stevens R, Wilke A, et al. 2008. The metagenomics RAST server - a public resource for the automatic phylogenetic and functional analysis of metagenomes. BMC Bioinformatics. 9(1):386.
- Miyauchi E, O’Callaghan J, Buttó LF, Hurley G, Melgar S, Tanabe S, Shanahan F, Nally K, O'Toole PW. 2012. Mechanism of protection of transepithelial barrier function by Lactobacillus salivarius: strain dependence and attenuation by bacteriocin production. Am J Physiol Gastrointest Liver Physiol. 303(9):G1029–G1041.
- Mizrahi I. 2013. 21 Rumen symbioses. In Rosenberg E, editor. The prokaryotes. 4 ed. Berlin, Germany: Springer.
- Mintert J. 2021. The importance of managing hog operation feed costs. West Lafayette (IN): Purdue University Center for Commercial Agriculture; p. 2.
- Mulder IE, Schmidt B, Lewis M, Delday M, Stokes CR, Bailey M, Aminov RI, Gill BP, Pluske JR, Mayer CD, et al. 2011. Restricting microbial exposure in early life negates the immune benefits associated with gut colonization in environments of high microbial diversity. PLoS One. 6(12):e28279.
- Myer PR, Smith TP, Wells JE, Kuehn LA, Freetly HC. 2015. Rumen microbiome from steers differing in feed efficiency. PLoS One. 10(6):e0129174.
- Nagaraja TG, Chengappa MM. 1998. Liver abscesses in feedlot cattle: a review. J Anim Sci. 76(1):287–298.
- Nakajima A, Vogelzang A, Maruya M, Miyajima M, Murata M, Son A, Kuwahara T, Tsuruyama T, Yamada S, Matsuura M, et al. 2018. IgA regulates the composition and metabolic function of gut microbiota by promoting symbiosis between bacteria. J Exp Med. 215(8):2019–2034.
- Nygaard AB, Tunsjø HS, Meisal R, Charnock C. 2020. A preliminary study on the potential of Nanopore MinION and Illumina MiSeq 16S rRNA gene sequencing to characterize building-dust microbiomes. Sci Rep. 10, 3209 (2020). https://doi.org/10.1038/s41598-020-59771-0.
- O’Hara E, Neves ALA, Song Y, Guan LL. 2020. The role of the gut microbiome in cattle production and health: driver or passenger? Annu Rev Anim Biosci. 8(1):199–220.
- Okumura R, Takeda K. 2018. Maintenance of intestinal homeostasis by mucosal barriers. Inflamm Regen. 38(1):5.
- Pham VT, Dold S, Rehman A, Bird JK, Steinert RE. 2021. Vitamins, the gut microbiome and gastrointestinal health in humans. Nutr Res. 95:35–53.
- Pollock J, Glendinning L, Wisedchanwet T, Watson M, Liu S-J. 2018. The madness of microbiome: attempting to find consensus “ best practice” for 16S microbiome studies. Appl Environ Microbiol. 84(7):e02627–02617.
- Rajasekaran SA, Beyenbach KW, Rajasekaran AK. 2008. Interactions of tight junctions with membrane channels and transporters. Biochim Biophys Acta (BBA) - Biomembran. 1778(3):757–769.
- Rakotoarivonina H, Larson MA, Morrison M, Girardeau JP, Gaillard-Martinie B, Forano E, Mosoni P. 2005. The Ruminococcus albus pilA1-pilA2 locus: expression and putative role of two adjacent pil genes in pilus formation and bacterial adhesion to cellulose. Microbiology (Reading). 151(Pt 4):1291–1299.
- Reyer H, Oster M, McCormack UM, Muráni E, Gardiner GE, Ponsuksili S, Lawlor PG, Wimmers K. 2020. Host-microbiota interactions in ileum and caecum of pigs divergent in feed efficiency contribute to nutrient utilization. Microorganisms. 8(4):563.
- Rothrock MJ, Jr, Hiett KL, Gamble J, Caudill AC, Cicconi-Hogan KM, Caporaso JG. 2014. A hybrid DNA extraction method for the qualitative and quantitative assessment of bacterial communities from poultry production samples. JoVE. (94):e52161.
- Sawada N, Murata M, Kikuchi K, Osanai M, Tobioka H, Kojima T, Chiba H. 2003. Tight junctions and human diseases. Med Electron Microsc. 36(3):147–156.
- Schloss PD, Westcott SL, Ryabin T, Hall JR, Hartmann M, Hollister EB, Lesniewski RA, Oakley BB, Parks DH, Robinson CJ, et al. 2009. Introducing mothur: open-source, platform-independent, community-supported software for describing and comparing microbial communities. Appl Environ Microbiol. 75(23):7537–7541.
- Schoch CL, Seifert KA, Huhndorf S, Robert V, Spouge JL, Levesque CA, Chen W, Bolchacova E, Voigt K, Crous PW, et al. 2012. Nuclear ribosomal internal transcribed spacer (ITS) region as a universal DNA barcode marker for Fungi. Proc Natl Acad Sci USA 109(16):6241–6246.
- Shabat SKB, Sasson G, Doron-Faigenboim A, Durman T, Yaacoby S, Berg Miller ME, White BA, Shterzer N, Mizrahi I. 2016. Specific microbiome-dependent mechanisms underlie the energy harvest efficiency of ruminants. ISME J. 10(12):2958–2972.
- Shang Y, Kumar S, Oakley B, Kim WK. 2018. Chicken gut microbiota: importance and detection technology. Front Vet Sci. 5:254.
- Shroff KE, Meslin K, Cebra JJ. 1995. Commensal enteric bacteria engender a self-limiting humoral mucosal immune response while permanently colonizing the gut. Infect Immun. 63(10):3904–3913.
- Smits HH, Engering A, van der Kleij D, de Jong EC, Schipper K, van Capel TMM, Zaat BAJ, Yazdanbakhsh M, Wierenga EA, van Kooyk Y, et al. 2005. Selective probiotic bacteria induce IL-10-producing regulatory T cells in vitro by modulating dendritic cell function through dendritic cell-specific intercellular adhesion molecule 3-grabbing nonintegrin. J Allergy Clin Immunol. 115(6):1260–1267.
- Stanley D, Denman SE, Hughes RJ, Geier MS, Crowley TM, Chen H, Haring VR, Moore RJ. 2012. Intestinal microbiota associated with differential feed conversion efficiency in chickens. Appl Microbiol Biotechnol. 96(5):1361–1369.
- Stanley D, Geier MS, Chen H, Hughes RJ, Moore RJ. 2015. Comparison of fecal and cecal microbiotas reveals qualitative similarities but quantitative differences. BMC Microbiol. 15(1):51.
- Stillie R, Farooq SM, Gordon JR, Stadnyk AW. 2009. The functional significance behind expressing two IL-8 receptor types on PMN. J Leukoc Biol. 86(3):529–543.
- Sultana R, McBain AJ, O’Neill CA. 2013. Strain-dependent augmentation of tight-junction barrier function in human primary epidermal keratinocytes by Lactobacillus and bifidobacterium lysates. Appl Environ Microbiol. 79(16):4887–4894.
- Sylvester JT, Karnati SKR, Yu Z, Morrison M, Firkins JL. 2004. Development of an assay to quantify rumen ciliate protozoal biomass in cows using real-time PCR. J Nutr. 134(12):3378–3384.
- Szentkuti L, Riedesel H, Enss ML, Gaertner K, von Engelhardt W. 1990. Pre-epithelial mucus layer in the colon of conventional and germ-free rats. Histochem J. 22(9):491–497.
- Thijs S, Op De Beeck M, Beckers B, Truyens S, Stevens V, Van Hamme JD, Weyens N, Vangronsveld J. 2017. Comparative evaluation of four bacteria-specific primer pairs for 16S rRNA gene surveys. Front Microbiol. 8:494.
- Turnbaugh PJ, Ley RE, Hamady M, Fraser-Liggett CM, Knight R, Gordon JI. 2007. The human microbiome project. Nature. 449(7164):804–810.
- USDA/APHIS. 1995. Feedlot health management report: part II: cattle on feed evaluation. Fort Collins (CO): USDA/APHIS/Veterinary Services, Centers for Epidemiology and Animal Health; p. 0195.
- Wallace RJ, Rooke JA, Duthie C-A, Hyslop JJ, Ross DW, McKain N, de Souza SM, Snelling TJ, Waterhouse A, Roehe R. 2015. Archaeal abundance in post-mortem ruminal digesta may help predict methane emissions from beef cattle. Sci Rep. 4(1):5892.
- Wallace RJ, Sasson G, Garnsworthy PC, Tapio I, Gregson E, Bani P, Huhtanen P, Bayat AR, Strozzi F, Biscarini F, et al. 2019. A heritable subset of the core rumen microbiome dictates dairy cow productivity and emissions. Sci Adv. 5(7):eaav8391.
- Welch CB, Lourenco JM, Davis DB, Krause TR, Carmichael MN, Rothrock MJ, Pringle TD, Callaway TR. 2020. The impact of feed efficiency selection on the ruminal, cecal, and fecal microbiomes of Angus steers from a commercial feedlot. J Anim Sci. 98(7):skaa230, https://doi.org/10.1093/jas/skaa230.
- Welch CB, Lourenco JM, Krause TR, Seidel DS, Fluharty FL, Pringle TD, Callaway TR. 2021. Evaluation of the fecal bacterial communities of Angus steers with divergent feed efficiencies across the lifespan from weaning to slaughter. Front Vet Sci. 8(694):597405.
- Willis AD. 2019. Rarefaction, alpha diversity, and statistics perspective. Front Microbiol. 10: 2407.
- Wisener LV, Sargeant JM, O’Connor AM, Faires MC, Glass-Kaastra SK. 2015. The use of direct-fed microbials to reduce shedding of Escherichia coli O157 in beef cattle: a systematic review and meta-analysis. Zoonoses Public Health. 62(2):75–89.
- Xue M, Sun H, Wu X, Guan LL, Liu J, Nojiri H. 2018. Assessment of rumen microbiota from a large dairy cattle cohort reveals the pan and core bacteriomes contributing to varied phenotypes. Appl Environ Microbiol. 84(19):e00970–00918.
- Xue MY, Sun HZ, Wu XH, Liu JX, Guan LL. 2020. Multi-omics reveals that the rumen microbiome and its metabolome together with the host metabolome contribute to individualized dairy cow performance. Microbiome. 8(1):1–19.
- Yan W, Sun C, Yuan J, Yang N. 2017. Gut metagenomic analysis reveals prominent roles of Lactobacillus and cecal microbiota in chicken feed efficiency. Sci Rep. 7(1):45308.
- Yang B, Wang Y, Qian PY. 2016. Sensitivity and correlation of hypervariable regions in 16S rRNA genes in phylogenetic analysis. BMC Bioinf. 17(1):135.
- Yu S, Zhang G, Liu Z, Wu P, Yu Z, Wang J. 2020. Repeated inoculation with fresh rumen fluid before or during weaning modulates the microbiota composition and co-occurrence of the rumen and colon of lambs. BMC Microbiol. 20(1): 29. https://doi.org/10.1186/s12866-020-1716-z.
- Zehavi T, Probst M, Mizrahi I. 2018. Insights into culturomics of the rumen microbiome. Front Microbiol. 9:1999.
- Zhang J, Su L, Wang Y, Deng S. 2020. Improved high-throughput sequencing of the human oral microbiome: from illumina to PacBio. Can J Infect Dis Med Microbiol. 2020:6678872.
- Zhang R, Zhou M, Tu Y, Zhang NF, Deng KD, Ma T, Diao QY. 2016. Effect of oral administration of probiotics on growth performance, apparent nutrient digestibility and stress-related indicators in Holstein calves. J Anim Physiol Anim Nutr (Berl). 100(1):33–38.
- Zhou M, Hernandez-Sanabria E, Guan LL. 2009. Assessment of the microbial ecology of ruminal methanogens in cattle with different feed efficiencies. Appl Environ Microbiol. 75(20):6524–6533.